Objective
Although a higher maternal body mass index is associated with preterm birth, it is unclear whether excess gestational weight gain (GWG) or obesity drives increased risk. We and others have shown that the placenta harbors microbiota, which is significantly different among preterm births. Our aim in this study was to investigate whether the preterm placental microbiome varies by virtue of obesity or alternately by excess GWG.
Study Design
Placentas (n = 320) were collected from term and preterm pregnancies. Genomic DNA was extracted and subjected to metagenomic sequencing. Data were analyzed by clinical covariates that included the 2009 Institute of Medicine’s GWG guideline and obesity.
Results
Analysis of 16S recombinant RNA–based metagenomics revealed no clustering of the microbiome by virtue of obesity ( P = .161). Among women who spontaneously delivered preterm, there was again no clustering by obesity ( P = .480), but there was significant clustering by excess GWG ( P = .022). Moreover, among preterm births, detailed analysis identified microbial genera (family and genus level) and bacterial metabolic gene pathways that varied among pregnancies with excess GWG. Notably, excess GWG was associated with decreased microbial folate biosynthesis pathways and decreased butanoate metabolism (linear discriminate analysis, >3.0-fold).
Conclusion
Although there were no significant alterations in the microbiome by virtue of obesity per se, excess GWG was associated with an altered microbiome and its metabolic profile among those women who experienced a preterm birth.
The human microbiome encompasses the totality of the microbes that live on and within our bodies and is the core of the emerging fields of metagenomic science and medicine ( Table 1 ). In 2012, the healthy microbiome in nonpregnant and pregnant women was defined across body sites and was recently expanded to now include in-depth characterization of the placental microbiome and longitudinal profiling of the vaginal (posterior fornix) microbiome throughout pregnancy.
Term | Definition |
---|---|
Microbiota | Microbial population present in different ecosystems (niches) of the body |
Microbiome | The totality of the microbes (and their genomes) who share our body space : can also refer to the totality of microbes (and their genomes) in a given body site or niche |
Metagenome | Entire community genomic repertoire and metabolic profile. |
Community | The entirety of microbes in a given body site or niche |
Dysbiosis | Microbial imbalance; altered community structure |
16S recombinant RNA sequencing | Highly conserved gene in different species of bacteria that enables its use as a universal primer |
Whole genome shotgun sequencing | WGS : used for metagenomic analysis |
Operational taxonomic units | OUT : a distinct sequence |
Diversity | Difference in the bacteria, either within a group of samples (alpha-diversity) or between a group of samples (beta-diversity) |
Richness | Number of OTUs (or species) in a sample: richness reflects the abundance of microbes across multiple taxa |
Alpha-diversity | Biodiversity within a group of samples |
Beta-diversity | Biodiversity between groups of samples |
Principle Coordinate Analysis | PCoA : used to examine beta-diversity between 2 groups of samples |
UniFrac | Used to calculate a distance measure between organismal communities with the use of phylogenetic information; with the use of UniFrac, all taxa that are found are placed on a phylogenetic tree. A branch leading to taxa from both samples is marked as “shared”; branches that lead to taxa that appear in only 1 sample are “unshared.” |
Phylogenetic distance | Uses UniFrac: distance is defined as the sum of unshared branch lengths divided by the sum of all shared and unshared branches |
Permutational multivariate analysis of variance | PERMANOVA : does not assume a normal distribution. |
Contrary to the commonly held paradigm that the upper reproductive tract and placenta are “sterile,” we and others have shown that microbiota is present, even in the absence of any clinical evidence of intraamniotic infection.
Using metagenomic approaches, we have observed that the placental microbiome profile significantly varied in association with spontaneous preterm birth and that the taxonomic profiles that are associated with term or preterm pregnancies were accompanied by variations in bacterial-encoded metabolic pathways. Collectively, these findings are consistent with multiple other published reports over the years that have demonstrated the presence of microbes in previously held “sterile” sites, including the endometrium, placenta, and chorion/amnion and amniotic fluid.
Although the cause of preterm birth is multifactorial, its association with infection and inflammation has long been suspected. The aforementioned variations in commensal microbiota–driven metabolism may result in the placental microbiome emerging as a key mediator in preterm birth. For example, although bacterial species that are present in preterm pregnancies may not be pathogenic necessarily, a relatively altered microbial community structure (dysbiosis) may convey an environment of localized inflammation that results in preterm birth.
