Objective
Metabolomics has the potential to reveal novel pathways involved in the pathogenesis of preterm birth (PTB). The objective of this study was to investigate whether the cervicovaginal (CV) metabolome was different in asymptomatic women destined to have a PTB compared with term birth.
Study Design
A nested case-control study was performed using CV fluid collected from a larger prospective cohort. The CV fluid was collected between 20-24 weeks (V1) and 24-28 weeks (V2). The metabolome was compared between women with a spontaneous PTB (n = 10) to women who delivered at term (n = 10). Samples were extracted and prepared for analysis using a standard extraction solvent method. Global biochemical profiles were determined using gas chromatography/mass spectrometry and ultra-performance liquid chromatography/tandem mass spectrometry. An ANOVA was used to detect differences in biochemical compounds between the groups. A false discovery rate was estimated to account for multiple comparisons.
Results
A total of 313 biochemicals were identified in CV fluid. Eighty-two biochemicals were different in the CV fluid at V1 in those destined to have a PTB compared with term birth, whereas 48 were different at V2. Amino acid, carbohydrate, and peptide metabolites were distinct between women with and without PTB.
Conclusion
These data suggest that the CV space is metabolically active during pregnancy. Changes in the CV metabolome may be observed weeks, if not months, prior to any clinical symptoms. Understanding the CV metabolome may hold promise for unraveling the pathogenesis of PTB and may provide novel biomarkers to identify women most at risk.
Preterm birth (PTB) is among the leading cause of neonatal morbidity and mortality, accounting for 11.2% of live births in the United States as of 2012. Studies have shown that women with a short cervix are at an increased risk for PTB, yet most cases of PTB are not preceded by a sonographic short cervix. The sonographic short cervix could be considered a phenotype of premature cervical modeling, but in fact, the immunological, biochemical, molecular, and cellular processes involved in premature cervical remodeling have not been fully elucidated.
Although there is some emerging evidence regarding the cervicovaginal (CV) microbiota and PTB, how the CV microbiota might alter cervical remodeling has not been studied. Whereas clinical data support the role of infection and/or inflammation in the pathogenesis of preterm birth and understanding that cervical remodeling must occur at any gestational age for parturition to occur, the interaction of these biological and molecular events to induce spontaneous preterm birth have not been elucidated.
Metabolomics, the study of metabolites less than 1 kDa necessary for structure and function of an organism at the cellular level, is able to provide information on multiple biological pathways acting in concert. It is a powerful tool of fundamental discovery that relays information from the host, the microbiota, and the environment to give a comprehensive view of a biological system in both healthy and diseased states. Metabolomics has the potential to uncover novel mechanistic pathways involved in premature cervical remodeling and PTB.
The importance of the metabolome may be best conceptualized by recent advances in understanding the role of gut microbiota in the development of colorectal cancer in patients with inflammatory bowel disease. Although it is well known that patients with inflammatory bowel disease are at an increased risk for colorectal cancer, it seems that the bacterial metabolites, more than the bacterial composition itself, may protect from or predispose toward cancer within the gastrointestinal tract. Metabolomic analysis has revealed the actions of the microbiota may result in the production of tumor-promoting metabolites, such as acetylaldehyde and nitrosamine, and may mediate tumor suppressive effects through inactivation of carcinogens. Furthermore, metabolomics have led to the discovery of novel targets for therapeutics in preventing cell proliferation in cancer. Examination of the metabolome has the potential to reveal novel mechanistic pathways involved in the pathophysiology of disease and provide therapeutic targets to prevent disease.
Metabolomics in pregnancy is in its infancy. Existing studies have demonstrated the ability to test the metabolome in maternal plasma, amniotic fluid, placenta, cord blood, and urine. These studies have explored the metabolome among women with preeclampsia, gestational diabetes, fetal growth restriction, and fetal malformations and have shown key differences in metabolites between cases and controls.
Despite these studies, the CV fluid metabolome and how it might be altered in women with preterm birth has not been studied. Investigating the metabolome in CV fluid may provide insight into the cellular metabolism as it relates to the host and/or existing microbiota involved with premature cervical remodeling. Therefore, the objective of this study was to investigate whether the CV fluid metabolome was different in asymptomatic women destined to have a PTB compared with term birth.
