The article below summarizes a roundtable discussion of a study published in this issue of the Journal in light of its methodology, relevance to practice, and implications for future research. Article discussed:
Cohen-Cline HN, Kahn TR, Hutter, CM. A population-based study of the risk of repeat clinical chorioamnionitis in Washington State, 1989–2008. Am J Obstet Gynecol 2012;207:473.e1-7.
Discussion questions
- ■
Why is this study question important?
- ■
What is your opinion of the dataset?
- ■
Were inclusion and exclusion criteria clearly described?
- ■
Why employ 4 unexposed subjects for each exposed subject?
- ■
What information is contained in the tables?
- ■
What can we take away from this study?
Chorioamnionitis complicates pregnancy, though severe problems are uncommon among women who receive timely antibiotics. Even so, some 10% can develop bacteremia, and risks for postpartum hemorrhage, cesarean section, and surgical complications rise. Infants are at greater peril because their odds for consequences like cerebral palsy (CP), neonatal sepsis, or pneumonia climb. For example, chorioamnionitis boosts the incidence of CP from 3/1000 to 8/1000 live term births. When the membranes rupture before 37 weeks’ gestation, neonates exposed to infection and inflammation have more than 3 times the mortality rate of those who are not. A new study indicates that an episode makes women more susceptible to recurrence.
See related article, page 473
Journal Club participants agreed that work by Cohen-Cline and colleagues, intriguing for clinical and biological reasons, was an important contribution to the literature. Because chorioamnionitis is particularly dangerous for neonates, understanding which women might be at risk for recurrence is essential. To investigate this issue, the authors used the well-established Washington State linked birth certificate-hospital discharge dataset.
The main advantage to this dataset was the large number of observations it offered. Second, the use of ICD-9 codes for hospital discharge diagnoses is likely more valid than simply using the information provided on birth certificates. At the same time, because ICD-9 codes are generally identified by hospital-employed “coders,” the potential for random misclassification exists. Nonetheless, Journal Club participants felt that the dataset was an excellent source for the research question pursued by the authors.
Building power into a study
When assessing any research, be it clinical, translational, or basic, it is essential to have a clear sense of the assumptions underlying the estimation of sample size. While some study designs have specific requirements for sample-size estimations, both clinical trials and cohort studies like the study by Cohen-Cline et al have similar underlying suppositions. In general, 5 key pieces of information are needed to ensure a study has the statistical power to detect genuine differences between groups. The first is α error, which is conventionally set at 0.05, but might also be set at 0.01 or 0.001. This tells readers that that the likelihood researchers identified a difference that does not truly exist—that they made a Type 1 error—is less than 5%, 1%, or 0.1%.
Investigators often aim for a statistical power of 80-90%. In statistical terms, power is defined as 1-β, where β is the chance of making a Type II error; that is, wrongly presuming that the identified difference is the result of chance. If the researchers want to set their power at 80%, β would be set at 0.2, and for 90%, β would be set at 0.1. As researchers try to achieve a higher statistical power, they must increase the sample size accordingly.
In addition, researchers determine the incidence of the outcome they are looking for in the “unexposed” group. For example, in the study under discussion, 139 members of the unexposed group—women who did not have chorioamnionitis in their first pregnancy—did have chorioamnionitis in their second pregnancy. Researchers often base their determination on data in the literature or the results of well-conceived pilot studies. Minimum detectable relative risk is another critical assumption, and the goal is to seek the smallest value that is also clinically important. As the minimum detectable relative risk decreases, the sample size must increase. Frequently, some compromise must be made between the competing issues of clinical importance and feasibility.
An often-overlooked factor is the ratio of unexposed participants to exposed subjects, but this is a vital issue for sample-size estimation. Normally, increasing the ratio of unexposed people to those who have been exposed increases the study power. A ratio of 4 unexposed per 1 exposed generally yields the most favorable trade-off between increasing power and maintaining feasibility. Beyond this 4:1 ratio, gains in power are relatively small. In the Cohen-Cline manuscript, the authors used a 4:1 ratio, likely for the reasons outlined above.
These sample-size assumptions may seem obscure to some, but they are crucial for the assessment of clinical research. One way to understand them better is to experiment with a commercially-available sample-size calculator. This can provide hands-on experience in determining how estimates of sample size change when any of these assumptions are altered.
