Key Points
- •
Screening is the identification of unrecognised disease or defect found by testing an asymptomatic population.
- •
Prenatal screening detects conditions that are deleterious to the mother, fetus or both.
- •
Prenatal screening allows for diagnostic testing and subsequent pregnancy options, including termination of the pregnancy, preparation for the birth of a child with chronic or fatal illness or the use of advanced reproductive technology to avoid carrying a fetus with the disease in question.
- •
The validity of a screening test is described by its sensitivity, specificity, and positive and negative predictive values.
- •
Likelihood ratios allow the calculation of posttest odds based on pretest odds and test results.
- •
To set cutoffs for tests with continuous results, a receiver operator characteristic curve can be used.
- •
Pursuing multiple tests in sequence raises specificity while sacrificing sensitivity; conversely testing in parallel improves sensitivity at the expense of specificity.
- •
An effective screening test must have excellent specificity and sensitivity, must be acceptable to the population, must screen for a prevalent and clinically important disease, must offer potential for diagnostic testing and intervention in the natural course of the disease and must be cost effective.
- •
Harms of screening include psychological distress and false-positive results as well as harms resulting from subsequent diagnostic testing.
- •
Patients often do not fully understand the testing being offered to them.
Definition and Brief History
Screening was first formally defined in 1951 by the United States Commission of Chronic Illness as:
. . . the presumptive identification of unrecognised disease or defect by the application of tests, examinations, or other procedures which can be applied rapidly. Screening tests sort out apparently well persons who probably have a disease from those who probably do not. A screening test is not intended to be diagnostic. Persons with positive or suspicious findings must be referred to their physicians for diagnosis and necessary treatment.
Put simply, the purpose of screening is to identify patients at high risk for a specific condition within a group of apparently healthy and asymptomatic people. Prenatal screening should be differentiated from prenatal diagnosis, in which a definitive diagnosis is made.
Prenatal diagnosis first became available in the 1960s with the introduction of amniocentesis for Down syndrome. At that time, the only screen was maternal age; patients with advanced maternal age were offered amniocentesis as a diagnostic test. In the 1970s, the first maternal serum screen became available with the discovery of differences in maternal serum alpha-fetoprotein (AFP) with neural tube defects. In 1984, associations between reduced serum AFP and Down syndrome were recognised, and screening with this test was introduced shortly thereafter. Since then, the field has progressed rapidly to include advanced ultrasound and noninvasive prenatal testing (NIPT) using cell-free fetal DNA.
Goal and Scope of Prenatal Screening
The goal of prenatal screening is to detect conditions that can be deleterious to the mother, fetus or both. Prenatal screening includes both maternal (and occasionally paternal) and fetal screening. In the course of routine prenatal care, mothers are screened for a number of conditions such as sexually transmitted diseases and gestational diabetes that can affect both the mother and fetus. Patients can be screened for carrying genetic diseases such as cystic fibrosis, haemoglobin S trait and Tay Sachs disease. Based on these results, further testing such as invasive fetal testing or paternal genetic testing can be recommended. Finally, fetal screening focuses on screening the fetus for conditions such as aneuploidy or congenital defects, and this can be accomplished either through maternal blood testing or fetal ultrasound. The results of prenatal screening and subsequent diagnostic testing may be used to make a decision to terminate a pregnancy, to prepare for the birth of a child with chronic or fatal illness or to use advanced reproductive technology to avoid carrying a fetus with the disease in question in a subsequent pregnancy. The purpose of this chapter is to explore the basic principles underlying all of these screening tests.
Basic Parameters of Diagnostic and Screening Tests
To be useful, a screening test must be valid . The validity of a screening test is defined as its ability to distinguish between those who have a disease and those who do not. This is further broken down into sensitivity and specificity . Sensitivity is the ability of a test to correctly identify those who have a disease. Specificity is the ability of the test to identify those who do not have the disease in question. Accuracy is another important aspect of a test and refers to the ‘closeness of the measured value to the correct value’.
Imagine a population of 1000 gravidas. One hundred of them are carrying a fetus with Down syndrome, and the rest are not. Table 16.1 illustrates the four possibilities of a screening test in this population. Sensitivity is defined as True positives/(True positives + False negatives). Specificity is defined as True negatives/(True negatives + False positives). If we put numbers into our table ( Table 16.2 ), we can calculate the sensitivity and specificity of the screening test. In this case, the sensitivity is 70/(70 + 30) = 70%, and the specificity is 800/(800 + 100) = 88.9%.