Materials and Methods
Study population
A nested case-control study was performed from a prospective cohort. The UK Prognostic Study of the Interferon Gamma Release Assay for Tuberculosis (PREDICT) study was performed at a single, urban tertiary care institution between August 2011 and November 2012. This study was approved by the Institutional Review Board at the University of Pennsylvania.
Women with a singleton pregnancy who were at high risk for preterm birth based on a prior history of preterm birth or second-trimester loss, previous cervical surgery without a subsequent full term birth, and/or uterine anomaly were approached for recruitment into the study. Women who were receiving systemic steroids or immunosuppressive therapy, had human immunodeficiency virus, lupus, pregestational diabetes mellitus, rheumatoid arthritis, Crohn’s disease, ulcerative colitis, cancer or an organ transplant were excluded. Furthermore, women with activity in the vagina in the 24 hours prior to specimen collection (including sexual intercourse, transvaginal ultrasound, digital cervical examination, or vaginal lubricants), had active vaginal bleeding, had evidence of a rupture of membranes, and/or had a cervical dilation of 3 cm or greater at the time of enrollment were excluded.
Pertinent demographic, clinical, and obstetric data were abstracted from the medical record as previously described. Women with spontaneous preterm birth at less than 37 weeks of gestation were identified (n = 10). Controls were selected at random from women having deliveries at longer than 38 weeks of gestation without evidence of chorioamnionitis. Cervicovaginal biospecimens were collected at 2 time points: visit 1 (V1) occurred at 20 weeks to 23 weeks 6 days of gestation and visit 2 (V2) occurred at 24 weeks to 27 weeks 6 days of gestation. All cases of preterm birth were reviewed and confirmed that they were spontaneous preterm births.
Biospecimen collection
CV fluid was collected following insertion of a sterile speculum using a sterile cotton-tipped swab. Samples were collected in 0.5 mL phosphate-buffered saline, immediately placed in liquid nitrogen, and stored at –80°C for future use.
Metabolomics analysis
Metabolomics analysis was performed by Metabolon, Inc (Research Triangle Park, NC) as previously described. Samples were accessioned into the Metabolon Laboratory Information Management System (LIMS) and assigned a unique identifier. The samples (and all derived aliquots) were bar coded and tracked by the LIMS. All samples were maintained at –80°C until processed. The samples were prepared using the MicroLab STAR system from Hamilton Co. (Reno, NV).
To remove the protein fraction while maximizing the recovery of small molecules, the samples were prepared using a proprietary series of organic and aqueous extractions. The resulting extract was divided into 2 fractions: one for analysis by ultraperformance liquid chromatography (UPLC)/tandem mass spectrometry and one for analysis by gas chromatography/mass spectrometry (GC/MS). Samples were placed briefly on TurboVap (Zymark, Hopkinton, MA) to remove the organic solvent. Each sample was then frozen and underwent vacuum desiccation. Samples were then prepared for either liquid chromatography/mass spectrometry or GC/MS.
For quality assurance/quality control purposes, a selection of quality control compounds was added to every sample, including those that were being examined. These compounds were carefully chosen so as not to interfere with the measurement of the endogenous compounds. The quality control samples are primarily used to evaluate the control process for each study as well as aiding in data curation.
Liquid chromatography/mass spectrometry
The liquid chromatography/mass spectrometry portion of the platform was based on a Waters ACQUITY UPLC and Thermo Finnigan (San Jose, CA) linear trap quadropole mass spectrometer, which consisted of an electrospray ionization source and linear ion trap mass analyzer. The sample extract was split into 2 aliquots, dried, and then reconstituted in acidic or basic liquid chromatography-compatible solvents, each of which contained 11 or more injection standards at fixed concentrations.
One aliquot was analyzed using acidic positive ion optimized conditions and the other using basic negative ion optimized conditions in 2 independent injections, using separate dedicated columns. Extracts reconstituted in acidic conditions were gradient eluted using water and methanol, both containing 0.1% formic acid, whereas the basic extracts, which also used water/methanol, contained 6.5 mM ammonium bicarbonate.
The mass spectrometry (MS) analysis alternated between MS and data-dependent MS 2 scans using dynamic exclusion, and the scan range was from 80 to 1000 mass to charge ratio. Raw data files were archived and extracted as described below.