Previously, obesity has been shown to be associated with variation in placental and systemic inflammation. Additionally, obesity is associated with preterm birth. Altogether, these observations have been assumed to suggest that obesity may contribute to preterm birth through increased inflammation. However, excess gestational weight gain (GWG) has also been associated with increased risk of preterm birth and alterations in metabolic markers. Thus this begs the question, is it obesity or excess GWG that modulates the triad of inflammation, an altered placental microbiome, and spontaneous preterm birth?
We hypothesized that excess GWG (but not obesity per se) would associate with distinct alterations in the placental microbiome and that these alterations would be pronounced significantly among women who spontaneously deliver preterm. The aims of this study were (1) to discriminate whether maternal obesity or GWG was associated with significant variation in the placental microbiome profile, (2) to determine whether this was observed in both the term and preterm interval, and (3) to determine which placental microbial metabolic pathways were affected by excess maternal GWG.
Materials and Methods
Subjects and sample materials
This study was approved by the Institutional Review Board at Baylor College of Medicine (H-26589) and Harris Health System and was performed with the use of specimens from our universal perinatal database and biospecimen repository (PeriBank database, Institutional Review Board H-26364; Baylor College of Medicine). All pregnant women who come to labor and delivery for delivery are recruited for enrollment into PeriBank, which is Baylor College of Medicine’s universal perinatal database and biospecimen repository. A detailed description of PeriBank and the selection criteria for this study, including the clinical metadata that are extracted, is available in our previous publication on the placental microbiome. Subjects for this study were recruited between August 2011 and November 2012 by trained PeriBank study personnel. As part of the consent process, we discussed with participants the potential risks of participation, including the physical risks that are associated with specimen collection and the possibility that protected health information or deidentified project data that are stored in a public repository could be released accidentally. The protocol and consent form described the precautions that are taken to reduce these risks. Additional protections for participants included coding genomic and metagenomic specimens and sequence data, with the use of controlled-access repositories for extracted nucleic acids and deidentified medical and human genome sequence data, and making efforts to remove any human sequence traces from the microbial data that are deposited in open access databases. If a participant withdrew consent after providing specimens, the remaining specimens and extracted nucleic acids were to be destroyed; however, any metagenomic sequence data that were already published in open access databases could not be retracted.
After written consent was obtained, clinical information was extracted from the medical record and subject interviews. The parent study (H-26589) was a case-cohort study that used 2 case-cohorts. Briefly, for the first case-cohort (preterm birth), the PeriBank database was queried to identify subjects who spontaneously delivered at <37 weeks’ gestation by best obstetric estimate. Spontaneous preterm birth was defined by standard obstetrics definitions as adequately documented gestational age >24 and <37 weeks’ gestation (by last menstrual period with <20-week gestation sonogram or by <14-week sonogram with subsequent second-trimester sonogram) and no evidence of maternal or fetal comorbidity that indicated induction of labor (such as preeclampsia or placenta previa). For the second case-cohort (antenatal infection), the database was queried for women with laboratory evidence of antenatal infection, which included sexually transmitted diseases, urinary tract infections, skin abscesses, appendicitis, periodontal disease, or bacterial meningitis. The control groups for cohort comparison were created by a 1:1 match with previously identified case subjects; women were matched for gestational age (except in the preterm case-cohort), mode of delivery, race/ethnicity, body-mass index, age, and diabetic status. In this manner, we enabled preterm birth cases potentially to carry a diagnosis of a remote history of antenatal infection and vice versa; this is consistent with the principles of a population-based case-cohort study design. Subjects with fatal fetal anomalies, fetal growth restriction, prelabor preeclampsia, placenta previa, and multifetal gestation were excluded because of the likelihood of indicated preterm birth. Women with intrapartum chorioamnionitis that was suspected clinically and had been treated with intrapartum antibiotics and confirmed by positive maternal or infant culture were excluded from this analysis.
