The processes of diagnosis and management involve clinical decision-making. However, decision-making is often affected by cognitive biases that can lead to medical errors. This statement presents a framework of clinical thinking and decision-making and shows how these processes can be bias-prone. We review examples of cognitive bias in obstetrics and introduce debiasing tools and strategies. When an adverse event or near miss is reviewed, the concept of a cognitive autopsy —a root cause analysis of medical decision-making and the potential influence of cognitive biases—is promoted as part of the review process. Finally, areas for future research on cognitive bias in obstetrics are suggested.
Hypothetical Case
After an uneventful repeat cesarean delivery, a patient complains of mild dizziness while in the recovery room. The patient’s blood pressure is 100/60 mm Hg and heart rate is 120 beats per minute. The patient reports moderate pain. Intraoperative blood loss had been estimated at 800 mL. A blood count sent 2 hours after delivery demonstrates a drop in hemoglobin from 10.5 to 7.7 g/dL. When the nurse informs the obstetrician about the patient’s status, the obstetrician states, “I’m sure she’s fine.” Without evaluating the patient, the obstetrician attributes the change in hemoglobin to an underestimation of blood loss and hemodilution and attributes the tachycardia to uncontrolled pain.
Introduction
Medical error is prevalent, resulting in preventable harm to approximately 5% of patients across care settings. Errors span the spectrum of clinical care and can occur with diagnosis, treatment, preventive services, communication, and teamwork. Obstetrics is no exception. , A review of closed malpractice claims in obstetrics showed that adverse events were attributable, in part, to communication problems in 31% of cases, clinical performance issues in 31%, and diagnostic errors in 18%.
Why do we make medical errors? Our understanding of the factors that contribute to medical error continues to evolve. , Within a few years after the Institute of Medicine’s landmark report “To err is human,” several key conceptual models of patient safety were introduced. Adverse events are often not attributable to a single omission or commission. The Swiss-cheese model posits that several errors must “align” for an adverse event to occur. We have started to understand patient safety, for the most part, as a system function and value rather than the sole responsibility of isolated individuals. However, despite these helpful frameworks, progress in patient safety has recently stalled.
Drawing on the Swiss-cheese model, the contribution of healthcare professionals to patient safety is one of the most “downstream” layers of safety within the healthcare system. As error-prone individuals, it is our responsibility to improve our performance by working on reducing our fallibility (making the holes within our Swiss-cheese layer smaller) and thus reducing the chance of adverse events. This examination pertains mostly to our medical decision-making as we formulate diagnoses and prescribe treatments. Errors in medical decision-making are often attributable to cognitive bias. Diagnostic errors are associated with 6% to 17% of adverse events in hospitals, and 28% of diagnostic errors have been attributed to cognitive error.
Cognitive bias is defined as an implicit systematic error in thinking. Such errors can lead the clinician to make an erroneous judgment about a case. There are more than 180 types of cognitive bias. In this review, we (1) discuss how cognitive bias can affect decision-making, (2) highlight some cognitive biases with examples from obstetrics, (3) examine how cognitive bias can be the medium through which racism or other forms of discrimination can take shape, (4) briefly describe strategies and tools for debiasing, and, finally, (5) list potential areas for future research on cognitive bias in obstetrics. Our objectives are to raise awareness about cognitive bias and provide concepts, resources, and tools to promote unbiased clinical decision-making.
Cognitive Bias and How it Affects Decision-Making
There is increased awareness about the contribution of cognitive bias to medical error. To illustrate how cognitive bias operates, the Figure summarizes the dual-process theory, a psychological model of human cognition, as interpreted by Daniel Kahneman, a psychologist, economist, and Nobel prize laureate. Kahneman distinguishes 2 systems of the mind: “System 1 operates automatically and quickly, with little or no effort and no sense of voluntary control. System 2 allocates attention to the effortful mental activities that demand it, including complex computations. The operations of system 2 are often associated with the subjective experience of agency, choice, and concentrations.”

