In medicine in general, and in obstetrics in particular, it is common practice to use arbitrary cutoffs in expressing continuous variables. However, the dichotomy of continuous data is associated with loss of statistical power, which may result in inaccurate estimates in clinical prognosis or prediction of outcomes and, consequently, may lead to incorrect inferences. If the predictor is a continuous variable, arbitrary percentile-based categorizations without clinical justification is an anathema (Greek word meaning “curse”) and should be discouraged. Instead, the clinical outcome of interest should be defined first and then a receiver operating characteristic curve analysis or other appropriate statistical techniques should be employed to determine the most optimal cutoff of the predictor. The next step should be to validate the cutoff in a different population before introducing it to clinical use or interventional trials.
Obstetricians and researchers are faced with countless variables that are measured on a continuous scale–maternal age, weight, body mass index (BMI), and cervical length are typical examples. However, it is rare for these variables to be used in a continuous fashion to predict pregnancy outcomes. The most frequent scenario is to use these continuous variables in a dichotomous manner treating them as though they were categorical variables. Several cutoffs have been used to dichotomize these variables. Sometimes these cutoffs are entirely arbitrary, while in other situations, the cutoffs are based on some frequency criteria (eg, <10th percentile, >2 standard deviations below the mean, >95th percentile). However, the dichotomy by using arbitrary cutoffs is associated with loss of statistical power and this may mask the true predictive ability of a continuous variable. It is much more precise to use predictors in a continuous fashion such as is the use of tables predicting neonatal survival (or death) based on each individual gestational week at birth. Unfortunately, in most other instances in obstetrics, most predictor cutoffs have not been used in a continuous fashion; instead, they have been arbitrarily defined by choosing a frequency percentile without validation with respect to the clinical outcome of interest. However, such practice may lead to inaccurate clinical predictions resulting in problems in patient care and counseling as well as in designing and interpreting the results of interventional trials.
The purpose of this article is to describe the clinical and research pitfalls of arbitrarily dichotomizing continuous variables and provide some examples related to aneuploidy risk assessment, maternal age, BMI, cervical length, and birthweight. We note that this manuscript is not a portrayal against the use of categorization of continuous predictors, but rather that the adoption of such categorizations should be derived on the basis of sound statistical analysis and adopted after rigorous testing. The focus in our manuscript is on prediction, but the recommendations we propose for categorizing continuous predictors apply equally well for estimating associations.
Pitfalls in obstetric practice
Risk assessment for aneuploidy
Various tests are available for Down syndrome screening, with the most common being the first trimester nuchal translucency measurement combined with levels of serum analytes, and the second trimester serum screen consisting typically of 3 or 4 other analytes. Based on these measurements and maternal age, risks are reported for Trisomy 21, 18, and 13 by using various cutoffs including 1 in 100, 150, 250, 270, or 300, depending on the laboratory and the particular test. A risk that is greater than the threshold cutoff is reported as positive, although 1 that is less than the cutoff is reported as negative for Down syndrome risk. For instance, if a cutoff of 1 in 300 is used as the threshold, a risk of 1 in 299 is reported as positive, although 1 of 1 in 301 is reported as negative. This may give the false impression that risks of 1 in 2 and 1 in 299 have the same weight or that a risk of 1 in 299 is clinically different from 1 in 300. Reporting the test simply as “positive” or “negative” may lead to undue anxiety and misunderstanding of the results. Consideration should be given to reporting the patient’s actual risk, which is more objective and may be easier to understand.
Another example is nuchal fold measurements for the prediction of fetal Down syndrome. Generally, it is recognized that an isolated nuchal fold thickness >6 mm at 18-22 weeks of gestation confers a more than 10-fold increase in the risk for Down syndrome. However, this again inappropriately simplifies the issue. A patient with a nuchal fold measurement of 6.1 mm is different from 1 with a measurement of 15 mm. To place them both in an equal footing for the same risk category is inappropriate. Down syndrome risk cannot jump 10-fold based on a change of nuchal fold thickness from 6.0 mm to 6.1 mm. Furthermore, even the gestational age at which the nuchal fold is measured matters. Thus, a nuchal fold of 5.9 mm at 15 weeks may have different implications from a measurement of 5.9 mm at 22 weeks.
