Materials and Methods
Study population
We performed a retrospective analysis of 238,315 newborn metabolic screening records collected by the Iowa Newborn Screening Program between 2004 and 2009. Forty-four metabolites were measured on all subjects during the entire study period, including 2 enzymes (biotinidase and galactose-1-phosphate uridyl transferase), 2 hormones (thyroid-stimulating hormone [TSH] and 17-hydroxyprogesterone [17-OHP]), 9 amino acids, 30 acylcarnitines, and free carnitine (C0) ( Supplementary Table 1 ). Blood spot specimens were collected, dried, and handled as part of routine clinical care according to the Clinical Laboratory Standards Institute guidelines. At the time of neonatal screening, the health care provider records the gestational age in weeks, the sex of the infant, current weight in grams, if the infant is currently on total parenteral nutrition, and age of the newborn in hours. This information is included with each newborn screening specimen. Data such as delivery mode or maternal characteristics were not available. The method of gestational dating, ie, last menstrual period or fetal ultrasound, is provider dependent and is not distinguished on the newborn screen record. All specimens were analyzed as part of the Iowa Newborn Screening Program by the State Hygienic Laboratory in Ankeny, Iowa. Screening procedures in Iowa are based on previously established methodology.
The State Hygienic Laboratory identified multiple gestations by examining birth date, gestational age, mother’s first name, and facility identification number. The data were deidentified by the State Hygienic Laboratory and provided for use in this study. Approval for use of the deidentified data was obtained from the Iowa Department of Public Health and a waiver of consent from the Institutional Review Board at the University of Iowa (IRB#200908793).
Only initial newborn screening specimens, not repeats, were included in the analysis. We excluded screening records with missing gestational age data (n = 5749) or those with a recorded gestational age day outside the range of 20−45 completed weeks (n = 108). Records for specimens that were rejected by the screening laboratory as being of poor quality (n = 2445) were excluded from analysis. The remaining dataset consisted of 230,013 neonatal metabolic screening records. To determine the final performance of the predictive model, the data were randomly split into a model-building dataset (n = 153,342) and a model-testing dataset (n = 76,671). The predictive model was created using the model-building dataset and the performance of this model was then evaluated in the model-testing dataset.
Statistical analysis
Univariate analysis was performed with each metabolite and gestational age. Linearity between gestational age and single metabolite levels was inspected visually by plots of the residuals vs the predicted values. To address nonlinearity between each metabolite and gestational age, squared terms and then the cubed terms were included for each model. We performed multiple linear regression modeling with gestational age, in weeks, as the outcome measure, using metabolites that were significant in the univariate analysis. The regression was estimated by the use of ordinary least squares. In the model-building dataset, all metabolites significant at P < .01 from the univariate models were included in the initial model, and significant terms ( P < .05) were retained for subsequent modeling. Squared and cubed terms of significant metabolites were included successively in the model after which nonsignificant ( P > .05) terms were removed. Cubic terms were examined only when squared terms were significant.
Next, within the model-building dataset, we determined whether the final selected model was robust in the presence of covariates that could affect the prediction of gestational age by the metabolic panel. These covariates included the child’s sex, age at time of sample collection (in hours, month, and year of sample collection), neonatal weight at time of screening in grams, weight for gestational age categorized as small-for-gestational age (<10th percentile for each gestational age week), large-for-gestational age (>90th percentile for each gestational age week), and average-for-gestational age and multiple gestation. Residuals vs the predicted values were inspected visually for the relationship between gestational age and age at time of screening as well as gestational age with weight. To address nonlinearity between age and weight with gestational age, squared terms and then the cubed terms were included for each model.
We performed a sensitivity analysis excluding newborns identified as potentially affected with an endocrine disorder or inborn error of metabolism, ie, one or more metabolite levels exceeded the threshold considered within the normal range for a healthy newborn. Statistical outliers also were evaluated with studentized residuals by excluding those observations with residuals less than −1.96 and greater than 1.96. The coefficient of determination (R 2 ) and the root-mean-square error (RMSE) are presented for each regression model. All analyses were performed in STATA version 12.0 (College Station, TX).
