Background
Same-day discharge following minimally invasive hysterectomy has been shown to be safe and feasible in select populations, but many nonclinical factors influencing same-day discharge remain unexplored.
Objective
To develop prediction models for same-day discharge following minimally invasive hysterectomy using both clinical and nonclinical attributes and to compare model concordance of individual attribute groups.
Study Design
We performed a retrospective study of patients who underwent elective minimally invasive hysterectomy for benign gynecologic indications at 69 hospitals in a statewide quality improvement collaborative between 2012 and 2019. Potential predictors of same-day discharge were determined a priori and placed into 1 of 7 attribute groupings: intraoperative, surgeon, hospital, surgical timing, patient clinical, patient socioeconomic, and patient geographic attributes. To account for clustering of same-day discharge practices among surgeons and within hospitals, hierarchical multivariable logistic regression models were fitted using predictors from each attribute group individually and all predictors in a composite model. Receiver operator characteristic curves were generated for each model. The Hanley–McNeil test was used for comparisons, 95% confidence intervals for the areas under the receiver operator characteristic curve were calculated, and a P value of <.05 was considered significant.
Results
Of the 23,513 patients in our study, 5062 (21.5%) had same-day discharge. The composite model had an area under the receiver operator characteristic curve of 0.770 (95% confidence interval, 0.763–0.777). Among models using factors from individual attribute groups, the model using intraoperative attributes had the highest concordance for same-day discharge (area under the receiver operator characteristic curve, 0.720; 95% confidence interval, 0.712–0.727). The models using surgeon and hospital attributes were the second and third most concordant, respectively (area under the receiver operator characteristic curve, 0.678; 95% confidence interval, 0.670–0.685; area under the receiver operator characteristic curve, 0.655; 95% confidence interval, 0.656–0.664). Models using surgical timing and patient clinical, socioeconomic, and geographic attributes had poor predictive ability (all areas under the receiver operator characteristic curve <0.6).
Conclusion
Clinical and nonclinical attributes contributed to a composite prediction model with good discrimination in predicting same-day discharge following minimally invasive hysterectomy. Factors related to intraoperative, hospital, and surgeon attributes produced models with the strongest predictive ability. Focusing on these attributes may aid efforts to improve utilization of same-day discharge following minimally invasive hysterectomy.
Why was this study conducted?
Same-day discharge (SDD) following minimally invasive hysterectomy (MIH) has been shown to be safe and feasible in select populations, but many nonclinical factors and their relative association with SDD remain unexplored.
Key findings
Prediction models that were fitted using intraoperative, surgeon-level, and hospital-level factors produced the greatest discrimination for predicting SDD, more so than patient-level factors.
What does this add to what is known?
Although previous studies have shown that intraoperative management and patient characteristics are associated with SDD after MIH, we found that surgeon-level and hospital-level factors are also predictive of SDD; these nonclinical factors had a higher predictive ability than patient characteristics.
Introduction
Hysterectomy is one of the most common surgical procedures performed on women. Although hysterectomy has historically been performed through laparotomy or vaginal approaches, the adoption of laparoscopic surgical techniques in the 1990s ushered in significant changes to gynecologic surgical approaches. Between 2010 and 2013, laparoscopic hysterectomy emerged as the most common approach for hysterectomy in the United States. Compared with open abdominal hysterectomy, minimally invasive hysterectomy (MIH) offers many advantages, including the possibility of providing outpatient surgery. Studies have shown that same-day discharge (SDD) following MIH is safe and feasible for selected patients. , In addition, SDD is associated with reduced healthcare costs and iatrogenic complications from inpatient hospitalization. ,
Given these advantages, there have been efforts to improve the utilization of SDD following MIH through the implementation of standardized enhanced recovery after surgery (ERAS) protocols. Implementation of ERAS protocols has been successful in improving SDD practices and decreasing hospital length of stay by including careful patient selection criteria, incorporating strategies for postoperative pain control, and providing patient education on postoperative expectations. Alongside these efforts, other studies have sought to identify predictors of SDD so that SDD practices can be implemented in an evidence-based manner. There are myriad factors that may contribute to the decision of hospital admission following MIH, including clinical and nonclinical factors. Although patient clinical and intraoperative factors have been evaluated, there has been less emphasis placed on other potential predictors of SDD, including surgeon-level and hospital-level factors that may have an influence on SDD. Furthermore, most previous studies assessing predictors of SDD were conducted at single institutions and are thus unable to account for varying practices across different hospitals.
