Materials and Methods
Study population
The Montreal Preeclampsia Study was nested within the established Montreal Prematurity Study, a population-based cohort of >5000 women. The McGill Faculty of Medicine Institutional Review Board approved the current study in November 2012. Consecutive pregnant women up to 24 weeks of gestation were recruited during 1999 through 2004 from 4 participating hospitals in Montreal, Quebec, Canada. At the time of recruitment to the cohort, participants were asked to provide separate consent for the Montreal Preeclampsia Study.
Inclusion criteria were age ≥18 years, fluency in English or French, and plan to deliver at a study hospitals. Women were excluded if they had any preexisting chronic conditions, except for diabetes, hypertension, or asthma. Women diagnosed with fetal abnormality, multifetal gestation, or history of incompetent cervix were also excluded.
All participants attended a study visit at 24–26 weeks’ gestation. A baseline questionnaire that assessed demographic, medical, obstetrical, and family history was administered by a research nurse, and a nonfasting blood sample was obtained for later analysis of case and control samples.
Case and control selection
Preeclampsia cases and controls were identified at the time of delivery. Each woman identified by the case room obstetrician, nurse, or self-report as having had hypertension or preeclampsia during pregnancy had her chart reviewed independently by 2 physicians using a standardized checklist to ensure that the definition of preeclampsia was consistent with the Canadian Hypertension Society Consensus Conference definition. The current version of these guidelines is largely consistent with the previous version employed in this study, as well as with the recently updated American Congress of Obstetrics and Gynecology guidelines.
Preeclampsia was defined in 1 of 4 ways: (1) gestational hypertension without proteinuria but with adverse conditions; (2) gestational hypertension with proteinuria but without adverse conditions; (3) gestational hypertension with both proteinuria and adverse conditions; (4) preexisting hypertension and superimposed gestational hypertension with proteinuria (worsening blood pressure in pregnancy with proteinuria >3 g/d >20 weeks).
Gestational hypertension was defined as diastolic hypertension ≥90 mm Hg measured on 2 occasions at least 4–6 hours apart >20 weeks’ gestation. Proteinuria was defined as 24-hour urine protein excretion of ≥0.3 g/d or positive dipstick result ≥2. Adverse conditions included convulsions, diastolic blood pressure >110 mm Hg, low platelet count (<100,000 × 10 9 /L), oliguria, protein excretion >3 g/d, pulmonary edema, elevated liver enzymes, severe nausea and vomiting, frontal headache, visual disturbances, persistent abdominal pain in upper right quadrant, chest pain or shortness of breath, suspected abruptio placentae, HELLP syndrome (hemolysis, elevated liver enzymes, and low platelet count), intrauterine growth restriction, oligohydramnios, and absent or reverse umbilical artery end-diastolic flow (detected by Doppler). Severe preeclampsia was defined as the presence of ≥1 of the following: seizures, HELLP syndrome, proteinuria >3 g/d, or diastolic blood pressure >110 mm Hg. Early-onset preeclampsia was defined as preeclampsia diagnosed <34 completed weeks of gestation.
Controls were selected from study women who delivered closest in time to each case at the same hospital and included women who delivered preterm, to represent the population that gave rise to the cases. Whenever possible, 3 or 4 controls per case were identified among women who did not develop preeclampsia or gestational hypertension during pregnancy.
Exposure measurement
We measured midpregnancy levels of antioxidant micronutrients, capable of scavenging free radicals and inhibiting reactive oxygen species. Given our single nonfasting blood sample, we focused on lipid-soluble antioxidants that are generally present in measurable quantities in plasma, have limited diurnal variation, and change slowly over time. At the 24- to 26-week study visit, the research nurse drew blood into two 7-mL EDTA-containing tubes by venipuncture. Tubes were placed on ice and brought immediately to each hospital’s biochemistry laboratory where plasma was separated by centrifugation. Five aliquots of 1.0 mL of plasma were stored in 2-mL cryovials. These samples were stored for up to 1 week at –20°C. Each week samples were transported on dry ice to a central –80°C freezer for later analysis. Retinol, α-tocopherol, and carotenoids have been shown to be stable for at least 5 months at –20°C, and for 15 years at –70°C. Thus, we were confident that neither short-term storage (≤1 week) at –20°C, nor long-term storage (0–6 years) at –80°C would affect the sample stability.
