Background
Spontaneous preterm birth remains the main driver of childhood morbidity and mortality. Because of an incomplete understanding of the molecular pathways that result in spontaneous preterm birth, accurate predictive markers and target therapeutics remain elusive.
Objective
This study sought to determine if a cell-free RNA profile could reveal a molecular signature in maternal blood months before the onset of spontaneous preterm birth.
Study Design
Maternal samples (n=242) were obtained from a prospective cohort of individuals with a singleton pregnancy across 4 clinical sites at 12–24 weeks (nested case-control; n=46 spontaneous preterm birth <35 weeks and n=194 term controls). Plasma was processed via a next-generation sequencing pipeline for cell-free RNA using the Mirvie RNA platform. Transcripts that were differentially expressed in next-generation sequencing cases and controls were identified. Enriched pathways were identified in the Reactome database using overrepresentation analysis.
Results
Twenty five transcripts associated with an increased risk of spontaneous preterm birth were identified. A logistic regression model was developed using these transcripts to predict spontaneous preterm birth with an area under the curve =0.80 (95% confidence interval, 0.72–0.87) (sensitivity=0.76, specificity=0.72). The gene discovery and model were validated through leave-one-out cross-validation. A unique set of 39 genes was identified from cases of very early spontaneous preterm birth (<25 weeks, n=14 cases with time to delivery of 2.5±1.8 weeks); a logistic regression classifier on the basis of these genes yielded an area under the curve=0.76 (95% confidence interval, 0.63–0.87) in leave-one-out cross validation. Pathway analysis for the transcripts associated with spontaneous preterm birth revealed enrichment of genes related to collagen or the extracellular matrix in those who ultimately had a spontaneous preterm birth at <35 weeks. Enrichment for genes in insulin-like growth factor transport and amino acid metabolism pathways were associated with spontaneous preterm birth at <25 weeks.
Conclusion
Second trimester cell-free RNA profiles in maternal blood provide a noninvasive window to future occurrence of spontaneous preterm birth. The systemic finding of changes in collagen and extracellular matrix pathways may serve to identify individuals at risk for premature cervical remodeling, with growth factor and metabolic pathways implicated more often in very early spontaneous preterm birth. The use of cell-free RNA profiles has the potential to accurately identify those at risk for spontaneous preterm birth by revealing the underlying pathophysiology, creating an opportunity for more targeted therapeutics and effective interventions.
Introduction
Despite ongoing research efforts, preterm birth (PTB) still affects millions of pregnancies every year, with the rates remaining unchanged or increasing over the past 20 years. , Spontaneous preterm birth (sPTB) contributes to two-thirds of these births and is the leading cause of neonatal morbidity and short- and long-term complications later in life. The underlying etiology of PTB is complex, and currently, its single-best predictor is a previous medical history of PTB. However, a large number of reported pregnancies that delivered preterm occurred in the absence of any known risk, and risk assessment in nulliparous women remains difficult. Thus, the development of predictive tools that are independent of pregnancy history to identify pregnancies from the antenatal population at risk of sPTB is of clinical relevance. These tools would allow for early pregnancy stratification with different pathways of surveillance and access to prophylactic interventions if needed.
Why was this study conducted?
Spontaneous preterm birth (sPTB) remains the main driver of childhood morbidity and mortality, and there is incomplete understanding of the underlying biology. This study sought to determine if a cell-free RNA (cfRNA) profile could reveal a molecular signature in maternal blood months before the onset of sPTB.
Key findings
This study has successfully identified distinct RNA profiles in maternal plasma that are predictive of early and very early sPTB, implicating different biological pathways that drive the underlying pathophysiology.
What does this add to what is known?
A deep transcriptomic characterization of sPTB contributes to its better mechanistic understanding by identifying biological events that precede early delivery. This approach to molecular diagnostics could also help guide patient management and preventive and therapeutic interventions by triggering treatments tailored to the observed molecular pathophysiology.
