Background
The current health care system has an urgent need for tools to measure quality. A wide range of quality indicators have been developed in an attempt to differentiate between high-quality and low-quality health care processes. However, one of the main issues of currently used indicators is the lack of case-mix correction and improvement possibilities. Case-mix is defined as specific (patient) characteristics that are known to potentially affect (surgical) outcome. If these characteristics are not taken into consideration, comparisons of outcome among health care providers may not be valid.
Objective
The objective of the study was to develop and test a quality assessment tool for laparoscopic hysterectomy, which can serve as a new outcome quality indicator.
Study Design
This is a prospective, international, multicenter implementation study. A web-based application was developed with 3 main goals: (1) to measure the surgeon’s performance using 3 primary outcomes (blood loss, operative time, and complications); (2) to provide immediate individual feedback using cumulative observed-minus-expected graphs; and (3) to detect consistently suboptimal performance after correcting for case-mix characteristics. All gynecologists who perform laparoscopic hysterectomies were requested to register their procedures in the application. A patient safety risk factor checklist was used by the surgeon for reflection. Thereafter a prospective implementation study was performed, and the application was tested using a survey that included the System Usability Scale.
Results
A total of 2066 laparoscopic hysterectomies were registered by 81 gynecologists. Mean operative time was 100 ± 39 minutes, blood loss 127 ± 163 mL, and the complication rate 6.1%. The overall survey response rate was 75%, and the mean System Usability Scale was 76.5 ± 13.6, which indicates that the application was good to excellent. The majority of surgeons reported that the application made them more aware of their performance, the outcomes, and patient safety, and they noted that the application provided motivation for improving future performance.
Conclusion
We report the development and test of a real-time, dynamic, quality assessment tool for measuring individual surgical outcome for laparoscopic hysterectomy. Importantly, this tool provides opportunities for improving surgical performance. Our study provides a foundation for helping clinicians develop evidence-based quality indicators for other surgical procedures.
Click Video under article title in Contents at ajog.org
To ensure that patients receive the highest level of care, the health care system needs reliable tools for assessing quality; indeed, measuring outcome values is an essential principle. A wide range of quality indicators have been developed in an attempt to differentiate between high-quality and low-quality health care processes.
Nearly 2 decades ago, Donabedian defined 3 categories of quality indicators: structure indicators, process indicators, and outcome indicators. Structure indicators reflect the setting in which the care is provided (eg, case volume, access to specific technologies, etc). Process indicators reflect the total care system (eg, multidisciplinary team management).
Finally, outcome indicators reflect direct clinical outcomes and are most commonly used by health care professionals to assess the quality of surgical care. Ideally, an optimum indicator of quality should measure, compare, monitor, and, most importantly, improve the quality of delivered care. Thus, suboptimal performance relative to an established standard can be recognized and, ideally, corrected. Therefore, a quality indicator must be included in an improvement strategy.
In this context, because benchmarking and providing the physician with instant feedback can have positive effects on the quality of surgical care, they are recognized as important areas for improvement. However, developing and selecting quality indicators are complex tasks. The majority of quality indicators have limitations because they usually are not evidence-based, are not easily available, and/or are not suitable for quality improvement.
The principal shortcoming of most currently used outcome indicators is their low applicability due to a lack of case-mix adjustment. In the context of health care, case-mix is defined as specific (patient) characteristics that are known to potentially affect (surgical) outcome. If these characteristics are not taken into consideration, comparisons of outcome among health care providers may not be valid, and patients, clinicians, insurance companies, and government organizations may develop a false sense of security and/or insecurity.
Assessing quality is an indispensable step in ensuring patient safety, particularly with respect to the field of surgery, in which evaluating surgical performance is essential for maintaining high quality. Because emerging surgical technologies are frequently introduced, particularly with respect to minimally invasive surgery, this field is highly prone to factors that compromise patient safety. However, no tested quality assessment tool is currently available to measure individual surgical performance and provide the surgeon with direct feedback while adjusting for case-mix characteristics.
To address this need, we developed and tested an evidence-based quality assessment tool that can serve as an indicator of outcome quality for surgical procedures. For this study, we focused on laparoscopic hysterectomy because it is an advanced and technically complex procedure that is performed relatively frequently.