Risk assessment based on maternal age
One of the most important predictors of perinatal outcomes is maternal age. Despite a clear dose-response relationship between maternal age and most adverse pregnancy outcomes, maternal age has been rarely used as a continuous variable. For most pregnancy complications, the cutoff of ≥35 years (advanced maternal age) has been traditionally used to identify patients as “high risk.” However, this dichotomy of maternal age as ≥35 vs <35 does not take into consideration the gradual increase in pregnancy complications with increasing maternal age (dose-response relationship). Yet, there is evidence to suggest that the risks for most pregnancy complications increase with advancing maternal age, including chromosomal disorders, congenital anomalies, spontaneous and recurrent abortions, fetal growth restriction, macrosomia, hypertensive disorders, gestational diabetes, placenta previa, placental abruption, preterm delivery, operative vaginal delivery, cesarean delivery, postpartum hemorrhage, as well as maternal and perinatal morbidity and mortality. Thus, maternal age has a continuum rather than a threshold or cutoff effect. Despite the wide-spread realization of risks, most clinicians and investigators estimate patients’ risks based on the “35 years old” dichotomy rather than the specific maternal age-related risks. The prevalent approach in patient counseling, as well as in research, to group together the risks of a 35 year old and a 44 year old pregnant woman is not reasonable. A problem of using age as a dichotomous variable is that this approach leads to the physician considering women of ages 34½ and 35½ years as belonging to different risk groups although not recognizing a difference between a woman aged 20 and one aged 34. One example of using maternal age as a continuous predictor is the use of tables of fetal aneuploidy with each specific maternal age and this is extremely helpful for the individual patient. Similarly, it would be ideal if prior research studies had treated maternal age as a continuous (predictor) variable in predicting other pregnancy complications. However, most studies that use maternal age as predictor have grouped it into 2 or more groups; thus, we do not have tables or algorithms showing the specific maternal age related risks for developing pregnancy complications other than aneuploidy.
Risk assessment based on maternal BMI
Similar observations can be made with using BMI as predictor of pregnancy outcome. Although pregnancy complications increase with increasing BMI categories, the BMI is seldom analyzed as a continuous variable but as categorical groups. Thus, the use of grouping in risk assignment does not allow for an accurate risk prediction based on the patient specific BMI. Hopefully, future research will use maternal age and BMI as continuous predictor variables. Such research could produce tables or algorithms depicting the expected risk of various pregnancy complications based on the specific maternal age in combination with BMI or other factors, thus allowing for more accurate patient counseling and exact tailoring of prenatal care content.
Risk assessment for spontaneous preterm birth based on cervical length
Cervical length, as determined by second trimester transvaginal ultrasound, has been used as a predictor of spontaneous preterm birth. In a large Maternal Fetal Medicine Networks Unit’s observational study, the risk of spontaneous preterm birth was increased as the length of cervix decreased in a dose-response relationship. The authors performed a logistic regression analysis and demonstrated the expected probability for spontaneous preterm birth <35 weeks for all possible cervical lengths. Despite this dose-response relationship, surprisingly subsequent observations and even some interventional trials relied on an arbitrary percentile-based definition of “short cervical length” ≤25 mm (10th percentile) without any prior clinical validation. In our view, the dichotomy of cervical lengths at ≤25 mm vs >25 mm without clinical validation has resulted in problems in patient care and counseling as well as in research.
With respect to individual patient care and counseling, it is not logical to group all cervical lengths (≤25 mm) together. The combined risk (rate) for preterm birth for all patients with cervical lengths ≤25 mm cannot be used for the individual patient who has a specific cervical length. For instance, as a group, asymptomatic low-risk women with cervical length ≤25 mm at 24 weeks have a risk for spontaneous preterm birth of 17.8%. However, the individual risks within the ≤25 mm group vary. Assuming the same set of circumstances (ie, asymptomatic high-risk patients at 24 weeks), the risks for spontaneous preterm birth (<35 weeks) for cervical lengths of 25, 20, 15, 10, 5, and 0 mm are 22%, 28%, 35%, 43%, 51%, and 59%, respectively. Another issue to consider is that cervical length is a continuous variable, but so is gestational age, thus the prognosis of the same “short” cervical measurement depend on the gestational age at which is diagnosed ; yet, the studies tend to lump them all together. Therefore, in counseling patients the individual cervical length, as well as gestational age at diagnosis of cervical shortening, should be ideally used to determine the risk of preterm birth rather than a dichotomized range or group of cervical lengths at a wide range of gestational ages (ie, midtrimester). Unfortunately, it is not unusual in daily practice for all patients with a cervical length below the percentile-based cutoff of <25 mm to be treated similarly regardless of the specific measurement and the specific gestational age. Another important issue to consider is that the most optimal cutoff may vary according to the outcome of interest. For instance, if the outcome of interest is not spontaneous preterm birth but prediction of hemorrhage, as is the case in patients with placenta previa, the most optimal cervical length cutoff has been shown to be 30 mm that is not one of the generally used cutoffs for preterm labor prediction in asymptomatic patients.