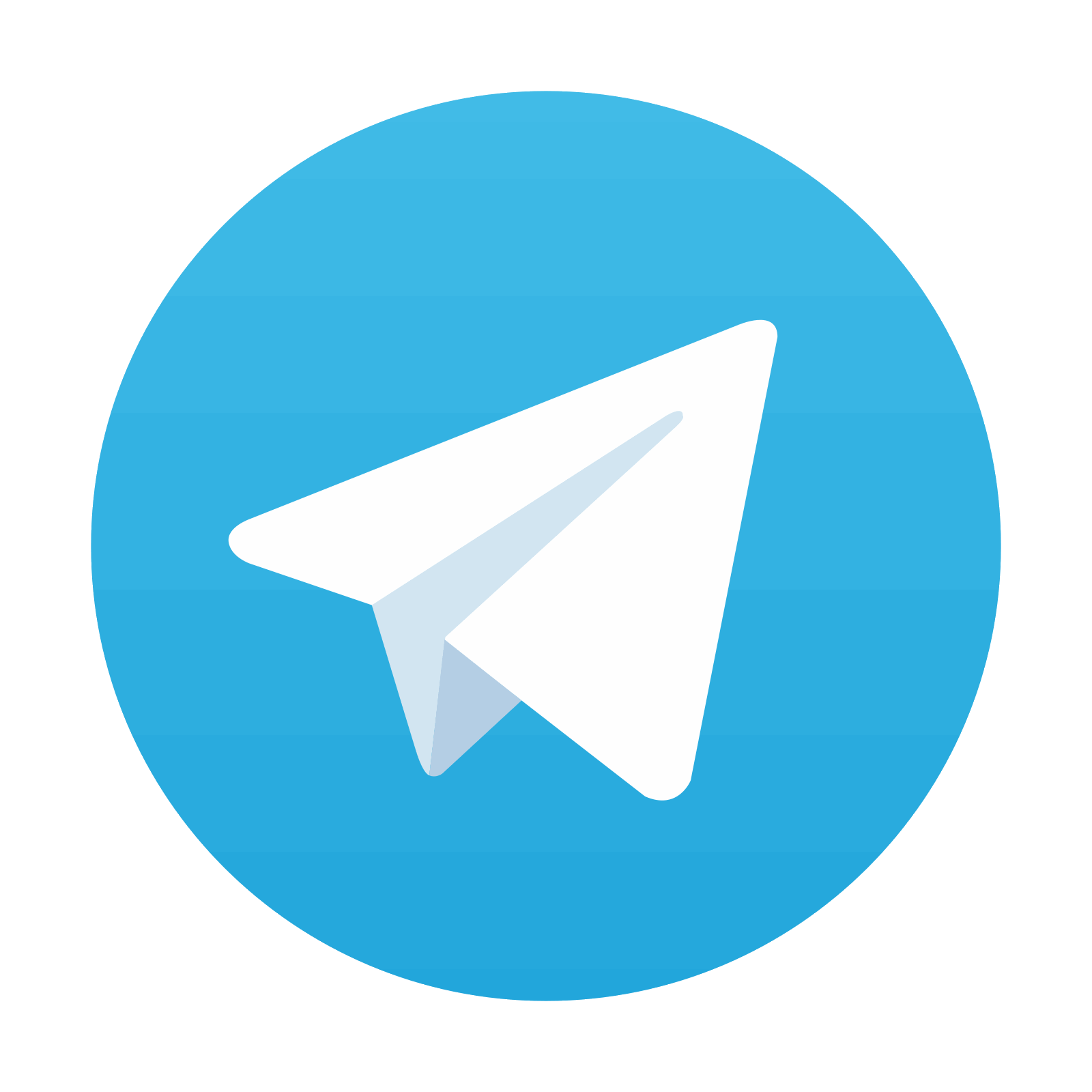
Stay updated, free articles. Join our Telegram channel

Full access? Get Clinical Tree
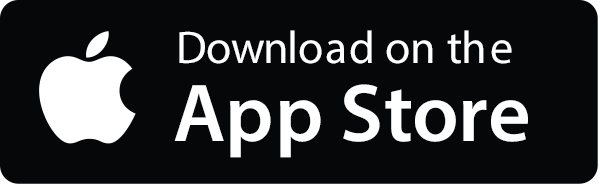