The final model was used to predict gestational age in the model-testing dataset (n = 76,671). The coefficient of determination (R 2 ) and the RMSE are presented for the entire model-testing dataset as well as stratified by sex and weight for gestational age. Sensitivity and specificity were calculated by gestational age cut-points (in weeks) for prediction of preterm birth less than <37 weeks’ gestation compared with term birth (≥37 weeks). Because birthweight can also predict gestational age, we evaluated the sensitivity and specificity with each preterm birth outcome in a model that included only infant weight and its squared and cubed terms. Next, we evaluated the sensitivity and specificity of each preterm birth outcome using a model that contained the final set of metabolites plus weight and their subsequent squared and cubed terms to examine the extent to which adding weight improves prediction over that based on the metabolites alone.
Results
General characteristics of the population and the newborn metabolic model
Gestational age distributions were comparable in both the model-building and model-testing datasets, with 8.9% of the total population being born preterm (<37 weeks) ( Supplementary Table 2 ). Distributions of neonatal weight, small- and large-for-gestational age, sex, total parenteral nutrition, and age at time of screening were similar in both the model-building and model-testing datasets ( Supplementary Table 2 ). All univariate analyses between each metabolite (including the squared and cubed terms) with gestational age were significant at P < .01 (data not shown). Within the model-building dataset, the full model including 1 categorical variable (biotinidase), 43 continuous metabolite measurements, and their squared and cubed terms produced comparable performance statistics (R 2 = 53.2%, RMSE = 1.3) to the metabolic model including only significant terms (R 2 = 53.1%, RMSE = 1.3). Therefore, our final newborn metabolic linear regression model consisted of 88 parameters, including the intercept, 37 metabolite measures, 29 squared metabolite measures, and 21 cubed metabolite measures ( Table 1 ). Metabolites in the final model included TSH, 17-OHP, galactose-1-phosphate uridyl transferase, 7 amino acids, and 27 acylcarnitines.
Metabolite | Metabolite | Metabolite Squared | Metabolite Cubed | |||
---|---|---|---|---|---|---|
Coefficient | SE | Coefficient | SE | Coefficient | SE | |
TSH | 0.01 | 8.8×10 -4 | −8.8×10 -5 | 1.2×10 -5 | 1.3×10 -7 | 2.3×10 -8 |
17-OHP | −0.05 | 5.2×10 -4 | 1.1×10 -4 | 3.5×10 -6 | N/A | N/A |
GALT | −0.07 | 0.01 | 3.5×10 -3 | 7.5×10 -4 | N/A | N/A |
ALA | 9.6×10 -3 | 2.7×10 -4 | −1.3×10 -5 | 6.3×10 -7 | 5.3×10 -9 | 3.7×10 -10 |
ARG | −0.02 | 1.5×10 -3 | 2.9×10 -5 a | 1.0×10 -5 | N/A | N/A |
LEU | −0.01 | 3.3×10 -4 | 1.7×10 -5 | 8.3×10 -7 | −4.3×10 -9 | 2.1×10 -10 |
MET | 0.03 | 1.2×10 -3 | −2.3×10 -4 | 1.2×10 -5 | 3.5×10 -7 | 1.8×10 -8 |
