Objective
We sought to determine the impact of geographic location on advanced-stage ovarian cancer mortality in relation to adherence to National Comprehensive Cancer Network (NCCN) treatment guidelines and hospital case volume.
Study Design
This was a retrospective observational cohort study of patients diagnosed with stage IIIC/IV epithelial ovarian cancer (Jan. 1, 1996, through Dec. 31, 2006) identified from the California Cancer Registry. Generalized additive models were created to assess the effect of spatial distributions of geographic location, demographic characteristics, disease-related variables, adherence to NCCN guidelines, and hospital case volume, with simultaneous smoothing of geographic location and adjustment for confounding variables.
Results
A total of 11,765 patients were identified. Twelve of the 378 hospitals (3.2%) were high-volume hospitals (HVH) (≥20 cases/y) and cared for 2112 patients (17.9%). For all patients, the median distance to an HVH was 22.7 km/14.1 miles and 80% were located within 79.6 km/49.5 miles of an HVH. Overall, 45.4% of patients were treated according to NCCN guidelines. The global test for location revealed that geographic position within the state was significantly correlated with ovarian cancer mortality after adjusting for other variables ( P < .001). Distance to receive care ≥32 km/20 miles was protective against mortality (hazard ratio [HR], 0.86; 95% confidence interval [CI], 0.79–0.93), while distance from an HVH ≥80 km/50 miles was associated with an increased risk of death (HR, 1.13; 95% CI, 1.03–1.23). The effects of geographic predictors were attenuated when nonadherence to NCCN guidelines (HR, 1.25; 95% CI, 1.18–1.32) and care at an HVH (HR, 0.87; 95% CI, 0.81–0.93) were introduced into the model.
Conclusion
Geographic location is a significant predictor of advanced-stage ovarian cancer mortality and the effect is primarily related to the likelihood of receiving NCCN guideline adherent care and treatment at an HVH.
Click Supplementary Content under the article title in the online Table of Contents
In the United States, there are 22,000 new cases of ovarian cancer diagnosed and >14,000 disease-related deaths annually, which account for more deaths than all other gynecologic cancers combined. Survival determinants are multifactorial; however, health care delivery system characteristics have emerged as important predictors of ovarian cancer-related mortality. Adherence to National Comprehensive Cancer Network (NCCN) treatment guidelines for ovarian cancer has recently been validated as correlating with improved disease-specific and overall survival, with the demonstrable survival benefit being proportionally greater for women with advanced-stage disease. In addition, average annual hospital ovarian cancer case volume has been correlated with improved outcomes. Specifically, hospitals treating ≥20 cases/y, or high-volume hospitals (HVHs), are more likely to administer standard recommended treatment but are also associated with an independent survival benefit over and above adherence to treatment guidelines.
The contribution of geographic location to differences in access to health care, resource utilization, and cancer survival is becoming increasingly relevant. Recently, our group examined the impact of geographic location on adherence to NCCN treatment guidelines among patients with advanced-stage ovarian cancer and found that increasing distance from an HVH was an independent predictor of receiving non-guideline treatment, while increasing patient travel distance to receive care was protective. While both adherence to NCCN treatment guidelines and care at an HVH have been previously correlated with improved ovarian cancer survival, the interaction between these survival determinants and geographic location has not been examined. The objective of the current study, therefore, was to expand upon our initial work to determine the impact of geographic location on advanced-stage ovarian cancer mortality in relation to adherence treatment guidelines and average annual case volume of the hospital where care was received.
Materials and Methods
Study design
The study design was a retrospective population-based analysis of the effect of geographic variation on advanced-stage invasive epithelial ovarian cancer mortality for cases reported to the California Cancer Registry using generalized additive models (GAMs), with simultaneous smoothing of location and adjustment for known confounders. The study received exempt status by the Institutional Review Board of the University of California, Irvine (HS no. 2011-8317). Registry case reporting is estimated to be 99% for the entire state of California, with follow-up completion rates exceeding 95%. International Classification of Diseases for Oncology codes based on World Health Organization’s criteria were used for tumor location and histology. Cases were identified using ovarian Surveillance, Epidemiology, and End Results (SEER) primary site code (C569).