Healthcare professionals tend to perform most of the time in system 1, which is fast and intuitive. It uses mental shortcuts known as heuristics (“rules of thumb”) that clinicians develop through experience and repetition using pattern recognition. Heuristics allow us to function faster and think less. However, they can fail when a clinician faces a new pattern (such as a rare disease) or a pattern that is similar to a familiar one but different in important ways. Thus, system 1 is prone to systematic errors, ie, cognitive bias. , Most of the time, system 1 performs well (as shown by the green lines in the Figure ), but sometimes it fails as a result of cognitive biases (as shown by the red line in the Figure ) and could lead to a false diagnosis or treatment. Many factors affect the performance of these 2 modes of thinking, including lack of knowledge, past experiences, improper statistical reasoning, logical failures, fatigue, sleep deprivation, stress, burnout, affective state, ambient conditions (interruptions, limitation of resources, task overload), patient factors, and team factors. ,
In contrast, system 2 reflects the analytical, rational aspects of the thinking process. It is characterized by logical judgment and a deliberate mental search for information, often acquired through past learning and experience. , In principle, it should be less prone to cognitive bias than system 1, but it can be subject to bias if it is founded on flawed or biased information.
The delivery of healthcare, specifically the iterative process of diagnosis and treatment, often involves toggling between these 2 systems. An executive override allows system 2 to take over, for example, when the clinician or team members decide to take a pause (sometimes in the form of a “time out” or huddle) and think deliberately about the problem at hand. An irrational override occurs when the clinician ignores evidence-based rules in favor of more reflexive decision-making, such as when tired or overwhelmed, and they start to take mental shortcuts.
Examples of Cognitive Biases
Tolerance of ambiguity is the ability to perceive uncertainties, contradictory issues that may be difficult to understand, and information with vague, contrary, or multiple meanings in a neutral and open way. Many aspects of obstetrics can lend themselves to this bias. , For example, a category II (indeterminate) fetal heart rate pattern is often ambiguous, and its interpretation and management are subject to individual variation. If a clinician has a high tolerance for ambiguity, that clinician may be more likely to wait rather than rush to cesarean delivery. Conversely, if a clinician has a low tolerance of ambiguity, that clinician may be more likely to proceed with cesarean delivery in the setting of a category II tracing. In obstetrics, we are often faced with uncertain or ambiguous situations. As noted by Simpkin et al, a physician who has difficulty accepting uncertainty may order an excessive number of tests, which may lead to false-positive results and iatrogenic injury. Attempting to achieve a sense of certainty too soon risks premature closure of our decision-making process. Rather than avoiding uncertainty, learning to embrace this aspect of clinical medicine, especially in high-risk pregnancies, can help obstetricians grappling with complex cases keep an open mind about diagnostic possibilities and reduce excessive interventionism (as in the case of cesarean delivery in the example above).
Confirmation bias is the tendency to overweigh evidence that supports existing beliefs and dismiss evidence that does not. For example, in 1 study, clinicians were shown a simulated blood loss after a vaginal delivery. Despite being shown the same amount of blood, clinicians reported higher estimated blood loss when told the patient was hypotensive than when they were told the patient was normotensive. Here, clinicians interpreted data in a way that supported their assumed diagnosis (postpartum hemorrhage).
As another example, consider a sonographer measuring femur length in a fetus known to be at 21 weeks of gestation. The first measured femur length on the ultrasound monitor is equivalent to 17 weeks of gestation. The sonographer subconsciously rejects the information that the femur is abnormally short, assuming instead that the measurement is inaccurate. The sonographer remeasures the femur several times, ultimately selecting a measurement equivalent to 20 weeks of gestation and deleting the other images. These actions result in a lost opportunity to diagnose skeletal dysplasia.
Confirmation bias may be the most pervasive bias. Not only does it affect our diagnostic impression, but it also affects how we interpret new literature. Clinicians often refuse to change practice even when new high-quality data arises. For example, faced with conflicting evidence about whether skin closure with staples or subcuticular sutures has a lower rate of cesarean surgical site infections, a surgeon who prefers staples is likely to downplay studies suggesting that sutures are better, assigning more value to studies that suggest the opposite. This surgeon will likely continue to use staples even after updated evidence favors suture closure over staples. , Clinicians tend to allot more weight to their anecdotal experience than to clinical trial evidence, often using the phrase “in my experience” to justify deviating from established guidelines or protocols. This extreme form of confirmation bias may jeopardize patient safety.
