Objective
The purpose of this study was to determine whether nuclear magnetic resonance–based metabolomic markers in first-trimester maternal serum can detect fetuses with trisomy 18.
Study Design
This was a study of pregnancies between 11 weeks and 13 weeks 6 days’ gestation. We analyzed 30 cases of trisomy 18 and a total of 114 euploid cases. Nuclear magnetic resonance–based metabolomic analysis was performed. A further analysis was performed that compared 30 cases with trisomy 18 and 30 trisomy 21 (T21) cases.
Results
Metabolomic markers were sensitive for trisomy 18 detection. A combination of 2-hydroxybutyrate, glycerol and maternal age had a 73.3% sensitivity and 96.6% specificity for trisomy 18 detection, with an area under the receiver operating curve: 0.92 ( P < .001). Other metabolite markers, which include trimethylamine, were sensitive for distinguishing trisomy 18 from T21 cases.
Conclusion
This is the first report of prenatal trisomy 18 detection that has been based on metabolomic analysis. Preliminary results suggest that such markers are sensitive not only for the detection of fetal trisomy 18 but also for distinguishing this aneuploidy from T21.
Metabolomics involves the comprehensive cataloging and characterization of the small molecules that are found in cells, organs, and tissues. Metabolomics has allowed scientists to develop an increasingly detailed picture of the biochemical activity of cells in both their normal and diseased states. The impact of external influences (eg, toxins, medications, and environmental changes) can be mapped based on metabolic profiles or metabolic signatures that have been measured by modern metabolomic techniques. Advances in instrumentation and improvements to bioinformatic tools are now permitting very extensive analysis of human biofluids with the use of these techniques.
Metabolomics has shown significant promise for the discovery of new biomarkers for the detection of a number of complex clinical disorders. However, the use of metabolomics in obstetrics is a relatively new phenomenon. Nevertheless, a number of recent studies have shown its value in the prediction of or detection of biomarkers for preeclampsia and preterm labor. More recently, we identified a novel set of maternal serum metabolomic markers for the first-trimester detection of fetuses with Down syndrome. Trisomy 18 is the second most common aneuploidy after Down syndrome. Maternal first-trimester screening for trisomy 18 detection has been an area of research interest for many years and the standard of clinical care for more than a decade.
Our objectives were, first, to determine whether maternal first-trimester serum from trisomy 18 pregnancies had a qualitatively or quantitatively distinct metabolomic profile relative to normal pregnancies. Second, we wanted to evaluate the sensitivity and specificity of serum metabolite markers for the detection of trisomy 18. Finally, we wanted to compare trisomy 18 with previously reported cases of Down syndrome to determine whether these 2 aneuploidies could be distinguished with the use of first-trimester maternal serum metabolomic analysis.
Materials and Methods
Patients and specimen samples were obtained as part of an ongoing prospective study by the Fetal Medicine Foundation in London, England. The study was approved by the institutional review board of King’s College Hospital, London, England. In this study, an average risk population of British women were screened and recruited prospectively from 2003-2009. Written consent was obtained from all participating women. The details of the study population, consent approval by institutional authorities, specimen collection, storage, transportation, and metabolomic analysis have been described in a previous publication. Briefly, maternal demographic data, sonographic measurements, and karyotype data were entered prospectively in a database. Nuchal translucency measurement was performed; pregnancy-associated plasma protein–A and free-β human chorionic gonadotropin measured as a part of routine clinical screening for aneuploidy. Delta nuchal translucency (ΔNT) values or the difference between the observed NT measurement and the median expected value based on the crown rump length (CRL) were calculated. Maternal venous blood was collected in plain vacutainer tubes (Becton Dickinson UK Limited, Oxfordshire, UK), processed within 15 minutes of blood collection, and centrifuged at 3000 rpm for 10 minutes to separate serum from packed cells. The serum that was obtained was separated into 0.5-mL aliquots and stored at –80°C until subsequent analysis. None of the samples had been thawed and refrozen previously. The serum samples were transported by air courier to the laboratory in Alberta, Canada, for metabolomic testing; the samples were packed in dry ice and maintained in a frozen state at all times. Case and control specimens for the primary study were collected within 1 week of each other. The cases with trisomy 18 had a fetal karyotype confirmed based on chorionic villus sampling; control subjects were selected from women who delivered live born neonates at full term with a normal karyotype or a normal phenotypic examination.
