Objective
Maternal infection is a common complication of childbirth, yet little is known about the extent to which infection rates vary among hospitals. We estimated hospital-level risk-adjusted maternal infection rates (RAIR) in a large sample of US hospitals and explored associations between RAIR and select hospital features.
Study Design
This retrospective cohort study included hospitals in the Perspective database with >100 deliveries over 2 years. Using a composite measure of infection, we estimated and compared RAIR across hospitals using hierarchical generalized linear models. We then estimated the amount of variation in RAIR attributable to hospital features.
Results
Of the 1,001,189 deliveries at 355 hospitals, 4.1% were complicated by infection. Patients aged 15-19 years were 50% more likely to experience infection than those aged 25-29 years. Rupture of membranes >24 hours (odds ratio [OR], 3.0; 95% confidence interval [CI], 3.24−3.5), unengaged fetal head (OR, 3.11; 95% CI, 2.97−3.27), and blood loss anemia (OR, 2.42; 95% CI, 2.34−2.49) had the highest OR among comorbidities commonly found in patients with infection. RAIR ranged from 1.0−14.4% (median, 4.0%; interquartile range, 2.8−5.7%). Hospital features such as geographic region, teaching status, urban setting, and higher number of obstetric beds were associated with higher infection rates, accounting for 14.8% of the variation observed.
Conclusion
Obstetric RAIR vary among hospitals, suggesting an opportunity to improve obstetric quality of care. Hospital features such as region, number of obstetric beds, and teaching status account for only a small portion of the observed variation in infection rates.
Childbirth is the most common reason for hospital admission in the United States, with >4,000,000 admissions for labor and delivery occurring annually. Although most births are uncomplicated, a small but significant number of women experience complications such as infection, trauma, and hemorrhage during childbirth. Reducing obstetric complications has emerged as a national priority in the US, as reflected in goals established by Healthy People 2020 4 and the Centers for Medicare and Medicaid Services’ Partnership for Patients.
For Editors’ Commentary, see Contents
See related editorial, page 427
Maternal infection is one of the most common perinatal complications, affecting nearly 6% of deliveries, and many of these infections may be preventable. Several small studies and reviews have described clinical practices that can increase the risk of infection, primarily related to cesarean deliveries. Some larger epidemiologic studies have estimated overall regional and national obstetric infection rates and still others have explored the associations between complications and factors such as an obstetrician’s residency training site. However, little is known about the extent to which obstetric infection rates vary across hospitals or what impact structural and organizational features of a hospital may have on these rates.
To support the national goal of improving maternal outcomes following childbirth, we used hierarchical generalized linear modeling to estimate risk-adjusted maternal infection rates (RAIR) in a large sample of US hospitals. We then examined whether hospital features, such as the number of hospital beds, teaching status, geographic region, volume of deliveries, and level of implementation of electronic health records (EHR), were associated with higher rates of infection.
Materials and Methods
Study sample and data source
We conducted a cross-sectional study using Perspective, a voluntary, fee-supported database developed by Premier Inc (Charlotte, NC) that enables participating hospitals to analyze care quality and costs at their institution and to compare their performance to other institutions within the database. The database is comprised of a structurally and geographically diverse set of approximately 450 US hospitals that together account for approximately 20% of all annual hospital admissions in the United States. In addition to information derived from standard hospital discharge files (ie, Uniform Billing form-04) Perspective contains a date-stamped log of all items (eg, medications, laboratory, diagnostic tests) and therapeutic services billed to the patient or their insurer.