Sample population
The initial study population included women who were age ≥18 years at diagnosis of a first or only invasive epithelial ovarian cancer. A total of 21,044 incident cases were identified during the time period Jan. 1, 1996, through Dec. 31, 2006. We sequentially excluded: 101 borderline tumors, 165 of nonepithelial histology, 246 cases that had missing International Classification of Diseases for Oncology, Second Edition morphology code, 742 cases prepared from autopsy or death certificate only, 1415 with incomplete clinical information, 78 with incomplete hospital information or location outside of California, and 98 with missing census tract information. In all, 2845 cases were excluded. Among the remaining 18,199 cases of all stages, 11,765 patients diagnosed with International Federation of Gynecology and Obstetrics (FIGO) stage IIIC/IV disease were selected as the study population and represents a subset of a prior analyses investigating predictors of access to high-volume providers and the impact of geographic location on adherence to treatment guidelines.
Statistical analysis
The primary analysis was the effect of geographic variation on disease-specific mortality for stage IIIC/IV epithelial ovarian cancer. We examined geographic variation of ovarian cancer mortality by smoothing location while controlling for known individual-level risk factors using a GAM framework. The GAM estimates the effect of location on the log-hazard function for mortality throughout California by applying a bivariate smooth of the latitude and longitude of each subject’s location, represented by the centroid of the address census block.
The first model, GAM-1, included age at diagnosis as a continuous variable, tumor characteristics (FIGO stage, grade, histology, and tumor size), insurance type, race, and socioeconomic status (SES). Insurance type was grouped into 6 categories: managed care (managed care, health maintenance organization, preferred provider organization, other private insurance), Medicare, Medicaid, other insurance (military, county-funded), not insured (self-pay), and unknown. SES was classified according to increasing quintile of Yost score: lowest (SES-1), low-middle (SES-2), middle (SES-3), high-middle (SES-4), and highest (SES-5). The Yost score is an index of SES level based on a principal components analysis of variables at the census block group-level and includes education, household income, proportion <200% poverty level, house value, rent, percent employed, and percent with blue-collar employment. In addition, we included variables for distance between patients and the hospital where they sought treatment as well as distance to the closest HVH. Hospital volume was calculated based on the average annual number of all ovarian cancer cases (stages I-IV) that were admitted to that hospital. Hospitals with ≥20 cases/y were classified as HVH, while hospitals treating <20 cases/y were considered low-volume hospitals (LVH). Distances were calculated using ArcMap (version 10.0; Esri, Redlands, CA) and categorized by quintiles: distance to care (<5 km/3 miles; 5-9 km/3-5 miles; 10-16 km/6-9 miles; 17-31 km/10-19 miles; ≥32 km/20 miles) and distance to closest HVH (<9 km/5 miles; 9-17 km/5-10 miles; 18-33 km/11-20 miles; 34-79 km/21-49 miles; ≥80 km/50 miles).
The second model, GAM-2, was identical to GAM-1 but introduced additional variables for whether the hospital where treatment was received was an HVH or an LVH and whether that treatment was adherent or nonadherent to NCCN guidelines for advanced-stage ovarian cancer. Adherence to guidelines was based on recommendations for surgery and chemotherapy according to the time period of diagnosis. A minimum of oophorectomy (with or without hysterectomy) and omentectomy was considered adherent surgical care, and either initial surgery or chemotherapy was characterized as appropriate. Administration of multiagent chemotherapy was characterized as adherent care. The California Cancer Registry does not provide the number of chemotherapy cycles; consequently, no minimum number of treatment cycles was incorporated into the criteria for chemotherapy adherence to guideline care. Dichotomous variables, adherence or nonadherence, were created for the overall treatment program.
We used a locally weighted regression smoother (loess) for the effect of location in the Cox model. GAM estimation for the bivariate smoother corresponding to location was carried out via backfitting on the linear predictor from the Cox model, using a smoothed estimate of the Fisher information matrix. The number of data points used for smoothing was determined by minimizing Akaike information criterion. We generated an evenly spaced grid of prediction points approximately 5 km apart that extended across the latitude and longitude coordinates of participants’ locations throughout California, resulting in in a grid of >7500 points. We did not predict for areas with sparse population data. At each point on the grid we calculated the log-hazard multiplier and hazard ratio (HR) using the average log hazard for the study area as the reference. Without the bivariate smooth term for location, the model reduces to a standard Cox proportional hazards regression model.
