Background
Robotic-assisted gynecologic surgery is common, but requires unique training. A validated assessment tool for evaluating trainees’ robotic surgery skills is Robotic-Objective Structured Assessments of Technical Skills.
Objective
We sought to assess whether crowdsourcing can be used as an alternative to expert surgical evaluators in scoring Robotic-Objective Structured Assessments of Technical Skills.
Study Design
The Robotic Training Network produced the Robotic-Objective Structured Assessments of Technical Skills, which evaluate trainees across 5 dry lab robotic surgical drills. Robotic-Objective Structured Assessments of Technical Skills were previously validated in a study of 105 participants, where dry lab surgical drills were recorded, de-identified, and scored by 3 expert surgeons using the Robotic-Objective Structured Assessments of Technical Skills checklist. Our methods-comparison study uses these previously obtained recordings and expert surgeon scores. Mean scores per participant from each drill were separated into quartiles. Crowdworkers were trained and calibrated on Robotic-Objective Structured Assessments of Technical Skills scoring using a representative recording of a skilled and novice surgeon. Following this, 3 recordings from each scoring quartile for each drill were randomly selected. Crowdworkers evaluated the randomly selected recordings using Robotic-Objective Structured Assessments of Technical Skills. Linear mixed effects models were used to derive mean crowdsourced ratings for each drill. Pearson correlation coefficients were calculated to assess the correlation between crowdsourced and expert surgeons’ ratings.
Results
In all, 448 crowdworkers reviewed videos from 60 dry lab drills, and completed a total of 2517 Robotic-Objective Structured Assessments of Technical Skills assessments within 16 hours. Crowdsourced Robotic-Objective Structured Assessments of Technical Skills ratings were highly correlated with expert surgeon ratings across each of the 5 dry lab drills ( r ranging from 0.75-0.91).
Conclusion
Crowdsourced assessments of recorded dry lab surgical drills using a validated assessment tool are a rapid and suitable alternative to expert surgeon evaluation.
Introduction
Robotic-assisted gynecologic surgery has become a widely used alternative to traditional laparoscopy. Robotic technology provides surgeons with opportunities for altered dexterity and flexibility within the operative field. However, robotic technology requires unique training to achieve proficiency and mastery.
For teaching institutions, it is difficult to know when to incorporate trainees into robotic surgeries. In an effort to develop a standardized method to determine when a trainee can safely operate under supervision from the robotic console, the Robotic Training Network (RTN) was initiated. The RTN was developed by surgical educators to standardize robotic training for residents and fellows. The initial meetings of the network were supported by travel grants from Intuitive Surgical. However, subsequent meetings, curriculum development, and research studies were all independently funded. The RTN produced a validated assessment checklist known as the Robotic-Objective Structured Assessments of Technical Skills (R-OSATS). The R-OSATS was adapted from previously described standardized assessment tools and is designed to be used specifically with 5 dry lab robotic surgical drills: (1) tower transfer; (2) roller coaster; (3) big dipper; (4) train tracks; and (5) figure-of-8. The first drill, “tower transfer,” requires the trainee to transfer rubber bands between towers of varying heights. The “roller coaster” drill involves moving a rubber band around a series of continuous wire loops. “Big dipper” is a drill requiring the participant to drive a needle through a sponge in specific directions. “Train tracks” is a drill simulating a running suture. The “figure-of-8” drill consists of throwing a needle in a figure-of-8 conformation and then tying a square surgical knot. Within R-OSATS, each drill is scored using a 5-point Likert scale based on the following metrics: (a) depth perception/accuracy of movements; (b) force/tissue handling; (c) dexterity; and (d) efficiency of movements. The maximum R-OSATS score is 20 points per drill.
Currently, R-OSATS are completed by expert surgeons directly observing trainee performance on robotic simulation drills. Although this is a reliable method of assessment, it requires at least 30 minutes of the evaluator’s time per trainee. This is in addition to any setup and practice time that the trainee uses prior to the formal assessment. Given that expert evaluators are also surgeons with busy clinical practices, this time requirement creates a limitation to any objective structured training and assessment process. Thus, we sought to assess whether we could use crowdworkers in place of expert surgeons to assess dry lab surgical skill drills.
Crowdsourcing is the process of obtaining work or ideas from a large group of people. The people who compose the group are known as crowdworkers. Crowdworkers come from the general public and, in the case of this study, they do not necessarily have any prior medical experience or training. A crowdworker may be from anywhere in the world. Typically, crowdsourcing occurs through an online forum or marketplace. Amazon.com Mechanical Turk is one such Internet-based crowdsourcing marketplace where an entity can post tasks for crowdworkers to complete. Through the marketplace, crowdworkers are able to view posted tasks and choose which tasks they are interested in completing. Since crowdworkers are not required to have experience prior to beginning a task, they receive specific training for the tasks they choose to complete. This training is a part of the posted task and is created by the entity requesting the task. After completing a task, crowdworkers receive financial compensation. Essentially, crowdworkers replace traditional employees and they can be solicited by anyone. Crowdsourcing is inexpensive, fast, flexible, and scalable, although studies evaluating the utility of crowdsourcing for assessing complex technical skills are limited.