Currently, ultrasonography measurements of cervical length (CL) and tests for fetal fibronectin (fFN) in the cervicovaginal fluid are the most widely used clinical tools for sPTB risk prediction in the second trimester. , In particular, molecular tests such as fFN are useful as a rule-out method because of a high negative predictive value within 2 weeks of delivery. Indeed, a combination of CL and quantitative fFN measurement with clinical parameters using the QUantitative Innovation in Predicting Preterm birth (QUiPP) algorithm has proven to be useful to inform management, particularly for threatened preterm labor. , Although of use, it is neither the standard of care in the United States nor recommended by the American College of Obstetricians and Gynecologists or the Society for Maternal-Fetal Medicine. Beside, to date, no clinically available molecular tests can reliably predict the risk of sPTB early in pregnancy. For instance, proteomics approaches on the basis of the insulin-like growth factor-binding protein 4 (IBP4)/sex hormone-binding globulin ratio still require body mass index (BMI) stratification and are not specific to sPTB , , and the promising early-pregnancy cervicovaginal metabolite markers require additional external validation. , Similarly, noninvasive RNA-based methods, particularly cell-free RNA (cfRNA), , are promising tools but require validation in ethnically diverse populations. Given the complex etiology of this heterogeneous syndrome, it would be advantageous to develop predictive tests that provide insight on the specific pathophysiology that leads to PTB for each particular pregnancy. Such an approach could inform the development of preventive treatments and targeted therapeutics that are currently lacking or are difficult to implement because of the heterogeneous etiology of sPTB.
This study aimed to identify potential plasma cfRNA biomarkers to predict sPTB and inform our understanding of the underlying pathophysiology.
Materials and Methods
Study design and cohort
The “Insight: investigation into biomarkers for the prediction of spontaneous preterm birth” study is an ongoing observational cohort study designed to study women at a high risk of sPTB and low-risk controls. Using a nested case-control design, the sPTB cases were matched with 2 high-risk term controls and 1 low-risk term control; additional controls from the same cohort were also added. Matching was done in a prioritized order on the basis of ethnicity, BMI, smoking status, and maternal age. The participants were asked to list their ethnicity (The United Kingdom uses the term ethnicity rather than race). They were classified into groups as follows: White-European, Indian, Pakistani, Bangladeshi, Black-Caribbean, Black African, Middle Eastern, Far East Asian, South-East Asian, or Other. Because of the small numbers in some of the groups, we grouped them into Asian, Black, White and other. Plasma samples (taken within 12–23 +6 weeks of gestation) were identified for the current analyses from women with singleton pregnancies recruited from 4 tertiary antenatal clinics in the United Kingdom. High-risk pregnancies were defined by at least 1 of the following: previous sPTB or late miscarriage (between 12 and 37 weeks of gestation), previous destructive cervical surgery, or incidental finding of a CL <25 mm on transvaginal ultrasound scan. Women with no risk factors for sPTB and otherwise well at the time of enrollment were recruited as low-risk controls from routine antenatal or ultrasonography clinics at these centers. The exclusion criteria for both the high- and low-risk groups were multiple pregnancies, known major congenital fetal abnormalities, rupture of membranes, or current vaginal bleeding. The pregnancy outcome data were obtained from case note reviews by trained clinical staff and were monitored by dedicated clinical research team members; ambiguous cases were referred to a senior clinician for the final decision. Women were considered to have had a sPTB if labor was spontaneous in onset or there was premature rupture of membranes and they delivered before 37 weeks of gestation (including late miscarriages), regardless of the mode of delivery. Women with iatrogenic deliveries (including those because of maternal medical conditions and intrauterine fetal demise) were excluded from the case group only if delivery occurred before the specific gestational outcome of interest (n=21, < 37 weeks). Approval from London City and the East Research Ethics Committee was granted (13/LO/1393). Informed written consent was obtained from all the participants.