Women were included in the study if they were discharged from Jan. 1, 2008, through Dec. 31, 2009, and had an International Classification of Diseases, Ninth Revision, Clinical Modification ( ICD-9-CM ) principal or secondary diagnosis or procedure code for a vaginal delivery (650, 640.0x-676.9x [x = 1 or 2], or 73.59) or cesarean delivery (763.4, 669.71, 74.x [x = 0-2, 4], or 74.99). We excluded discharges for ectopic and molar pregnancies and for pregnancies ending in spontaneous or elective abortion because we were interested in exploring intrapartum/peripartum infections. We also excluded patients who were transferred from or to another institution, because we did not have information about the clinical course or treatments prior to admission or subsequent outcomes, and women age <15 or >44 years because 15-44 years is a common age range for childbearing. In addition we excluded hospitals that recorded <100 deliveries over the 2-year study period to provide stable estimates of infection rates, and because these institutions do not routinely provide obstetric care. Permission to conduct the study was obtained from the institutional review board at Baystate Medical Center in Springfield, MA.
Obstetric infection
A delivery was considered complicated by infection if the patient received ≥1 diagnoses consistent with infection using a broad set of ICD-9-CM codes that have been used in earlier studies of infections associated with childbirth ( Appendix ; Supplementary Table 1 ). We excluded ICD-9-CM infection codes with a fifth digit of 3, which indicates an antepartum condition, because we were most interested in risk-adjusted infection rates occurring in the intrapartum/peripartum period as well as the association between these risk-adjusted infection rates and hospital features. We organized infection codes into groups of related diagnoses for descriptive purposes ( Table 1 ). Each infection code was counted toward the overall frequency of each type of infection. When calculating hospital-level infection rates, a patient was considered to either have experienced or not experienced an infectious complication regardless of the number of infection codes associated with a single delivery.
Infection | n (%) |
---|---|
Any infection below | 40,605 (4.1) |
Puerperal infection | 20,519 (2.1) |
Maternal pyrexia | 16,067 (1.6) |
Surgical site infection | 3523 (0.4) |
Infection of genitourinary tract | 1964 (0.2) |
Sepsis | 1319 (0.1) |
Other maternal infection | 1456 (0.2) |
Patient characteristics
We recorded patient demographics (age, gender, race/ethnicity, marital status, and insurance status) and conditions that might confer elevated risk for obstetric infection. We used 2 complementary methods to identify maternal comorbidities and pregnancy-specific conditions that could influence a patient’s risk of infection. The presence of any of 29 comorbidities was computed using Elixhauser Comorbidity Software, version 3.1, developed by the Agency for Healthcare Research and Quality. In addition, we identified the presence of a set of pregnancy-specific conditions that may confer higher risk for infection. These conditions were originally developed to predict risk for cesarean delivery, but have also been used for risk adjustment for infection rates in obstetric patients. For conditions that appeared in both sets, such as hypertension and substance abuse, we created combined indicators for patients identified by either method. Gestational diabetes and diabetes existing prior to pregnancy were assessed separately because they confer different risk for infection. A total of 41 maternal comorbidities and pregnancy-specific conditions were evaluated for inclusion in risk-adjustment modeling ( Table 2 ).
