33 Dharmintra Pasupathy Department of Women and Children’s School of Life Course Sciences, King’s College London, London, UK John Last in the Dictionary of Epidemiology defines epidemiology as the ‘The study of the distribution and determinants of health‐related states or events in specified populations, and the application of this study to the control of health problems’. It does not represent a body of knowledge or a specific organ system such as cardiology or neurology but instead represents methodology focused on improved understanding of the determinants of illness and disease. Modern epidemiology remains a young discipline of science. Although many excellent studies were conducted before, it was in the twentieth century where highly informative epidemiological studies were performed that have significantly informed clinical practice and public health. This includes, amongst others, the Framingham Heart Study, an ambitious cohort study which commenced in 1948 with the primary objective of identifying common factors or characteristics that contribute to cardiovascular disease (CVD) by following its development over a long period of time in a large group of participants, who had not yet developed overt symptoms of CVD or suffered a heart attack or stroke. In the original cohort of 5209 men and women between the ages of 30 and 62 from the town of Framingham, Massachusetts, extensive physical examinations and lifestyle interviews were conducted that were used to study the common patterns related to CVD development. From 1948 onwards, the original participants returned at regular intervals for collection of detailed information on medical history, clinical examination and laboratory tests. Subsequent second‐generation participants were also recruited and in 2002 the study entered a new phase, the enrolment of a third generation of participants, the grandchildren of the original cohort. In the UK there have also been large‐scale cohort studies, including the MRC‐funded Richard Doll study in the 1950s, in which a cohort of general practitioners were followed up to study the influence of smoking on health and disease [1]. This pioneering work has had a global impact and was key in understanding the harmful effects of smoking. The UK is also home to a number of large‐scale cohort studies, including the longest running birth cohort in the world, the 1946 Birth Cohort, and the Southampton Women’s Survey, which recruited women prior to conception. In the MRC Cohort Strategic Review in 2014 it was reported that approximately 3.5% of the UK population are cohort members [2]. Reproductive and perinatal epidemiology is a branch of epidemiology that focuses on diseases and disorders of reproduction and/or events in the perinatal period. Perinatal epidemiology includes the study of both maternal and neonatal events. The interrelated nature of human reproduction and development provides the opportunity to develop well‐designed prospective cohort studies with collection of longitudinal data at key periods of development in pregnancy and following birth, to understand the impact of pregnancy and development on long‐term maternal and offspring health. The Avon Longitudinal Study of Parents and Children (ALSPAC), also known as Children of the 90s, based at the University of Bristol is a world‐leading birth cohort study in which, between April 1991 and December 1992, more than 14 000 pregnant women were recruited. These women and the children of ALSPAC have since been followed up over two decades with annual questionnaires for the mothers, fathers and the children from age 5. Over 1200 publications have been published from data collected in this cohort. Perinatal medicine has also been informed by randomized controlled trials including some large international multicentre studies. These have informed and improved our obstetric practice in many areas, including the management of severe pre‐eclampsia (MAGPIE Trial 2002), fetal growth restriction (GRIT Trial 2003, TRUFFLE Trial 2015) and breech delivery (Term Breech Trial 2000). This chapter provides an overview of the different study design and quantitative methodologies available to address many of the key research questions in the discipline. Finally, it also provides an overview of the commonly used definitions, statistical methodology and inferences. The premise of any research question or hypothesis is based on the relationship between an exposure and outcome of interest. In epidemiology, exposures are potential causal characteristics. It can refer to treatment (pharmacological and non‐pharmacological behavioural interventions), behaviour (e.g. smoking, drug use), genotype or trait (e.g sickle cell disease, BRCA1 gene mutation) or environmental (e.g. dust, pollution). Outcome refers to either disease states (e.g stillbirth) or clinical phenotypes that have the potential to increase adverse outcomes (e.g small for gestational age). Study design is defined as the process in which a researcher or research team translates the hypothesis of interest through a detailed project plan into an operational study. The types of research conducted are broadly divided into observational and interventional research (Fig. 33.1). An observational study is when research is focused on the collection of health and socio‐demographic variables related to exposure and outcome. These variables can range from population‐based data (e.g census data) to specifically designed health‐related questionnaires, clinical examination and collection of biomarker samples. Specifically in observational studies the data available from the subjects in the study are not dependent on an intervention