Objective
The objective of the study was to identify metabolomic markers in maternal first-trimester serum for the detection of fetal congenital heart defects (CHDs).
Study Design
Mass spectrometry (direct injection/liquid chromatography and tandem mass spectrometry) and nuclear magnetic resonance spectrometry–based metabolomic analyses were performed between 11 weeks’ and 13 weeks 6 days’ gestation on maternal serum. A total of 27 CHD cases and 59 controls were compared. There were no known or suspected chromosomal or syndromic abnormalities indicated.
Results
A total of 174 metabolites were identified and quantified using the 2 analytical methods. There were 14 overlapping metabolites between platforms. We identified 123 metabolites that demonstrated significant differences on a univariate analysis in maternal first-trimester serum in CHD vs normal cases. There was a significant disturbance in acylcarnitine, sphingomyelin, and other metabolite levels in CHD pregnancies. Predictive algorithms were developed for CHD detection. High sensitivity (0.929; 95% confidence interval [CI], 0.92–1.00) and specificity (0.932; 95% CI, 0.78–1.00) for CHD detection were achieved (area under the curve, 0.992; 95% CI, 0.973–1.0).
Conclusion
In the first such report, we demonstrated the feasibility of the use of metabolomic developing biomarkers for the first-trimester prediction of CHD. Abnormal lipid metabolism appeared to be a significant feature of CHD pregnancies.
Congenital heart defect (CHD) is the most important category of congenital anomalies based both on its frequency, 0.6-0.8% of all the births, and health care costs. In contrast to the routine population pregnancy screening for the detection of less common fetal anomalies such as aneuploidies and neural tube defects, there is no comparable screening policy for CHD.
Ultrasound remains the most widely used prenatal tool for the detection of fetal CHD. Although specialist centers that care for high-risk patients report high sensitivities for CHD detection, the overall performance of prenatal ultrasound in the general population remains substantially below that required for an effective screening test. A recent study in the United States found that slightly less than 40% of CHD cases were detected prenatally in a state-wide obstetric population that had an ultrasound examination at the appropriate gestational age.
The overall accuracy of prenatal ultrasound is significantly constrained by its dependence on operator expertise, equipment quality, and uncontrollable variables such as fetal position and maternal obesity. The difficulties associated with the of diagnosis of CHD, moreover, is not limited to the prenatal period because a relatively high percentage of critical CHD fails to be diagnosed in newborns prior to discharge home.
The prenatal diagnosis of CHD has distinct advantages including the opportunity for early counseling of families, facilitating reproductive choices, and permitting the transfer of care to expert physicians in tertiary level facilities. Finally, in some categories of CHD prenatal diagnosis reportedly may improve overall outcome compared with those in which the diagnosis is made after birth. An area of concern with respect to the prenatal diagnosis of any congenital anomalies related to potential medical selection against affected fetuses. Data from France have, however, shown that pregnancy termination rates have not increased in proportion to improving prenatal diagnosis of CHD. Indeed, termination rates have leveled off and pregnancy termination was exceptional among the more common categories of CHD, whereas at the same time, there has been a reduction in early neonatal deaths.
Metabolomics is a branch of the omics sciences in which high through-put techniques are used for the identification and quantification of the small molecules that constitute the metabolome. Metabolites are a very diverse group of molecules including but not limited to amino acids, nucleic acids, lipids, peptides, sugars, and organic acids. They represent the substrates and byproducts of the various enzymatic reactions within the cells but also respond to and reflect various physiological (eg, age and gender); moreover, pathological and environmental influences including diet, toxins, pharmacological agents and stress, which are important causes and modifiers of disease, significantly influence the metabolome. Based on the latter, metabolomics reportedly may give a more complete description of cellular phenotype than the genome, transcriptome, or proteome.
There has been a dramatic rise in the number of scientific publications related to metabolomics. Increasingly, metabolomics is being used to develop biomarkers for the detection, screening, and monitoring of complex diseases. There is limited prior evidence that CHD may either be caused by or associated with metabolic disturbance in humans. Abnormalities of folate and single carbon metabolism has been linked to the development of CHD. To our knowledge, comprehensive metabolomic analysis for the prediction of fetal CHD has not been previously reported.