Has Down Syndrome | Does Not Nave Down Syndrome | |
---|---|---|
Test positive | True positive | False positive |
Test negative | False negative | True negative |
Has Down Syndrome ( n = 100) | Does Not Nave Down Syndrome ( n = 900) | |
---|---|---|
Test positive | 70 | 100 |
Test negative | 30 | 800 |
Predictive Values
The concepts of sensitivity and specificity are properties of the test itself. The real clinical question is: ‘In this patient with a positive test result, what is the chance that her fetus really has Down syndrome’? To answer this question, we must look at the test’s positive predictive value (PPV) and negative predictive value (NPV).
Going back to Table 16.1 , PPV is the chance that a patient with a positive test result really has the disease in question: (True positive)/(True positive + False positive), which in this case is 70/(70 + 100) = 41%. The NPV is the chance that a patient with a negative test result truly does not have the disease, or (True Negative)/(True Negative + False Negative) which in our example is 800/(800+30) = 96%.
Unlike sensitivity and specificity, which are test characteristics without any relationship to the population under study, the positive and NPVs depend on the prevalence of the disease in a population. In a rare disease, the specificity also greatly impacts the PPV and NPV. Table 16.3 shows the same specificity and sensitivity as shown in Table 16.2 but now in a population with a prevalence of Down syndrome of only 5%. The sensitivity and specificity are unchanged at 70% and 88.9%, respectively; however, the PPV is now only 25%, and the NPV is now 98%.
Has Down Syndrome ( n = 50) | Does Not Nave Down Syndrome ( n = 950) | |
---|---|---|
Test positive | 35 | 105 |
Test negative | 15 | 845 |
A general rule of thumb regarding the relationship between prevalence and predictive values is the following: given the same sensitivity and specificity, as the prevalence rises, the PPV increases, and the NPV decreases. Likewise, given the same sensitivity and specificity, as the prevalence of disease decreases, the PPV decreases, and the NPV increases. Therefore an important principle of screening is that the condition in question must have a reasonably high prevalence so that the tests will have acceptable PPV and NPV. This concept can be difficult for both patients and clinicians to grasp.
A recent illustration of this principle is in regards to NIPT with cell-free fetal DNA. Although the sensitivity and specificity of the various commercially available tests are high, the PPV is quite different depending upon the woman’s basic risk level, particularly in regards to age. For example, the sensitivity and specificity for detecting Down syndrome are greater than 99%, but in a 25-year-old woman (in whom the prevalence of Down syndrome is 0.7–0.9/1000), the PPV is 33%, but in a 40-year old woman (baseline prevalence of Down syndrome, 8.5–13.7/1000), the PPV is 87%. Because of this, there has been hesitancy on the part of perinatologists to extend NIPT to ‘low-risk’ women.
There is one particularly interesting aspect to the history of prenatal screening and diagnosis that deserves mention. Specifically, in the history of research on prenatal screening and diagnosis, new tests are usually evaluated in ‘high-risk’ populations first. When acceptable sensitivities and specificities are seen in these initial high-risk population studies, there is a call from the prenatal diagnosis community for additional studies in low-risk populations to assess the validity of the screening test in a lower risk population before implementation in the general population. This line of thought is incorrect. As was mentioned previously, sensitivity and specificity are characteristics of the test itself, and those characteristics do not change in a different population. The predictive values will change, however, as the prevalence changes. Thus the constant call for validation of new tests in low-risk populations is improper because the sensitivity and specificity will obviously be the same regardless of the population tested. This has been borne out most recently in studies of NIPT, in which, as expected, the sensitivity and specificity were the same in low- and high-risk studies. This flawed thought process has likely led to a significant delay in offering new screening tests to the general population. When considering extending a new screening test to a ‘low-risk’ population, the question should not be, ‘Will this test be valid in the low-risk population?’ but rather, ‘What are the costs and benefits of using this test given that the PPV will inherently be very low?’
Likelihood Ratios
A different method of analysing a screening test is the concept of likelihood ratio (LR) . A LR is the ‘ratio of the likelihood of that result in someone with the disease to the likelihood of that result in someone without the disease’. In other words, the LR represents the probability of having a certain test result in a patient with disease divided by the probability of having that ratio in someone without the disease.
A positive LR (LR + ) is the ratio of the proportion of diseased individuals who test positive (sensitivity) divided by the proportion of nondiseased people who test positive (1 – specificity). The negative LR (LR – ) is the ratio of the proportion of diseased people who test negative (1 – sensitivity) divided by the proportion of nondiseased people who test negative (specificity). To use our example from Table 16.2 , the LR + is (0.7/[1 – 0.889]) = 6.3. The LR – is ([1 – 0.7]/0.889) = 0.33. The higher the LR + and the lower the LR – , the better the test. We can express the LR + in words by saying that a positive test result is about six times more likely to be found in a patient whose fetus has Down syndrome than in a patient whose fetus does not.