GAMs also provide a useful framework for hypothesis testing. We implemented a permutation test of the null hypothesis that ovarian cancer survival does not depend on the geographic location of participants, adjusting for other risk factors. Residential locations were permuted 999 times while preserving participants’ outcome status and covariates. For each permutation, the Cox model, including a smoothed effect of location, was refit and a global deviance statistic was computed by taking the difference of the deviances of the Cox model with and without the location term. Spatial analyses were conducted in the R Package (version 2.12.02; Vienna, Austria) using the MapGAM package.
Results
Population characteristics
The median age at diagnosis for the 11,765 subjects was 65.0 years (mean, 63.8 years; range, 18–104 years), and 7216 patients (61.3%) had stage IIIC disease ( Table 1 ). Overall, 5343 patients (45.4%) were treated according to NCCN guidelines ( Figure 1 ). A total of 378 hospitals provided care to the study population ( Figure 2 ). Of these, 12 hospitals (3.2%) were HVHs (2112 patients, 17.9% of cases), while 366 hospitals were LVHs (9653 patients, 82.0% of cases). For patients receiving care at an HVH, the median driving distance was 20.4 km/12.7 miles, with 80% of patients driving a distance of ≤56.2 km/34.9 miles. For patients treated at an LVH, the median driving distance was 11.4 km/7.1 miles, and 80% of patients travelled ≤28.3 km/17.6 miles to receive care. For all patients, the median Euclidean distance to an HVH was 22.7 km/14.1 miles, with 80% of patients living within 79.6 km/49.5 miles of an HVH.
Characteristic | n | Percent |
---|---|---|
Total | 11,765 | 100.0 |
Age at diagnosis, y | ||
<45 | 1035 | 8.8 |
45-54 | 2062 | 17.5 |
55-69 | 4179 | 35.5 |
≥70 | 4489 | 38.2 |
Race/ethnicity | ||
White | 8432 | 71.7 |
Black | 556 | 4.7 |
Asian/Pacific Islander | 1803 | 15.3 |
Hispanic | 974 | 8.3 |
Insurance | ||
Managed care | 5272 | 44.8 |
Medicare | 3798 | 32.3 |
Medicaid | 941 | 8.0 |
Other insurance | 1109 | 9.4 |
Not insured | 318 | 2.7 |
Unknown | 327 | 2.8 |
SES | ||
Lowest (SES-1) | 1528 | 13.0 |
Low-middle (SES-2) | 2137 | 18.2 |
Middle (SES-3) | 2481 | 21.1 |
High-middle (SES-4) | 2751 | 23.4 |
Highest (SES-5) | 2868 | 24.4 |
Tumor stage | ||
IIIC | 7216 | 61.3 |
IV | 4549 | 38.7 |
Tumor grade | ||
1 | 344 | 2.9 |
2 | 1562 | 13.3 |
3 | 4992 | 42.4 |
4 | 1239 | 10.5 |
Unknown | 3628 | 30.9 |
Tumor histology | ||
Serous | 5740 | 48.8 |
Mucinous | 415 | 3.5 |
Endometrioid | 638 | 5.4 |
Clear cell | 321 | 2.7 |
Adenocarcinoma, NOS | 1969 | 16.7 |
Tumor size, cm | ||
<5 | 1274 | 10.8 |
5-10 | 2114 | 18.0 |
≥10 | 2000 | 17.0 |
Unknown | 6377 | 54.2 |
Hospital volume | ||
High | 2112 | 18.0 |
Low | 9653 | 82.0 |
NCCN treatment guideline adherence | ||
Adherent | 5342 | 45.4 |
Nonadherent | 6423 | 54.6 |
Spatial analysis of advanced-stage ovarian cancer mortality
In GAM-1, the initial iteration of the spatial analysis indicates the effect of geographic location on ovarian cancer mortality without adjusting for the effects of other variables and reflects the HR at each location using the average log hazard for the entire state as the reference (map HR range, 0.73–1.25; P < .001). The impact of geographic distribution on ovarian cancer mortality was affected as additional variables were introduced into the model in a stepwise fashion: age at diagnosis, tumor stage, tumor histology, and tumor grade (map HR range, 0.81–1.23; P < .001); race/ethnicity (map HR range, 0.80–1.30; P < .001); insurance status (map HR range, 0.80–1.34; P < .001); SES (map HR range, 0.86–1.44; P < .001); distance to receive care (map HR range, 0.85–1.48; P < .001), and distance to the closest HVH (map HR range, 0.85–1.48; P < .001). The global test for location was highly significant for all analyses ( P < .001), indicating that ovarian cancer mortality was significantly associated with the geographic location of subjects. In GAM-1, after controlling for disease-related characteristics, the model showed that distance to receive care 17-31 km/10-19 miles (HR, 0.87; 95% confidence interval [CI], 0.81–0.94) and ≥32 km/20 miles (HR, 0.86; 95% CI, 0.79–0.93) were significantly protective against ovarian cancer mortality, while distance from an HVH ≥80 km/50 miles was associated with an increased risk of death (HR, 1.13; 95% CI, 1.03–1.23) ( Table 2 ).