Sample collection
On the basis of an estimated due date from a first trimester ultrasound, blood samples were collected between 12 and 24 weeks of gestation (242 blood samples, 1 sample per pregnancy). For sPTB cases, the samples were collected on average 9.4 ± 5.3 weeks before delivery. The samples were collected in EDTA tubes and centrifuged to separate plasma within 4 hours from collection. Blood was centrifuged at 2500 g for 10 minutes at 4°C, and plasma aliquots were stored at −80°C until further processing.
Cell-free RNA extraction and library preparation
For a detailed description of the Mirvie RNA platform, refer to the study by Rasmussen et al. To obtain cfRNA, frozen plasma was briefly thawed on ice before cfRNA extraction using the Mirvie RNA platform. Then, the circulating plasma or serum and exosomal RNA purification kit (Norgen Biotek, Ontario, Canada) was briefly used followed by DNAse treatment using Baseline-ZERO DNase (Lucigen, Middleton, WI). An RNA spike-in was added to the samples during extraction. After DNAse treatment, cfRNA was eluted using the RNA Clean and Concentrator-5 kit (Zymo Research, Irvine, CA). cfRNA libraries were prepared using the SMARTer Stranded Total RNAseq-Pico Input Mammalian kit (Takara Bio, San Jose, CA) and enriched for the human transcriptome using the SureSelect Target Enrichment kit (Agilent Technologies, Santa Clara, CA).
The extracted cfRNA and libraries were quality-control (QC)-monitored using a reverse transcription-quantitative polymerase chain reaction (RT-qPCR) assay to follow 3 targets of interest as follows: a housekeeping gene (actin beta [ ACTB ]), an RNA spike-in, and a cfDNA contamination assay. The library quality was also assessed using a Fragment Analyzer® system (Agilent Technologies, Santa Clara, CA). Libraries of multiple samples were then pooled and sequenced to an average depth of 30 million reads on the Illumina NovaSeq platform. Individual samples more than 3 standard deviations from the mean in QC metrics were removed as outliers. On average, we obtained at least 1 count for 14,620 genes after removing duplicate reads. There were no significant differences in sequencing depth between the cases and controls ( P =.2). Raw counts were normalized by log 2 (Counts Per Million +1).
Computational analysis
The sequencing reads were demultiplexed (bcl2fastq) and trimmed (trimmomatic v0.36) to remove sequencing adaptors. The reads were then mapped to the human genome (GRCh38) using STAR (v2.6.1). The mapped reads were deduplicated to remove bias because of sequencing amplification and other artifacts (Picard MarkDuplicates v2.18.3). Finally, a table containing the number of reads mapping to each human transcript was obtained with HTSeq-count v0.11.2 using a gene transfer format annotation with Ensembl 89 release genes. All samples used in this study passed sequencing QC metrics. Downstream analyses were performed using custom python and R scripts.
Statistical analysis
No statistical analysis was used to predetermine the sample size, and the samples were not blinded for analysis. A nonparametric Mann-Whitney U-test was used to determine significance across all cohort demographic metrics except for the ethnicity frequency ( Table 1 ) where a Chi-square test of independence was used (SciPy package, Python). To test for significance in the survival analysis using a Kaplan-Meier analysis, a Kolmogorov-Smirnov test of samples predicted to deliver preterm vs those predicted to deliver at term was performed on the cumulative distribution function.
Characteristic | Combined (all sites) | ||
---|---|---|---|
Cases | Controls | P value | |
Pregnancies (#) | 46 | 194 | — |
% White | 52.2 | 59.8 | .64 |
% Black | 30.4 | 27.3 | |
% Asian | 10.9 | 9.8 | |
% Unknown | 6.5 | 3.1 | |
% Total | 100 | 100 | |
Low risk at enrollment (%) | 10.9 | 56.2 | — |
GA at blood draw (wk) | 18.9±1.9 | 20.0±1.7 | 1.98×10 −4 |
Time from blood draw to delivery (wk) | 9.2±5.4 | 19.6±1.9 | 2.70×10 −25 |
GA at delivery (wk) | 28.1±6.0 | 39.6±1.3 | 5.40×10 −26 |
Body mass index (kg/m 2 ) | 27.2±6.0 | 26.5±6.0 | .54 |
Maternal age (y) | 33.5±6.0 | 32.8±5.4 | .62 |
% primigravida | 6.5 | 31.4 | 1.15×10 −3 |
Gene discovery and preterm birth classifier
Early spontaneous preterm birth gene discovery
Differential expression (DE) was performed using DESeq2. All P values were corrected for multiple hypothesis testing using the Benjamini-Hochberg procedure. Gene discovery and model performance were validated through leave-one-out cross validation (LOOCV).
