Objective
‘Omics analysis of large datasets has an increasingly important role in perinatal research, but understanding gene expression analyses in the fetal context remains a challenge. We compared the interpretation provided by a widely used systems biology resource (ingenuity pathway analysis [IPA]) with that from gene set enrichment analysis (GSEA) with functional annotation curated specifically for the fetus (Developmental FunctionaL Annotation at Tufts [DFLAT]).
Study Design
Using amniotic fluid supernatant transcriptome datasets previously produced by our group, we analyzed 3 different developmental perturbations: aneuploidy (Trisomy 21 [T21]), hemodynamic (twin-twin transfusion syndrome [TTTS]), and metabolic (maternal obesity) vs sex- and gestational age-matched control subjects. Differentially expressed probe sets were identified with the use of paired t -tests with the Benjamini-Hochberg correction for multiple testing ( P < .05). Functional analyses were performed with IPA and GSEA/DFLAT. Outputs were compared for biologic relevance to the fetus.
Results
Compared with control subjects, there were 414 significantly dysregulated probe sets in T21 fetuses, 2226 in TTTS recipient twins, and 470 in fetuses of obese women. Each analytic output was unique but complementary. For T21, both IPA and GSEA/DFLAT identified dysregulation of brain, cardiovascular, and integumentary system development. For TTTS, both analytic tools identified dysregulation of cell growth/proliferation, immune and inflammatory signaling, brain, and cardiovascular development. For maternal obesity, both tools identified dysregulation of immune and inflammatory signaling, brain and musculoskeletal development, and cell death. GSEA/DFLAT identified substantially more dysregulated biologic functions in fetuses of obese women (1203 vs 151). For all 3 datasets, GSEA/DFLAT provided more comprehensive information about brain development. IPA consistently provided more detailed annotation about cell death. IPA produced many dysregulated terms that pertained to cancer (14 in T21, 109 in TTTS, 26 in maternal obesity); GSEA/DFLAT did not.
Conclusion
Interpretation of the fetal amniotic fluid supernatant transcriptome depends on the analytic program, which suggests that >1 resource should be used. Within IPA, physiologic cellular proliferation in the fetus produced many “false positive” annotations that pertained to cancer, which reflects its bias toward adult diseases. This study supports the use of gene annotation resources with a developmental focus, such as DFLAT, for ‘omics studies in perinatal medicine.
Click Supplementary Content under the article title in the online Table of Contents
The growing awareness of the impact of the in utero environment on life-long health has coincided with the recognition of the ability to obtain real-time information about fetal development from cell-free fetal RNA in amniotic fluid. The amniotic fluid transcriptome has been used by our group and others to obtain valuable information about fetal development in a variety of health and disease states. The literature on fetal molecular biology has expanded exponentially in recent years, with an increasing focus on a variety of fetal transcriptomic studies. The need to adapt or customize transcriptomic bioinformatics analysis to obtain more relevant and interpretable output has been recognized by a wide variety of other disciplines that range from researchers studying breast cancer to chicken models of disease. Obstetrician-gynecologists face unique issues in interpreting ‘omics data, because the performance of widely used systems of biology analytic resources has never been specifically evaluated for application in the fetus or placenta. Members of our group previously have addressed the need for more fetal-focused gene expression analytic tools by adding human-specific, developmentally relevant annotation to the Gene Ontology (GO) database and maintaining a collection of gene sets that are tailored for use in studying human development, called “Developmental FunctionaL Annotation at Tufts” (DFLAT) ( http://dflat.cs.tufts.edu ). Using these gene sets in the Gene Set Enrichment Analysis (GSEA/DFLAT), we sought to compare the interpretation provided by this publicly available fetus-specific functional annotation with that of a commercially available widely used functional analytic tool, Ingenuity Pathway Analysis (IPA).
Materials and Methods
To compare the functional analytic output of GSEA/DFLAT vs IPA, we performed an in silico experiment that used 3 amniotic fluid supernatant (AFS) transcriptome datasets previously produced by our group and publicly available in the Gene Expression Omnibus (GSE16176, GSE47393, GSE48521). These datasets represent 3 different developmental perturbations in second-trimester fetuses: aneuploidy (Trisomy 21 [T21]), hemodynamic (twin-twin transfusion syndrome [TTTS]), and metabolic (maternal obesity [MAT OB]). Each dataset contains information that was obtained from cell-free RNA in AFS from 14-16 fetuses. Within each dataset, cases were matched to control subjects for gestational age and fetal sex, both of which have been demonstrated to influence fetal gene expression. There was no pooling of samples.