The objectives of the current study are 2-fold. First, we were interested in determining whether there are significant differences in the first-trimester maternal metabolomic profile in pregnancies with a chromosomally normal fetus compared with those affected with a CHD. Second, we wanted to evaluate metabolite biomarker algorithms that might be useful for the first-trimester prediction of fetal CHD.
Materials and Methods
This study is part of an ongoing prospective study for the first-trimester detection and prediction of fetal and maternal disorders. The details on specimen collection have been extensively described elsewhere. The patients were prospectively recruited from an average risk population in Britain between 2006 and 2009. Institutional review board approval was obtained through the Institutional Review Board of King’s College Hospital, London, England. Each recruited patient signed a written consent.
Crown rump length (CRL) was used to estimate gestational age. Routine first-trimester screening for aneuploidy is the current standard of care. Maternal demographic and clinical data were obtained along with serum for pregnancy-associated plasma protein-A and free B-human chorionic gonadotropin. Nuchal translucency (NT) thickness was measured for aneuploidy risk estimation. Karyotype and/or newborn examinations were performed to assess chromosomal status. CHD status was determined by prenatal imaging and/or postnatal imaging and based on physical examination in the normal cases.
Samples are immediately transferred to the laboratory within 5 minutes of collection. They are processed after a standing time of 10-15 minutes at room temperature to allow for clotting. The tubes are centrifuged at 3000 rpm for 10 minutes to separate the serum. Then the serum is aliquoted in 8 0.5 mL prelabeled screw tubes (serum) using Gilson micropipette (mark 050). The samples are then subsequently stored in a blue box, previously numbered. The blue box is temporarily stored in a –20°C freezer and then transferred to racks and stored in a –80°C freezer within 24 hours.
We searched our database to identify singleton pregnancies in which the fetus was diagnosed antenatally to have an isolated major cardiac defect with available sample stored at 11–13 weeks’ gestation. Cardiac defects were considered to be major if they were lethal or required surgery or interventional cardiac catheterization within the first year of life. We excluded all cases with aneuploidy or noncardiac defects diagnosed prenatally or in the neonatal period. All the CHD diagnoses were made by a specialist in fetal echocardiography. Pregnancies that resulted in live births had newborn confirmation. For cases that underwent termination of pregnancy but for which an autopsy was not performed, the diagnosis was made based on the prenatal examination performed by expert fetal echocardiologists. The study population included 30 cases with major cardiac defects, and each case was matched with 2 controls with no pregnancy complications that were scanned on the same day and that resulted in the live birth of phenotypically normal neonates. A total of 86 sample specimens were processed at the testing laboratory.
Nuclear magnetic resonance metabolomic analysis
In prior publications, we have extensively described the use of the nuclear magnetic resonance (NMR) platform for metabolomic analysis of the serum. Serum samples were filtered through 3 kDa cutoff centrifuge filter units (Amicon Micoron YM-3; Sigma-Aldrich, St. Louis, MO) to remove blood proteins. Three hundred fifty microliters of samples was added to the centrifuge filter device and spun (10,000 rpm for 20 minutes) to remove macromolecules such as protein and lipoproteins. If the total volume of sample was less than 300 μL, a 50 mmol NaH 2 PO 4 buffer (pH 7) was added to reach a total sample volume of 300 μL. Metabolite concentrations were adjusted for the dilution because of the buffer. Thereafter, 35 μL of D 2 O and 15 μL of buffer solution containing (233 Na 2 PO 4 at pH 7, 11.667 mmol disodium-2, 2-dimethyl-2-silceptentane-5-sulphonate, and 0.1% NaN 3 in H 2 O) were added to the sample.