For the present study, extracted and analyzed clinical metadata included self-reported and/or documented prepregnancy weight, documented early pregnancy (defined as <20 weeks’ gestation) weight, documented maternal delivery weight, and documented maternal height. Self-reported prepregnancy weight frequently is used in the literature, and the use of self-reported prepregnancy weight has been reported to be within a few pounds of actual prepregnancy weight. The use of first-trimester weight as a surrogate when prepregnancy weight is unknown is also a reasonable substitution when the prepregnancy weight is unknown. Of the 237 subjects in this study, the prepregnancy weight was used in 130 of the women (54.8%). Of the remaining 107 women, the first-trimester weight was used in 98 of 107 pregnancies (91.6%); weights up to 20 weeks’ gestation were used for the remaining 9 women. Ergo, of the 237 women who were included in the analysis herein, 228 of 237 women (96.2%) used prepregnancy or first-trimester documented weights, although only 3.8% arose from documented weight in the early midtrimester (>14 and <20 weeks’ gestation). Patients who had none of these variables were excluded because data were insufficient to calculate GWG. Other extracted clinical metadata included gravidity, parity, the presence or absence of Streptococcus agalactiae , the presence of antepartum infections and antibiotic use (ie, sexually transmitted infection, urinary tract infection, skin abscesses, appendicitis, periodontal disease, or bacterial meningitis), routine demographic variables and maternal health comorbidities (eg, age, race/ethnicity, smoking status, diabetes mellitus, hypertensive, hypothyroid status), and pregnancy outcomes (ie, gestational age at delivery, mode of delivery, presence of gestational hypertensive, and preeclamptic disorders). For the purposes of this study, all classes of diabetes mellitus and all hypertensive disorders were assessed in aggregate.
GWG calculation
GWG was calculated by subtracting the prepregnancy or early pregnancy weight (defined as <20 weeks’ gestation) from the delivery weight. Baseline body mass index (BMI) was determined with the use of prepregnancy or early pregnancy (<20 weeks’ gestation) weight. As noted previously, 96.2% of study subjects had a documented prepregnancy or first-trimester maternal weight. To calculate whether GWG exceeded recommendations, we used previously validated, BMI-specific formulas that were derived from the Institute of Medicine. Excess weight gain was defined as weight gain that was in excess of the maximum recommended weight gain for a given gestational age and prepregnancy BMI. Specifically, the Institute of Medicine recommends a maximum of 4.4 pounds in the first trimester for all women, regardless of BMI; thus, this was used as the first-trimester component and was added to the maximum recommended weekly weight gain, which is BMI-specific. These values are 1.3, 1, 0.7, and 0.6 pounds per week for underweight, normal weight, overweight, and obese women, respectively. This rate was multiplied by the number of weeks of pregnancy from the second trimester through delivery to yield the formulas shown in Table 2 , which shows the maximum recommended GWG by BMI ; GWG that exceeded the maximum recommended GWG was defined as excessive GWG . The women were then assigned to 2 cohorts: those with excess GWG (excess GWG) and those without excess GWG (no excess GWG).
Body mass index | Calculation |
---|---|
Underweight prepregnancy | = 4.4 + 1.3 (GADelivery – 13) |
Normal prepregnancy | = 4.4 + 1 (GADelivery – 13) |
Overweight prepregnancy | = 4.4 + 0.7 (GADelivery – 13) |
Obese prepregnancy | = 4.4 + 0.6 (GADelivery 13) |
Placental samples
All samples were collected by placental pathology-trained personnel according to the PeriBank Manual of Procedures. As described in the parent publication, after the placenta is delivered by the obstetrics provider, it is immediately passed off to PeriBank personnel in a clean container. In a separate room, 4- 6 1 cm × 1 cm × 1 cm cuboidal sections are excised circumferentially from separate areas of the placenta; each section was located approximately 4 cm from the cord insertion site by PeriBank personnel who wore facial masks and gloves and used sterile scalpels and tissue forceps. These clean and sterile conditions are part of the standardized technique that is used in the banking of all PeriBank specimens. After excision, the placental surfaces, namely the maternal decidua and the fetal chorionamnion, were excised and discarded to avoid potential contamination from maternal, fetal, or environmental surface exposure during delivery (vaginal or cesarean) or at any time during transport.
Metagenomics sequencing
This study was a subgroup analysis of our initial case-cohort study of the placental microbiome among subjects with antepartum infections or subjects with spontaneous preterm delivery (<37 weeks’ gestation). In the previous study, after we identified subjects with any antepartum infections or spontaneous preterm birth, we then queried the database for 1:1 matches for women without antepartum infection or preterm birth. As described in our previous publication, the placental samples from 48 women were selected randomly from the overall case-cohort to undergo whole-genome shotgun sequencing. Approximately one-fourth of these samples were from the preterm birth case-cohort; one-fourth of the samples were from the remote antenatal infection case-cohort, and the remaining one-half of the samples were from the control subjects. Of note, the nested 48 subjects did not significantly differ from their derived cohort counterparts by any measure other than their case definition (not for maternal age, mode of delivery, race/ethnicity, BMI, maternal comorbidities, or tobacco use; Student t test on continuous variables and chi-squared analysis for categoric variables with lack of significance designated to be P > .05; 7). The present study used women (n = 237 of 320) for whom pregestational weight or early gestational weight was documented.