Demographics b | Overall (N = 1,001,189) | Infection present (N = 40,605) | P value a | ||
---|---|---|---|---|---|
n | % | n | (%) | ||
Age, y | < .0001 | ||||
15-19 | 94,738 | (9.5) | 6161 | (15.2) | |
20-24 | 236,439 | (23.6) | 10,892 | (26.8) | |
25-29 | 280,433 | (28.0) | 10,835 | (26.7) | |
30-34 | 232,606 | (23.2) | 8038 | (19.8) | |
35-44 | 156,973 | (15.7) | 4679 | (11.5) | |
Marital status | < .0001 | ||||
Married | 497,959 | (49.7) | 17,283 | (42.6) | |
Single | 363,647 | (36.3) | 18,557 | (45.7) | |
Other/unknown | 139,583 | (13.9) | 4765 | (11.7) | |
Race/ethnicity | < .0001 | ||||
White | 500,170 | (50.0) | 17,434 | (42.9) | |
Black | 153,258 | (15.3) | 7963 | (19.6) | |
Hispanic | 127,105 | (12.7) | 5323 | (13.1) | |
Other | 220,656 | (22.0) | 9885 | (24.3) | |
Insurance | < .0001 | ||||
Managed care | 419,879 | (41.9) | 16,145 | (39.8) | |
Medicaid | 417,643 | (41.7) | 17,895 | (44.1) | |
Medicare | 6957 | (0.7) | 283 | (0.7) | |
Commercial−indemnity | 80,777 | (8.1) | 3002 | (7.4) | |
Self-pay | 26,820 | (2.7) | 979 | (2.4) | |
Other | 49,113 | (4.9) | 2301 | (5.7) | |
Elixhauser comorbidities | |||||
Deficiency anemias | 71,578 | (7.2) | 5528 | (13.6) | < .0001 |
Blood loss anemia b | 70,964 | (7.1) | 7146 | (17.6) | < .0001 |
Valvular disease b | 3941 | (0.4) | 176 | (0.4) | .191 |
Other neurological disorders b | 3771 | (0.4) | 224 | (0.6) | < .0001 |
Rheumatoid arthritis/CVD b | 1660 | (0.2) | 112 | (0.3) | < .0001 |
Paralysis c | 212 | (<0.1) | 16 | (<0.1) | .010 |
Cancer: lymphoma, metastatic, or solid tumor b | 188 | (<0.1) | 17 | (<0.1) | .001 |
Peripheral vascular disease c | 56 | (<0.1) | 5 | (<0.1) | .064 |
Pregnancy risk factors | |||||
Prior cesarean b | 182,821 | (18.3) | 4149 | (10.2) | < .0001 |
Advanced maternal age | 156,973 | (15.7) | 4679 | (11.5) | < .0001 |
Preterm gestation b | 75,730 | (7.6) | 5027 | (12.4) | < .0001 |
Fetal malpresentation | 68,696 | (6.9) | 3175 | (7.8) | < .0001 |
Maternal soft-tissue disorder b | 36,724 | (3.7) | 2196 | (5.4) | < .0001 |
Macrosomia b | 31,931 | (3.2) | 1246 | (3.1) | .158 |
Oligohydramnios b | 31,213 | (3.1) | 1250 | (3.1) | .662 |
Intrauterine growth restriction b | 25,629 | (2.6) | 778 | (1.9) | < .0001 |
Isoimmunization | 26,145 | (2.6) | 981 | (2.4) | .012 |
Herpes b | 21,818 | (2.2) | 1096 | (2.7) | < .0001 |
AP bleed/placental abruption b | 18,657 | (1.9) | 1167 | (2.9) | < .0001 |
Unengaged fetal head b | 18,446 | (1.8) | 2204 | (5.4) | < .0001 |
Multiple gestation b | 18,446 | (1.8) | 909 | (2.2) | < .0001 |
Rupture of membranes >24 h b | 11,820 | (1.2) | 2066 | (5.1) | < .0001 |
Polyhydramnios b | 9442 | (0.9) | 374 | (0.9) | .639 |
Uterine scar unrelated to cesarean | 2082 | (0.2) | 72 | (0.2) | .166 |
Congenital fetal anomaly | 1371 | (0.1) | 65 | (0.2) | .198 |
Maternal pulmonary embolism | 215 | (<0.1) | 49 | (0.12) | < .0001 |
Maternal hypotension or obstetric shock b | 186 | (<0.1) | 65 | (0.2) | < .0001 |
Cerebral hemorrhage b | 60 | (<0.1) | 19 | (0.1) | < .0001 |
Gestational diabetes | 56,182 | (5.6) | 2163 | (5.3) | .011 |
Premature rupture of membranes b | 40,963 | (4.1) | 2940 | (7.2) | < .0001 |
Combined risk factors | |||||
Severe hypertension: eclampsia, preeclampsia b | 14,092 | (1.4) | 900 | (2.2) | < .0001 |
Other types of hypertension b | 81,223 | (8.1) | 4191 | (10.3) | < .0001 |
Mental disorder | 39,802 | (4.0) | 1933 | (4.8) | < .0001 |
Obesity | 37,928 | (3.8) | 2087 | (5.1) | < .0001 |
Chronic pulmonary condition b | 32,761 | (3.3) | 1777 | (4.4) | < .0001 |
Thyroid condition | 23,361 | (2.3) | 922 | (2.3) | .393 |
Abuse of any substance c | 12,332 | (1.2) | 618 | (1.5) | < .0001 |
Preexisting DM c | 9247 | (0.9) | 447 | (1.1) | .0002 |
CHF and other heart disease b | 7375 | (0.7) | 580 | (1.4) | < .0001 |
Renal condition b | 2210 | (0.2) | 192 | (0.5) | < .0001 |
Liver condition c | 1743 | (0.2) | 101 | (0.2) | .0002 |
a P value for χ 2 test of association with any infection present vs not present;