Base rate neglect bias is the failure to incorporate the true rate of a disease into the diagnostic reasoning. This is common when approaching diagnosis with a “rule out worst-case” mindset, regardless of the likelihood of the disease. For example, during a routine scheduled prenatal visit at 34 weeks of gestation, a patient with no cardiovascular risk factors mentions that they have had some mild dyspnea. There are no “red flags” in the assessment, ie, the patient has no orthopnea, normal vital signs, and a benign physical examination. Despite the high likelihood that this patient’s diagnosis is dyspnea of pregnancy, the clinician with base rate neglect bias will order an extensive workup to rule out pulmonary embolism or cardiomyopathy.
Zebra retreat bias is the hesitation to consider a rare diagnosis (zebra) when other, more common diagnoses are part of the differential. For example, a patient presents at 30 weeks of gestation with headache and confusion. Blood pressure is 150/100 mm Hg, and laboratory values include urinary protein/creatinine ratio of 0.7, platelet count of 28,000 μ/L, normal transaminases, and elevated lactate dehydrogenase. The diagnosis of severe preeclampsia and HELLP (hemolysis, elevated liver enzymes, and low platelets) syndrome is made. Three hours later, the patient’s temperature spikes to 39°C. The clinician fails to consider thrombotic thrombocytopenic purpura (the zebra).
Availability (recent case) bias occurs when the memory of a recent diagnosis makes it more available to the mind of the physician, raising its rank in the current differential diagnosis. This may drive a clinician to order the related workup for that diagnosis, despite the low likelihood of that diagnosis in the current case. In the previous example of the patient with mild dyspnea, the clinician who would ordinarily simply reassure the patient will be more likely to pursue a diagnostic workup for pulmonary embolism if there was a recent case of pulmonary embolism in the practice.
As another example, consider a clinician who had a recent patient with appendicitis in pregnancy misdiagnosed as round ligament pain resulting in a ruptured appendix. This clinician would be more likely to order diagnostic imaging for subsequent patients with abdominal pain in pregnancy, even though the likelihood of appendicitis is very low.
Anchoring (and diagnosis momentum) is prematurely settling on a single diagnosis based on initial data. Anchoring can make it hard to change the diagnosis after new data emerge. For example, a patient is admitted with preterm labor. During hospitalization, the pain becomes more localized and acute. Because of anchoring bias, the covering clinician does not consider other diagnoses, such as appendicitis or degenerating leiomyoma.
Diagnosis momentum often occurs during handoffs. The incoming clinician may find it difficult to consider a different diagnosis than the one signed out to them. A diagnosis may therefore be carried over from clinician to clinician despite new data. The handoff information acts as an anchor for the next clinician even as the patient’s condition evolves.
Aggregate bias (ecological fallacy) is the belief that the conclusion drawn from aggregate data used in clinical decision instruments does not apply to the patient being evaluated. This bias is often implicated in cases where clinicians are more comfortable with their gestalt or intuition than with “imposed” decision rules. Clinicians may perceive these tools as a means to save money at the risk of missing a grave diagnosis.
For example, consider a hospital that recently adopted a decision rule for ordering a computed tomography (CT) scan in pregnancy to rule out pulmonary embolism based on D-dimer levels. The D-dimer level on a patient with dyspnea is <500 ng/mL, and the decision rule states that no further evaluation or treatment is needed. However, the clinician with aggregate bias cannot believe that the decision rule applies to this patient and therefore proceeds to order a CT scan.
There are many situations in obstetrics in which there is insufficient evidence to indicate the most beneficial treatment option. However, the clinician faced with such a situation must decide to recommend treatment or nontreatment despite the uncertain evidence. Commission bias is the tendency to systematically favor treatment in such situations, and omission bias is the tendency to systematically favor nontreatment. Because the optimal decision is unknown, either decision may possibly be an error.