administered by the study investigators. Observational research can further be categorized as descriptive or analytical. Descriptive studies are cross‐sectional studies that report the incidence or prevalence of health‐related states at a specific time point. They do not attempt to provide an association between the exposure and outcome. Observational studies which are analytical in nature are focused on determining the relationship between exposure and outcome through different study designs. In contrast, interventional studies are studies in which the investigator specifically provides an intervention with the aim of exploring the effect of the intervention on health‐related outcomes. The choice of study design adopted by researchers will depend on the type of exposure and outcome being studied. All types of observational and interventional studies are described in detail in the following sections. Fig. 33.1 Study design. Cross‐sectional studies involve observation of a total population, or a random subset of it, at a defined time. They provide information on an entire population under study and can describe absolute risks and not just relative risks. They can also describe the prevalence of disease. National audits of maternal and perinatal deaths are variants of cross‐sectional surveys [3]. Cohort studies are a form of longitudinal observational study used to analyse risk factors by studying groups of people who do not have the disease either retrospectively or prospectively (the preferred method). These are analytical observational studies in which the comparison of health outcomes between groups is defined by the exposure of interest. Participants in the cohort are defined by exposure and are followed up for a specific period. In pregnancy, examples of cohort studies in recent years include the SCOPE cohort (Screening for Pregnancy Endpoints), a multicentre international prospective cohort study of nulliparous women, and the POPS study (Pregnancy Outcome Predictive Study), also a prospective cohort of low‐risk nulliparous women in Cambridge [4,5]. Both cohorts are focused on identifying predictors of preterm birth, pre‐eclampsia and small‐for‐gestational‐age (SGA) infants. SCOPE is primarily focused on early pregnancy factors associated with the outcomes of interest, whilst in the POPS study longitudinal clinical data, serum biomarker samples and ultrasound measurements were collected throughout pregnancy which may also provide mechanistic insight to development of disease. The cohort can also be selected by a specific event such as year of birth (a birth cohort). Examples include the 1946 Birth Cohort and the ALPSAC study previously described. The cohort design, which can be either retrospective or prospective, allows the study of multiple outcomes and exposures and specifically rare exposures (e.g. asbestos exposure). It is costly and not appropriate for rare diseases with a long latent period for the development of the outcome of interest. Case–control studies can be used for both retrospective and prospective studies. In a case–control study, participants are selected on the basis of the outcome of interest. People with the disease are cases and appropriate controls are selected without the outcome of interest to allow comparisons to be made between exposures of interest. Often controls are matched for characteristics within the group to reduce the bias in the comparison between the groups. For example, in the determinants of factors associated with endometrial cancer in postmenopausal women (cases), healthy controls are matched for age. Data are then collected in both groups and factor(s) that are different between the groups may demonstrate an association with the outcome of interest. Matching in case–control studies precludes the factor in which the groups are matched to be explored as a factor associated with the disease. In the example cited, the effect of age on endometrial cancer cannot be determined. In common with all observational studies, case–control studies do not prove causation but instead demonstrate association. The strength of association, coupled with biological plausibility, are some of the criteria used to suggest association as reported by the Bradford Hill Criteria (Table 33.1) [6]. Table 33.1 Bradford Hill Criteria. Inherent within the design of case–control studies, the selection of cases and controls by the investigator precludes any estimation of disease incidence. However, relative risk can be demonstrated between the groups. The control group is a sample of the total population and selected because they do not have the disease. To determine true population risk (incidence of the disease in a population) a larger cross‐sectional study or cohort study is required. A variation of this method is the nested case–control study, where cases with a disease in a defined cohort (the nest) are identified and, for each, a specified number of matched controls are selected from the same cohort who have not yet developed the disease. The nested case–control design is easier and less expensive than a full cohort approach. Case–control studies are also particularly useful for rare diseases and diseases with long latency periods. If well designed, this approach will also allow examination of multiple exposures of interest. The temporal sequence of exposure and disease can sometimes be difficult to study in a case–control study and certainly the size of the study will need to be sufficiently large to study rare exposures, which often limits this design in some settings. A retrospective study is one where the records are studied after all the events and outcomes have already occurred. The