The established method of the assessment of the risk for development of preeclampsia is to identify risk factors from maternal demographic characteristics and medical history; in the presence of such factors, the patient is classified as high risk and in their absence as low risk. Although this approach is simple to perform, it has poor performance of the prediction of preeclampsia and does not provide patient-specific risks. This review describes a new approach that allows the estimation of patient-specific risks of delivery with preeclampsia before any specified gestational age by maternal demographic characteristics and medical history with biomarkers obtained either individually or in combination at any stage in pregnancy. In the competing risks approach, every woman has a personalized distribution of gestational age at delivery with preeclampsia; whether she experiences preeclampsia or not before a specified gestational age depends on competition between delivery before or after the development of preeclampsia. The personalized distribution comes from the application of Bayes theorem to combine a previous distribution, which is determined from maternal factors, with likelihoods from biomarkers. As new data become available, what were posterior probabilities take the role as the previous probability, and data collected at different stages are combined by repeating the application of Bayes theorem to form a new posterior at each stage, which allows for dynamic prediction of preeclampsia. The competing risk model can be used for precision medicine and risk stratification at different stages of pregnancy. In the first trimester, the model has been applied to identify a high-risk group that would benefit from preventative therapeutic interventions. In the second trimester, the model has been used to stratify the population into high-, intermediate-, and low-risk groups in need of different intensities of subsequent monitoring, thereby minimizing unexpected adverse perinatal events. The competing risks model can also be used in surveillance of women presenting to specialist clinics with signs or symptoms of hypertensive disorders; combination of maternal factors and biomarkers provide patient-specific risks for preeclampsia that lead to personalized stratification of the intensity of monitoring, with risks updated on each visit on the basis of biomarker measurements.
Click Supplemental Materials under article title in Contents at ajog.org
Identification of pregnancies at high risk of the development of preeclampsia is beneficial because therapeutic interventions in such pregnancies, which include prophylactic use of aspirin, closer surveillance, and earlier delivery, can reduce the incidence of the disease and/or its associated maternal and perinatal complications.
Why was this study conducted?
The purpose of this study was to explain the competing risks approach for the prediction of patient-specific risks of delivery with preeclampsia.
Key findings
The competing risks approach is based on a survival-time model for the gestational age at delivery with preeclampsia. Every pregnant woman has a personalized distribution of gestational age at delivery with preeclampsia that comes from the application of Bayes theorem to combine a prior distribution, which is determined from maternal demographic characteristics and medical history, with likelihoods from biomarkers. This approach allows the estimation of the individual patient-specific risks of delivery with preeclampsia before any specified gestational age by maternal factors and biomarkers that are obtained either individually or in combination at any stage in pregnancy.
What does this add to what is known?
The competing risks approach is superior to the established method of classifying women as high or low risk based on the presence or absence of risk factors from maternal demographic characteristics and medical history.
This review describes a new approach of assessing the risk for the development preeclampsia. The competing risks approach is based on a survival-time model that allows an estimation of the individual patient-specific risks of delivery with preeclampsia before any specified gestational age by a combination of maternal demographic characteristics and medical history with biomarkers that are obtained either individually or in combination at any stage in pregnancy.
Prediction of Preeclampsia
Prediction by risk scoring systems
The established method of assessing the risk for development of preeclampsia is to identify risk factors from maternal demographic characteristics and medical history; in the presence of such factors, the patient is classified as high risk and, in their absence, as low risk. , In the United Kingdom, according to guidelines by the National Institute for Health and Clinical Excellence, women should be considered to be at high risk of the development of preeclampsia if they have any 1 high-risk factor or any 2 moderate-risk factors. The high-risk factors are history of hypertensive disease in previous pregnancy, chronic kidney disease, autoimmune disease, diabetes mellitus, or chronic hypertension; the moderate-risk factors are first pregnancy, age ≥40 years, interpregnancy interval >10 years, body mass index at first visit of ≥35 kg/m 2 , or family history of preeclampsia. A similar approach was recommended recently by The American College of Obstetricians and Gynecologists and the Society for Maternal–Fetal Medicine: the high-risk factors were identical to those of National Institute for Health and Clinical Excellence, and the moderate-risk factors were first pregnancy, age ≥35 years, interpregnancy interval >10 years, body mass index >30 kg/m 2 , family history of preeclampsia, black race, or low socioeconomic status and history of low birthweight or adverse pregnancy outcome.
The advantage of these approaches is that they are simple to perform; however, the disadvantages are that (1) they have poor performance of the prediction of preeclampsia , and (2) they do not quantify individual patient-specific risks.
Prediction by probability models
Another approach of assessing the risk for the development of preeclampsia is to use probabilistic models that treat preeclampsia as a binary outcome. This approach involves different models for early, late, or all preeclampsia and includes the use of logistic regression models. These models use maternal characteristics and medical history alone or in combination with biomarkers to quantify the individual patient-specific risk for preeclampsia, rather than just classifying women into high- and low-risk groups. However, they do not allow the flexibility of selecting different gestational age cut-offs for the categorization of the severity of preeclampsia; they do not take into account the increasing effect size on biomarkers with severity of the disease, and they cannot be expanded easily to include additional biomarkers that are measured at different stages in pregnancy.
Prediction by the competing risks approach
Personalized distribution of gestational age at delivery with preeclampsia
Although other approaches to prediction treat preeclampsia as a binary outcome, the competing risk model , treats preeclampsia as an event in time. Risks of delivery with preeclampsia, assuming no other cause delivery, are determined from a personalized distribution of gestational age at delivery with preeclampsia ( Figure 1 ). These are given by the area under the probability density curve 1 or the height of the cumulative risk curve. The cumulative risk curve shows the area under the probability density curve as a function of gestational age at delivery. Figure 1 shows the survival curve that gives the probability of the pregnancy continuing without delivery because of preeclampsia. For any given gestation, the cumulative risk and the survival probabilities add to 1.0. It is notable that the distribution that is shown attaches probabilities well beyond the gestational ages at which pregnancies are delivered. This reflects the fact that most pregnancies deliver without preeclampsia because of other causes, predominantly normal delivery, that compete with preeclampsia. The part of the distribution beyond 41 +3 weeks gestation is irrelevant to the calculation of risks.