Consider a situation in which the physician must decide whether to administer antenatal corticosteroids to a patient with threatened preterm labor (eg, frequent contractions at 28 weeks of gestation, cervix 1 cm dilated, ultrasound cervical length 15 mm, and negative cervicovaginal fetal fibronectin). Assuming a probability of 20% that such a patient will deliver within the next 7 days, clinicians operating under commission bias will administer antenatal corticosteroids, even though there is an 80% chance that the patient will not deliver within the 7-day window when such treatment is beneficial. Clinicians operating under omission bias will not administer antenatal corticosteroids to the patient, preferring to reserve their use until the risk of imminent preterm birth becomes higher. There is no way to know which treatment option is correct at the time of presentation because the outcome cannot be predicted with certainty.
Racial and cultural biases are often categorized as explicit vs implicit biases. In explicit bias, the person is aware of their attitudes and overt in their behavior, whereas implicit bias is largely unconscious and may directly contradict the person’s expressed beliefs and values.
Racism causes racial disparities in health through 3 main pathways: (1) persistent discrimination (via personal or systemic racism) that causes physiological and psychological stress, (2) implicit bias affecting the physician’s decision-making, (3) implicit bias affecting the clinical communication (eg, spending less time with the patient or dominating the conversation).
Stereotyping is a cognitive bias that consists of a fixed overgeneralized belief about a group or class of people. During a medical encounter, patients who belong to the stereotyped group may be under a “stereotyping threat” from a provider who assumes certain attitudes or behaviors solely based on the patient’s belonging to that group and thus modifies the medical intervention, whether diagnostic or therapeutic, based on that assumption. Interestingly, in providers who have low explicit bias and high implicit bias, these prejudices have the most effect on patients, specifically leading to anxiety, discomfort, and decreased satisfaction in the short term and affecting adherence and healthcare utilization in the long term. ,
Implicit racism can be layered onto other cognitive biases. For example, omission bias may be more prevalent when treating a patient from a racial or ethnic minority group. A physician may believe that a patient would not be adherent to a prescribed treatment regimen because of a language barrier and a perception that the plan of care is too complicated for the patient to comprehend. A physician may associate noncompliance with certain racial groups, not appreciating that barriers to compliance are often a direct result of structural racism and the social determinants of health. Therefore, a provider may not prescribe a recommended treatment plan to a patient of a racial minority based on the stereotype and unconscious bias that the patient will not adhere to the plan.
It is often difficult to pinpoint the exact points at which these biases occur along the diagnostic and therapeutic continuum, especially when other factors may trigger these mental shortcuts, such as provider fatigue, anxiety, or high cognitive load. However, implicit racism can be reduced if providers can recognize that bias exists, are willing to change, and are provided with a certain skill set. Such skills include perspective-taking, individuation, patient-centered communication, and others. , ,
In this review, we have only touched on a few known types of cognitive bias that can affect diagnostic reasoning and medical decision-making. Comprehensive reviews of the many other types can be found in monographs by Howard and Croskerry.
Debiasing Strategies and Tools
Clinicians integrate patient presentation with medical knowledge and other contextual factors to make a diagnostic impression. For this process to be accurate, one needs not only an adequate fund of knowledge but also the ability to think critically. Thus, we have an ethical obligation to improve how we think and make decisions and to study how we can mitigate cognitive bias as a cause of errors. This frontier of patient safety, examining how we think and make clinical decisions, is an important area for improvement in obstetrics.
Some debiasing interventions have been found to be useful. Table 1 lists several tools and strategies that may remediate or debias clinical decision-making. , , ,
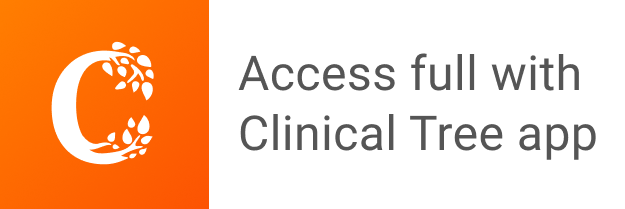