One advantage of LRs is that they can be applied to individual patients to determine the likelihood of that specific patient having the disease in question. To do this, the LR is multiplied by the pretest odds of the patient having the disease; this calculation yields the posttest odds of the patient having the disease. Thus if the pretest odds are high and the LR + is high, the posttest odds will be higher still, which is another way of saying that if the disease is prevalent in a certain population, the PPV is higher than if the disease is less prevalent, as discussed earlier.
We can illustrate the practical use of the LR with our Down syndrome example. The posttest odds of the fetus having Down syndrome will be equal to (pretest odds × LR + ). We calculated the LR + above as 6.3. Odds are defined as (probability/[1 – probability]). In our case, assume a pretest probability of Down syndrome of 10%, which was the prevalence of the syndrome in our fictitious population. Therefore the pretest odds are (0.1/0.9) = 0.11. Thus our posttest odds are 0.11 × 6.3 = 0.693. We can covert the posttest odds back into a probability using the formula (probability = odds/[1 + odds]), which in our case is ( P = .693/1.693) = 0.41. We can explain this to a patient by saying that before the test there was a 10% chance of carrying a fetus with Down syndrome; now that we have a positive result, the chance is 41%. However, one of the benefits of LRs is that they can be applied sequentially. If we took this example further, suppose the patient now underwent a second, perhaps more invasive, screening test with an LR + of 10 and had a positive result. In this situation, the pretest odds for her were 0.693, so the posttests odds are now 0.693 × 10 = 6.93, which corresponds to a probability of 0.87 or 87%. This sequential testing is the basis of the genetic sonogram in which the baseline pretest odds (usually based on age) can be multiplied by the LRs for any positive findings to compute a posttest odds. However, it is important to also account for negative findings by using the LR – as well. In our earlier example, assume that the second test has a LR – of 0.3 and that the patient has a negative result on this test. Therefore her posttest odds will be 0.693 × 0.3 = 0.21 for a posttest probability of 0.17 or 17%. It is important to note that such sequential use of the LR assumes independence of each test, which may not be a valid assumption depending on the circumstances. Although the LR on its own can be a difficult concept for a patient to grasp, applying the LR clinically can help personalise results for an individual patient.
Receiver Operator Characteristic Curves
The previous discussion is predicated on having a test that gives a binary response (test positive or test negative). However, the results of most tests are not binary but rather are continuous, and therefore the test designers must decide the appropriate cut points to define positive and negative results. The placement of these cut points will greatly influence the test performance, specifically the sensitivity and specificity of the test.
A classic example of cut points in prenatal care is testing for gestational diabetes. The usual approach in the United States is a screening test with a serum glucose level obtained 1 hour after the patient drinks a 50-g glucose load (glucose challenge test (GCT)). Various studies have attempted to determine the optimal cut point to differentiate between those who screen positive and require a confirmatory diagnostic glucose tolerance test versus those who screen negative. In a recent paper, Rebarber and colleagues investigated the sensitivity and specificity of a cutoff of 130 mg/dL, 135 mg/dL or 140 mg/dL in diagnosing patients with GDM in twin gestations and found that a GCT cutoff of 135 mg/dL or greater was 100% sensitive and 76.4% specific for gestational diabetes, and a cutoff of 130 mg/dL or greater was still 100% sensitive but only 69.8% specific. On the other hand, a cutoff of 140 mg/dL or greater was only 93.5% sensitive, but it was 81.5% specific. As this illustrates, the assignment of a cutoff in any continuous scale determines the sensitivity and specificity of the test. Furthermore, there is usually a tradeoff between the two values: a higher specificity is coupled with a lower sensitivity.
To maximise sensitivity and specificity as much as possible, the designers of a test may use a receiver operator characteristic (ROC) curve to find the ideal cutoff for a diagnostic test. To do this, the sensitivity for multiple cut points is plotted on the y-axis of a graph as a function of 1 – specificity on the x-axis. An example of an ROC curve is shown in Fig. 16.1 . A number of points can be made about the figure. First, as described previously, the y-axis is the sensitivity, and the x-axis is 1 – specificity. The ideal diagnostic test maximises sensitivity and specificity and is represented by the upper left corner, where the sensitivity and specificity are both 100%. The dashed diagonal line represents a ‘useless’ diagnostic test because any gain in specificity is directly lost in sensitivity; for example, where sensitivity is 100%, specificity is 0. Another way to understand this ‘useless’ line is that it represents a test with 50% sensitivity and 50% specificity, which is no better than flipping a coin.
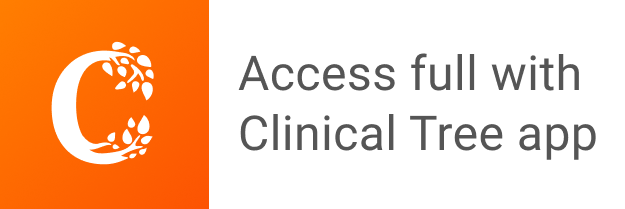