The original amniotic fluid samples for these studies were collected with human subject approval from the Institutional Review Board at Tufts Medical Center and from each of the participating centers. Subjects signed informed consent for amniocentesis that was performed for routine clinical indications. Details of subject recruitment and sample collection, RNA extraction, amplification, and microarray hybridization have been described previously. All studies used the same whole genome expression array (Affymetrix HGU133 Plus 2.0; Affymetrix, Santa Clara, CA). The matched case and control gene expression data, experimental conditions, and data normalization methods are publicly available in the associated Gene Expression Omnibus records. Microarray data for all 3 datasets were normalized with the 3-step command from the affyPLM package in Bioconductor, with the use of the ideal-mismatch background-signal adjustment, quantile normalization, and the Tukey biweight summary method. This summary method includes a logarithmic transformation to improve the normality of the data. Identification of differentially regulated probe sets in cases vs control subjects was performed via 2-sided paired t -tests, with the Benjamini Hochberg (BH) adjustment for multiple testing. BH- P < .05 was defined as significant. Three working files that contained significantly differentially regulated probe sets were generated to perform the IPA analyses on the 3 datasets. Supplementary Table 1 ( Appendix ) contains the “working files” for the IPA analyses.
Functional genomic analysis
Functional analyses were performed with the IPA “Core Analysis” function (content version 18841524, release date 6/24/13) and GSEA, using the DFLAT-augmented Gene Ontology Biological Process gene sets. Outputs were compared for biologic relevance to the fetus.
Within IPA, both up- and down-regulated probe sets were incorporated into the analysis. We considered pathways and functional annotations to be dysregulated significantly if they were associated with a right-tailed Fisher exact test with a probability value of < .01 or a bias-corrected absolute Z score of ≥2. Only those functional annotations or terms that were associated with ≥3 genes were considered in the IPA and GSEA/DFLAT analyses. Only the “Diseases & Functions” aspect of the IPA analysis could be compared directly with the DFLAT/GSEA analysis, given that there is no direct GSEA correlate for IPA’s Canonical Pathways, Upstream Analysis, Regulator Effects, and Networks analysis modes. For this in silico experiment, we focused on the “Diseases and Functions” “Canonical Pathways,” and “Upstream Analysis” modes within IPA.
In the Canonical Pathways function of IPA, pathways were considered to be dysregulated significantly if they were associated with a right-tailed Fisher exact test probability value of < .01. The Upstream Analysis feature of IPA was used to predict the activation or inhibition of transcriptional regulators that were based on the direction of gene expression changes in our dataset. We defined upstream regulators as significantly activated or inhibited if the activation Z-score was ≥2.0 or ≤–2.0, in accordance with recommended thresholds.
The combined DFLAT and GO annotations of human genes can be downloaded as gene sets formatted for use in GSEA. The DFLAT annotation contains 13,344 new terms to use in conjunction with the existing GO annotation. The derivation and validation of DFLAT has been described in detail in a previous publication. Briefly, DFLAT was created via manual curation from the literature with the use of the Protein2GO curation tool and the methods of the GO Consortium and GO Non-Eligible annotations and mouse-to-human orthologs-derived annotations. DFLAT was then validated with the use of both external datasets and those that were produced by our own laboratory.
For all analyses, the Java implementation of GSEA (version 2-2.07) was run in batch mode. GSEA was run with the use of the preranked option, which ranks by paired t -scores, for greater consistency with the IPA input and to preserve the original matching of AFS case and control samples for gestational age and fetal sex. Gene sets were considered to be dysregulated significantly if they were associated with false discovery rate q-values of <0.25, in accordance with recommended stringency thresholds. We extended the analysis to include gene sets with raw probability values of < .01, given controversy about adjustment for multiple testing between highly overlapping gene sets.
Because the Gene Sets in the DFLAT annotation of GO have different names than the categories and functional annotations within IPA, which compares the 2 outputs that are required to identify and categorize common and unique annotations. All significantly dysregulated gene sets and IPA annotation terms that were identified in the functional analysis of the 3 datasets were reviewed manually by the first author, and a list of 23 developmental categories and 443 associated keywords was created ( Supplementary Table 2 ). These categories and keywords encompassed significantly dysregulated annotations within IPA Molecular and Cellular Functions, Physiological System Development and Function, and Diseases and Disorders categories, in addition to unique Gene Set categories within GSEA/DFLAT that did not correspond to any categories within IPA. We wrote Perl scripts to count the number of terms within each category that was identified by DFLAT/GSEA vs IPA. Outputs were reviewed manually for accuracy. A category was designated as “common to both” GSEA/DFLAT and IPA if there were at least 5 significantly dysregulated functional annotations or terms in that category for each analysis. A category was determined to be “more frequent” in IPA or GSEA/DFLAT if there were at least 5 significantly dysregulated functional annotations or terms in that category and if there was at least a 3-fold difference between the 2 methods for that category.