Characteristic | GAM-1 a | GAM-2 b | ||
---|---|---|---|---|
HR | 95% CI | HR | 95% CI | |
Age at diagnosis | 1.02 | 1.02–1.03 | 1.02 | 1.02–1.03 |
Race/ethnicity | ||||
White | 1.00 | 1.00 | ||
Black | 1.20 | 1.06–1.36 | 1.18 | 1.04–1.34 |
Asian/Pacific Islander | 0.93 | 0.85–1.02 | 0.93 | 0.85–1.01 |
Hispanic | 0.92 | 0.86–0.99 | 0.92 | 0.85–1.01 |
SES | ||||
Lowest (SES-1) | 1.25 | 1.14–1.38 | 1.23 | 1.11–1.35 |
Low-middle (SES-2) | 1.19 | 1.10–1.30 | 1.18 | 1.08–1.28 |
Middle (SES-3) | 1.16 | 1.07–1.25 | 1.13 | 1.05–1.22 |
High-middle (SES-4) | 1.09 | 1.02–1.17 | 1.08 | 1.00–1.16 |
Highest (SES-5) | 1.00 | 1.00 | ||
Insurance status | ||||
Managed care | 1.00 | 1.00 | ||
Medicare | 1.00 | 0.94–1.07 | 0.99 | 0.93–1.06 |
Medicaid | 1.05 | 0.95–1.15 | 1.05 | 0.95–1.14 |
Other | 0.96 | 0.88–1.19 | 0.94 | 0.86–1.02 |