Plasma α- and γ-tocopherol, α- and β-carotene, retinol, lycopene, lutein, anhydrolutein, and α- and β-cryptoxanthin concentrations were analyzed in a blinded manner by high-performance liquid chromatography according to the procedures of Talwar et al. The intraassay coefficients of variation were: retinol, 2.60%; α-tocopherol, 3.84%; γ-tocopherol, 4.43%; lycopene, 8.71%; α-carotene, 7.48%; and β-carotene, 5.23%. We adjusted α-tocopherol for cholesterol, since the ratio of α-tocopherol to cholesterol has been reported to be the most useful measurement of vitamin E status in plasma. There is no similar recommendation for γ-tocopherol in the literature, and γ-tocopherol was not correlated with cholesterol in study controls. Total cholesterol was determined using the enzymatic method of Allain et al.
Statistical analysis
Antioxidant levels were examined as continuous variables in all analyses. We assessed each antioxidant for the presence of extreme outliers, which were excluded only from models including the antioxidant in question. As some of the antioxidants had a skewed distribution, we report the median and interquartile range and compare cases and controls using the nonparametric Wilcoxon test.
We then transformed each biomarker value to a z-score (units correspond to population SD), based on the distribution of the marker or its log transformation if positively skewed, in controls ( Supplementary Table 2 ). We expected levels of these biomarkers to be correlated, owing to their derivation from common dietary sources. We assessed the correlations among the antioxidant biomarkers to rule out potential collinearity. If antioxidant levels were moderately correlated (r 2 > 0.3, based on a priori discussion among coauthors), the sum was used as the main predictor variable in further analyses. We also performed a principal components analysis based on the control sample to identify a set of uncorrelated factors (weighted sum of individual antioxidant components) to assess for association with preeclampsia.
We assessed the overall association between each antioxidant z-score as the exposure of interest and preeclampsia (yes/no) through (unconditional) logistic regression models. We estimated the odds ratio (OR) for a 1-U SD increment in the biomarker. We investigated potential nonlinear effects using fractional polynomials and found that none fit the data better than the linear model. We thus included the z-score as a linear term in all analyses (data not shown). In a secondary analysis, we examined early- and late-onset preeclampsia separately through multinomial logistic regression that estimates the relative risk ratio (RRR) for each outcome associated with a 1-U SD increase in each antioxidant biomarker.
We additionally used survival analysis to assess the association between antioxidant levels and the timing of onset of preeclampsia, based on the onset of sustained hypertension, proteinuria, and time of delivery. Physicians were instructed to report the gestational week when a sustained blood pressure increase was first identified and our algorithm most often used the gestational week in which sustained hypertension first arose to assign the week of preeclampsia onset. Details on the timing of onset algorithm are available in the Appendix ( Supplementary Table 1 ). To be able to carry out survival analysis in the context of case-control data, we applied inverse probability of selection weights to reconstruct a representation of the cohort from which the cases and controls were sampled. Since every case was selected, cases received a weight of 1. Only a sample of potential controls (441 of 4932) was selected for biomarker analysis after excluding ineligible subjects, ie, those who were lost to follow-up or developed gestational hypertension or preeclampsia. Therefore, all controls received a weight of 11.18. We used extended Cox proportional hazards models for the survival analysis. Pregnancies were censored at the time of delivery if the pregnancy did not result in preeclampsia. Preeclampsia after delivery is a rare occurrence, and we found no such cases after reviewing the medical records of study participants. We used the Schoenfeld residuals test to assess the proportional hazards assumption and plotted the scaled Schoenfeld residuals, which represent a smoothed function of the time-dependent log hazard ratio (HR) for a 1-U SD increase in the antioxidant level.
We considered the following known risk factors for preeclampsia to be potential confounders: maternal age; body mass index (BMI); parity (nulliparous yes/no); smoking status; preexisting medical conditions (diabetes, hypertension); maternal education, income, and marital status (proxies socioeconomic status); and geographic region of birth, and language spoken at home (proxies for maternal ethnicity). Data on potential confounding variables were obtained from the prenatal questionnaire.
We had some missing data for covariates, especially income and BMI. Based on the assumption that, conditional on other covariates, these data were missing at random (ie, their values did not depend on unobserved variables), we imputed values using the other data available. Multiple imputation using chained equations was used to estimate 50 complete datasets, according to the procedures described by White et al.
We determined that we had 90% power to detect a difference of 0.345 SD using a t test with a 2-sided type 1 error rate (α) of 5%. Analyses were carried out in Stata 11.2 (StataCorp, College Station, TX) and R version 3.0.2 (R Foundation for Statistical Computing, Vienna, Austria).