The study was limited to 11-13 weeks 6 days’ gestation. An important advantage of metabolomic analysis is that a relatively large number of markers can be identified simultaneously that leads to the development of several different predictive algorithms. We looked at metabolomics-only algorithms and algorithms that combined metabolites with maternal demographic and other markers. In the primary metabolomic analysis, we conducted a case-controlled study of 30 cases with trisomy 18 and 60 matched euploid control specimens. Subsequently, to increase the study power and to facilitate the performance logistic regression analyses with an expanded number of predictive variables, an additional set of 54 euploid control specimens from a previously reported trisomy 21 study was also added for further analyses. Only those measured metabolites that were common to each of the trisomy 18 and expanded control cases were considered for this particular analysis. In addition, the metabolomic analysis was limited to cases in which all demographic and sonographic measurements were available. This resulted in a total of 30 cases with trisomy 18 and 114 normal control subjects. For the sake of uniformity, the 30 cases with trisomy 18 and combined 114 control subjects were also used for TheGmax analyses (version 11.9.23; Available at: www.thegmax.com . Accessed April 30, 2013) so that the results of standard logistic regression and TheGmax analyses could be compared. Specimen collection, processing, and metabolomic analysis for the 54 control subjects who were used in the trisomy 21 study were performed with the same standards and were conducted at the same time.
Metabolomic data collection was performed with the Varian Inova 500 MHz NMR (nuclear magnetic resonance) spectrometer (International Equipment Trading Ltd, Vernon Hills, IL) as previously described. Plasma samples were filtered through 3-kd cutoff centrifugal filter units (Amicon Microcon YM-3; Sigma-Aldrich, St. Louis, MO) to remove plasma proteins. Aliquots (350 μL) of each plasma sample were transferred into the centrifuge filter devices and spun (10,000 rpm for 20 minutes) to remove macromolecules (primarily protein and lipoproteins) from the sample. If the total volume of the sample was <300 μL, an appropriate amount from a 50-mmol NaH 2 PO 4 buffer (pH 7) was added until the total volume of the sample was 300 μL. Any sample that had to have buffer added to bring the solution volume to 300 μL was annotated with the dilution factor, and metabolite concentrations were corrected in the subsequent analysis. Subsequently, 35 μL of D 2 O and 15 μL of a standard buffer solution (11.667 mmol disodium-2, 2-dimethyl-2-silcepentane-5-sulphonate, 730 mmol imidazole, and 0.47% NaN 3 in H 2 O) was added to the sample.
The plasma sample (350 μL) was then transferred to a standard microcell NMR tube (Shigemi, Inc, Allison Park, PA) for subsequent spectral analysis. All 1 H-NMR spectra were collected on a 500-MHz Inova (Varian Inc, Palo Alto, CA) spectrometer equipped with a 5-mm ITCN Z-gradient PFG cold-probe. The singlet produced by the disodium-2, 2-dimethyl-2-silcepentane-5-sulphonate methyl groups was used as an internal standard for chemical shift referencing (set to 0 ppm) and for quantification. All 1 H-NMR spectra were processed and analyzed with the Chenomx NMR Suite Professional Software package (version 7.1; Chenomx Inc, Edmonton, Alberta, Canada) that allows for qualitative and quantitative analysis of an NMR spectrum by manually fitting spectral signatures from an internal database to the observed spectrum. Specifically, the spectral fitting for all detected metabolites was done with the use of the standard Chenomx 500 MHz metabolite library. Typically, 90% of the visible peaks were assigned to a compound, and >90% of the spectral area could be fit routinely with the use of the Chenomx spectral analysis software. It has been shown previously that this fitting procedure provides absolute concentration accuracy of ≥90%. Each spectrum was processed and analyzed by at least 2 NMR spectroscopists to minimize compound misidentification and misquantification. Metabolomic data analysis was performed with MetaboAnalyst, which is a web-based server that supports a wide range of univariate and multivariate statistical techniques. Log normalization of the metabolomic data was performed before further analysis. Principal component analysis (PCA) is a multivariate statistical technique that we used to identify combinations of metabolites (principal components) that best distinguish trisomy 18 from euploid cases. On the PCA plot, the first principal component, which is the most important discriminator, is represented on the x-axis; the second and third principal components, in order of discriminating power, are plotted on the y- and z-axis in the 2- and 3-dimensional PCA plots, respectively.