PHE | −0.01 | 8.3×10 -4 | 4.2×10 -5 | 4.7×10 -6 | −2.9×10 -8 | 3.9×10 -9 |
TYR | −5.3×10 -3 | 1.2×10 -4 | N/A | N/A | N/A | N/A |
VAL | 0.02 | 6.0×10 -4 | −3.6×10 -5 | 2.5×10 -6 | 2.0×10 -8 | 1.0×10 -9 |
C2 | −0.10 | 4.4×10 -3 | 2.0×10 -3 | 1.1×10 -4 | −1.2×10 -5 | 8.5×10 -7 |
C3 | 0.06 | 5.6×10 -3 | N/A | N/A | N/A | N/A |
C4 | −0.20 | 0.03 | N/A | N/A | N/A | N/A |
C5 | −9.29 | 0.26 | −6.93 | 0.78 | 6.81 | 0.43 |
C5:1 | −3.89 | 0.56 | N/A | N/A | N/A | N/A |
C5-OH | −0.36 | 0.08 | N/A | N/A | N/A | N/A |
C3-DC | 0.91 | 0.10 | N/A | N/A | N/A | N/A |
C4-DC | 18.56 | 0.41 | −50.43 | 1.67 | 40.19 | 1.92 |
C5-DC | −25.06 | 0.92 | 85.79 | 8.60 | −118.09 | 23.90 |
C6 | 10.16 | 0.31 | −2.24 | 0.42 | N/A | N/A |
C8 | −1.47 | 0.20 | 0.11 | 0.02 | −2.0×10 -3 | 3.0×10 -4 |
C8:1 | 13.29 | 0.50 | −52.96 | 2.51 | 55.61 | 3.64 |
C10 | 4.99 | 0.32 | −10.87 | 0.91 | 3.97 | 0.52 |
C10:1 | −2.33 | 0.27 | N/A | N/A | N/A | N/A |
C12 | 2.47 | 0.19 | −4.00 | 0.37 | 2.10 | 0.22 |
C12:1 | 5.17 | 0.30 | −13.00 | 0.85 | 9.71 | 0.72 |
C6-DC | −4.20 | 0.36 | 9.65 | 2.13 | N/A | N/A |
C14 | −3.03 | 0.32 | 1.94 | 0.48 | N/A | N/A |
C16 | 2.99 | 0.07 | −0.58 | 0.02 | 0.04 | 1.5×10 -3 |
C16:1 | −9.73 | 0.55 | 18.06 | 1.63 | −10.40 | 1.59 |
C18 | −7.96 | 0.23 | 4.96 | 0.20 | −1.02 | 0.05 |
C18:1 | 4.09 | 0.18 | −1.70 | 0.11 | 0.24 | 0.02 |
C18:2 | −4.22 | 0.19 | 3.01 | 0.35 | −1.22 | 0.17 |
C14-OH | 27.02 | 1.83 | −287.34 | 35.03 | N/A | N/A |
C16-OH | 10.91 | 2.58 | −239.18 | 62.78 | 1585.56 a | 460.58 |
C16:1-OH | 7.32 | 1.09 | 18.72 b | 8.87 | N/A | N/A |
C18:1-OH | 3.84 | 0.82 | N/A | N/A | N/A | N/A |
Constant | 36.72 | 0.11 | N/A | N/A | N/A | N/A |
Model performance in model-building dataset
The newborn metabolic model explained 53.1% of the variation in gestational age in the model-building dataset. The average difference between gestational ages predicted by the newborn metabolic model and the recorded gestational age was 1.3 weeks ( Table 2 ). The prediction of gestational age by the use of the newborn metabolic model was robust to inclusion of covariates. Specifically, including month and year of sample collection, multiple gestations, infant age at time of sample collection in hours, infant sex, and weight for gestational age did not improve the prediction of gestational age. Each of these covariates alone, explained very little of the variation in gestational age (R 2 < 5%; RMSE ∼ 1.9 weeks). Sensitivity analyses demonstrate that gestational age estimated by the newborn metabolic model was not impacted by exclusion of newborns with potential endocrine disorders or inborn errors of metabolism, newborns receiving total parenteral nutrition or multiple gestations ( Table 2 ). The removal of statistical outliers unsurprisingly improved model performance (R 2 = 55.4; RMSE = 1.1); however, there was no reason to suspect that the metabolite values of these individuals were not true values and we chose not to remove these individuals from further analyses.