Materials and Methods
We developed a real-time, web-based quality measurement tool called QUSUM. The primary function of this tool is to measure the surgeon’s performance, provide immediate individual feedback and to detect consistently suboptimal performance after correcting for case-mix characteristics. To assess the general usability of this tool, it was tested by a prospective multicenter study using the System Usability Scale score as reported by Bangor et al. The QUSUM tool was developed in 4 phases.
Phase 1: determination of the benchmark and case-mix characteristics
Operative time, intraoperative blood loss, and complications were selected as primary outcomes. Operative time was defined as the number of minutes between the first incision and insertion of the final stitch and blood loss measured in milliliters directly after the procedure. Complications included infection (local, organ, and/or systemic); injury (vascular, bowel, bladder, and/or ureter); wound dehiscence; hemorrhage (defined as >1000 mL or postoperative bleeding); thromboembolism formation; organ dysfunction (eg, urinary retention or incontinence, ileus, liver or kidney dysfunction, etc); systemic events (eg, medication error, adverse drug reaction. etc); technical complications (eg, failed procedure, corpus alienum, etc); reactive conversion (as defined by Blikkendaal et al ); and other (ie, not specified).
Complications were classified into 4 levels based on severity: level A, recovery without re(operation); level B, reoperation indicated; level C, permanent injury and/or loss of function; and level D, death.
The benchmark data and case-mix characteristics specific to laparoscopic hysterectomies were calculated based on a multicenter prospective cohort study that included 1534 laparoscopic hysterectomy procedures. Using these data, regression models were fitted to the primary outcomes as follows: blood loss (numerical), operative time (numerical), and complication (categorical).
Because blood loss and operative time had severely right-skewed distributions, a gamma regression model with the logarithmic link function was used. The independent variables (case-mix) included the logarithm of uterine weight and body mass index for the outcome blood loss, and the logarithm uterine weight for the outcome operative time. For complications, a multinomial regression model with the cumulative logistic link function was used; the logarithm of uterine weight and the number of previous abdominal surgeries were used as independent variables.
Phase 2: development of the type of individual feedback: outcome-expected cumulative graphs
The regression models were used to compute the expected value of each outcome after correcting for the case-mix variables. The difference between the observed outcome and expected value was calculated (observed outcome – expected value) and the cumulative sum of outcome-expected value was plotted as a time series. A graph depicting the performance of the 3 outcomes over time is shown in Figure 1 . An out-of-control signal was generated when cumulative blood loss exceeded 2000 mL after correcting for the benchmark and case-mix values, and this was defined as consistently suboptimal performance.
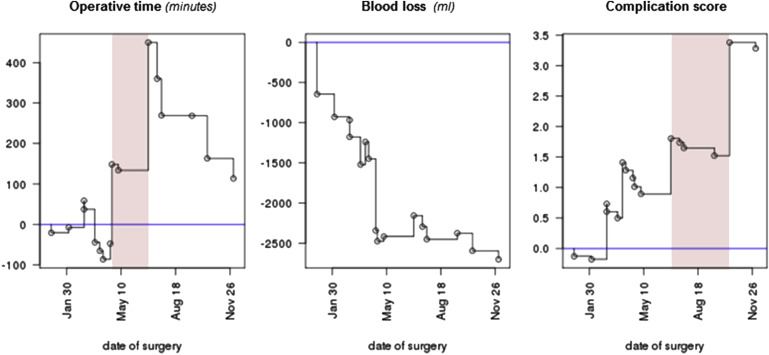
For operative time, this applies for 180 minutes, and for complication score when this value exceeded 2, these cutoff values were calculated based on an out-of-control signal rate of 10-20% of the benchmark data. The complication score was weighted according to the severity: level A was rated as 1 point, whereas levels B, C, and D were each rated as 2 points.
The application instantly calculated updated graphs (including out-of-control signals) when the surgeon entered new data. For example, when a reoperation was needed and this was retrospectively entered in the application, the application instantly updated the graphs (including out-of-control signals). In the event of an out-of-control signal, the surgeon was asked to reflect upon the underperformance by completing a validated patient safety risk factor checklist; each surgeon also had the option to complete this checklist after each procedure ( Table 1 ).