Metagenomic DNA extraction and sequencing
All samples were collected under uniform protocol (PeriBank) by placental pathology-trained personnel who wore facial masks and gloves, as described earlier. Approximately 100-150 mg of frozen placental tissue were taken from each sample and were homogenized and heated then processed with the use of PowerSoil DNA Isolation Kits (MO-BIO Laboratories, Carlsbad, CA). Briefly, genomic DNA was isolated according to the standard protocol from the MO-BIO PowerSoil DNA Isolation Kit (MO-BIO Laboratories). DNA was quantified by Nanodrop assay (Thermo Fisher Scientific, Wilmington, DE) and Picogreen assay (Invitrogen, Carlsbad, CA) and extracted DNA samples were stored at –20°C. Bacterial genomic DNA that had been isolated from placental samples was sheared to approximately 700 base pairs, and Illumina adapters (Illumina Inc, San Diego, CA) were ligated to the sheared fragments for whole genome shotgun sequencing, and analysis. DNA fragments were then paired-end sequenced (150 base pair [bp] reads) in multiplexed pools on the Illumina HiSeq 2500 platform (Illumina Inc) in the Baylor College of Medicine-Human Genome Sequencing Center, as described in our previous papers, including the parent publication on the placental microbiome. Because of the concern for bias in low-abundance samples, all specimens were thrice extracted and thrice run to enable triplicate extraction and sequencing. Sequences from all 3 extractions were combined for analysis.
16S recombinant DNA-based metagenomic sequencing and data analysis
Variable region amplicons of the bacteria 16S rRNA gene were sequenced as previously described. For whole genome shotgun sequencing and analysis, bacterial genomic DNA isolated from placental samples was sheared to approximately 700 bp and Illumina adapters were ligated to the sheared fragments. DNA fragments were then paired-end sequenced (150 bp reads) in multiplexed pools on the Illumina HiSeq 2500 platform. From 30 ng/μL of DNA, variable region amplicons were sequenced as previously described. The variable regions 1-3 of the 16S rRNA gene were amplified by polymerase chain reaction with bar-coded universal primers 27F (F, forward primer) and 534R (R, reverse primer) that contained linker sequences for 454-pyrosequencing. Samples that contained no template and those that contained known 16S rDNA sequences were used as positive and negative controls in these polymerase chain reaction steps. Variable-region amplicons were quantified by Picogreen assay and pooled in equimolar amounts. Multiplex sequencing was performed at the Baylor College of Medicine–Human Genome Sequencing Center on a 454-FLX Titanium sequencer (454 Life Sciences, Banford, CT). Raw 16S sequences were demultiplexed with quantitative insights into microbial ecology. Taxonomy was assigned with the use of the Ribosomal Database Project (RDP) classifier (version 2.2, re-trained with GreenGenes; Center for Microbial Ecology, Michigan State University, East Lansing, MI).
Concordant with previous work of ours and others, all 16S data were quality filtered to attain high-quality reads. Sequences from each file were preprocessed; thereby, sequences with length <200 nucleotides or >1000 nucleotides and sequences with minimum average quality score <20 and sequences without precisely matched 5′ primers were removed. Sequences were assigned thereafter to their sample of origin based on barcode matches and the 5′ primer, and the barcodes were excised after the filtering. RDP classifier (version 2.2 [re-trained using the release Feb. 4, 2011, of the GreenGenes taxonomy ]) was used to assign taxonomy for each read at various levels. We used reads with confidence level >50% at lowest level of assignment for further analysis. The read counts at each taxonomy level (phylum, order, family, genus) were normalized to relative abundance.
For operational taxonomic unit (OTU) analysis, quality, and RDP-filtered reads were analyzed with the quantitative insights into microbial ecology pipeline and AbundantOTU and to assign each read to OTUs. Quantitative insights into microbial ecology performed OTU picking, with error correction and OTU centroid choice at 97% similarity. ChimeraSlayer was used to identify and remove chimeric sequences from the following analyses: Artifact caused by sequencing error was removed with the use of AbundantOTU as a denoising approach. OTUs were analyzed by RDP classifier (version 2.2) and well to find out the corresponding taxonomy. The number of reads assigned to each OTU was counted and normalized. The generated OTU table, combined with the clinical metadata, was used as input for follow-up analysis.