data are collected from a given population. Risk factors or disease outcomes are compared between subgroups that have different known outcomes. The study can be designed using a case–control or cohort model. This differs from a prospective study, which is conducted by starting with two groups that are selected by risk factor or randomized and subsequent future outcomes are noted. Retrospective studies have the following benefits: they are cheap, it is easier to collect large numbers and to select cohorts of disease and non‐disease, and they are less time‐consuming since the main effort required is the collection of data. However, they are limited by incomplete collection of data and recall bias. In contrast, prospective studies follow populations over time to study outcome. In population risk studies, a large number of negative controls will be collected depending on the disease incidence. Although the costs of these studies are significantly higher, they provide an opportunity to specifically tailor data collection. Issues of confounding and bias limit all observational studies. A well‐designed study does have the potential to limit these issues. Confounding is defined as the alternative explanation to the association found between exposure and outcomes. A confounder must be associated with both the exposure and the outcome and not be on the causal pathway between the exposure and the outcome. An example of a confounder in the association between coffee drinking and cancer is smoking. It is recognized that coffee drinkers are more likely to smoke and smoking is certainly associated with cancer. Identifying potential confounders allows investigators to design and collect data appropriately, which then has the potential to minimize confounding. At the design stage this includes restricting the study population, matching in case–control studies and randomization in interventional studies. At analysis stratification, standardization and statistical modelling (regression) are methods that can be utilized to minimize confounding. However, this is reliant on the data collected on potential confounders. Bias is a systematic error that leads to a result which does not represent the truth (e.g. information bias, bias in data collection and reporting, selection of study participants). Unfortunately, bias cannot be corrected through statistical analysis and can only be minimized by appropriate study design. Randomized controlled trials (RCTs) are a type of interventional study design with superior methodology in medical statistical research because they reduce the potential for bias by random selection of participants to one intervention arm or to another intervention, non‐intervention or placebo arm. The participants can be patients, health volunteers or communities (randomized cluster trials). This minimizes the possibility that confounding variables will differ between the two groups. However, not all studies are suitable for RCTs and the methodologies mentioned previously may be more suitable. In order to further reduce bias, the randomized trial may be designed as a double‐blind RCT, where neither the clinician nor the participant knows which treatment arm the participant is in, or a single‐blind RCT, where the clinician knows but the participant does not. In some circumstances an open label trial is carried out, where it is not possible to blind either the clinician or the patient but randomization is performed without bias at time of therapy. Independent trials although well powered and designed at conception, occasionally do not demonstrate an effect of the intervention. Often trials are also repeated with conflicting results. Meta‐analysis provides the opportunity to pool results from different studies if sufficiently similar (heterogeneity) with respect to trial population and outcomes of interest. This approach increases the power to detect smaller differences that were not identified in individual studies. It also increases the precision of the effect size observed. The weighted average of the combined effect size is estimated. This is related to the sample size of the individual studies and the effect size observed. This process of meta‐analysis can be part of a systematic review, for example Boulvain et al. [7] reported to the Cochrane Collaboration. Figure 33.2 demonstrates the results of a meta‐analysis of the effect of induction of labour at 37–40 weeks for suspected fetal macrosomia on the risk of shoulder dystocia. This is a forest (Peto) plot, a graphical display of the relative strength of treatment effects in the different trials. Down the left‐hand side are the trials included. The trial quoted above is the last of the four trials listed (first author Boulvain). On the right‐hand side is a plot of the risk ratio for each of these studies incorporating confidence intervals represented by horizontal lines. The graph is plotted on a logarithmic scale so that the confidence intervals are symmetrical about the means to prevent apparent exaggeration in ratios greater than 1 when compared with those less than 1. The area of each square is proportional to the study’s weight. The overall meta‐analysed measure of effect is represented as the diamond on the bottom and the lateral points indicate the confidence intervals. A vertical line is plotted at unity and if the confidence intervals for individual studies or the total effect overlap with this line, then the results are not significant. One study included in this meta‐analysis did not study this outcome of interest (LIBBY 1998). Two studies did not reach significance (Gonen 1997, Tey 1995) but the most recent published in 2015 (Boulvain) provided