The article below summarizes a roundtable discussion of a study published in this issue of the Journal in light of its methodology, relevance to practice, and implications for future research. Article discussed:
Kaimal AJ, Little SE, Odibo AO, et al. Cost-effectiveness of elective induction of labor at 41 weeks in nulliparous women. Am J Obstet Gynecol 2011;204:137.e1-9.
The full discussion appears at www.AJOG.org , pages e1-3.
Discussion Questions
- ■
What are the key components of a cost-effectiveness analysis?
- ■
What is a QALY?
- ■
What is discounting?
- ■
What were the primary findings?
- ■
How do you test the model’s robustness?
- ■
What additional research might be useful?
See related article, page 137
This month, Journal Club members discussed an excellent article on the role of elective induction at 41 weeks’ compared with expectant management of pregnant women. The authors of the article used a cost-effectiveness analysis to compare these 2 strategies. Discussants found the article to be well-executed and interesting. Given that we will likely see more such studies, it is important for readers to understand the methods behind this type of investigation.
Dissecting the cost-effectiveness analysis
As is implicit in the name, a cost-effectiveness analysis involves careful evaluation of the cost and effectiveness of alternate courses of action. Rather than simply comparing the expenses of 2 routes, the analysis takes into consideration the consequences of each decision and transforms these into nonmonetary outcome measures, such as quality-adjusted life years (QALYs) or years of life saved. Using decision analysis as a conceptual model, the effectiveness of alternate strategies is determined based on clinical probabilities. Costs of the outcomes are then identified and valued, and the net cost of each strategy is determined per unit of outcome measure. Typically, various assumptions within the model are then tested using sensitivity analysis.
Costs can be difficult to measure, and readers should look carefully at these. Contributors to cost must be pinpointed and assessed. Valuing costs can be done either by micro-costing, which is direct enumeration and costing out of every input consumed in the intervention, such as laboratory, pharmaceutical, and nursing costs, or by gross-costing. Gross-costing, the more common approach, estimates costs as a measure of typical cost (eg, average cost of a hospital day or physician visit). Many of these costs can be obtained by doing a thorough literature search on the topic; government reports are often helpful. If no published data exist, costs can be estimated using fee schedules or institutional reimbursement data. The final cost of a particular strategy can then be estimated by summing the costs of all components in that pathway.
Profit now; pay later
When comparisons between strategies in a model are made at a single time point, variations in the timing of costs and benefits associated with each separate approach must be accounted for, and this is accomplished with discounting. Benefits may occur at the present time, or they may come later. Similarly, variability exists in when costs are incurred.
Discounting is based on the principle that as a society, we value benefits that occur at the present time—the time frame in which most models are built—while costs that occur at a later time are preferred. In other words, like money, the desired outcome is worth more today than it might be 5 years from now. To account for these temporal differences, costs and utilities are discounted at an annual rate for each year beyond the present.
The utility, or the patient’s preference for a particular outcome, is represented by a number on a scale that generally ranges from 0–1. A common example is a scale in which 0 signifies death and 1 stands for optimal health. Different methods can be used to designate values for each possible outcome in between.
QALYs, which can be computed by multiplying the utility by the number of years the patient lives with a particular result, are useful because they assign a metric value to the effects of various interventions and therefore provide a measure of health effect. The problem is that summing them assumes all QALYs are equal. However, an intervention with a small benefit for many might have the same value as a treatment with a large benefit for a few. For example, 2 QALYs can be the result of a patient’s getting 2 years of the best possible health (1 ×2 ) or 4 years at a lesser outcome (0.5 ×4 ). In the article by Dr Kaimal et al, a 3% annual discount rate was employed to account for the progressive reduction in value of health benefits, or QALYs, gained in future years.
No sure thing
Cost-effectiveness analyses have an element of uncertainty in that the baseline decision of the model is built on the point estimates of each parameter considered in the model. It then becomes important to test the robustness of the model by examining the effect of uncertainty in the various estimates on the decision of the model. For example, consider a model that includes an estimate of treatment effect that is a 20% risk reduction. Yet, the literature contains a plausible range of 15–40% reduction in risk. It would be important to explore the impact on the decision of the model by varying the risk reduction estimate across the range. This is referred to as a 1-way sensitivity analysis.
Several estimates of probability, utility, and costs, are necessary to cost-effectiveness models, and a degree of uncertainty is inherent in each factor. Therefore, sensitivity analyses that consider variation in each estimate individually and then in combination (2-way, and multi-way) are crucial. It is possible to test a model’s robustness further by simultaneously considering all or a selected number of estimates across their likely ranges. These authors used Monte Carlo simulation, a probabilistic form of multivariable sensitivity analysis that allows repetitive simulations of the model to run with point estimates sampled at random from probable distributions.