Principal coordinate analysis (PCoA) was used to measure beta diversity on normalized OTU tables with Unifrac distance. Briefly, these plots demonstrate the degree to which each placenta’s microbiome differs from that of other placentas. With Unifrac, all taxa that are identified are placed on a phylogenetic tree. A branch that leads to taxa from both samples is marked as shared, and those taxa that appear only in one sample are marked as unshared. Distance in this sense is defined as the sum of unshared branch lengths over the sum of all branch lengths (both shared and unshared). Each sample is then plotted in its own dimension at the appropriate distance for each other point on the plot. The aggregate data from the 2 groups of placentas (obese or nonobese women or women with and without excess GWG, respectively) are used to calculate the overall degree of difference between the 2 groups, which is reflected in the permutational analysis of variance (PERMANOVA). Boruta feature selection and linear discriminate analysis effect size algorithms were used to detect significant features (taxonomy and pathway abundance) that differentiate groups and rank these features by effect size.
Whole genome shotgun metagenomic analysis
As anticipated, there was a large amount of human DNA sequence in our placental metagenomics datasets. Thus, to process the whole genome shotgun (WGS) reads, human contamination was identified and removed with Best Match Tagger according to the steps described in the HMP Human Sequence Removal Standard Operating Procedure and elsewhere. This ensured quality and privacy. Metabolic pathway reconstruction was inferred directly based on short reads with the HMP Unified Metabolic Analysis Network. Quality filtered reads were uploaded to metagenomic-RAST server (MG-RAST) for taxonomy identification with the use of WGS reads. Taxonomy assignment was based on best hit classification, with the use of the M5NR database as an annotation source with a maximum e-value cutoff of 1, minimum percent identity cutoff of 50%, and minimum alignment length cutoff of 15. MG-RAST was used to generate low level Kyoto Encyclopedia of Genes and Genomes (KEGG) pathway abundance for comparison between case and control-cohort samples. MG-RAST–generated taxonomy and pathway abundance were further normalized by the number of reads that were mapped to each sample. HUMAnN (the HMP Unified Metabolic Analysis Network) was used to KEGG-based community functions for metabolic reconstruction from WGS reads.
Metabolic pathway reconstruction directly inferred the KEGG-based community functions as described elsewhere. For linear discriminate analysis effect size analysis, the alpha value that was used for the factorial Kruskal-Wallis test was 0.05. The threshold used on the logarithmic linear discriminate analysis score for discriminative feature is 2.0, as we previously demonstrated.
Statistical analysis
Descriptive findings of clinical data were reported. Where appropriate, χ 2 testing was performed to assess categoric variables; the Student t test was performed on continuous variables with normal distribution, and the Wilcoxon rank-sum test was performed on continuous variables without normal distribution. A probability value of < .05 was considered statistically significant. Statistical analysis was performed with SPSS software (version 22.0; SPSS Incorporated, IBM Corp, Armonk, NY). The significance of PCoA plots were analyzed with PERMANOVA, which uses distance metrics to confirm the strength and statistical significance of sample groupings.
All sequencing files have been submitted to the Sequence Read Archive.
Results
Of the 320 women in our original data set, 237 women had sufficiently early prenatal care to calculate GWG; of these 237, 228 women (96.2%) had documented prepregnancy or <14-week maternal weight values. As shown in Figure 1 , we had 16S sequencing data on 237 women (144 women [60.8%] without excess GWG and 93 women [39.2%] with excess GWG) and WGS data on 37 women (24 women [64.9%] without excess GWG and 13 women [35.1%] with excess GWG). As shown in Table 3 , most of the women were Hispanic (85.6%) and multiparous (48.9%). Most of the subjects with excess GWG were obese or overweight at baseline ( P < .001), with an overall higher prepregnancy BMI (27.9 vs 30.2 kg/m 2 ; P = .007). However, among women who delivered preterm, there was no significant difference in BMI among subjects with excess GWG (mean BMI, 28.3 vs 30.6 kg/m 2 , respectively; P = .203; Table 3 ). Infant birthweight was statistically higher among women with excess GWG when compared with those without excess GWG by an average of 201.1 g ( P = .015). There were no statistically significant differences between excess and no excess GWG cohorts by race/ethnicity, mode of delivery, or other comorbidities or demographic variables. In total, 22.8% of women who were included in this study underwent cesarean delivery.