an overall significant result. This is the largest of the four studies. The overall pooled results also suggest a reduction in the risk of shoulder dystocia associated with induction of labour at 37–40 weeks. Fig. 33.2 Summary output from meta‐analysis [7]. The primary principle in statistics that is often not well described nor understood is that analysis performed on a study population represents only a sample of the population. Therefore any findings from a study group or comparison between study groups reflect the population from which the study participants are sampled. The robustness of the sampling process will influence the strength of statistical inference which can be applied from the findings in the study population to the underlying population. An example of this is reflected in the size of the study population. If the sample of study population is small compared with the underlying population or in relation to the frequency of outcome, then the uncertainty of the statistical findings will increase, reflected by wider confidence intervals. Incidence is a measure of the risk of developing some new condition within a specified period of time. Although sometimes loosely expressed simply as the number of new cases during some time period, it is better expressed as a proportion or a rate within a specific denominator to allow meaningful comparison. Therefore, the incidence (rate) is usually given as the number of new cases per given population in a given time period. In the examples above in pregnancy, the population and time period is self‐selecting: the population is pregnant women and the time period is pregnancy and the puerperium. In the non‐pregnant population it is more difficult. The time period is usually fixed at a year but the population denominator is more of a problem. In gynaecology, depending on the disease being studied, the at‐risk female population may be different. Endometriosis is typically, but not absolutely, seen during the reproductive years; it has been estimated that it affects approximately 10% of all women at some point, but this is lifetime risk not incidence, which is risk of new cases within a risk group over a fixed period of time. Nor is it prevalence. Prevalence is a measure of the total number of cases of disease in a specific population at any one period of time, rather than the rate of occurrence of new cases. This indicates the burden of the disease on society and is dependent on both the number of new cases and the length of time the disease is present (prevalence = incidence × duration). This equation demonstrates the relationship between prevalence and incidence: when the incidence goes up, prevalence must also rise. Incidence is more useful in understanding disease aetiology as it reflects disease occurrence and also the response to interventions. In the classical example of the cases of puerperal fever described by Semmelweis [8], the higher incidence in one group suggested an aetiological factor related to that group alone and the fall in incidence that followed the hand‐washing initiative demonstrated a successful intervention. Therefore, incidence will vary with changes in aetiological factors and prevention. Prevalence is dependent on the duration of disease and the availability of cure. The longer the duration of disease, the higher the prevalence. The prevalence is also dependent on the study population. Using endometriosis as an example, its overall prevalence varies, ranging from 5 to 10%. A study of asymptomatic women undergoing sterilization reported a figure of 6% but this rises to 21% in women with infertility and as high as 60% in those with pelvic pain [9]. Endometriosis is recognized to influence fertility and also cause pelvic pain. This would explain the higher prevalence observed in those subgroups. The incidence over time can also be studied using Kaplan–Meier plots, which present incidence data as a plot of cumulative incidence over time, taking into account variations in rate of events. This method was used in our recent study exploring the effects of maternal placental syndrome (MPS) in pregnancy on long‐term incidence of maternal cardiovascular event (CVE) in women with systemic lupus erythematosus (SLE) (Fig. 33.3) [10]. This plot demonstrates the disease‐free incidence over time (probability of CVE‐free survival). In all groups this decreases over time. However, in women with a history of MPS in pregnancy, the disease‐free incidence decreases further, especially if MPS is also associated with preterm delivery less than 34 weeks. This may suggest shared etiological pathways leading to MPS in pregnancy and longer‐term cardiovascular disease. Fig. 33.3 Kaplan–Meier survival estimates for CVE in parous women with SLE [10]. The incidence of a disease or event is often quoted in rate per cent per year, for example the failure rate of contraceptives is quoted using the Pearl Index [11]. The following information is required to calculate the Pearl Index: the number of pregnancies and the total number of months or cycles of exposure of women. The index can then be calculated as follows: These are normally calculated over a trial period of 1–2 years and claims to give the risk of pregnancy in 100 women over 1 year of use or 10 women over 10 years. This assumes that the pregnancy risk is static over the years of use and based on the rate in the first 1–2 years. Again, a Kaplan–Meier plot could be used to test for accumulative pregnancy rates over time when comparing different methods of contraception rather than the Pearl Index alone. In studies comparing outcomes between two or more groups, it is important to determine if any