Model description | No. model parameters a | No. observations | R 2 (%) | RMSE |
---|---|---|---|---|
Final metabolite model | 88 | 153,342 | 53.1 | 1.3 |
Final metabolite model excluding infants with abnormal neonatal screens | 88 | 148,104 | 50.1 | 1.3 |
Final metabolite model excluding infants on total parenteral nutrition | 88 | 151,293 | 47.9 | 1.3 |
Final metabolite model excluding statistical outliers as defined as those with residuals less than −1.96 and greater than 1.96 | 88 | 146,083 | 55.4 | 1.1 |
Final metabolite model + month and year of collection | 104 | 153,342 | 53.4 | 1.3 |
Month and year of collection only | 17 | 153,342 | 1.2 | 1.9 |
Final metabolite model + multiple gestation status (yes/no) | 89 | 153,342 | 53.7 | 1.3 |
Multiple gestation status only | 2 | 153,342 | 4.8 | 1.9 |
Final metabolite model including only singleton births | 88 | 147,597 | 49.4 | 1.3 |
Final metabolite model + age at collection (hours) | 91 | 153,322 | 53.1 | 1.3 |
Age at collection (hours) only | 4 | 153,322 | 0.05 | 1.9 |
Final metabolite model in subset with nonmissing age at collection | 88 | 153,322 | 53.1 | 1.3 |
Final metabolite model + infant sex | 89 | 152,674 | 53.2 | 1.3 |
Infant sex only | 2 | 152,674 | 0.02 | 1.9 |
Final metabolite model in subset with non-missing infant sex | 88 | 152,674 | 53.1 | 1.3 |
Final metabolite model + infant weight, g | 91 | 153,008 | 66.1 | 1.1 |
Infant weight, g only | 4 | 153,008 | 54.5 | 1.3 |
Final metabolite model + weight for gestational age (AGA, LGA, and SGA) | 90 | 153,008 | 53.1 | 1.3 |
Weight for gestational age (AGA, LGA, and SGA) only | 3 | 153,008 | 0 | 1.9 |
Final metabolite model in subset with nonmissing weight | 88 | 153,008 | 52.9 | 1.3 |
Comparison of the newborn metabolic model to including only neonatal weight in the model-building dataset
In the model-building dataset, neonatal weight alone explained 54.5% of the variation in gestational age. The average difference between gestational ages predicted by neonatal weight alone and the recorded gestational age was 1.3 weeks ( Table 2 ). Including neonatal weight in the newborn metabolic model improved the difference between the predicted gestational ages and recorded gestational age by 0.2 weeks and explained 66.1% of the variation in gestational age. Inclusion of neonatal metabolite measurements explained an additional 12% of the variation in gestational age above and beyond neonatal weight ( Table 2 ).
Performance of the newborn metabolic model in the model-testing dataset
In the model-testing dataset, the newborn metabolic model estimated gestational age within 1 week for 78% of the individuals and within 2 weeks of gestation for 95% of the individuals. One individual had a predicted gestational age of 172 weeks, which was likely attributable to the fact that this individual had multiple abnormal metabolite levels. We excluded this individual from subsequent evaluation of model performance. In the model-testing dataset, the newborn metabolic model (n = 76,670) predicted 52.8% of the variation in gestational age. The average difference between the gestational ages predicted by the newborn metabolic model and the recorded gestational age was 1.3 weeks, which was comparable (R 2 = 52.8%, RMSE = 1.3) to the model including the excluded individual (n = 76,671).
Comparison of the newborn metabolic model to including only neonatal weight in the model-testing dataset
In the model-testing dataset neonatal weight alone explained 54.6% of the variation in gestational age. The average difference between gestational ages predicted by neonatal weight alone and the recorded gestational age was 1.3 weeks. Similar to the findings in the model-building dataset, including neonatal weight in the newborn metabolic model improved the difference between the predicted gestational ages and recorded gestational age by 0.2 weeks in the model-testing dataset. The model including neonatal weight with the newborn metabolic model explained 66.2% of the variation in gestational age in the model-testing dataset. Therefore, inclusion of neonatal metabolite measurements explained an additional 12% of the variation in gestational age above and beyond neonatal weight.
The overall estimated prevalence of preterm birth (5.0%) when we used the newborn metabolic model was slightly closer to the percentage of preterm birth (8.9%) estimated with the recorded gestational age than the model including only neonatal weight (4.7%). The newborn metabolic model yielded an area under the curve (AUC) of 0.899 (95% confidence interval [95% CI] 0.895−0.903) in differentiating those born preterm (<37 weeks) from those born term (≥37 weeks) ( Figure and Supplementary Table 3 ). The newborn metabolic model offers slight improvement in identifying those born preterm (<37 weeks) over the model including only neonatal weight (AUC 0.881, 95% CI 0.876−0.886). Including neonatal weight in the newborn metabolic model provides the best estimation of gestational age with an AUC of 0.938 (95% CI 0.934−0.941).