Domain | Risk factors |
---|---|
Surgeon (functioning of the surgeon) | Lack of experience (of surgeon or resident) |
Lack of technical skills (of surgeon or resident) | |
Lack of leadership | |
Surgical team (functioning of the scrub or circulating nurse) | No qualified staffing (eg, student/pupil because of shortage of staff or unqualified staffing) |
Lack of experience of the scrub nurse (concerning this procedure) | |
Lack of knowledge of the procedure of scrub nurse | |
Lack of experience of circulating nurse | |
Technology (availability and functioning of equipment and instruments) | Instrument(s) not present or available |
Instrument(s) do(es) not work properly | |
It is not known how to handle instruments (either surgeon or scrub nurse) | |
Equipment is not present | |
Equipment does not work properly | |
Limited vision (eg, because of condensation and/or smoke) | |
It is not known how to handle equipment (either surgeon or scrub nurse) | |
Social interaction (teamwork and communication) | Poor communication between OR team members (eg, misunderstandings) |
Failure of professional communication (either verbal or nonverbal) | |
Poor collaboration between OR team members | |
Environment (potentially cause distraction or disruptions of the surgical process) | Distractions (eg, telephone calls, case irrelevant conversations, door movements) |
Disruption of the surgical process (surgical process has to be interrupted because of distractions) | |
Too many people in the OR | |
Patient (patient-related risk factors) | Severe adhesions |
Unexpected comorbidity, please specify (eg, unknown bleeding disorder [eg, von Willebrand disease, hemophilia]) | |
Fallibility (factors that influence the fallibility of the surgeon) | Moment of day surgery takes place (eg, during evening or night shifts) |
Perceived high workload | |
Fatigue of the surgeon | |
Safety (compliance or safety protocols) | Poor compliance of briefing procedure |
Poor compliance of debriefing procedure | |
Poor compliance of (surpass) checklist (if applicable) | |
Anesthesiology | Anesthesiology-related problems |
Other | Free text option (please specify) |
Phase 3: development and testing of the application
The following key requirements were used in the development of the QUSUM application: web based, immediate feedback, platform independent, user friendly, and privacy secured. The QUSUM application ( https://www.QUSUM.org ) was developed in collaboration with experts from the Faculty of Social and Behavioral Sciences at Leiden University. The application complied with NEN 7510 standards (Dutch certification regarding informatics and security in the health care field) and was approved by the privacy officer at Leiden University Medical Center. Because the requested patient data were anonymous, this study was exempt for approval by our institutional review board (C14.002).
Phase 4: multicenter implementation
A prospective multicenter study was conducted using the QUSUM application. Gynecologists who performed laparoscopic hysterectomy procedures were requested to register their consecutive laparoscopic hysterectomies performed from April 2014 through November 2015 at our web site ( https://www.QUSUM.org ).
A personal email invitation was send to all Dutch gynecologists performing laparoscopic hysterectomies, and gynecologists were recruited through conferences and meetings. The following patient characteristics were registered: age; body mass index (kilograms per square meter); number of previous abdominal procedures; date the current hysterectomy was performed; type of hysterectomy (total laparoscopic hysterectomy, supracervical laparoscopic hysterectomy, laparoscopic assisted vaginal hysterectomy, or robotic-assisted hysterectomy); uterine weight (grams); and the 3 primary outcomes (blood loss, operative time, and complications). Follow-up for complications lasted up to 6 weeks postoperatively, and gynecologists were automatically reminded to fill in the complication form using an automatic pop-up function.
Participants could register immediately postoperatively or at any given moment after surgery.
Directly after entering the data, a risk-adjusted observed outcome–expected value cumulative graph was generated for each primary outcome ( Figure 1 ). This graph provided the surgeon with immediate individual feedback. A flow chart depicting the entire QUSUM application and data registration is shown in Figure 2 (also see Video , Supplemental Digital Content 1 , which demonstrates and explains the QUSUM application).
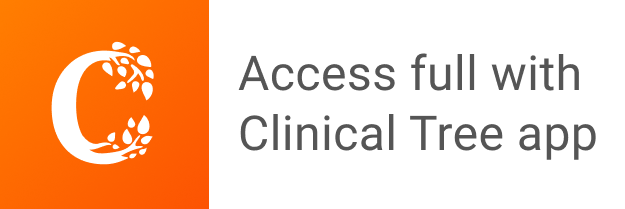