observed difference is simply due to chance or is truly different. A result is called statistically significant if it is unlikely to have occurred by chance alone. In the comparison between two groups, investigators are motivated to determine if there is any difference between groups. In the example on the effect of MPS and long‐term CVE, are the differences observed between the groups merely due to chance or is there a true difference? It is important to remember that being statistically significant does not mean the result is important or clinically relevant. In large studies small differences can be found to be statistically significant but have little clinical or practical relevance. Tests of correlation may show significant correlations but have no or minor causative relation. Tests of significance should always be accompanied by assessments of relevance and effect‐size statistics, which assess the size and thus the practical importance of the difference. Tested often enough, in theory any result is possible but the likelihood that any given result would have occurred by chance is known as the significance level or P‐value. In traditional statistical testing, the P‐value is the probability of observing data similar to that observed by chance alone. If the obtained P‐value is small, then it can be said that this is unlikely and the results are significantly different. To test whether the results are true or not, they are compared with those expected if the null hypothesis is true or there is no difference. So the basis of comparison of data is testing whether the null hypothesis is true and the level of significance desired. Statistical convention assumes that the experimental hypothesis (e.g. that one treatment is better than another) is wrong and assumes the null hypothesis, or no difference, as correct and that testing will assess whether this is wrong (i.e. that the treatment is better). When the null hypothesis is nullified (not supported within accepted confidence limits), the alternative hypothesis (that one treatment is better than the other) is accepted. Therefore, the null hypothesis is generally a statement that a particular treatment has no effect or benefit or that there is no difference between two particular measured variables in a study. The result of the statistical test is given as a P‐value. The size of the P‐value relates to the likelihood of the result occurring by chance. The lower the P‐value, the more likely the null hypothesis is nullified and the results are significantly different. A result of P <0.05 means that the probability of this result being due to chance is less than 5% so the results are different to a 95% probability. The nearer to unity the P‐value, the more likely that the null hypothesis is accepted and that there is no difference, but it should be remembered that ‘the null hypothesis is never proved or established, but is possibly disproved’. In other words, if no significant difference is found, the test has not proven that there is no difference but has failed to show difference. As stated previously, statistical findings are dependent on the population studied. The P‐value of any test is dependent on the degree of difference in the test results and the number of people or values in the trial. If the trial is not of appropriate size, then two basic statistical errors can be made, type I and type II errors. Type I error, also known as the false positive, occurs when a statistical test falsely rejects a null hypothesis, for example where there is no difference between treatment arms in a trial as stated by the null hypothesis but the test rejects the hypothesis, falsely suggesting that there is benefit of treatment. The rate of type I error is denoted by the Greek letter alpha (α) and equals the significance level of the test, which by convention is usually taken as 0.05 or below. This means that any positive result is correct to a level of 95% probability. Type II error, also known as the false negative, occurs when the test fails to reject a false null hypothesis, for example where there is a difference between treatment arms in a trial but the null hypothesis states that there is no difference and the test fails to reject the null hypothesis, falsely suggesting that there is no difference. The rate of type II error is denoted by the Greek letter beta (β) and is usually taken as 0.80, i.e. an 80% chance of rejecting a false null hypothesis. Therefore when designing a trial, two considerations must be assessed: The ability of a study to achieve this is assessed by the power. The power of a statistical test is calculated on the probability that the test will reject the null hypothesis when the null hypothesis is false and not produce a type II error or a false‐negative result. As the power increases, the chance of a type II error occurring decreases as calculated by power = 1 – β. Statistical power may depend on a number of factors but, at a minimum, power nearly always depends on the following three factors: The statistical difference desired is the chosen maximum P‐value where the results are accepted as statistically significant, which is usually 0.05 but may be less than this if multiple testing is to be carried out. Once this P‐value is agreed, power analysis can be used to calculate the minimum sample size required that is likely to detect a specified effect size (or difference). Similarly, the reverse is true and power analysis can be used to calculate the minimum effect size that is likely to be detected in a study using a given sample size. In general, a larger sample size will allow testing for a larger effect size and boost statistical power.