Demographic | Overall cohort (n = 237) | Gestational weight gain | P value | |
---|---|---|---|---|
Not excess (n = 144; 60.76%) | Excess (n = 93; 39.24%) | |||
Age, y a | 27.2 ± 6.0 | 27.3 ± 6.1 | 27.0 ± 5.9 | .640 |
Race/ethnicity, n (%) b | ||||
Hispanic | 202 (85.6) | 126 (88.1) | 76 (81.7) | |
Black | 26 (11.0) | 14 (9.8) | 12 (12.9) | |
White | 4 (1.7) | 1 (0.7) | 3 (3.2) | |
Other | 4 (1.7) | 2 (1.4) | 2 (2.2) | .380 |
Prepregnancy body mass index a,c | 28.8 ± 6.4 | 27.9 ± 6.4 | 30.2 ± 6.3 | .007 d |
Underweight | 3 ± 1.3 | 3 ± 2.1 | 0 | |
Normal | 66 ± 27.8 | 53 ± 36.8 | 13 ± 14.0 | |
Overweight | 84 ± 35.4 | 46 ± 31.9 | 38 ± 40.9 | |
Obese | 84 ± 35.4 | 42 ± 29.2 | 42 ± 45.2 | < .001 d |
Parity a | 1.6 ± 1.4 | 1.61 ± 1.4 | 1.48 ± 1.3 | .488 |
0, n (%) | 66 (27.8) | 37 (25.7) | 29 (31.2) | |
1, n (%) | 55 (23.2) | 36 (25.0) | 19 (20.4) | |
≥2, n (%) | 116 (48.9) | 71 (49.3) | 45 (48.4) | .569 |
Antenatal infection, n (%) | 78 (32.9) | 42 (29.2) | 36 (38.7) | .127 |
Sexually transmitted infection | 45 (19.0) | 24 (16.7) | 21 (22.6) | .257 |
Urinary tract infection | 42 (17.7) | 20 (13.9) | 22 (23.7) | .055 |
Other | 5 (2.1) | 3 (2.1) | 2 (2.2) | .972 |
Streptococcus agalactiae positive, n (%) | 47 (21.9) | 30 (22.9) | 17 (20.2) | .645 |
Delivery | ||||
Infant birthweight, g a | 3173.8 ± 618.0 | 3095.6 ± 597.7 | 3296.7 ± 632.6 | .015 d |
Gestational age at delivery, wk a | 38.2 ± 2.4 | 38.1 ± 2.5 | 38.5 ± 2.4 | .21 |
Preterm delivery, n (%) | 62 (26.2) | 42 (29.2) | 20 (21.5) | .190 |
Cesarean delivery, n (%) | 54 (22.8) | 33 (22.9) | 21 (22.6) | .952 |
Maternal comorbidities/outcomes, n (%) | ||||
Diabetes mellitus | 58 (24.5) | 38 (26.4) | 20 (21.5) | .393 |
Uncontrolled diabetes mellitus | 10 (4.2) | 5 (3.5) | 5 (5.4) | .476 |
Hypertensive disorder | 7 (3.0) | 5 (3.5) | 2 (2.2) | .557 |
b One woman did not report her race/ethnicity
c When the prepregnancy body mass index was examined among preterm deliveries, there was no difference in the prevalence of obesity among women with excess gestational weight gain; additionally, the mean body mass index was 28.3 ± 7.233 kg/m 2 vs 30.64 ± 5.355 kg/m 2 ( P = .203)
To investigate whether the placental microbiome varied with excess GWG, we compared the phylum-level relative abundance by BMI and then by excess GWG in all women. As shown in Figure 2 , A, and delineated in Table 4 , among all subjects there are no appreciable significant differences in the relative abundance of Proteobacteria, Firmicutes, Bacteroidetes, Actinobacteria, Cyanobacteria, Chloroflexi, Aquificae, nor Verrucomicrobia between either obese and nonobese subjects nor with and without excess GWG ( Figure 2 , A; Table 4 ). However, when we specifically examined phyla level relative taxonomic abundance among preterm women by virtue of maternal weight gain ( Figure 2 , A), we observed appreciable and significant increased abundance of Firmicutes, Actinobacteria, and Cyanobacteria ( Figure 2 , A; Table 4 ) and decreased relative abundance of Proteobacteria ( Figure 2 , A; Table 4 ). This was not unique to only phyla level relative taxonomic abundance, but also held true at the family ( Figure 2 , B) and genus level ( Vibrio, Burkholderia , and Beijerinckia ; Table 4 ). Although we could reach species-level resolution with our WGS data, we did not distinguish significantly distinct abundance of any given single species by virtue of GWG nor obesity (data not shown). Collectively, these findings are consistent with composite genus level and courser distinctions among preterm placental microbiome communities when comparing subjects with and without excess GWG, but not obesity per se.