Gas chromatography/mass spectrometry
The samples destined for GC/MS analysis were redried under vacuum desiccation for a minimum of 24 hours prior to being derivatized under dried nitrogen using bistrimethyl-silyl-triflouroacetamide. The gas chromatography column was 5% phenyl and the temperature ramp was from 40°C to 300°C in a 16 minute period. The samples were analyzed on a Thermo-Finnigan Trace DSQ fast-scanning, single-quadrupole mass spectrometer using electron impact ionization. The instrument was tuned and calibrated for mass resolution and mass accuracy on a daily basis. The information output from the raw data files was automatically extracted as discussed below.
Bioinformatics
The informatics system consisted of 4 major components, the LIMS, the data extraction and peak-identification software, data processing tools for quality control and compound identification, and a collection of information interpretation and visualization tools for use by data analysts. The hardware and software foundations for these informatics components were the LAN backbone, and a database server running Oracle 10.2.0.1 Enterprise Edition (Redwood City, CA).
Data extraction and compound identification
Raw data were extracted, peak identified, and quality control processed using Metabolon’s hardware and software. Compounds were identified by comparison with library entries of purified standards or recurrent unknown entities. Metabolon maintains a library based on authenticated standards that contains the retention time/index, mass to charge ratio, and chromatographic data (including tandem MS [MS/MS] spectral data) on all molecules present in the library. Furthermore, biochemical identifications are based on 3 criteria: retention index within a narrow window of the proposed identification, nominal mass match to the library ± 0.4 amu, and the MS/MS forward and reverse scores between the experimental data and authentic standards.
The MS/MS scores are based on a comparison of the ions present in the experimental spectrum with the ions present in the library spectrum. Although there may be similarities between these molecules based on one of these factors, the use of all 3 data points can be utilized to distinguish and differentiate biochemicals. More than 3500 commercially available purified standard compounds have been acquired and registered into LIMS for distribution to both the liquid chromatography-MS and GC-MS platforms for the determination of their analytical characteristics.
Statistical analyses
An analysis of variance (ANOVA) was used to identify biochemicals that differed significantly between the groups following log transformation and imputation of missing values, if any, with the minimum observed value for each compound. The data were analyzed both normalized to protein and nonnormalized, and results were similar between the groups. However, because of the significant differences in the protein hydrolysis between cases and controls, the data presented here are those results that were not normalized to the protein content.
Two-way ANOVA with repeated measures was used to identify biochemicals exhibiting significant interaction and main effects for the experimental parameters of status and time. The data were analyzed in 2 ways to account for changes between the groups and changes that occurred over time within the same subject. ANOVA was used when comparing metabolites between groups across a single time point (in which each subject was unique), whereas a 2-way ANOVA with repeated measures was used to take into account metabolites that changed over time within each subject (accounting for within-subject characteristics). Only metabolites with a value of P < .05 were considered significant. Additionally, because this was a study of discovery, metabolites that approached significance (ie, had an alpha level between 0.05 and 0.1) were explored.
An estimate of the false discovery rate (q-value) was calculated to account for multiple comparisons that occur in metabolomics studies. When analyzing numerous compounds, statistical significance may be found because of random chance. The q-value describes the false discovery rate; a low q-value (q ≤ .10) is indicative of high confidence in a result.
Random forest (RF) is a supervised classification technique reporting on the consensus of a large number of decision trees. In this study, the term and preterm birth cohorts at both time points were classified to achieve the following: (1) assess the capacity to distinguish birth cohorts on the basis of global metabolic profiles and (2) identify biochemicals important to the classification. An accuracy of 25% is expected by random chance when comparing 4 groups, so the RF classification accuracy of 32% is better than random chance. Additionally, according to the confusion matrix, when the RF model misclassified samples, it tended to pick the wrong time point (ie, V1 vs V2) but the correct birth cohort (ie, term vs PTB), so the model may be even more accurate than the 32% accuracy belies.
Statistical analysis for clinical data
Categorical variables were compared between groups by a χ 2 or Fisher exact test, where appropriate. Continuous variables were compared by the Student t test or the Mann-Whitney U test, depending on the distribution of the data. Spearman’s correlation coefficients were calculated to determine correlations between metabolites and cervical length less than 2.9 cm (median). A value of P < .05 was considered statistically significant. Clinical data were analyzed using STATA (version 11.0; StataCorp, Inc, College Station, TX).
Results
Demographics
Clinical and obstetric data are listed in Table 1 . Women with a preterm delivery were similar in age, race, gestational age at V1 and V2, and cervical length at V1 compared with women with a term delivery. Not surprisingly, women who ultimately delivered preterm had a significantly higher number of prior PTB, a significantly lower cervical length at V2 and gestational age at delivery as compared with term birth ( Table 1 ).