Crowdsourcing is being explored in several ways within medicine. It has been used in ophthalmology to screen retinal images for evidence of diabetic retinopathy and evaluate optic disk images for changes associated with glaucoma. Pathologists have used crowdsourcing to quantify malarial parasites on blood smears and assess positivity on immunohistochemistry stains. Crowdsourcing has been proposed as a means of evaluating surgical skill, although these assessments are more complex than still images and thus require validation.
We hypothesized that when using a valid and reliable assessment tool, such as R-OSATS, to evaluate trainees performing dry lab drills, crowdworker and expert surgeon scores would be similar. Thus, our primary objective was to assess the degree of correlation between R-OSATS scores ascertained by crowdworkers vs expert surgeons.
Materials and Methods
This is a methods-comparison study comparing 2 methods of assessment for dry lab surgical drills. As a part of the prior R-OSATS validation study, 105 obstetrics and gynecology, urology, and general surgery resident, fellow, and expert robotic surgeons performed the 5 robotic dry lab drills: (1) tower transfer; (2) roller coaster; (3) big dipper; (4) train tracks; and (5) figure-of-8. These drills were recorded, de-identified, uploaded to a private World Wide Web–based location, and scored by 3 separate expert surgeons. Again, each drill was scored on: (a) depth perception/accuracy of movements; (b) force/tissue handling; (c) dexterity; and (d) efficiency of movements using a 5-point Likert scale for a maximum of 20 points per drill.
For the current methods-comparison study, we utilized these previously recorded videos with their accompanying expert surgeon evaluator scores. The expert surgeons had extensive robotic surgery backgrounds having completed a median of 108 robotic procedures (range 50-500 per surgeon). Furthermore, they were active resident and/or fellow robotic surgery educators.
After obtaining institutional review board approval, we reviewed the previously obtained R-OSATS scores. Since each drill had been viewed and scored by 3 expert surgeons, we calculated the mean expert R-OSATS scores, per video, for each drill. We used the mean expert R-OSATS score to separate the recordings of each dry lab drill into quartiles.
To ensure high-quality responses from crowdworkers, we used techniques previously described by Chen et al to select and train crowdworkers via Amazon.com Mechanical Turk. Only crowdworkers with an acceptance rating >95% from previous assignments on Amazon.com Mechanical Turk were able to sign up to evaluate our recordings. To assess crowdworkers’ discriminative ability, we used a screening test that required the crowdworkers to watch short side-by-side videos of 2 surgeons performing a robotic dry lab drill and identify which video showed the surgeon of higher skill. Separate training videos were used for each of the 5 dry lab drills and participants had to complete specific training videos for each task that they chose to complete. Additionally, to ensure that the crowdworkers were actively engaged in the scoring process, an attention question was embedded within the survey that directed the crowdworker to leave a particular question unanswered. If a crowdworker failed either the screening or attention questions, this crowdworker’s responses were excluded from further analyses. It should be noted that in the selection process, crowdworker education level, prior training, or past work experiences were not taken into consideration. After passing the selection process, 1 representative recording of a skilled surgeon and 1 representative recording of a novice surgeon, performing the dry lab drill the crowdworker was going to assess, was shown to the crowdworkers to train them on R-OSATS scoring. This was accomplished through a virtual online training suite (C-SATS Inc, Seattle, WA).
To compare crowdworker R-OSATS scores to expert evaluators, we recognized that we needed to provide videos that spanned a wide range of skill levels for each drill. Three unique video recordings of dry lab surgical drills were randomly selected from each scoring quartile, for a total of 12 videos per drill. As a result, crowdworkers evaluated videos that covered the entire skill spectrum. This was repeated for each of the 5 dry lab drills, providing a total of 60 unique recordings available for evaluation. Each video recording was posted on Amazon.com Mechanical Turk for crowdworkers to view and assess. The posted recordings included the appropriate training videos as described above since the crowdworkers did not necessarily have any formal medical education or prior experiences assessing surgical skills. Crowdworkers evaluated ≥1 of the 60 recordings, based on their desire, using R-OSATS.
Expert evaluator interrater reliability was calculated using intraclass correlation coefficients for each of the 5 drills. Linear mixed effects models were used to derive average crowd ratings for each drill. Pearson correlation coefficients were constructed to assess the correlation between the crowdsourced and expert R-OSATS scores. Two-sided tests with alpha = 0.05 were used to declare statistical significance.