Briefly, in each LOOCV iteration, we selected the top 100 DE genes between the sPTB cases and controls using DESeq2. These genes were filtered on the basis of their absolute median log fold change (|logMFC|>1) and used to build a classifier using L1-regularized logistic regression (Lasso). The filtering step increased the robustness of the model by selecting genes with large effect sizes. This feature discovery, filtering and modeling process was repeated for each cross-validation loop. The confidence intervals (CIs) of the receiver operating characteristic (ROC) curve were determined through bootstrapping (5000 iterations).
Very early spontaneous preterm birth gene discovery
DE analysis was performed in a subset of samples that included n=16 very early sPTB <25 weeks (which included late miscarriage >16 weeks), n=32 early sPTB between 25 and 35 weeks, and n=194 term birth ≥37 weeks. Given the reduction of cases of very early sPTB to one-third of those available for early sPTB, we performed an additional analysis where we excluded samples with preeclampsia (PE) from the control population and samples with low QC metrics, leading to the exclusion of 29 full-term samples and 6 sPTB samples; of these, 2 are very early PTB cases. The effect size genes were identified on the basis of maximizing both the up-regulated and down-regulated effect size of the summed log 2 counts per million across the genes, which was subsequently pruned by an L1-regularized logistic regression. Very early sPTB genes were identified as the effect size genes common across 2 comparisons within the training dataset. In 1 comparison, samples with very early sPTB (<25 weeks of gestational age [GA]) were compared against samples with term birth (≥37 weeks GA). In the second comparison, the samples with very early sPTB (<25 weeks GA) were compared against samples with delivery ≥ 25 weeks GA. A final model on the basis of the combined genes across the 2 comparisons was then trained on very early sPTB samples vs term births using an L1-regularized logistic regression model to prevent overfitting. The model performance was validated with LOOCV, and the CIs of the ROC were determined through bootstrapping as for the early sPTB model.
Pathway analysis
Pathway analysis was performed using overrepresentation analysis with the Reactome and Gene Ontology (Cellular Component) databases. All the P values were corrected for multiple hypothesis testing (Benjamini-Hochberg procedure), and pathways with a false discovery rate (FDR) <0.15 supported by multiple genes were deemed to be significant.
Results
In this study, we recruited 242 pregnant women, and we collected a single blood sample during the second trimester of their pregnancy ( Figure 1 ). Out of 242 pregnancies, 194 delivered at term (≥37 0/7 GA), and 48 spontaneously delivered preterm before 35 weeks gestation (early preterm, <35 0/7 ). A subset of 16 of these pregnancies delivered before 25 weeks gestation (very early preterm, <25 0/7 ). As the focus of this study is to develop a predictor for sPTB, we excluded the samples that had a medically-induced PTB because of PE and that delivered before the aforementioned cut-offs (2 cases in early preterm modeling <35 0/7 and 0 cases for very early preterm modeling <25 0/7 ). The control population included 12 suspected cases of PE who delivered at or after 37 0/7 . Late PTBs (≥35 0/7 and <37 0/7 ) were also excluded from the study to improve the identification of molecular markers specific to early sPTB ( Figure 1 ).

There were no significant differences in the BMI, maternal age or ethnicity between the cases and controls. There were significantly more nulliparous women in the controls because of the inclusion of low-risk pregnancies in the control population ( Table 1 ). By design, there was a significant difference in the GA at delivery between the cases and controls. We also observed a small but significant difference in the GA at collection (8 days on average) ( Table 1 ); this relates to the setting that women were recruited from, as low-risk women were identified at the 20-week routine ultrasound scan.