Pitfalls in research
The perils and assumptions of categorization
Categorization of continuous predictors has serious implications in the interpretation of the data. Analyzing continuous risk factors in the way they were measured, in general, has increased power to detect associations. Although categorization enables a fairly straight forward assessment of simple associations, there are some implications in transforming continuous variables to categorical variables. Foremost among them is the less realized concept of “risk averaging within categories” that assumes that values within a level of the categorical variable are homogeneous and that values between levels are heterogeneous leading to an inability to assess dose-response and trend effects. The concept of risk averaging within categories implies that risks are similar for all values within a category and that the risks within the same group are homogeneous. This could mean that both the risk and severity of preeclampsia are the same for women with BMI of 35.1 or 49.9 (assuming that BMIs 35.0 to 49.9 are grouped in a single level).
Interventional trials
There is no doubt that in trials the chosen cutoffs for a particular intervention will define the outcome. It is noteworthy that trials using the arbitrary 10th percentile cervical length ≤25 mm as a cutoff for intervention have produced results that are difficult to interpret. Case in point is the Owen multicenter randomized trial of cerclage for preterm birth prevention in high-risk women where a cervical length cutoff of <25 mm was used for intervention (cerclage placement). The primary hypothesis was that cerclage placement would reduce the risk of preterm birth (<35 weeks) in women with a prior spontaneous preterm birth (<34 weeks) whose midtrimester cervical length was <25 mm. No clinical justification was provided for using the 25 mm as cutoff. This cervical length cutoff was arbitrary and it was based solely on the fact that it was found to be the 10% percentile in a previous study. The Owen trial showed that the primary outcome (preterm birth <35 weeks) was not significantly different in the cerclage group (odds ratio, 0.67; 95% confidence interval, 0.42–1.07). Even the Kaplan-Meier survival analysis did not show significant difference in the duration of pregnancy between the cerclage and control group ( P = .053). However, when the cervical length was treated as continuous variable in a logistic regression analysis the association between cerclage and preterm birth prevention was statistically significant (odds ratio, 0.60; 95% confidence interval, 0.37–0.98). This observation suggests that conclusions are more precise if we analyze continuous data as such rather than based on arbitrarily defined categories.
The choice of a cutoff should not be arbitrary and should not be based on some frequency percentile without clinical validation. The validation should ideally include 2 steps. The first step is to determine the specific outcome of interest and the second is the use of receiver-operating characteristic (ROC) curve to visually identify the most optimal cutoff (balance between sensitivity and false positive rate) in predicting the chosen outcome of interest. Other methods to define optimal cutoffs for nonmonotonic associations include transformations (such as regression splines or polynomials) and visual inspection of the risk of the outcome in relation to the continuous predictor to guide the choice of the most optimal cutoff. Then, it is critical to validate this cutoff in a different population before using it clinically or undertaking any interventional trials. Failure to do so may result in a null (no effect) trial–not because the intervention does not work but perhaps because the cutoff chosen for intervention may not be appropriate; this may well have been the case with the Owen trial.
Indeed, in determining the optimal cervical length cutoff, investigators have conducted several clinical validation studies before undertaking any interventional trials. These studies used ROC curves and determined the sensitivity and false positive rate for different cervical length cutoffs (for predicting spontaneous preterm birth) and have concluded that the optimal cervical length cutoff is not the arbitrarily defined 10th percentile (ie, 25 mm) but the 1.7th percentile (ie, 15 mm); this cervical length cutoff was subsequently validated by several observational studies. A subsequent interventional trial with vaginal progesterone, using cervical length ≤15 mm as a cutoff for intervention showed significant reduction (approximately 45%) in the risk of preterm birth at <34 weeks’ gestation. Interestingly though, the same cervical length cutoff ≤15 mm was found to be the best cutoff in the cerclage trial by Owen et al, but only in a secondary analysis of their data.
Clinical prediction studies
In this study, the use of birthweight as predictor of pregnancy outcome is a classic example. Unfortunately, most studies have used various birthweight cutoffs as predictors of neonatal outcome despite the unambiguous evidence that there is a strong inverse dose-response relationship between decreasing birthweight percentiles and increased risk of adverse neonatal outcomes. However, it is unreasonable to expect that there is a “magic” birthweight cutoff where all perinatal mortality and morbidity suddenly surfaces, and the risks of these adverse outcomes are below that certain birthweight threshold. In our view, there may be many different birthweight cutoffs depending on the nature of the adverse outcome that is under scrutiny. The use of ROC curves or other appropriate statistical techniques should identify the best cutoff, if any, by choosing the appropriate balance between sensitivity and false positive rate depending on the outcome of interest. Future studies that use birthweight as a predictor of neonatal outcomes should analyze it as a continuous variable, if possible, so that information about its predictive ability is not lost in the process.