Performance of the newborn metabolic model in neonates born very preterm or small-for-gestational age
The newborn metabolic model underestimated very preterm birth (<32 weeks) compared with the model including only neonatal weight ( Table 3 ). There was no increased ability to discriminate between very preterm birth (<32 weeks) and all other births (≥32 weeks) using the newborn metabolic model over the model including only neonatal weight ( Table 3 and Supplementary Figure ).
Gestational age | Actual gestational age | Metabolic model | Weight-only model | ||||||
---|---|---|---|---|---|---|---|---|---|
n | % | Cum% | n | % | Cum% | n | % | Cum% | |
≤32 | 1177 | 1.54 | 0.00 | 674 | 0.88 | 0.00 | 891 | 1.16 | 0.00 |
33−34 | 1271 | 1.66 | 3.19 | 876 | 1.14 | 2.02 | 604 | 0.79 | 1.95 |
35−36 | 4364 | 5.69 | 8.88 | 2291 | 2.99 | 5.01 | 2089 | 2.72 | 4.67 |
37−38 | 18,927 | 24.69 | 33.57 | 20,994 | 27.38 | 32.39 | 15,306 | 19.96 | 24.64 |
39−40 | 44,151 | 57.59 | 89.62 | 50,600 | 66.00 | 97.51 | 57,613 | 75.14 | 98.62 |
>40 | 6781 | 8.84 | 100.00 | 1235 | 1.61 | 99.99 | 0 | 0.00 | 99.78 |
Missing | 0.00 | 0.00 | 100.00 | 1 | 0.00 | 100.00 | 168 | 0.22 | 100.00 |
The most evident difference in model performance was in small- and large-for-gestational age neonates. The average difference between gestational ages predicted by the newborn metabolic model and the reported gestational age was 1.5 weeks compared with the model with only neonatal weight (RMSE = 1.9 weeks). In large-for-gestational age neonates, the average difference between gestational ages predicted by the newborn metabolic model and the reported gestational age was 1.4 weeks, an improvement over the model with only neonatal weight (RMSE = 1.8 weeks).
Improvement over the model including only neonatal weight also was observed when we examined the predicted prevalence of preterm birth in the subset of small-for-gestational age neonates ( Table 4 ). The model including only neonatal weight overestimated the prevalence of very preterm birth (<32 weeks) by over twice that of the actual prevalence (3.45% vs 1.61%, respectively), whereas the newborn metabolic model only marginally underestimated the prevalence of very preterm birth compared with the actual prevalence (1.00% vs 1.61%, respectively). For preterm birth <37 weeks’ gestation, the model including only neonatal weight estimated the prevalence at 18.79% in small-for-gestational age neonates, over twice that of the actual prevalence of 9.20%. Again the newborn metabolic model underestimated preterm birth but was closer to the true prevalence at 6.94% than the models including only neonatal weight ( Table 4 ).