Model specification
For implementation, we adopt the same approach to that taken in risk assessment for aneuploidies ; the prediction model is specified in terms of a prior distribution from maternal characteristics and likelihood functions from biomarker measurements. Data from biomarker measurements are used to update the prior distribution with the use of the Bayes theorem to produce a posterior distribution. Although risk assessment for aneuploidies concerns prediction of an unknown karyotype, the competing risk model for preeclampsia concerns the prediction of gestation at delivery with preeclampsia.
By chaining Bayes theorem, the posterior distribution can be updated as new information becomes available at different stages in pregnancy. This way of specifying the model therefore provides a framework for dynamic prediction. It also allows the different marker combinations to be used within the same underlying model and new markers to be included without the need for a completely new model.
Prior model based on maternal factors
The prior model, which was based on maternal characteristics and medical history, was derived from a study of 120,492 singleton pregnancies that underwent a routine ultrasound examination at 11–13 weeks gestation. The model was fitted to data on gestational age (in weeks) at the time of delivery with preeclampsia with the use of a parametric survival model in which deliveries from other causes were treated as censored observations. Various parametric models for the time to delivery with preeclampsia were considered, and a Gaussian model was chosen on the basis of goodness of fit and simplicity of interpretation. In this model, the mean gestational age at delivery with preeclampsia for a reference population (white race; age, <35 years; weight, 69 kg; height, 164 cm; nulliparous; spontaneous conception; no family history of preeclampsia, and no history of diabetes mellitus, systemic lupus erythematosus, or antiphospholipid syndrome) is 54.4 weeks. The standard deviation is 6.8833. The risks of delivery with preeclampsia at <34, <37, and <42 weeks gestation are 0.15%, 0.58%, and 3.6%, respectively ( Figure 1 ).
Risk of development of preeclampsia was increased with advancing maternal age, increasing weight, black and South Asian racial origin, medical history of chronic hypertension, diabetes mellitus and systemic lupus erythematosus or antiphospholipid syndrome, conception by in vitro fertilization, family history of preeclampsia, and personal history of preeclampsia ( Figure 2 ); in the latter group, the risk was related inversely to the gestational age at delivery of the previous pregnancy. The risk for preeclampsia decreased, with consequent shift to the right in the distribution of the gestational age at delivery with preeclampsia, with increasing maternal height and in parous women with no previous preeclampsia; in the latter group, the maximum protective effect was when the interval between the current and previous pregnancy was 1-2 years, but the beneficial effect persisted for >15 years.