Partial least squares discriminant analysis (PLS-DA) further rotates the principal components that are identified on the PCA plot to find the combination of metabolites that optimally distinguishes cases from control subjects. Permutation testing was performed with random relabeling of the metabolomic data and the rerunning of the PLS-DA to minimize the probability that any observed separation between the 2 groups could be due to chance. A total of 2000 repeated relabelings were performed; the probability value that corresponded to the probability that the observed group separation was due to chance was determined. MetaboAnalyst was used for both PCA and PLS-DA. A variable importance in projection (VIP) plot, which indicates the contribution of individual metabolites in the separation of cases from control subjects was also constructed.
Finally, we determined whether serum metabolite markers were able to distinguish pregnancies with trisomy 18 from pregnancies with trisomy 21. PCA and PLS-DA were performed with the use of the metabolomic data for 30 cases with trisomy 18 and for the 30 maternal serum specimens with trisomy 21 that had been reported in a previous study. As noted previously, the specimens for trisomy 18 and 21 were collected during the same period from the same patient population and underwent metabolomic analysis at approximately the same time in the same laboratory. A VIP curve was also generated to determine the metabolites that best distinguished pregnancy specimens with trisomy 18 from specimens with trisomy 21.
The means and standard deviations of all measured serum metabolite concentrations were compared between the trisomy 18 and control groups. Using logistic regression, we determined the individual maternal risk of having a pregnancy with trisomy 18 based on metabolites, demographics, and ultrasound measurements. Receiver operator characteristic curves along with areas under the receiver operator characteristic curve (AUC) were determined. The sensitivity and specificity of several different algorithms for trisomy 18 detection were also calculated. In all cases, a probability value of < .05 was considered statistically significant.
Algorithms for trisomy 18 prediction were also generated with the use of genetic programming along with their corresponding sensitivity, specificity, AUC, and probability values. Genetic programming mimics the principle of evolutionary genetics (ie, selection, recombination, and mutation) to develop rules for the optimal prediction of an outcome of interest with the use of multiple and diverse prediction variables. The principles of genetic programming have been summarized in some of our previous publications. Genetic programming analysis is a robust technique and is not limited by a lack of normality (ie, a Gaussian distribution) in the data, by sample size, or by missing data and is used currently in diverse fields from the pharmaceutical industry to banking. We used a commercial computer program called TheGmax. We also tested for a possible correlation between maternal demographic characteristics, gestational age, and significant metabolites in both trisomy 18 and normal cases.
Results
In our initial metabolomic analysis, the 30 cases with trisomy 18 were compared with 60 matched normal control subjects. Table 1 gives a comparison of the maternal demographic characteristics of trisomy 18 and healthy euploid cases. As seen from these data, CRL was lower in the affected cases, and ΔNT and mean maternal age were higher in the affected cases. There was a significant difference in maternal racial heritage in the 2 groups with a disproportionately high percentage of white pregnancies among the euploid group. There were no significant differences in the frequency of smokers and medical disorders between the 2 groups (not shown). In Figure 1 , the 2- and 3-dimensional PLS-DA plots provide significant visual evidence of clustering or separation of the 30 trisomy 18 vs the 60 matched euploid control subjects. Permutation analysis with 2000 resamplings was performed to determine whether the observed separation was due to chance. The results of the permutation analysis indicated that the probability that the observed separation or discrimination between trisomy 18 and normal control specimens was due to chance was .0095.