Results
Among the cohort participants, 113 cases of preeclampsia arose and 443 controls were selected. Two cases and 2 controls were missing antioxidant biomarker data and were excluded from the present analyses ( Figure ). In all, 32 (29%) cases met the definition for severe preeclampsia. In all, 36 (32%) cases delivered preterm, <37 weeks, compared to 19 (4%) controls.

As expected, cases had higher mean BMI, were more likely to be nulliparous and nonsmokers, and to have diabetes and chronic hypertension compared to controls. Cases also had lower educational attainment, on average, than controls ( Table 1 ).
Maternal characteristic a | Preeclampsia cases (n = 111) | Preeclampsia controls (n = 441) |
---|---|---|
Mean ± SD or n (%) b | Mean ± SD or n (%) | |
Maternal age, y | 29.3 ± 5.7 | 29.3 ± 5.4 |
BMI, kg/m 2 | 25.7 ± 5.5 | 23.8 ± 5.1 |
Underweight, <18.5 | 2 (2) | 37 (8) |
Normal, 18.5–<25 | 54 (49) | 250 (57) |
Overweight, 25–<30 | 29 (26) | 77 (17) |
Obese, ≥30 | 19 (17) | 52 (12) |
Missing | 7 (6) | 25 (6) |
Nulliparous | 86 (77) | 253 (58) |
Current smoker at 24–26 wk | 11 (10) | 70 (16) |
Language spoken at home | ||
French | 52 (47) | 280 (64) |
English | 20 (18) | 72 (16) |
Other | 39 (35) | 86 (20) |
Region of birth of mother | ||
North America/Europe/Australia | 84 (75) | 362 (82) |
Sub-Saharan Africa/Caribbean | 15 (14) | 31 (7) |
Latin America | 6 (5) | 19 (4) |
Asia | 4 (4) | 16 (4) |
Middle East | 3 (3) | 11 (3) |
Maternal education | ||
High school or less | 20 (18) | 65 (15) |
Partial college | 21 (19) | 68 (15) |
Completed college or some university | 41 (37) | 136 (31) |
University graduate or more | 29 (26) | 172 (39) |
Family income, $/y | ||
<15,000 | 5 (5) | 50 (11) |
15,000 to <30,000 | 13 (12) | 66 (15) |
30,000 to <50,000 | 26 (23) | 88 (20) |
50,000 to <80,000 | 26 (23) | 114 (26) |
≥80,000 | 23 (21) | 85 (19) |
Missing | 18 (16) | 38 (9) |
Living arrangement | ||
Legally married | 57 (52) | 182 (42) |
Cohabiting | 45 (41) | 208 (47) |
Neither | 7 (6) | 48 (11) |
Preexisting chronic conditions | ||
Hypertension | 9 (9) | 13 (3) |
Diabetes | 7 (7) | 3 (1) |
Asthma | 9 (8) | 45 (10) |
a Missing information: parity for 1 control, smoking for 3 controls, language for 3 controls, geographic region of birth for 2 controls, living arrangement for 2 cases and 3 controls, hypertension for 9 cases and 18 controls, diabetes for 8 cases and 34 controls, asthma for 1 control
Cases had significantly lower levels of α-carotene, β-carotene, lutein, and anhydrolutein than controls ( Table 2 ). However, several of the biomarkers were highly correlated ( Supplementary Table 3 ). Hence, we pooled α-carotene, β-carotene, anhydrolutein, α-cryptoxanthin, and β-cryptoxanthin. After summing the concentrations of these carotenoids, some residual correlation with lutein remained ( Supplementary Table 4 ).
Biomarker a | Cases (n = 111) | Controls (n = 441) | P value b |
---|---|---|---|
Median (IQR) | Median (IQR) | ||
α-Tocopherol | 17.8 (14.7–21.7) | 18.9 (16.1–22.2) | .07 |
γ-Tocopherol | 1.75 (1.18–2.72) | 1.73 (1.21–2.46) | .60 |
Retinol c | 0.38 (0.32–0.45) | 0.38 (0.32–0.44) | .57 |
Lycopene | 224 (102–317) | 232 (135–357) | .29 |
α-Carotene | 119 (72–205) | 154 (84–250) | .05 |
β-Carotene | 319 (180–571) | 405 (250–667) | .02 |
Lutein | 96 (67.1–133) | 118 (87–158) | <.01 |
Anhydrolutein | 63.9 (44.6–87.7) | 73.4 (52.4–97.0) | .01 |
α-Cryptoxanthin | 37.4 (29.2–56.9) | 43.0 (31.5–60.0) | .08 |
β-Cryptoxanthin | 92.3 (62.8–153) | 108 (66.4–184) | .08 |
a μg/mL for tocopherols and retinol and ng/mL for carotenoids
b P value for nonparametric Wilcoxon rank sum test
c n = 110 cases, excluded 1 outlier for retinol in case (retinol = 0.92).