Uninsured | 1.03 | 0.89–1.19 | 1.05 | 0.91–1.22 |
Unknown | 1.06 | 0.91–1.24 | 1.07 | 0.91–1.25 |
Tumor stage | ||||
IIIC | 1.00 | 1.00 | ||
IV | 1.54 | 1.46–1.62 | 1.52 | 1.44 |
Tumor grade | ||||
1 | 0.61 | 0.53–0.69 | 0.59 | 0.52–0.67 |
2 | 0.91 | 0.84–0.98 | 0.89 | 0.82–0.96 |
3 | 1.00 | 1.00 | ||
4 | 1.04 | 0.95–1.12 | 1.05 | 0.96–1.14 |
Unknown | 1.33 | 1.25–1.42 | 1.28 | 1.20–1.37 |
Tumor histology | ||||
Serous | 1.00 | 1.00 | ||
Mucinous | 1.58 | 1.38–1.82 | 1.53 | 1.33–1.76 |
Endometrioid | 0.89 | 0.80–0.98 | 0.87 | 0.79–0.97 |
Clear cell | 1.42 | 1.22–1.65 | 1.42 | 1.21–1.66 |
Adenocarcinoma, NOS | 1.51 | 1.39–1.63 | 1.44 | 1.33–1.56 |
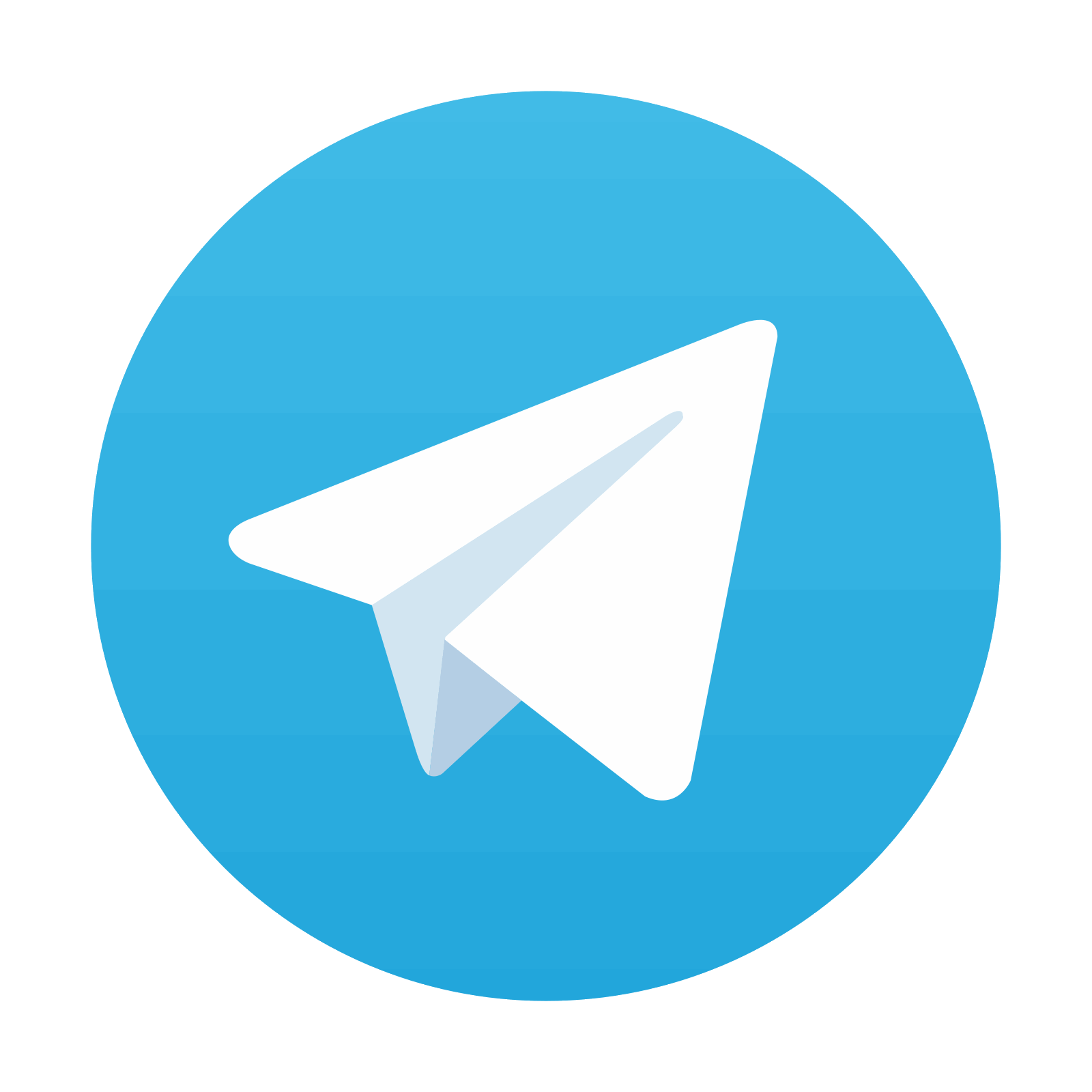
Stay updated, free articles. Join our Telegram channel

Full access? Get Clinical Tree
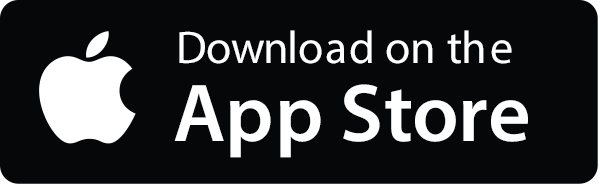