Twin pregnancies
In twin pregnancies, the incidence of preeclampsia is approximately 9%, which is 3 times higher than in singleton pregnancies. However, twins are delivered at an earlier gestational age than singletons; consequently. comparison of the overall rates of preeclampsia between twin and singleton pregnancies underestimates the relative risk of preterm-preeclampsia in twins, which is 9-times higher than in singletons. We have extended the prior model to include twins by lowering the prior mean for singletons by a twin effect that increases in magnitude with the singleton prior mean.
Biomarkers
Several studies have investigated the value of potential biomarkers for the prediction of preeclampsia. Those found to be useful at 11–13 and 19–24 weeks gestation are mean arterial pressure (MAP), uterine artery pulsatility index (UtA-PI), and placental growth factor (PLGF), in the early third trimester are MAP, UtA-PI, PLGF and serum soluble fms-like tyrosine kinase-1 (sFLT-1), and in late third trimester are MAP, PLGF, and sFLT-1. ,
Standardization: multiples of the median
In general, biomarker levels depend on gestational age, weight, and race, method of conception, medical conditions, and elements from the obstetric history that are associated with the individual on whom they are measured. They are also affected by the instrument that is used for measurement. The conventional method of standardization that is applied in screening is to express the measurements as multiples of the median (MoM) values that are specific to the individual from whom the measurements were taken. For MAP, UtA-PI, PLGF, and sFLT-1, MoM values are obtained from regression models of log transformed biomarker measurements. They are therefore the antilogarithm of the errors or residuals from the fitted regression model.
Distribution of marker MoM values in unaffected pregnancies
We illustrate the distributions of MoM values for first-trimester markers MAP, UtA-PI, and PLGF using data on 35,948 singleton pregnancies that included 1058 pregnancies (2.9%) that experienced preeclampsia. As shown in Figure 3 , for first trimester, the distribution of MAP, UtA-PI, and PLGF biomarker MoM values is fitted by a log-Gaussian distribution. Figure 4 shows estimated median MoM values in unaffected pregnancies and in pregnancies that experienced preeclampsia. For unaffected pregnancies, there is little evidence of any substantive dependency on gestational age, maternal weight, racial origin, smoking status, or history of chronic hypertension.


Distribution of marker MoM values in pregnancies that develop preeclampsia
In pregnancies that experience preeclampsia, MoM values of MAP, UtA-PI, and sFLT-1 tend to be higher, and PLGF tends to be lower than in normal pregnancies ( Figure 4 ). The effect sizes increase with increasing severity of the disease, quantified by the gestational age at delivery. Figure 5 shows log 10 MoM values at 11–13 weeks gestation for pregnancies with preeclampsia by the gestational age at delivery with preeclampsia. For early gestations, the means deviate from zero according to a linear regression relationship. As the gestational age at delivery with preeclampsia increases, the linear relationship continues until the regression line intersects zero, beyond which the mean is zero, which corresponds to a normal outcome for which the median MoM value is 1.0. The decreasing effect with gestational age at delivery explains to some extent the reason that screening performance is superior for early and preterm preeclampsia than for term preeclampsia.

In the univariate case, with only 1 biomarker for a given MoM value of a biomarker, the likelihood of a particular value of gestational age at delivery with preeclampsia is given by the height of the Gaussian curve as illustrated for UtA-PI in Figure 6 . In the multivariate case, with ≥2 biomarkers, the likelihood is a multivariate Gaussian density with correlations among log transformed MoM values that reflect the association among markers. In principle, risks can be obtained from any combination of biomarkers that are measured at different visits. It is important to recognize that attendance at a visit at a particular gestational age makes the likelihood of delivery before that gestation zero so that, even if no measurements are taken, the presence at the visit is informative.

Posterior distribution
The posterior distribution of gestational age at delivery with preeclampsia is obtained with the use of the Bayes theorem by multiplying the previous probability density from maternal factors by the likelihood function from biomarker MoM values ( Figure 7 ). To complete the posterior density, the area under the curve is made 1.0 by multiplying by a normalizing constant. The area defining the risk can be computed for other gestational ages to produce a cumulative distribution of risks that can be used as an individualized risk profile ( Figure 7 ).

Further details and parameter estimates can be found in the Appendix . The risk calculator is available free of charge at fetalmedicine.org
Clinical Implementation of the Competing Risks Approach
The competing risk model can be used for precision medicine and risk stratification at different stages of pregnancy. The objective of screening in the first trimester is the identification of a high-risk group that would benefit from preventative therapeutic interventions. The objective of screening in the second and third trimesters is the identification of a high-risk group that would benefit from close monitoring for early diagnosis of preeclampsia, thereby minimizing unexpected adverse perinatal events.
First trimester
In the first trimester, the competing risks approach that uses maternal factors, MAP, UtA-PI, and PLGF (triple test) was used to identify women at high risk of experiencing preterm preeclampsia; at a 10% screen positive rate, 90% of cases of early preeclampsia and 75% of cases with preterm preeclampsia were predicted in both a training and 2 validation datasets. , , It was then demonstrated through a randomized trial that, in women at high-risk of preeclampsia, the administration of aspirin (150 mg/day from 11–4 until 36 weeks gestation) reduces the risk of early preeclampsia and preterm preeclampsia by approximately 90% and 60%, respectively, and length of stay in neonatal intensive care by approximately 70%. ,
Recording maternal characteristics and medical history, measurement of blood pressure, and hospital attendance at 11–13 weeks gestation for an ultrasound scan are an integral part of routine antenatal care in many countries. In contrast, measurements of serum PLGF and UtA-PI are not part of routine care and would be associated with an additional cost. We examined the possibility of carrying out first-stage screening in the whole population by some of the components of the triple test and proceeding to second-stage screening by the triple test only for a subgroup of the population that were selected on the basis of the risk derived from first-stage screening ( Figure 8 ). , On the basis of the results of first-stage screening, the population was divided into a low-risk, screen-negative group, and a higher-risk group in need of further testing. After such testing, the patients were again classified as screen-negative and screen-positive. We found that a similar screen-positive rate and detection rate can be achieved with a 2-stage strategy of screening, at substantially lower costs, than with carrying out screening with all biomarkers in the whole population.