The objective of this study was to develop prediction models for SDD following MIH using a large statewide surgical quality collaborative database to determine the relative predictive ability of clinical and nonclinical variable clusters (which we termed “attribute groupings”) while accounting for surgeon-level and hospital-level effects.
Materials and Methods
We performed a retrospective analysis of patients who underwent MIH for benign indications from July 1, 2012 to December 31, 2018 within the Michigan Surgical Quality Collaborative (MSQC) registry. The MSQC is a clinical quality improvement collaborative of 69 hospitals funded by the Blue Cross Blue Shield of Michigan and Blue Care Network that includes patients from all insurance payers. The goal of the collaborative is to improve the quality of surgical care provided for patients, including those undergoing hysterectomy. Patient characteristics, intraoperative data, and 30-day postoperative outcomes in the MSQC registry were collected by trained nurse data abstractors via review of the entire medical record. The quality and validity of the data were ensured through routine site visits, conference calls, and internal audits. Surgical case sampling methods used by the MSQC have previously been described. Studies using the deidentified MSQC registry data are considered exempt by the Michigan Medicine Institutional Review Board (HUM00073978).
All hysterectomies within the MSQC registry that were performed for benign indications, as per the operative note, were eligible for inclusion. Cases were excluded if they were associated with preoperatively diagnosed malignancies, if hysterectomy was performed for cancer or obstetrical indications, if hysterectomy was performed through an abdominal route, or if they were classified as urgent or emergent cases. Cases were also excluded if the hysterectomy was performed on a weekend (Saturday or Sunday) because they were deemed to have a higher likelihood of having been performed under unique or extenuating circumstances, even if they were not classified as urgent or emergent cases. Finally, cases were excluded if they were missing patient clinical or perioperative data that were used to develop the prediction models.
The primary outcome (dependent variable in all analyses and prediction models) was SDD, defined as discharge from the hospital on the date of the procedure. Potential predictors of SDD were determined a priori and placed into the following attribute groupings based on clinical relevance: intraoperative, surgeon, hospital, surgical timing, patient clinical, patient socioeconomic, and patient geographic attributes. For example, the intraoperative attribute grouping contained hysterectomy route, conversion to laparotomy, estimated blood loss (EBL), and operative time as potential predictors.
Continuous predictors were transformed into categorical variables on the basis of clinical relevance or previous research studies. Hysterectomy route was categorized into laparoscopic, robotic-assisted laparoscopic, laparoscopic-assisted vaginal, and vaginal approaches. Length of surgery was categorized into quartiles based on values from the entire dataset. EBL was categorized by every 100 mL as <100, 100 to 199, 200 to 299, 300 to 399, 400 to 499, and ≥500 mL. Surgeon annual hysterectomy volume was represented by the annual volume quartile for individual surgeons in each year relative to other surgeons in that year. Similarly, annual hysterectomy volume was represented by the annual volume quartile for the hospital in each year relative to other hospitals in that year. This was performed to account for variations in surgeon and hospital volume across years. Metropolitan status of the hospital was determined by the United States Office of Management and Budget core-based statistical area information (CENSUS 2010). Age was categorized by decile as 18 to 29, 30 to 39, 40 to 49, 50 to 59, 60 to 69, 70 to 79, and ≥80 years. Body mass was dichotomized into nonobese and obese using a cutoff of 30 kg/m 2 , as defined by the National Institutes of Health (Conner 2021). American Society of Anesthesiologists (ASA) classification was dichotomized into classes <3 and ≥3. Surgery start time was categorized as 06:00 to 09:59, 10:00 to 13:59, 14:00 to 17:59, 18:00 to 23:59, and 00:00 to 05:59 hours, local time. Given collinearity between surgical start time and end time, we chose to use surgical start time only because it is a more easily modifiable predictor. Patient race was self-reported and categorized as White or non-White. Insurance type was classified as private insurance, Medicare, Medicaid, or both Medicare and Medicaid. The distance between the patient’s home address and the operating hospital’s address was calculated using Bing Maps ( http://dev.virtualearth.net/REST/V1/Routes , accessed September 17, 2021) with a method similar to those used in previous studies , Because of the large number of subjects in our study, a custom algorithm was developed to automatically clean and standardize input addresses from the source dataset, make automated application program interface calls to the Bing Maps server, and record distance and time outputs as determined by the Bing Maps backend. Response results produced by the algorithm were compared with results from manual query for 150 randomly selected cases, which ensured accuracy of the results. Distances were categorized by every 5 km for distances of 0 to 49 km, by every 10 km for distances of 50 to 99 km, and by 50 km for distances >99 km.