The researchers found that induction of labor at 41 weeks compared to expectant management at 42 weeks resulted in lower rates of all adverse obstetric outcomes, including perinatal demise, shoulder dystocia, meconium aspiration syndrome, and severe perineal lacerations. They also determined that the intervention was cost-effective. As a result, they recommended that women undergo elective induction at 41 weeks’ gestation. Journal Club members suggested more research was required before the proposal was widely implemented.
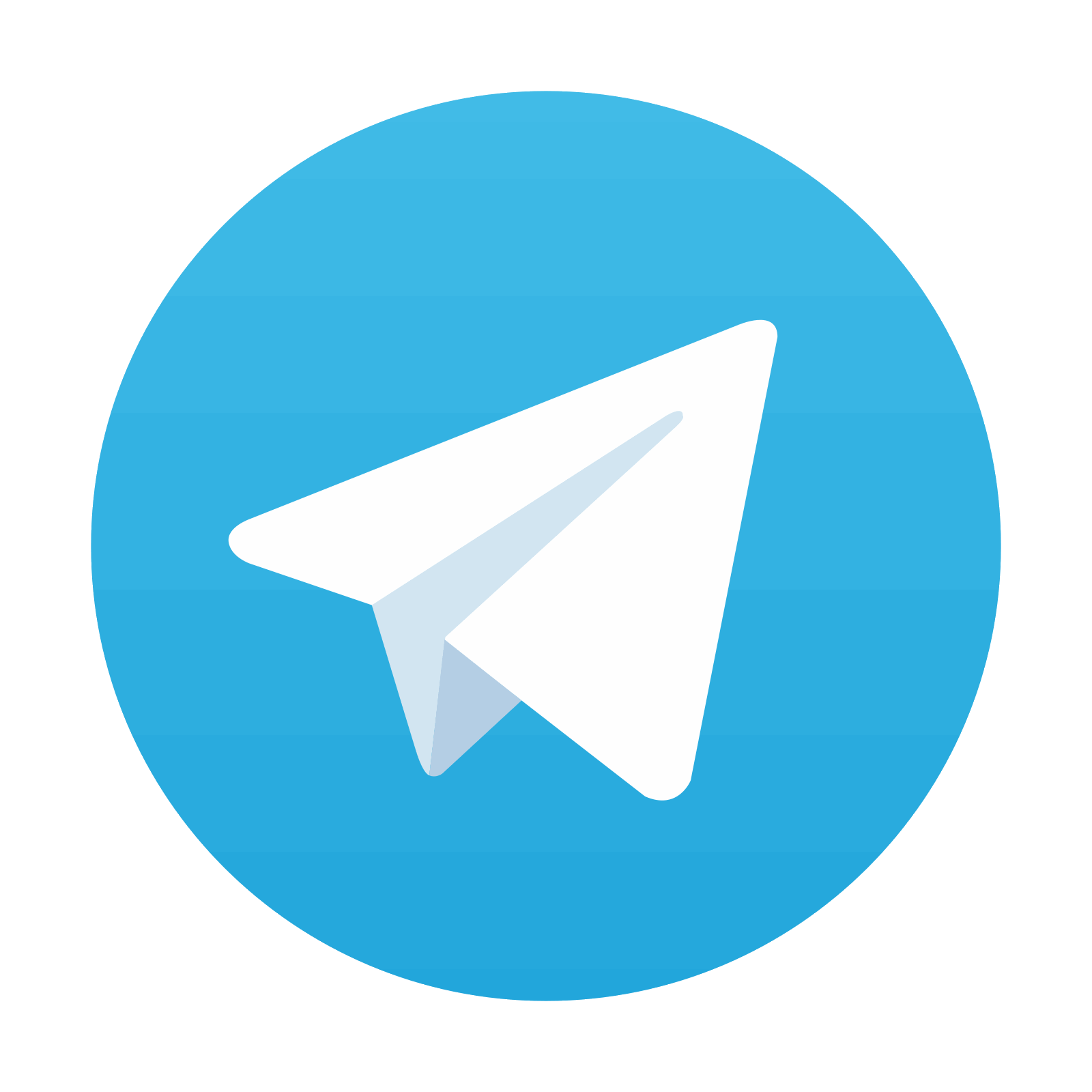
Stay updated, free articles. Join our Telegram channel

Full access? Get Clinical Tree
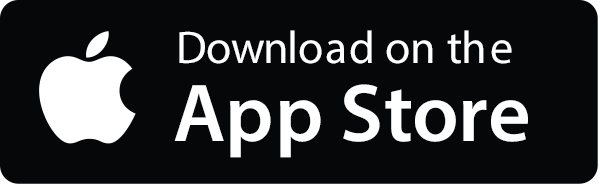