Perinatal Epidemiology and Statistics
Background
Study design
Cross‐sectional studies
Cohort studies
Case–control studies
1
Strength of effect size
A small association does not mean that there is not a causal effect, though the larger the association, the more likely that it is causal
2
Consistency
Consistent findings observed by different persons in different places with different samples strengthens the likelihood of an effect
3
Specificity
Causation is likely if there is a very specific population at a specific site and disease with no other likely explanation. The more specific an association between a factor and an effect, the bigger the probability of a causal relationship
4
Temporality
The effect has to occur after the cause (and if there is an expected delay between the cause and expected effect, then the effect must occur after that delay)
5
Biological gradient
Greater exposure should generally lead to greater incidence of the effect. However, in some cases, the mere presence of the factor can trigger the effect. In other cases, an inverse proportion is observed: greater exposure leads to lower incidence
6
Plausibility
A plausible mechanism between cause and effect is helpful
7
Coherence
Coherence between epidemiological and laboratory findings increases the likelihood of an effect
8
Experiment
Occasionally it is possible to appeal to experimental evidence
9
Analogy
The effect of similar factors may be considered
Randomized controlled trials
Meta‐analysis
Statistics
Incidence and prevalence
Pearl Index
Statistical significance
The null hypothesis and statistical tests
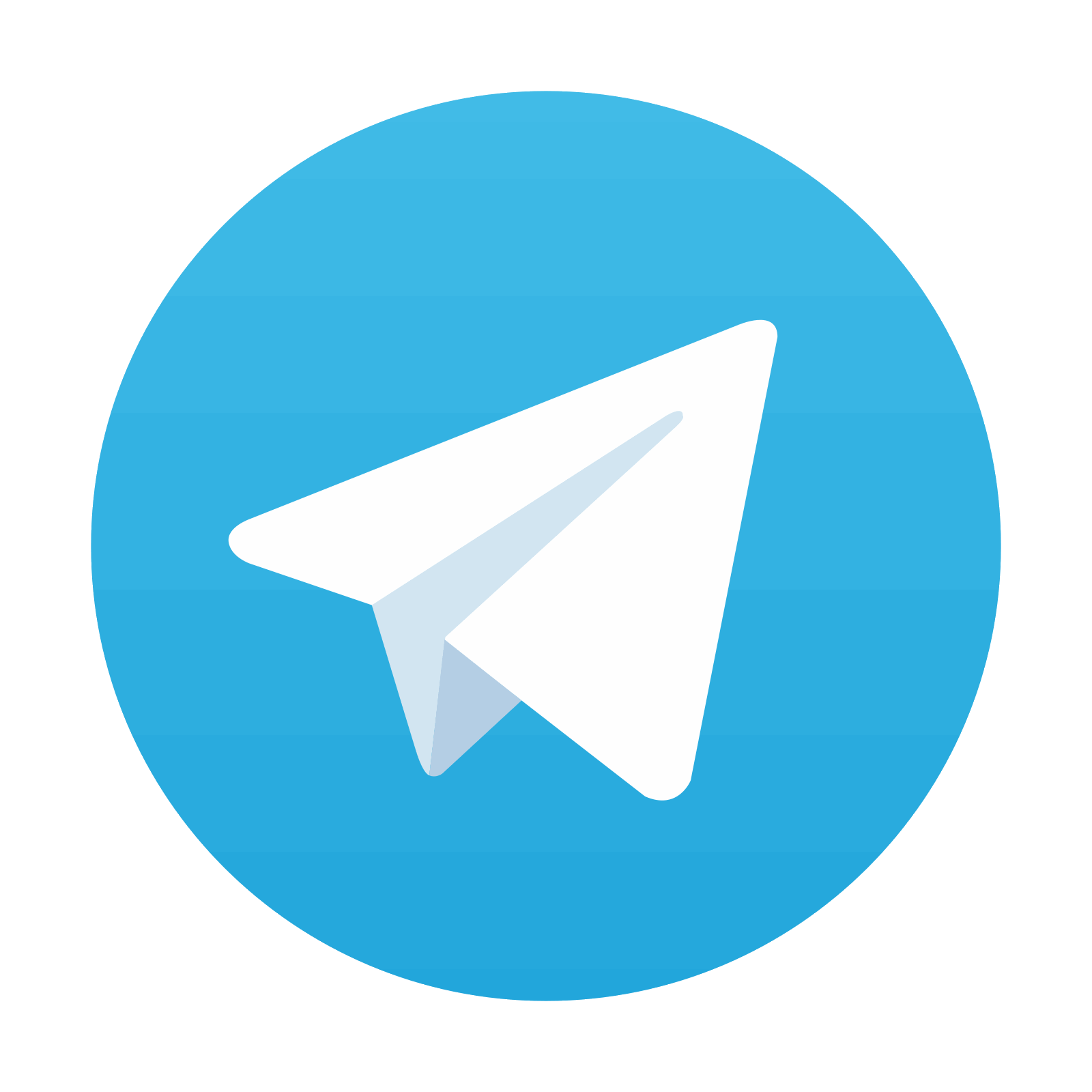
Stay updated, free articles. Join our Telegram channel

Full access? Get Clinical Tree
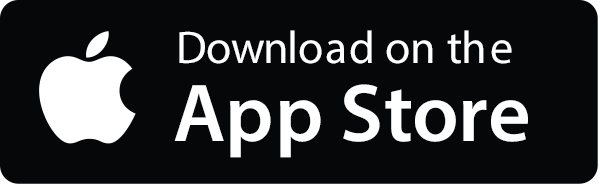