Bivariate analysis was performed for all potential predictors using the chi square and Fisher exact tests, as appropriate. Hierarchical multivariable logistic regression models were fitted to adjust for risk factors independently associated with SDD. In hierarchical modeling, clustering of SDD practice at the hospital and surgeon level (random effects) was considered alongside clinically relevant patient-level factors (fixed effects). Models were developed using: ( ) predictors from each attribute category individually, ( ) all predictors (ie, composite model), and ( ) all predictors with the individual exclusion of each attribute category. Receiver operator characteristic (ROC) curves were generated for each model, and the model performance was assessed by the area under the ROC curve (AUC) or the concordance index. The Hanley–McNeil test was used to compare the AUC between different models. A P value of.05 was considered statistically significant. All data were analyzed using STATA (v17.0; StataCorp, College Station, TX).
Results
Of the 23,513 patients in the MSQC database who met the inclusion criteria for our study, 5062 (21.5%) had SDD ( Figure 1 ). Preoperative and intraoperative characteristics of patients who had SDD and those who did not are compared in Table 1 . Patients who were discharged on the same day were more likely than those who were not to have had a laparoscopic rather than vaginal hysterectomy, a shorter operative time, a lower EBL, a surgeon with higher annual hysterectomy volume, and a hospital with higher annual hysterectomy volume. They were also more likely to have had surgery at a metropolitan hospital (vs micropolitan or rural hospital), but there was no association with teaching hospital status. The proportion of patients who had SDD increased from 12.2% in 2012 to 35.0% in 2018 ( Table 2 ).

Attribute groupings and predictors | No same-day discharge | Same-day discharge | P value |