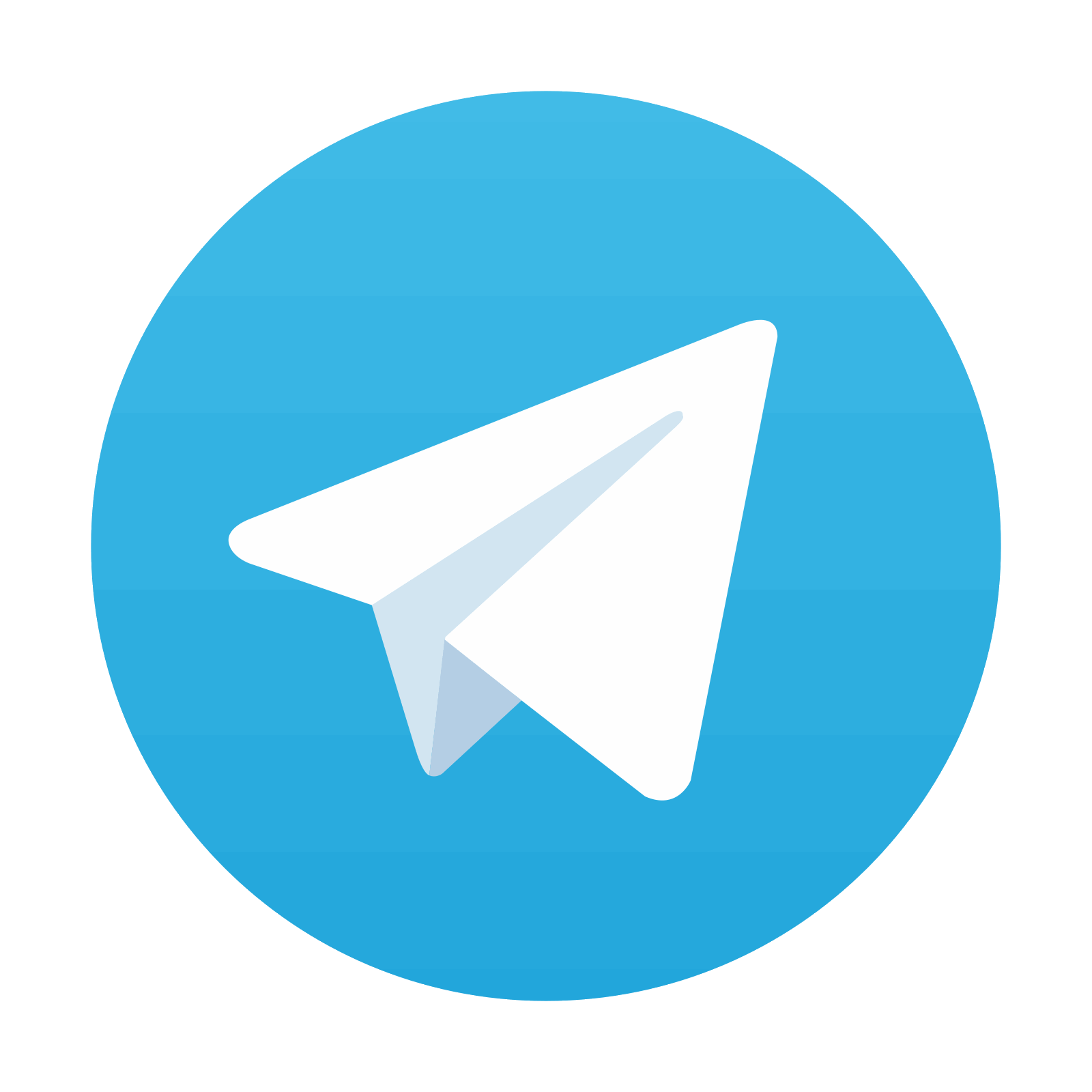
Stay updated, free articles. Join our Telegram channel

Full access? Get Clinical Tree
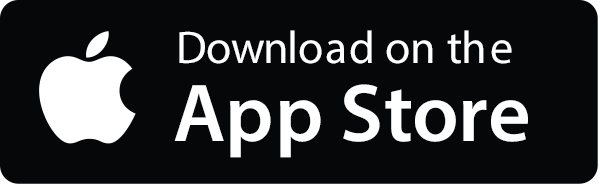