Variable | Term gestational weight gain abundance a | Preterm gestational weight gain abundance a | P value b | ||||||
---|---|---|---|---|---|---|---|---|---|
No excess | Excess | No excess | Excess | ||||||
Phyla | Proteobacteria | 0.529345 | Proteobacteria | 0.569424 | Proteobacteria | 0.587677 | Proteobacteria c | 0.51827 c | .013 c |
Actinobacteria | 0.176771 | Actinobacteria | 0.157384 | Actinobacteria | 0.17258 | Actinobacteria c | 0.198641 c | .037 c | |
Firmicutes | 0.12791 | Firmicutes | 0.12825 | Firmicutes | 0.103855 | Firmicutes c | 0.146677 c | .028 c | |
Bacteroidetes | 0.089904 | Bacteroidetes | 0.071546 | Bacteroidetes | 0.079576 | Bacteroidetes | 0.07039 | .351 | |
Cyanobacteria | 0.030468 | Cyanobacteria | 0.026107 | Cyanobacteria | 0.015286 | Cyanobacteria c | 0.02311 c | .011 c | |
Genus | Escherichia | 0.067054 | Escherichia | 0.121517 | Escherichia | 0.117615 | Escherichia | 0.090976 | .148 |
Vibrio | 0.051607 | Vibrio | 0.053834 | Vibrio | 0.098668 | Vibrio c | 0.073517 c | .022 c | |
Burkholderia | 0.04352 | Streptomyces | 0.038562 | Burkholderia | 0.05502 | Streptomyces | 0.049689 | .493 | |
Streptomyces | 0.042644 | Burkholderia | 0.034571 | Streptomyces | 0.049596 | Burkholderia c | 0.04134 c | .040 c | |
Bacteroides | 0.040631 | Bacteroides | 0.027125 | Bacteroides | 0.035619 | Bacteroides | 0.027274 | .128 | |
Prevotella | 0.025092 | Prevotella | 0.025053 | Prevotella | 0.027245 | Prevotella | 0.026016 | .343 | |
Coprobacillus | 0.02033 | Neisseria | 0.021161 | Beijerinckia | 0.023128 | Mycobacterium | 0.020863 | .113 | |
Mycobacterium | 0.017375 | Shigella | 0.020963 | Pseudomonas | 0.017131 | Coprobacillus | 0.019918 | .201 | |