According to the crude logistic regression analyses using the standardized z-score as the main predictor variable, corrected α-tocopherol (per mmol cholesterol), lutein, and carotenoids were all negatively associated with risk of preeclampsia ( Table 3 ). However, after adjusting for key confounders, only lutein remained statistically significantly associated with a reduced risk of preeclampsia. Our principal components analysis confirmed that, with the exception of lycopene, most of the carotenoids measured in our study were highly correlated ( Supplementary Table 5 ). The component dominated by the carotenoids was significantly associated with a reduced risk of preeclampsia ( Supplementary Table 6 ).
Biomarker | Crude OR (95% CI) | Adjusted OR (95% CI) a |
---|---|---|
α-Tocopherol | 0.82 (0.66–1.02) | 0.92 (0.72–1.17) |
α-Tocopherol/cholesterol | 0.75 (0.58–0.98) | 0.82 (0.65–1.04) |
Ln(γ-tocopherol) | 1.05 (0.85–1.30) | 1.01 (0.79–1.29) |
Retinol | 1.11 (0.90–1.35) | 1.13 (0.90–1.43) |
Ln(lycopene) | 0.89 (0.73–1.09) | 0.93 (0.75–1.16) |
Ln(lutein) | 0.59 (0.48–0.74) | 0.60 (0.46–0.77) |
Ln(carotenoids) b | 0.78 (0.64–0.97) | 0.82 (0.62–1.07) |
a Adjusted for diabetes, hypertension, body mass index, nulliparity, smoking, maternal education, living arrangement, region of birth, and language spoken at home
b Sum of α- and β- carotene, anhydrolutein, α- and β-cryptoxanthin.
When we separated early-onset (<34 weeks, n = 35) and late-onset (n = 76) preeclampsia, we observed stronger associations between each antioxidant and early-onset preeclampsia, with the exception of lycopene ( Table 4 ). Both positive (RRR >1; γ-tocopherol, retinol) and negative (RRR <1; α-tocopherol, lutein, carotenoids) associations arose. After adjustment for confounding, significant associations were observed between α-tocopherol:cholesterol and retinol with early- but not late-onset preeclampsia. The RRR estimates for carotenoids were similar in magnitude to α-tocopherol:cholesterol; in both cases, RRRs suggested inverse associations attenuated for late-onset preeclampsia. We saw a significant association between lutein and both early- and late-onset preeclampsia. Adjustment for confounding had a stronger impact on risk estimates in the early-onset subgroups.
Biomarker | RRR crude (95% CI) a | RRR adjusted (95% CI) b | |
---|---|---|---|
α-Tocopherol | Early onset | 0.59 (0.40–0.87) | 0.74 (0.49–1.13) |
Late onset | 0.94 (0.73–1.20) | 0.99 (0.75–1.30) | |
α-Tocopherol/cholesterol | Early onset | 0.55 (0.36–0.85) | 0.67 (0.46–0.99) |
Late onset | 0.85 (0.64–1.13) | 0.88 (0.69–1.14) | |
Ln(γ-tocopherol) | Early onset | 1.34 (0.94–1.92) | 1.22 (0.81–1.83) |
Late onset | 0.94 (0.74–1.20) | 0.94 (0.71–1.23) | |
Retinol | Early onset | 1.45 (1.04–2.01) | 1.61 (1.12–2.33) |
Late onset | 0.97 (0.76–1.24) | 0.96 (0.74–1.26) | |
Ln(lycopene) | Early onset | 0.96 (0.68–1.36) | 1.04 (0.72–1.50) |
Late onset | 0.84 (0.66–1.06) | 0.90 (0.71–1.16) | |
Ln(lutein) | Early onset | 0.50 (0.35–0.72) | 0.53 (0.35–0.80) |
Late onset | 0.64 (0.50–0.82) | 0.62 (0.47–0.82) | |
Ln(carotenoids) c | Early onset | 0.52 (0.37–0.73) | 0.66 (0.42–1.02) |
Late onset | 0.94 (0.74–1.21) | 0.89 (0.65–1.22) |
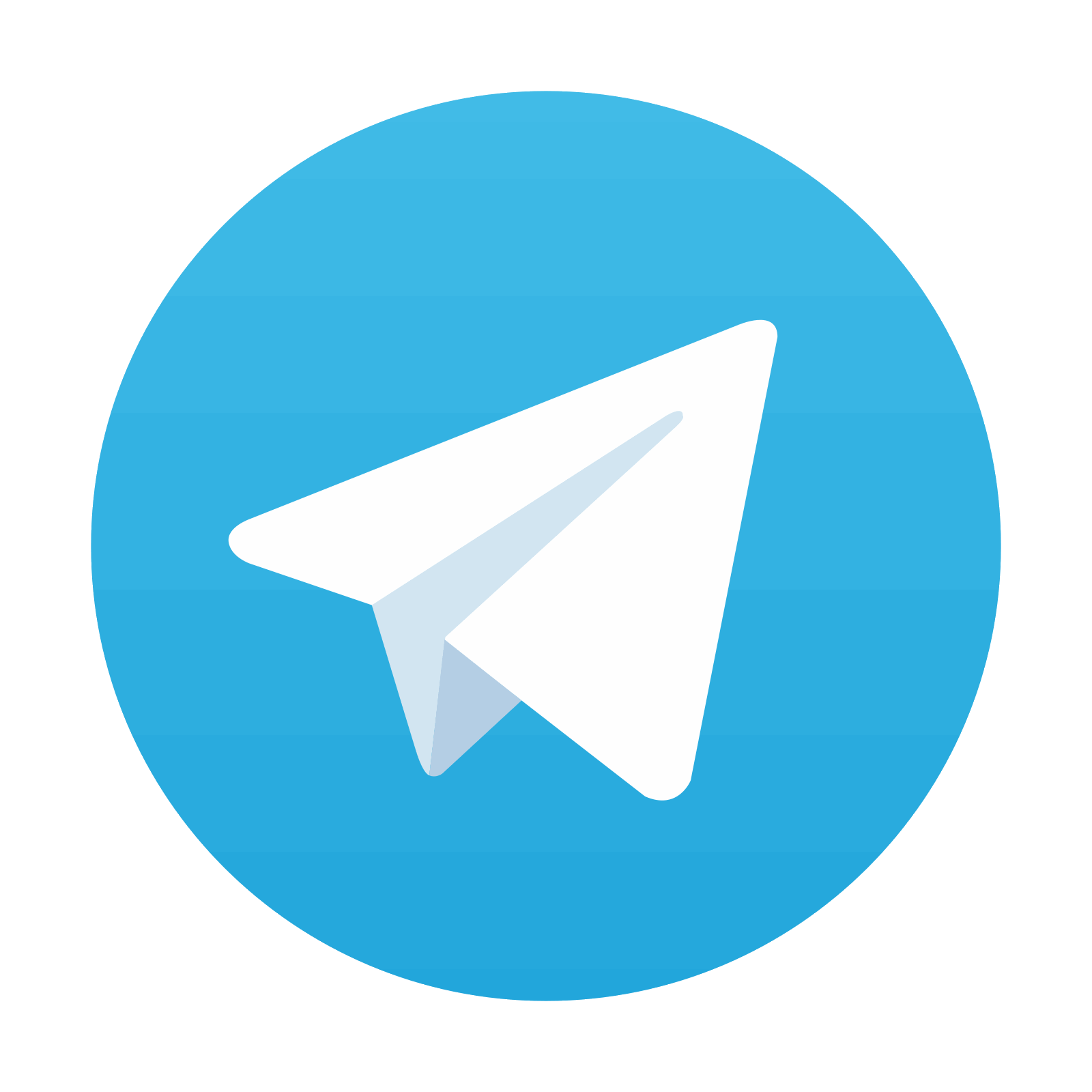
Stay updated, free articles. Join our Telegram channel

Full access? Get Clinical Tree
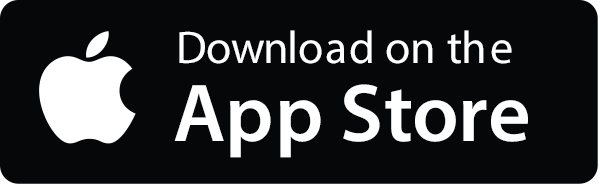