b Variables retained in model for P < .05;
Structural and organizational hospital features
Using data from the American Hospital Association (AHA) annual survey and Premier Inc, we noted each hospital’s geographic location, number of hospital beds, number of obstetric beds, number of deliveries in the 2-year period, whether the hospital was located in an urban or rural setting, teaching status, and whether a hospital reported full implementation of EHR. Four questions on the AHA Annual Survey (2008) were used to define a hospital’s level of implementation. The questions encompassed EHR use related to patient-level health information, results management, order entry management, and decision support. A hospital was categorized as having a fully implemented EHR if all 4 domains were reported as “fully implemented.”
Statistical analysis
We evaluated the association of patient demographics, maternal comorbidities, pregnancy-related conditions, and structural and organizational hospital features with the presence of “any infection” using χ 2 statistics. We used this composite measure of infection to assess hospital infection rates because it allowed for inclusion of rare diagnoses while reducing the risk that variation in coding practices across hospitals would result in biased rate estimates. Using a model-building strategy that retained factors with P < .05, or those that were theoretically important to obstetric infections, we employed hierarchical generalized linear modeling to model the log odds of experiencing infection related to childbirth adjusting for patient demographics, maternal comorbidities, and pregnancy-specific conditions that could increase risk of infection, while including a random hospital effect. Conditions, such as diabetes existing prior to pregnancy, which did not meet the significance criterion for inclusion in the model but were clinically important were forced into the model. Selected interaction terms were evaluated. From the final model, we calculated hospital-specific RAIR as the ratio of predicted (using hospital random effect) to expected (using average hospital effect) events multiplied by the overall unadjusted infection rate, a form of indirect standardization that is used in hospital outcomes measurement initiatives sponsored by the Centers for Medicare and Medicaid Services. Our primary model included all deliveries, and we stratified by vaginal or cesarean delivery in a secondary analysis.
We then evaluated the bivariate associations of structural and organizational hospital features with RAIR using analysis of variance and t tests. Lastly, we modeled RAIR across hospitals as a function of structural and organizational hospital features and estimated the proportion of variation in RAIR attributable to hospital features.
Results
Study sample
From the initial sample of 1,038,555 deliveries at 424 hospitals, 3913 were excluded due to presence of an ICD-9-CM code for ectopic or molar pregnancy or spontaneous or induced abortion, 29,888 due to transfer into or out of the hospital or unknown discharge status, 3140 for maternal age <15 or >44 years, and 425 because the delivery occurred at a hospital (n = 69) with <100 deliveries during the 2-year study period. Our final sample included 1,001,189 deliveries at 355 hospitals ( Figure 1 ).

The majority of women (75%) were between ages 20-34 years, 50% were married, 25% were black or Hispanic, and 42% had a public form of health insurance such as Medicaid ( Table 2 ). Cesarean deliveries accounted for 39% of the deliveries included in the study. The most commonly identified maternal comorbidities and pregnancy-specific conditions included cesarean delivery during a previous pregnancy (18.3%), advanced maternal age (≥35 years) (15.7%), hypertension (8.1%), and preterm delivery (7.6%) ( Table 2 ). Maternal mortality was 0.01% and median length of stay was 2 days (interquartile range [IQR], 2−3) for vaginal deliveries and 3 days (IQR, 3−4) for cesarean deliveries.