Parameter | Trisomy 18 (n = 30) a | Control cases (n = 60) a | P value |
---|---|---|---|
Crown rump length, mm | 58.7 ± 6.5 | 65.5 ± 8.8 | < .001 |
Delta nuchal translucency, mm | 4.0 ± 2.6 | 0.7 ± 0.3 | < .001 |
Maternal age, y | 36.7 ± 5.2 | 31.3 ± 6.4 | < .001 |
Maternal weight, kg | 69.7 ± 20.6 | 66.4 ± 13.7 | .29 |
Maternal race, % | .001 | ||
White | 27.0 ± 28.7 | 67.0 ± 71.3 | |
Nonwhite | 3.0 ± 10.0 | 47.0 ± 41.2 | |
Parity: nulliparous, % | 9.0 ± 30.0 | 52.0 ± 45.6 | .149 |

The VIP plot in Figure 2 indicates that 2-hydroxybutyrate was the most discriminating metabolite for the separation of cases with trisomy 18 from euploid control specimens in the primary analyses. The heat map on the right of the Y-axis indicates that 2-hydroxybutyrate was elevated in trisomy 18 compared with the control specimens.

We performed analyses in the primary study group (trisomy 18, 30 cases; euploid, 60 control subjects) to assess the performance of metabolomic markers in this smaller dataset in which cases were exactly matched with control subjects. The purpose of this exercise was 2-fold: First, we wanted to minimize any unrecognized bias that would affect the results (the smaller number of subjects in this group limits the number of predictive variables that can be considered in each regression analysis); second, we wanted to determine whether the findings in this matched group were consistent with that of the expanded study group in which a larger number of predictive markers can be considered simultaneously in the regression analysis and be presented subsequently. As shown in Table 2 , the combination of 2-hydroxybutyrate and glycerol was sensitive for trisomy 18 detection. Maternal race did not contribute to trisomy 18 prediction when considered with these markers.
Model | Sensitivity, % | Specificity, % | Area under the curve (95% CI) | P value |
---|---|---|---|---|
2-hydroxybutyrate, glycerol | 53.3 | 84.6 | 0.78 (0.68–0.88) | < .001 |
2-hydroxybutyrate, glycerol, maternal age b | 73.3 | 96.6 | 0.92 (0.85–0.98) | < .001 |
a Trisomy 18, 30 cases; matched normal control, 60 cases
For the second series of analyses, the 30 cases with trisomy 18 were compared with an expanded number of euploid control subjects (n = 114). As noted earlier, the purpose of evaluating an expanded patient group is to strengthen the power for logistic regression analyses. Table 3 compares the metabolite concentrations between these 2 groups. Of a total of 38 metabolites that were evaluated in this expanded analysis, 21 metabolites were present in significantly different maternal serum concentrations between the 2 groups. Several logistic regression analyses were performed on this expanded dataset. The independent variables that were used in the logistic regression analysis were chosen on the basis of the most discriminating metabolite that was indicated by VIP analysis and important maternal demographic markers (such as age, race, and fetal gestational age). These are used currently in standard clinical screening. These were used to develop prediction algorithms from which AUC curves along with the sensitivity and specificity were calculated. As shown in Table 3 , a metabolite-only algorithm that was based on 2-hydroxybutyrate had a 53.3% sensitivity and 86% specificity for trisomy 18 detection. When this metabolite was combined with maternal age, the sensitivity increased to 63.3% and specificity to 95.6%. Maternal race did not contribute significantly to trisomy 18 prediction in these analyses. When 2-hydroxybutrate was combined with ΔNT, a sensitivity of 90.0% and a specificity of 100.0% were achieved ( Table 4 ).