To identify the candidate genes that can be predictive of the risk of early sPTB (<35 0/7 ), we performed DE analyses between all the early deliveries (<35 0/7 ) and controls (≥37 0/7 ) and validated the results using LOOCV (Methods). This led to a list of 25 genes ( Supplemental Table 1 ) that were used to build a logistic regression classifier to predict the risk of PTB. The model achieved a validated LOOCV performance area under the curve (AUC) of 0.80 (95% CI, 0.72–0.87) ( Figure 2 , A) with sensitivity =0.76 and specificity =0.72 (n=46 early sPTB cases and n=194 at-term controls). The model also scored each sample with a risk probability of preterm delivery ( Figure 2 , B). The distribution of probabilities showed a significant separation between the cases and controls ( P =1.7×10 −10 ), spanning the whole range of probabilities. A sensitivity analysis showed that the performance of the model is not affected by the GA at collection ( Supplemental Figure 1 ) and that equivalent performance is obtained when excluding the preterm premature rupture of membranes (PPROM) population ( Supplemental Figure 2 ). We validated that the observed RNA profile and model performance are independent of other conditions such as PE by excluding all samples that developed PE from the control population finding a similar AUC=0.78 (95% CI, 0.69–0.85) ( Supplemental Figure 3 ).

Next, we asked whether our approach could also be used to identify molecular markers specific to very early sPTB (<25 weeks). To do so, we ran a DE analysis using the 16 very early PTB cases and 226 controls (Methods). This led to a list of 65 unique genes shared across the folds ( Supplemental Table 2 ) that were used to build a regularized logistic regression classifier to predict very early sPTB in cross validation. The model achieved a validated LOOCV performance of AUC=0.74 (95% CI, 0.64–0.83) ( Supplementary Figure 4 ). We found several genes in the list previously related to PE. To reduce crosstalk in the cfRNA signature given that 5% of the non-very early sPTB had preeclampsia, we repeated modeling excluding all the PE samples in addition to the samples with low-quality sequencing metrics, which reduced to 14 cases of very early preterm (<25 0/7 ). This led to a model with an improved LOOCV performance with AUC=0.76 (95% CI, 0.63–0.87) [sensitivity =0.64, specificity =0.80] for 14 very early sPTB cases (<25 0/7 ) and 193 samples that delivered at or after 25 weeks (≥25 0/7 ) ( Figure 2 , C). The model was based on a set of 39 genes ( Supplemental Table 3 ), from which a core set of 3 genes ( AC011043.1 , IGFBP2 , and SH3GL3 ) was identified in >95% of cross-validation folds ( Supplemental Table 3 provides an extended list of genes) and 13 genes overlapped with the genes discovered when training with the PE samples. The model probabilities showed a significant difference between the cases and controls, though we observed a longer tail of high sPTB probabilities for a subset of control samples ( Figure 2 , D).
To illustrate the utility of these predictors, we performed a Kaplan-Meier analysis to follow the delivery date for pregnancies predicted to be at risk of PTB ( Figure 3 ). This analysis shows that samples predicted to be at risk of early sPTB in our model (<35 0/7 ) have a higher probability of delivering early and significantly deviate from those predicted to deliver at term (≥37 0/7 ) ( P =1.8×10 -6 ). A similar trend is observed for the very early preterm predictor (<25 0/7 ) when compared with samples that do not deliver very early (≥25 0/7 ) ( P =.065).