Neisseria | 0.016759 | Coprobacillus | 0.018835 | Neisseria | 0.01678 | Clostridium | 0.018003 | .109 | |
Corynebacterium | 0.016255 | Mycobacterium | 0.017239 | Bacillus | 0.016083 | Beijerinckia c | 0.01687 c | .024 c | |
Species | Escherichia coli | 0.062505 | Escherichia coli | 0.113882 | Escherichia coli | 0.110747 | Escherichia coli | 0.086405 | .294 |
Vibrio sp AND4 | 0.044276 | Vibrio sp AND4 | 0.046344 | Vibrio sp AND4 | 0.089599 | Vibrio sp AND4 | 0.06796 | .081 | |
Coprobacillus sp D7 | 0.020276 | Prevotella tannerae | 0.020934 | Strep Avermitilis | 0.028329 | Strep Avermitilis | 0.029311 | .910 | |
Prevotella tannerae | 0.019398 | Coprobacillus sp D7 | 0.018469 | Bacteroides sp. D20 | 0.025244 | Prevotella tannerae | 0.028591 | .648 | |
Bacteroides sp D20 | 0.016667 | Bacteroides sp D20 | 0.017344 | Prevotella tannerae | 0.024715 | Bacteroides sp D20 | 0.026731 | .885 | |
Beijerinckia indica | 0.014912 | Beijerinckia indica | 0.015506 | Beijerinckia indic | 0.023128 | Coprobacillus sp D7 | 0.025636 | .265 | |
Strep. Avermitilis | 0.014021 | Strep. Avermitilis | 0.015452 | Burk. Cepacia | 0.019766 | Beijerinckia indica | 0.025358 | .795 | |
Salmonella enterica | 0.010835 | Salmonella enterica | 0.013655 | Burk. Pseudomallei | 0.017585 | Burk Cepacia | 0.023036 | .807 | |
Burk. Pseudomallei | 0.010509 | Steno Maltophilia | 0.01165 | Coprobacillus sp D7 | 0.013868 | Prop acnes | 0.020665 | .450 | |
Bacteroides helcogenes | 0.009771 | Burk. Pseudomallei | 0.010584 | Prop Acnes | 0.013009 | Salmonella enterica | 0.019542 | .358 |
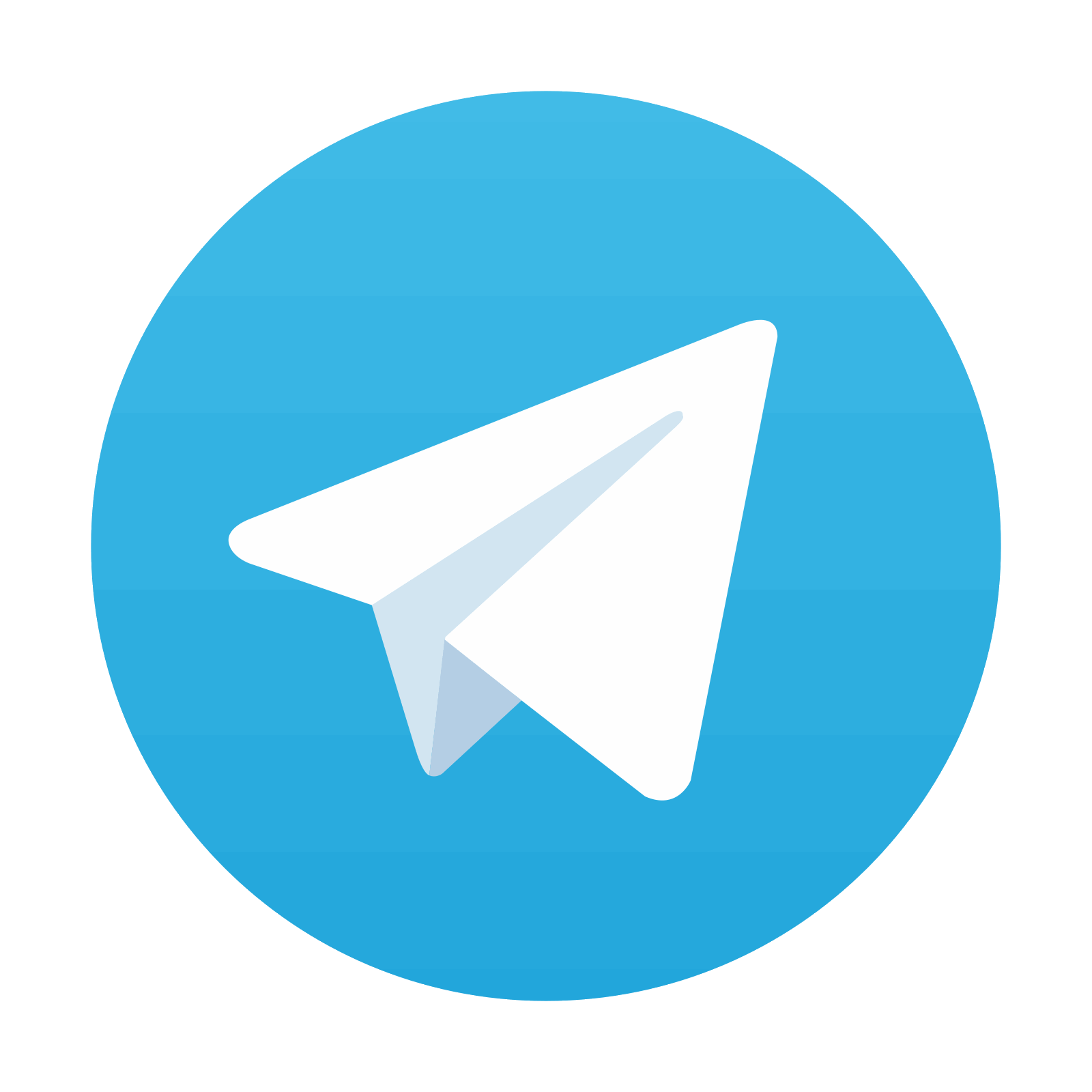
Stay updated, free articles. Join our Telegram channel

Full access? Get Clinical Tree
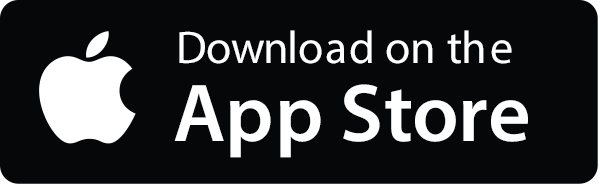

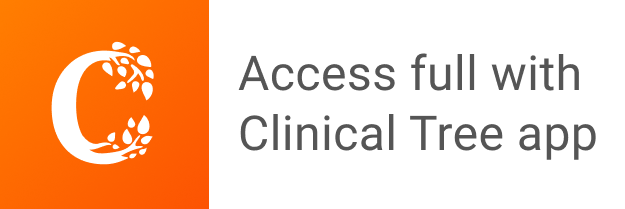