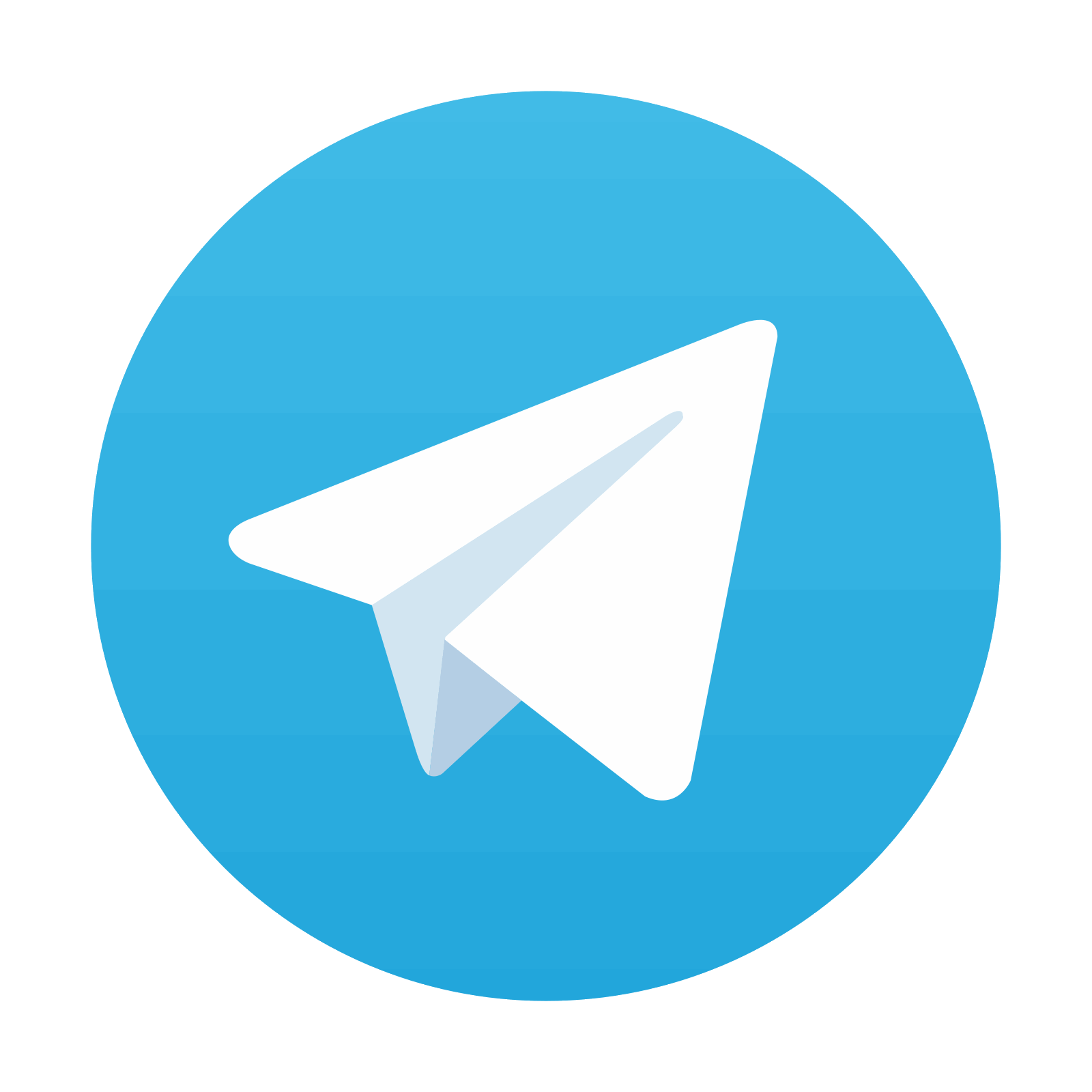
Stay updated, free articles. Join our Telegram channel

Full access? Get Clinical Tree
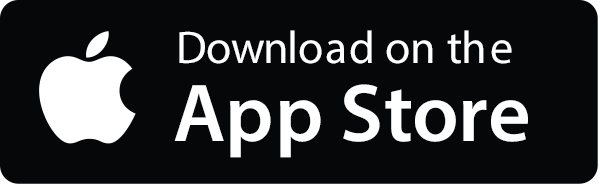