Metabolite | Concentration, μmol/L a | P value | |
---|---|---|---|
Trisomy 18 (n = 30) | Control cases (n = 114) | ||
2-hydroxybutyrate | 26.96 ± 13.11 | 16.93 ± 8.17 | < .001 |
3-hydroxybutyrate | 54.57 ± 64.53 | 32.9 ± 53.42 | < .004 |
3-hydroxyisovalerate | 7.38± 4.18 | 5.24± 3.84 | .009 |
Acetate | 28.26 ± 12.83 | 22.9 ± 31.703 | .79 |
Acetoacetate | 19.56 ± 10.63 | 16.15 ± 9.18 | .08 |
Acetone | 22.19 ± 10.86 | 15.98 ± 9.44 | < .001 |
Alanine | 293.4 ± 83.44 | 258.62 ± 85.38 | < .015 |
Arginine | 110.97 ± 26.59 | 122.82 ± 39.7 | < .04 |
Asparagine | 39.13 ± 16.35 | 31.89 ± 13.6 | < .04 |
Betaine | 26.51 ± 11.92 | 25.27 ± 10.03 | .98 |
Carnitine | 26.04 ± 10.5 | 24.3 ± 11.7 | .256 |
Choline | 10.2 ± 2.2 | 31.6 ± 100.6 | .875 |
Citrate | 77.6 ± 19.1 | 66.1 ± 22.9 | .008 |
Creatine | 30.3 ± 12.8 | 35.6 ± 15.15 | .054 |
Creatinine | 55.9 ± 14.0 | 53.7 ± 14.6 | .496 |
Ethanol | 58.8 ± 72.3 | 63.7 ± 54.1 | .346 |
Formate | 19.1 ± 21.3 | 18.2 ± 11.2 | .483 |
Glucose | 4133.0 ± 903.7 | 3848.99 ± 783.5 | .124 |
Glutamine | 292.9 ± 77.3 | 250.2 ± 77.3 | .007 |
Glycerol | 281.8 ± 111.7 | 194.3 ± 157.44 | < .001 |