Of the deliveries included in the study, 40,605 (4.1%) were complicated by infection. Puerperal infections were the most common, affecting 2.1% of deliveries, followed by maternal pyrexia (1.6%) and surgical site infections (0.4%). Genitourinary tract infections (0.2%) and sepsis (0.1%) were relatively uncommon ( Table 1 ). Of the deliveries complicated by infection, maternal mortality was 0.06% and median length of stay was 3 days (IQR, 2−3) for vaginal deliveries and 4 days (IQR, 3−5) for cesarean deliveries.
Among the hospitals, 28% were teaching hospitals, 77% were in an urban setting, 43% were in the south region, and 28% had >30 obstetric beds. Relatively few hospitals (19%) reported complete implementation of EHR ( Table 3 ).
Characteristic | n | Mean RAIR | 95% CI | P value | |
---|---|---|---|---|---|
LL | UL | ||||
Region | .0003 | ||||
South | 154 | 4.0 | 3.7 | 4.4 | |
Midwest | 83 | 4.5 | 4.1 | 5.0 | |
West | 71 | 5.3 | 4.7 | 5.9 | |
Northeast | 47 | 5.3 | 4.4 | 6.2 | |
No. of deliveries in 2 y | .0002 | ||||
100-999 | 93 | 4.0 | 3.6 | 4.4 | |
1000-2149 | 84 | 4.2 | 3.7 | 4.7 | |
2150-4099 | 87 | 4.7 | 4.2 | 5.2 | |
≥4100 | 91 | 5.4 | 4.9 | 5.9 | |
No. of obstetric beds | < .0001 | ||||
<15 | 103 | 3.8 | 3.5 | 4.2 | |
15-29 | 129 | 4.5 | 4.1 | 4.9 | |
≥30 | 101 | 5.4 | 4.9 | 5.9 | |
Unknown | 22 | 4.6 | 3.5 | 5.7 | |
No. of hospital beds | .0004 | ||||
<200 | 116 | 4.1 | 3.7 | 4.4 | |
200-399 | 131 | 4.5 | 4.0 | 4.8 | |
≥400 | 108 | 5.3 | 4.8 | 5.8 | |
Teaching status | < .0001 | ||||
Nonteaching | 256 | 4.3 | 4.0 | 4.6 | |
Teaching | 99 | 5.4 | 4.9 | 5.9 | |
Setting | .01 | ||||
Urban | 275 | 4.8 | 4.5 | 5.0 | |
Rural | 80 | 4.0 | 3.5 | 4.5 | |
Electronic health record | .67 | ||||
Not complete implementation | 289 | 4.6 | 4.3 | 4.8 | |
Complete implementation | 66 | 4.7 | 4.1 | 5.3 |
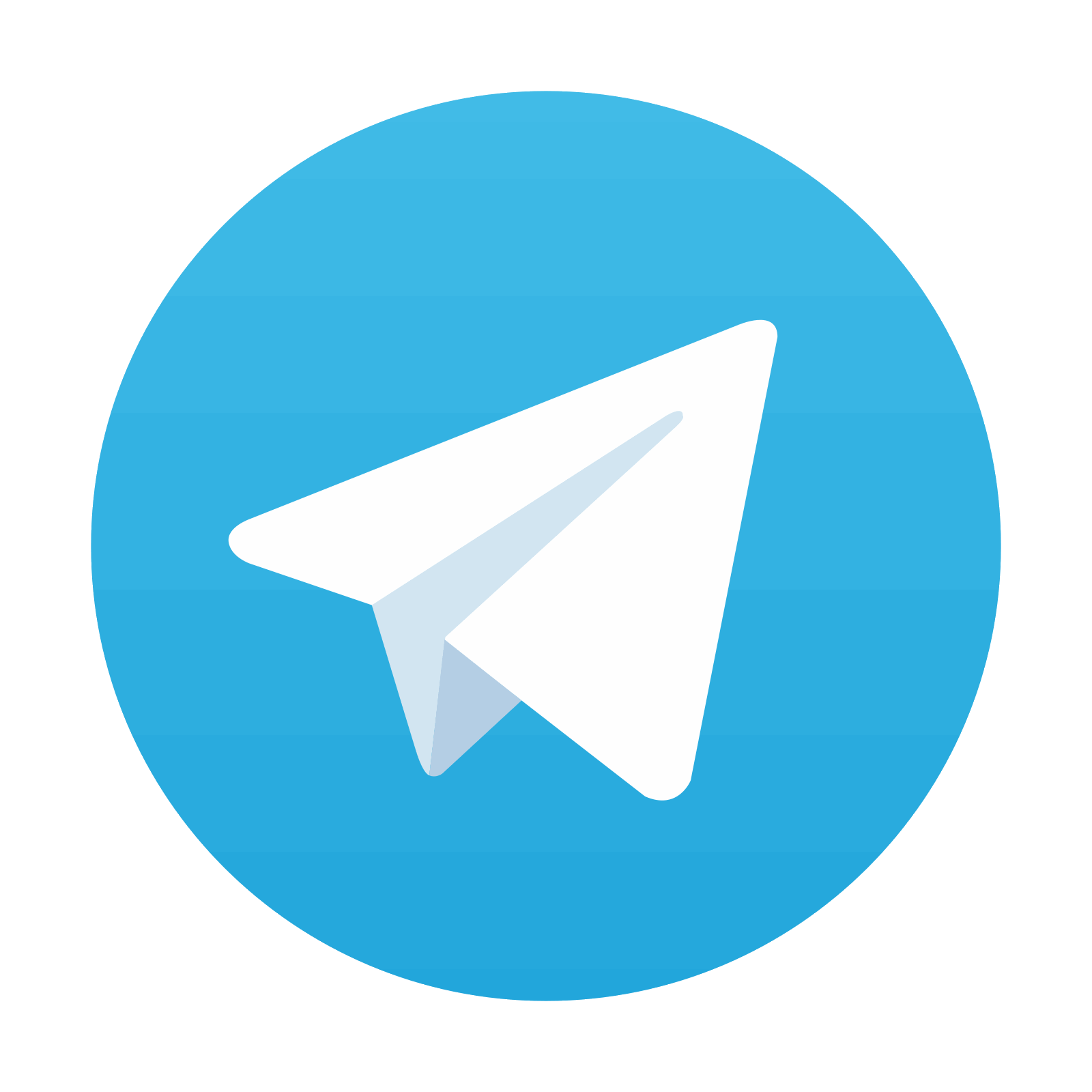
Stay updated, free articles. Join our Telegram channel

Full access? Get Clinical Tree
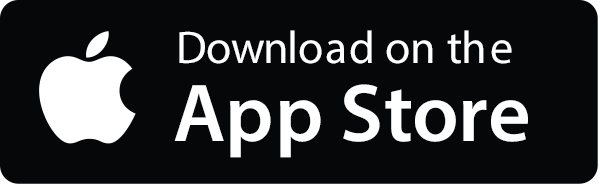

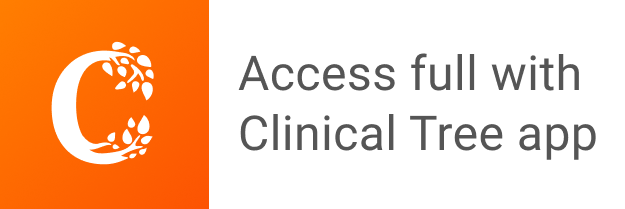