---|---|---|---|
N=18,451 | N=5062 | ||
A. Intraoperative attributes | |||
Hysterectomy route | <.001 | ||
Laparoscopic | 3043 (16) | 1187 (23) | |
Robotic | 10,521 (57) | 3402 (67) | |
Laparoscopic-assisted vaginal | 2618 (14) | 344 (7) | |
Vaginal | 2269 (12) | 129 (3) | |
Conversion to laparotomy | 723 (4) | 4 (<1) | <.001 |
Total operative time (min) by quartile | <.001 | ||
First quartile (16–83) | 3698 (20) | 1954 (39) | |
Second quartile (84–113) | 4574 (25) | 1514 (30) | |
Third quartile (114–153) | 4962 (27) | 1036 (20) | |
Fourth quartile (154–702) | 5217 (28) | 558 (11) | |
Estimated blood loss (mL) | <.001 | ||
<100 mL | 9387 (51) | 3652 (72) | |
100–199 mL | 5213 (28) | 1015 (20) | |
200–299 mL | 1919 (10) | 252 (5) | |
300–399 mL | 782 (4) | 68 (1) | |
400–499 mL | 399 (2) | 46 (1) | |
≥500 mL | 751 (4) | 29 (1) | |
B. Surgeon attributes | |||
Surgeon annual hysterectomy volume | <.001 | ||
First quartile (1–9 cases) | 5029 (27) | 883 (17) | |
Second quartile (10–17 cases) | 4830 (26) | 1170 (23) | |
Third quartile (18–29 cases) | 4809 (26) | 1067 (21) | |
Fourth quartile (30–110 cases) | 3783 (21) | 1942 (38) | |
C. Hospital attributes | |||
Hospital annual hysterectomy volume | <.001 | ||
First quartile (2–81 cases) | 5157 (28) | 751 (15) | |
Second quartile (82–115 cases) | 4695 (25) | 1251 (25) | |
Third quartile (116–171 cases) | 4704 (25) | 976 (19) | |
Fourth quartile (172–338 cases) | 3895 (21) | 2084 (41) | |
Metropolitan status | <.001 | ||
Metro | 16,555 (90) | 4852 (96) | |
Micro | 1491 (8) | 163 (3) | |
Rural | 405 (2) | 47 (1) | |
Teaching hospital | 2239 (12) | 639 (13) | .35 |
D. Patient clinical attributes | |||
Age (y) | <.001 | ||
18–29 | 373 (2) | 143 (3) | |
30–39 | 3750 (20) | 1385 (27) | |
40–49 | 8009 (43) | 2333 (46) | |
50–59 | 3485 (19) | 872 (17) | |
60–69 | 1885 (10) | 259 (5) | |
70–79 | 849 (5) | 68 (1) | |
≥80 | 130 (1) | 2 (<1) | |