Gestational age | Actual gestational age | Metabolic model | Weight-only model | ||||||
---|---|---|---|---|---|---|---|---|---|
n | % | Cum% | n | % | Cum% | n | % | Cum% | |
≤32 | 121 | 1.61 | 1.61 | 75 | 1.00 | 1.00 | 259 | 3.45 | 3.45 |
33−34 | 132 | 1.76 | 3.37 | 122 | 1.63 | 2.63 | 199 | 2.65 | 6.11 |
35−36 | 437 | 5.83 | 9.20 | 324 | 4.32 | 6.94 | 952 | 12.69 | 18.79 |
37−38 | 1831 | 24.41 | 33.60 | 2421 | 32.27 | 39.22 | 5861 | 78.13 | 96.92 |
39−40 | 4304 | 57.37 | 90.98 | 4481 | 59.73 | 98.95 | 231 | 3.08 | 100.00 |
>40 | 677 | 9.02 | 100.00 | 79 | 1.05 | 100.00 | 0 | 0.00 | 100.00 |
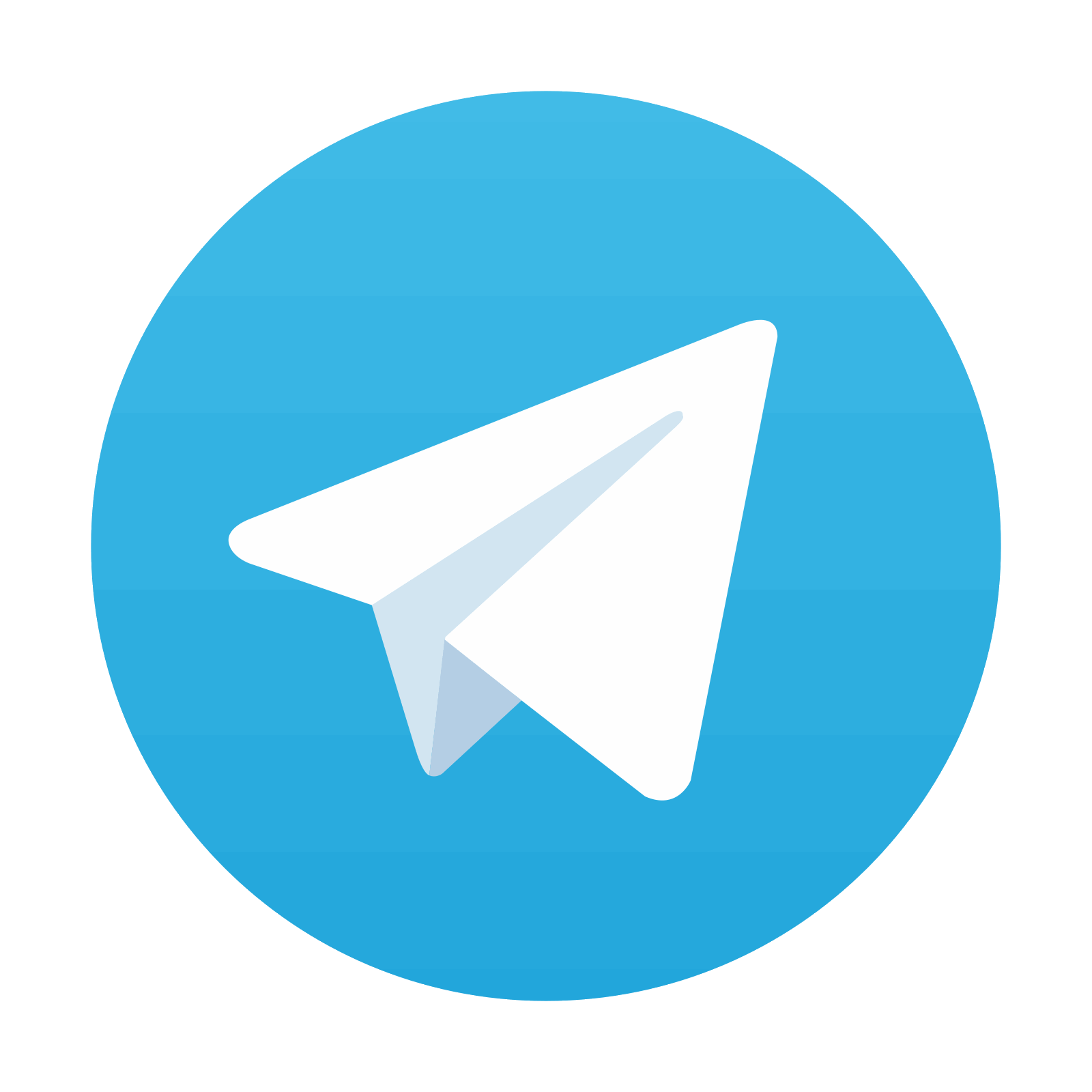
Stay updated, free articles. Join our Telegram channel

Full access? Get Clinical Tree
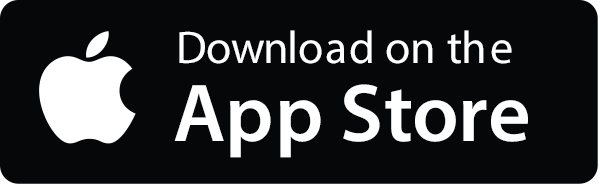