Using estimates from prior crowdsourcing studies, we estimated needing at least 30 successfully trained crowdworkers to evaluate each recording to derive average R-OSATS scores per video with 95% confidence intervals of ±1 point. In a secondary analysis aimed at determining the minimum number of crowdworker ratings needed to maintain high correlation with expert scores, we used bootstrapping to sample data sets of varying numbers of crowd ratings per video and reassessed the correlation with expert ratings. Bootstrapping is a technique that generates multiple random samples (with replacement) from the original data set and allows one to estimate specific statistical parameters of interest. All statistical analyses were conducted using R 3.1.1 (R Foundation for Statistical Computing, Vienna, Austria).
Materials and Methods
This is a methods-comparison study comparing 2 methods of assessment for dry lab surgical drills. As a part of the prior R-OSATS validation study, 105 obstetrics and gynecology, urology, and general surgery resident, fellow, and expert robotic surgeons performed the 5 robotic dry lab drills: (1) tower transfer; (2) roller coaster; (3) big dipper; (4) train tracks; and (5) figure-of-8. These drills were recorded, de-identified, uploaded to a private World Wide Web–based location, and scored by 3 separate expert surgeons. Again, each drill was scored on: (a) depth perception/accuracy of movements; (b) force/tissue handling; (c) dexterity; and (d) efficiency of movements using a 5-point Likert scale for a maximum of 20 points per drill.
For the current methods-comparison study, we utilized these previously recorded videos with their accompanying expert surgeon evaluator scores. The expert surgeons had extensive robotic surgery backgrounds having completed a median of 108 robotic procedures (range 50-500 per surgeon). Furthermore, they were active resident and/or fellow robotic surgery educators.
After obtaining institutional review board approval, we reviewed the previously obtained R-OSATS scores. Since each drill had been viewed and scored by 3 expert surgeons, we calculated the mean expert R-OSATS scores, per video, for each drill. We used the mean expert R-OSATS score to separate the recordings of each dry lab drill into quartiles.
To ensure high-quality responses from crowdworkers, we used techniques previously described by Chen et al to select and train crowdworkers via Amazon.com Mechanical Turk. Only crowdworkers with an acceptance rating >95% from previous assignments on Amazon.com Mechanical Turk were able to sign up to evaluate our recordings. To assess crowdworkers’ discriminative ability, we used a screening test that required the crowdworkers to watch short side-by-side videos of 2 surgeons performing a robotic dry lab drill and identify which video showed the surgeon of higher skill. Separate training videos were used for each of the 5 dry lab drills and participants had to complete specific training videos for each task that they chose to complete. Additionally, to ensure that the crowdworkers were actively engaged in the scoring process, an attention question was embedded within the survey that directed the crowdworker to leave a particular question unanswered. If a crowdworker failed either the screening or attention questions, this crowdworker’s responses were excluded from further analyses. It should be noted that in the selection process, crowdworker education level, prior training, or past work experiences were not taken into consideration. After passing the selection process, 1 representative recording of a skilled surgeon and 1 representative recording of a novice surgeon, performing the dry lab drill the crowdworker was going to assess, was shown to the crowdworkers to train them on R-OSATS scoring. This was accomplished through a virtual online training suite (C-SATS Inc, Seattle, WA).
To compare crowdworker R-OSATS scores to expert evaluators, we recognized that we needed to provide videos that spanned a wide range of skill levels for each drill. Three unique video recordings of dry lab surgical drills were randomly selected from each scoring quartile, for a total of 12 videos per drill. As a result, crowdworkers evaluated videos that covered the entire skill spectrum. This was repeated for each of the 5 dry lab drills, providing a total of 60 unique recordings available for evaluation. Each video recording was posted on Amazon.com Mechanical Turk for crowdworkers to view and assess. The posted recordings included the appropriate training videos as described above since the crowdworkers did not necessarily have any formal medical education or prior experiences assessing surgical skills. Crowdworkers evaluated ≥1 of the 60 recordings, based on their desire, using R-OSATS.
Expert evaluator interrater reliability was calculated using intraclass correlation coefficients for each of the 5 drills. Linear mixed effects models were used to derive average crowd ratings for each drill. Pearson correlation coefficients were constructed to assess the correlation between the crowdsourced and expert R-OSATS scores. Two-sided tests with alpha = 0.05 were used to declare statistical significance.