Characteristic | PTB | Term | P value a |
---|---|---|---|
Age, y b | 23.5 (17–38) | 31.5 (26–35) | .22 |
Race c | .80 | ||
White | 8 (20) | 7 (70) | |
Black | 1 (10) | 2 (20) | |
Asian | 1 (10) | 1 (10) | |
Prior PTB c | 8 (80) | 2 (20) | .02 |
Gestational age at V1, wks b | 20 4/7 (20–23) | 20 3/7 (19 6/7–23 3/7) | .70 |
Gestational age at V2, wks b | 26 3/7 (24 1/7–27 5/7) | 26 (24 6/7–27 4/7) | 1.00 |
Cervical length at V1, cm b | 3.3 (2.1–3.5) | 3.7 (2–4.8) | .07 |
Cervical length at V2, cm b | 2.9 (1.6–3.6) | 3.8 (2.4–4.7) | .03 |
Gestational age at delivery, wks b | 33 4/7 (26–36) | 40 (39–41) | < .001 |
Cervicovaginal metabolome
A total of 313 biochemicals were identified in the CV fluid. Significant differences were noted in the CV fluid metabolome between women destined to have a PTB compared with term birth. Overall, women who had a term delivery had significant changes in carbohydrate and lipid metabolism between the second and third trimesters. In women with a preterm delivery, significant changes in amino acid metabolism were noted between the second and third trimesters.
Of note, the significant change in carbohydrate metabolism observed among women with a term delivery from V1 to V2 does not occur in women with PTB. At visit 1, women with a preterm delivery had significant changes in amino acid and peptide metabolism as compared with women with a term delivery. Changes in multiple metabolic pathways were noted at V2; women with a preterm delivery had significant changes in amino acid, peptide, carbohydrate, and lipid metabolism as compared with women with a term delivery ( Table 2 ). A random forest plot depicting classes of metabolites that were important in distinguishing between preterm and term delivery can be found in the Figure .
Metabolic pathway | TermV2TermV1 Term V 2 Term V 1 | PTBV2PTBV1 PTB V 2 PTB V 1 | PTBV1TermV1 PTB V 1 Term V 1 | PTBV2TermV2 PTB V 2 Term V 2 |
---|---|---|---|---|
Amino acid | 0 | 5 | 6 | 3 |
Peptide | 1 | 0 | 22 | 4 |
Carbohydrate | 9 | 0 | 3 | 3 |
Energy | 0 | 0 | 1 | 0 |
Lipid | 3 | 0 | 3 | 3 |
Nucleotide | 0 | 0 | 2 | 0 |
Cofactors and vitamins | 1 | 0 | 1 | 1 |
Xenobiotics | 2 | 2 | 2 | 1 |
Total | 16 | 7 | 40 | 15 |
a Significantly different pathways between comparison groups with P < .05
b Total number of pathways that are significantly different (either increased or decreased) between comparison groups.

A more in-depth look into the metabolome among women with preterm and term delivery reveals distinct differences in metabolites. In women delivering at term, carbohydrate and lipid metabolism appear to be down-regulated between the second and third trimesters. Specifically for carbohydrate metabolism, glucose, glucose-6-phosphate, xylulose, maltohexose, N-acetylglucosamine, and N-acetylgalactosamine were significantly decreased by the third trimester (0.2-, 0.14-, 0.59-, 0.35-, 0.45-, and 0.32-fold change, respectively; Table 3 ) in women who had a term delivery. Additionally, an increase in methyl-4-hydroxybenzoate, an antimicrobial agent, was noted among women with term delivery between the second and third trimesters (8.8-fold, P < .1).