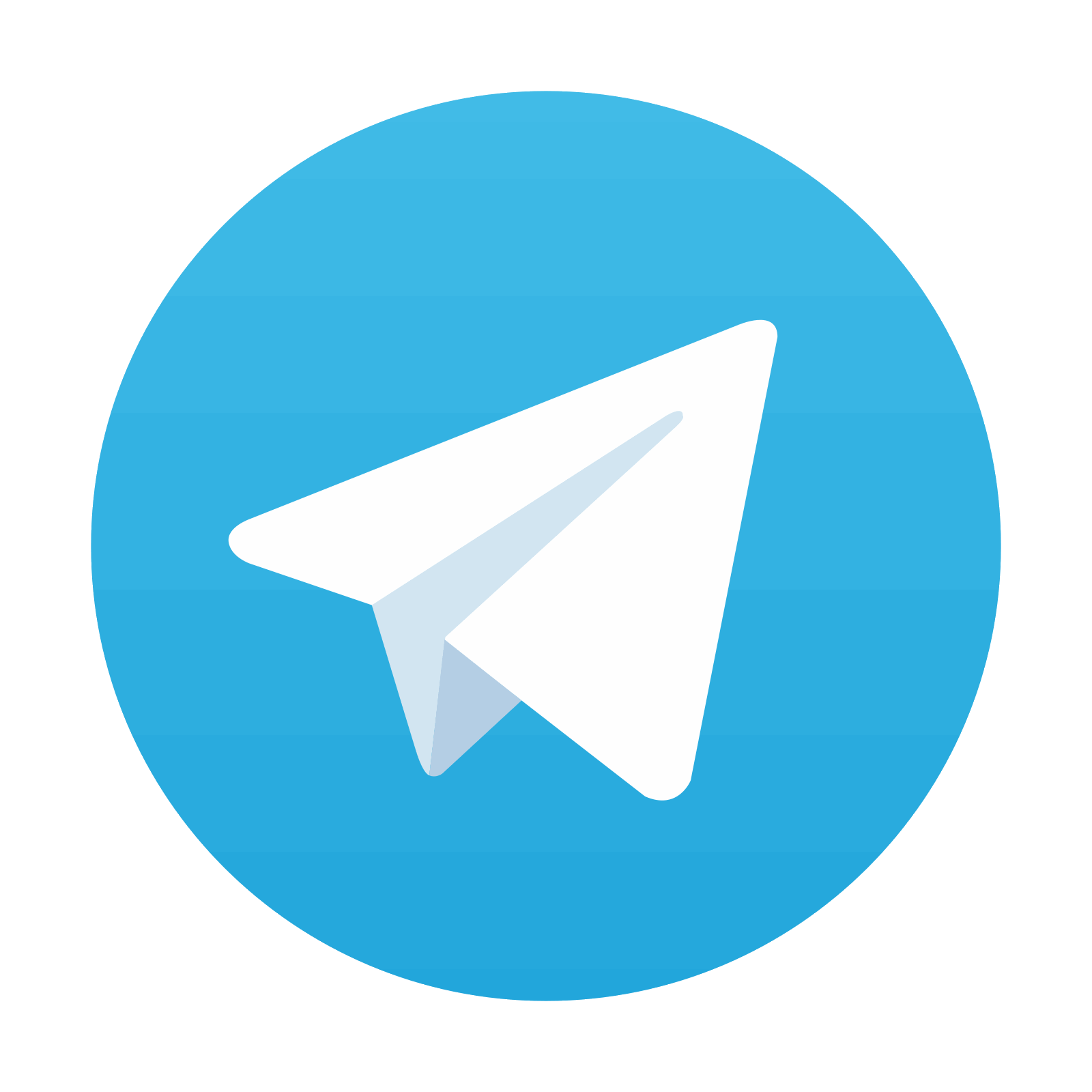
Stay updated, free articles. Join our Telegram channel

Full access? Get Clinical Tree
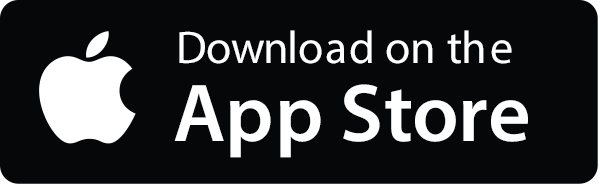