Second or third trimester
The competing risks approach can be used for stratification into high-, intermediate-, and low-risk treatment groups. For example, women who attended a routine hospital visit at 20 weeks gestation were allocated to the high-risk group if their risk for preeclampsia at <32 weeks gestation was above a high-risk threshold; they were allocated to the low-risk group if their risk for preeclampsia at <36 weeks gestation was below a low-risk threshold ( Figure 9 ). The high-risk group, which should be very small (aproximately 1% of the total population) and contain almost all cases of preeclampsia at <32 weeks gestation, would require close monitoring for high blood pressure, proteinuria, and hepatic, renal, and hematologic disturbance at 24–31 weeks gestation. The intermediate-risk group together with the undelivered pregnancies from the high-risk group (approximately 10% of the total population), which would contain approximately 90% of preeclampsia at 32–35 weeks gestation, would have reassessment of risk at 32 weeks gestation to identify those who would require close monitoring at 32–35 weeks gestation. The low-risk group (approximately 90% of the total population) can be reassured that they are unlikely to experience preeclampsia at <36 weeks gestation. However, all women who remain pregnant will require reassessment of risk at 36 weeks gestation because the performance of screening at 20 weeks gestation for preeclampsia at ≥36 weeks gestation is poor.

Surveillance of high-risk pregnancies
Development of preeclampsia is preceded by a decrease in the maternal serum concentration of the angiogenic PLGF and increase in the level of antiangiogenic sFLT-1. In women presenting to specialist clinics with signs or symptoms of hypertensive disorders, the use of cut-offs on the concentration of PLGF or the ratio of the concentrations of sFLT-1 and PlGF have been used to predict the development of preeclampsia within the subsequent 1–4 weeks. , , , This approach has the advantage of simplicity in clinical implementation. However, it does not take into account the previous risk of the individual patient in the study population or the measurement of blood pressure at examination, which is a prerequisite in the diagnosis of preeclampsia, and ignores the effects of maternal characteristics and gestational age on the measured serum concentrations.
An alternative approach for the prediction of preeclampsia at predefined intervals from assessment is the use of the competing risks model to derive patient-specific risks for preeclampsia by various combinations of maternal factors with MoM values of biomarkers, including PLGF, sFLT-1, and MAP. , , , In a large prospective observational study, we found that the performance of such approach is superior to that of PLGF alone or the sFLT-1/PLGF ratio. The competing risks model provides a personalized risk for delivery with preeclampsia that could lead to personalized stratification of the intensity of monitoring, with risks updated on each visit on the basis of biomarker measurements.
Validation
The competing risk model for use in first-trimester screening has been validated prospectively in 2 studies. In these studies, risks were produced, blinded to outcome with the use of a prespecified algorithm in 25,226 pregnancies, which included 712 pregnancies with preeclampsia of which 201 pregnancies were delivered at <37 weeks gestation and 84 pregnancies were delivered at <34 weeks gestation. Performance was assessed by (1) the ability of the model to discriminate between the preeclampsia and no-preeclampsia groups and (2) calibration, which assesses agreement between predicted risks and outcomes; for a well-calibrated model, among those women with a risk of 1 in n, the incidence should be 1 in n. Performance in the validation data sets were consistent with those from the training data set ( Figure 10 ), and there was good agreement between predicted risk and observed incidence ( Figure 11 ).
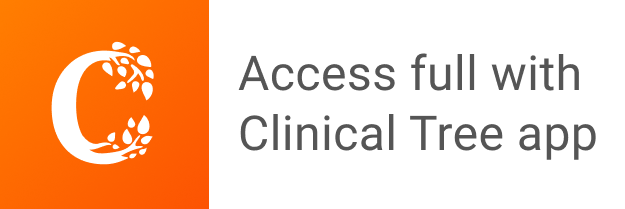