Metabolic pathway | Subpathway | Biochemical name | TermV2TermV1 Term V 2 Term V 1 | PTBV2PTBV1 PTB V 2 PTB V 1 | PTBV1TermV1 PTB V 1 Term V 1 | PTBV2TermV2 PTB V 2 Term V 2 |
---|---|---|---|---|---|---|
Amino acid | Lysine metabolism | Lysine | 0.48 b | |||
Phenylalanine and tyrosine metabolism | Phenylacetylglutamine | 0.19 b | ||||
3-(4-Hydroxyphenyl)lactate | 0.36 b | |||||
p-cresol sulfate | 0.34 b | |||||
Tryptophan metabolism | Tryptamine | 1.66 b | ||||
Indolelactate | 0.24 b | |||||
3-Indoxyl sulfate | 0.12 b | |||||
Methionine, cysteine, SAM, and taurine metabolism | Cystine | 0.25 b | ||||
Urea cycle; arginine and proline metabolism | Urea | 0.55 b | ||||
Ornithine | 0.07 b | |||||
Creatine metabolism | Creatinine | 0.65 b | 0.6 b | |||
Guanidino and acetamido metabolism | 4-Guanidinobutanoate | 0.66 b | ||||
Glutathione metabolism | 5-Oxoproline | 0.44 b | ||||
Peptide | Dipeptide | Dimethylarginine (SDMA + ADMA) | 0.37 b | |||
Alanylphenylalanine | 0.4 b | |||||
Arginylproline | 0.33 b | |||||
Glycylisoleucine | 0.42 b | |||||
Glycylleucine | 0.4 b | |||||
Isoleucylalanine | 0.47 b | |||||
Isoleucylglutamate | 0.41 b | |||||
Isoleucylglycine | 0.4 b | 0.48 b | ||||
Isoleucylserine | 0.37 b | |||||
Isoleucylthreonine | 0.31 b | 0.41 b | ||||
Leucylglutamate | 0.44 b | |||||
Leucylleucine | 0.49 b | |||||
Phenylalanylisoleucine | 0.52 b | |||||
Phenylalanylleucine | 0.45 b | |||||
Phenylalanylphenylalanine | 0.66 b | 0.64 b | ||||
Threonylproline | 0.59 b | |||||
Tyrosylglutamine | 0.37 b | |||||
Tyrosylleucine | 0.41 b | |||||
Valylalanine | 0.37 b | |||||
Valylaspartate | 0.34 b | |||||
Valylglycine | 0.34 b | |||||
Valylleucine | 0.33 b | |||||
Valylphenylalanine | 0.36 b | |||||
Valylthreonine | 0.49 b | |||||