Glycine | 261.6 (99.4) | 209.8 (74.9) | .001 |
Isobutyrate | 6.77 ± 2.5 | 5.6 ± 4.7 | .003 |
Isoleucine | 41.1 ± 10.7 | 38.6 ± 16.6 | .54 |
Lactate | 1247.6 ± 900.3 | 946.4 ± 451.0 | .03 |
Leucine | 72.95 ± 24.0 | 71.6 ± 40.6 | .04 |
Malonate | 14.8 ± 7.5 | 15.0 ± 8.5 | .54 |
Methionine | 20.15 ± 7.05 | 26.09 ± 11.26 | .007 |
Ornithine | 36.83 ± 13.32 | 28.20 ± 10.61 | .001 |
Phenylalanine | 74.91 ± 34.67 | 58.24 ± 28.67 | .003 |
Proline | 135.31 ± 42.98 | 125.42 ± 48.53 | .141 |
Propylene-glycol | 9.66 ± 4.10 | 7.94 ± 3.69 | .019 |
Pyruvate | 74.66 ± 35.11 | 59.70 ± 23.3 | .04 |
Serine | 118.06 ± 37.23 | 132.03 ± 50.64 | .067 |
Succinate | 6.90 ± 6.33 | 6.50 ± 10.83 | .018 |
Threonine | 104.34 ± 48.09 | 108.80 ± 42.53 | .36 |
Tyrosine | 56.9 ± 15.2 | 55.82 ± 19.6 | .46 |
Valine | 121.9 ± 44.00 | 119.6 ± 38.1 | .53 |
1-methylthistidine | 51.2 ± 17.6 | 43.4 ± 22.3 | .018 |
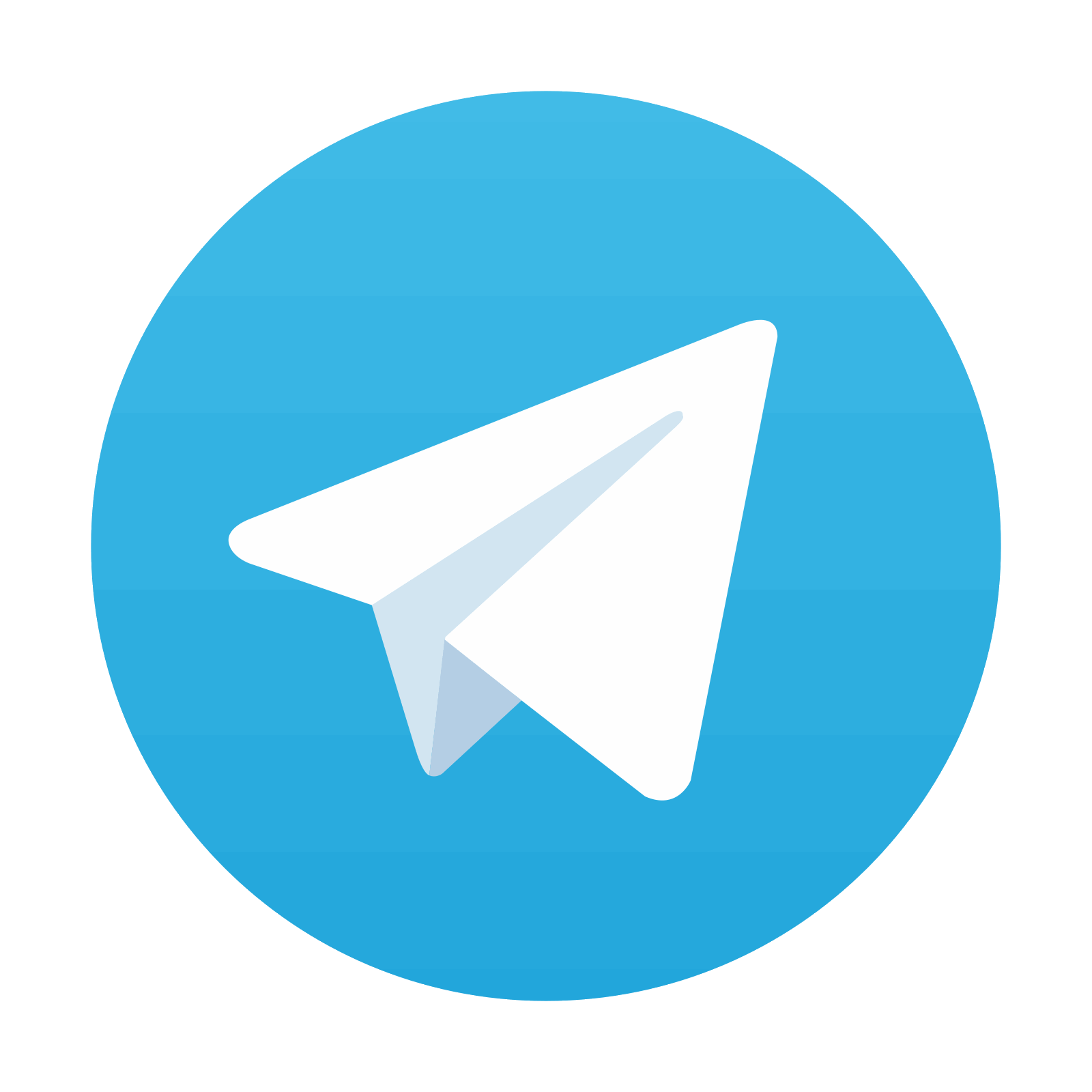
Stay updated, free articles. Join our Telegram channel

Full access? Get Clinical Tree
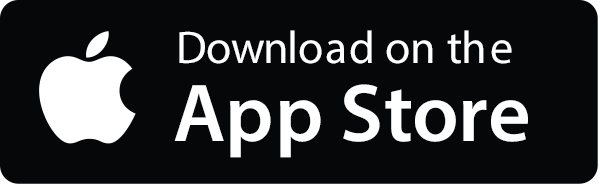

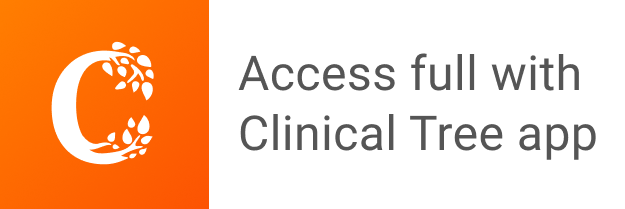