BMI ≥30 kg/m 2 | 8737 (47) | 2419 (48) | .58 |
ASA classification | <.001 | ||
1,2 | 14,831 (80) | 4187 (83) | |
3+ | 3620 (20) | 875 (17) | |
Diabetes mellitus | 1478 (8) | 263 (5) | <.001 |
COPD | 534 (3) | 103 (2) | <.001 |
Tobacco use | 3911 (21) | 1276 (25) | <.001 |
Hypertension | 4989 (27) | 968 (19) | <.001 |
Personal Hx of DVT/PE | 531 (3) | 97 (2) | <.001 |
Bleeding disorders | 194 (1) | 31 (1) | .004 |
E. Surgical timing attributes | |||
Surgery start time | <.001 | ||
06:00–09:59 | 9874 (54) | 2925 (58) | |
10:00–13:59 | 6717 (36) | 1750 (35) | |
14:00–17:59 | 1781 (10) | 381 (8) | |
00:00–05:59; 18:00–23:59 | 79 (<1) | 6 (<1) | |
Surgery day of week | <.001 | ||
Monday | 3818 (21) | 1075 (21) | |
Tuesday | 3947 (21) | 733 (14) | |
Wednesday | 3980 (22) | 926 (18) | |
Thursday | 3523 (19) | 1126 (22) | |
Friday | 3183 (17) | 1202 (24) | |
F. Patient socioeconomic attributes | |||
Race | <.001 | ||
Non-White | 2693 (15) | 444 (9) | |
White | 15,758 (85) | 4618 (91) | |
Insurance type | <.001 | ||
Private | 9692 (53) | 2917 (58) | |
Medicare | 5513 (30) | 1463 (29) | |
Medicaid | 3090 (17) | 607 (12) | |
Medicare and Medicaid | 156 (1) | 75 (1) | |
G. Patient geographic attributes | |||
Distance from patient home to hospital (km) | <.001 | ||
5 | 3236 (18) | 690 (14) | |
10 | 2698 (15) | 649 (13) | |
15 | 2227 (12) | 532 (11) | |
20 | 1932 (10) | 498 (10) | |
25 | 1602 (9) | 452 (9) | |
30 | 1397 (8) | 455 (9) | |
35 | 1037 (6) | 352 (7) | |
40 | 918 (5) | 304 (6) | |
45 | 694 (4) | 192 (4) | |
50 | 968 (5) | 329 (6) | |
60 | 554 (3) | 194 (4) | |
70 | 380 (2) | 127 (3) | |
80 | 230 (1) | 58 (1) | |
90 | 149 (1) | 57 (1) | |
100 | 315 (2) | 135 (3) | |
150 | 114 (1) | 38 (1) |
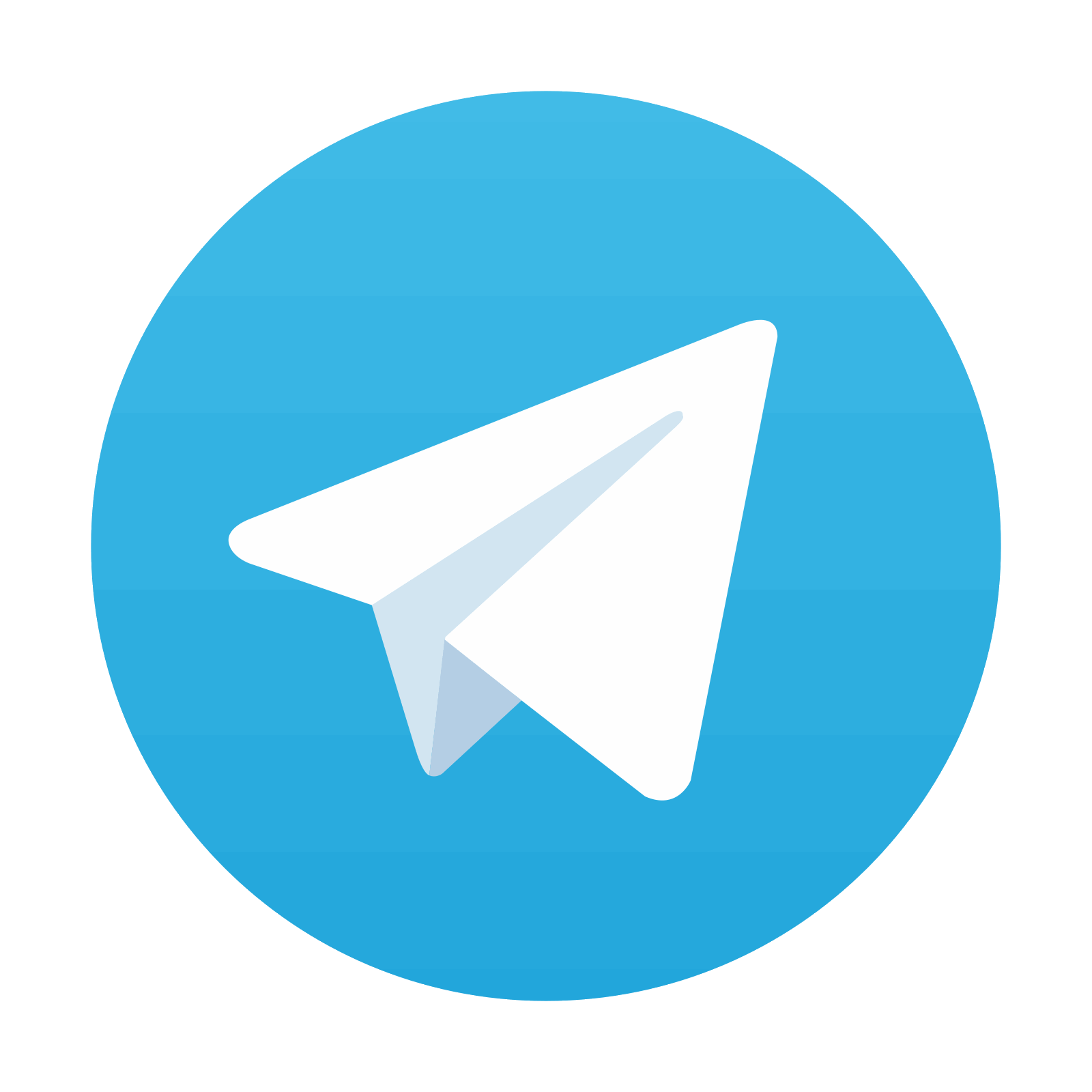
Stay updated, free articles. Join our Telegram channel

Full access? Get Clinical Tree
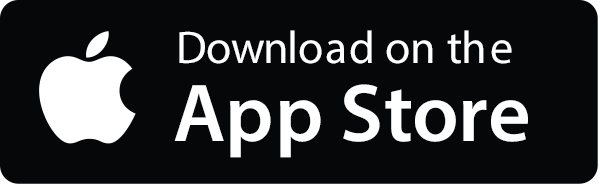

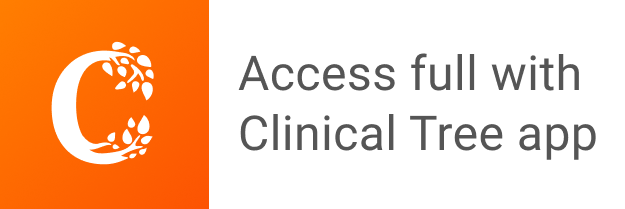