Carbohydrate | Glycolysis, gluconeogenesis, and pyruvate metabolism | Glucose | 0.2 b | 3.73 c | ||
Glucose-6-phosphate | 0.14 b | 3.04 c | ||||
Isobar: fructose 1,6-diphosphate, glucose 1,6-diphosphate, myoinositol 1,4, or 1,3-diphosphate | 0.4 b | |||||
Pentose metabolism | Xylulose | 0.59 b | ||||
Xylose | 0.57 b | |||||
Glycogen metabolism | Maltohexaose | 0.35 b | ||||
Maltopentaose | 0.31 b | |||||
Maltose | 0.1 b | |||||
Aminosugar metabolism | N-acetylglucosamine | 0.45 b | 0.43 b | |||
N-acetylgalactosamine | 0.32 b | 0.62 b | ||||
N-acetylneuraminate | 4.9 c | |||||
Energy | Oxidative phosphorylation | Phosphate | 0.93 b | |||
Lipid | Long-chain fatty acid | Palmitoleate (16:1n7) | 1.4 c | |||
10-Heptadecenoate (17:1n7) | 1.56 c | |||||
Inositol metabolism | Myoinositol | 0.47 b | 0.42 b | |||
Lysolipid | 1-Stearoylglycerophosphoserine | 0.45 b | ||||
Monoacylglycerol | 1-Palmitoylglycerol (1-monopalmitin) | 0.44 b | 0.64 b | 2.24 c | ||
Steroid | Dehydroisoandrosterone sulfate | 0.67 b | ||||
Nucleotide | Purine metabolism, (hypo)xanthine/inosine containing | Xanthine | 5.88 c | |||
Pyrimidine metabolism, cytidine containing | Cytosine | 0.25 b | ||||
Cofactors and vitamins | Nicotinate and nicotinamide metabolism | Nicotinamide | 0.43 b | 2.12 c | ||
Nicotinamide adenine dinucleotide reduced | 2.22 b | |||||
Xenobiotics | Benzoate metabolism | 3-Hydroxyhippurate | 0.36 b | |||
Catechol sulfate | 0.32 b | |||||
Food component/plant | Erythritol | 0.84 b | 0.85 b | |||
Chemical | 2-Pyrrolidinone | 1.21 c | 1.21 c | |||
Glycolate (hydroxyacetate) | 0.66 b |
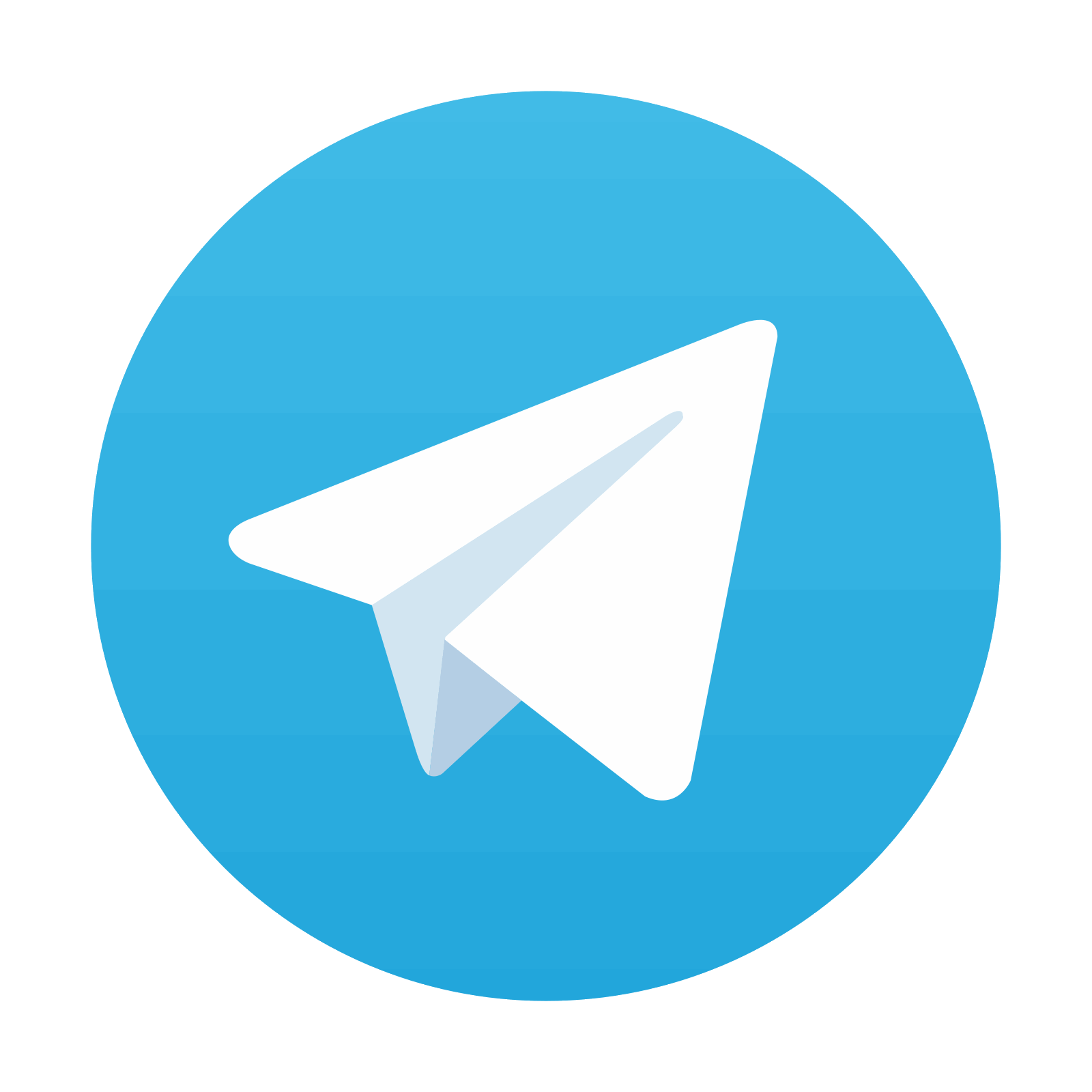
Stay updated, free articles. Join our Telegram channel

Full access? Get Clinical Tree
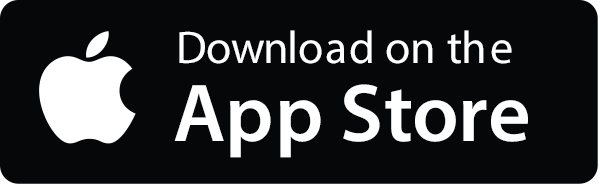