Using estimates from prior crowdsourcing studies, we estimated needing at least 30 successfully trained crowdworkers to evaluate each recording to derive average R-OSATS scores per video with 95% confidence intervals of ±1 point. In a secondary analysis aimed at determining the minimum number of crowdworker ratings needed to maintain high correlation with expert scores, we used bootstrapping to sample data sets of varying numbers of crowd ratings per video and reassessed the correlation with expert ratings. Bootstrapping is a technique that generates multiple random samples (with replacement) from the original data set and allows one to estimate specific statistical parameters of interest. All statistical analyses were conducted using R 3.1.1 (R Foundation for Statistical Computing, Vienna, Austria).
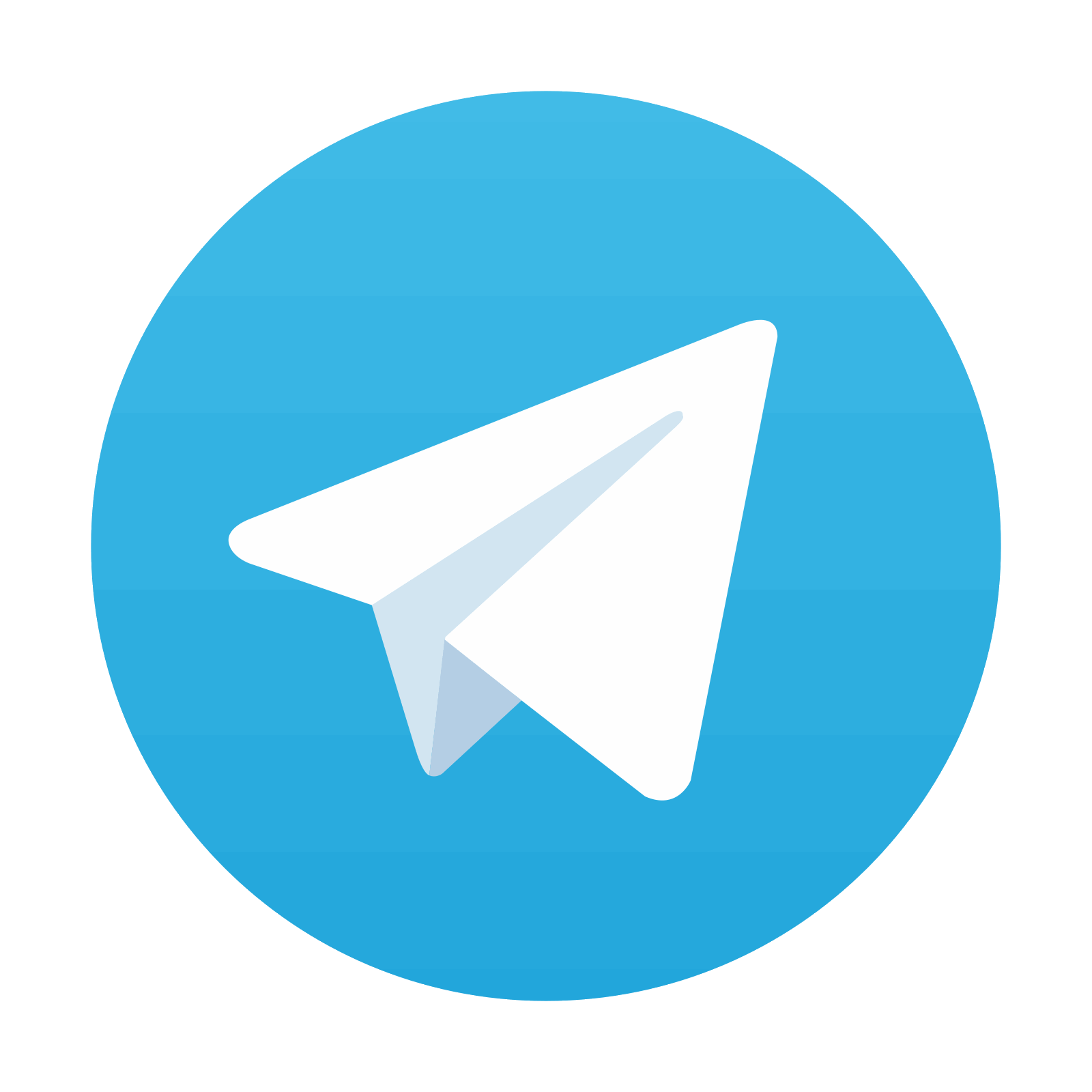
Stay updated, free articles. Join our Telegram channel

Full access? Get Clinical Tree
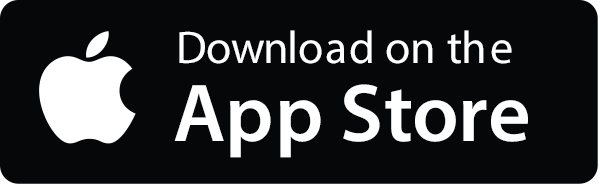