A total of 350 μL of sample was transferred to a microcell NMR tube (Shigemi, Inc, Allison Park, PA). 1 H-NMR spectra were collected on a 500 MHz Inova spectrometer (Varian Inc, Palo Alto, CA) with a 5 mm hydrogen, carbon, and nitrogen Z-gradient pulse field gradient probe. The singlet produced by the disodium-2, 2-dimethyl-2-silceptentane-5-sulphonate methyl groups was used as an internal standard for both chemical shift referencing and for metabolite quantification. The 1 H-NMR spectra were analyzed with a Chenomx NMR Suite Professional Software package (version 7.6; Chenomx Inc, Edmonton, ALB, Canada), which permitted both quantitative and qualitative analysis by manually fitting the NMR spectra to an internal metabolite database. Each spectrum was evaluated independently by at least 2 NMR spectroscopists to minimize errors of quantification and identification.
Combined direct injection and liquid chromatography and tandem mass spectrometry compound identification and quantification
We have applied a targeted quantitative metabolomics approach to analyze the serum samples using a combination of direct injection mass spectrometry (Absolute IDQ kit) with a reverse-phase liquid chromatography and tandem mass spectrometry (LC-MS/MS) kit. The kit is a commercially available assay from Biocrates Life Sciences AG (Innsbruck, Austria). This kit, in combination with an ABI 4000 Q-Trap (Applied Biosystems/MDS Sciex, Framingham, MA) mass spectrometer, can be used for the targeted identification and quantification of up to 180 different endogenous metabolites including amino acids, acylcarnitines, biogenic amines, glycerophospholipids, sphingolipids, and sugars. The method used combines the derivatization and extraction of analytes, and the selective mass-spectrometric detection using multiple reaction monitoring pairs. Isotope-labeled internal standards and other internal standards are integrated into a kit plate filter for metabolite quantification.
The Absolute IDQ kit contains a 96 deep-well plate with a filter plate attached with sealing tape and reagents and solvents used to prepare the plate assay. First, 14 wells in the kit were used for 1 blank, 3 zero samples, 7 standards, and 3 quality control samples provided with each kit. All the serum samples were analyzed with the Absolute IDQ kit using the protocol described in the Absolute IDQ user manual. Briefly, serum samples were thawed on ice and were vortexed and centrifuged at 13,000 × g . Ten microliters of each serum sample were loaded onto the center of the filter on the upper 96 well kit plate and dried in a stream of nitrogen. Subsequently, 20 μL of a 5% solution of phenylisothiocyanate was added for derivatization. After incubation, the filter spots were dried again using an evaporator.
Extraction of the metabolites was then achieved by adding 300 μL methanol containing 5 mM ammonium acetate. The extracts were obtained by centrifugation into the lower 96 deep-well plate, followed by a dilution step with kit MS running solvent. Mass spectrometric analysis was performed on an API4000 Qtrap tandem mass spectrometry instrument (Applied Biosystems/MDS Analytical Technologies, Foster City, CA) equipped with a solvent delivery system. The samples were delivered to the mass spectrometer by a liquid chromatography method followed by a DI method. The Biocrates MetIQ software was used to control the entire assay workflow, from sample registration to automated calculation of metabolite concentrations to the export of data into other data analysis programs.
A targeted profiling scheme was used to quantitatively screen for known small molecule metabolites using multiple reaction monitoring, neutral loss, and precursor ion scans. The metabolomic analyses were performed at the Metabolomics Innovation Centre, University of Alberta, Edmonton, Canada.
Statistical analysis
Analysis of the metabolomics data was performed with the MetaboAnalyst web-based statistical package. Univariate analysis of continuous data was conducted using Wilcoxon-Mann-Whitney test, and categorical data were analyzed using Pearson χ 2 and Fisher exact tests. Multivariate analyses were conducted using binary logistic regression with selected features using a Lasso algorithm. A significance level of P < .05 was used to define statistical significance.
Three different sets of analyses were performed. Metabolites were analyzed by themselves and also with the addition of demographic characteristics such as ethnicity, body mass index (BMI), parity, and an ultrasound measurement of fetal length (ie, CRL). Finally metabolites with NT thickness were evaluated. It should be pointed out that the CRL is the most precise measure of gestational age and therefore used to assess whether first-trimester gestational age affected the maternal serum level of the metabolites.
Data normalization of metabolite concentration is critical to creating a normal or Gaussian distribution. Normalization allows conventional statistical tests to be performed, and it simplifies data interpretation. In this study, we used log-transformed metabolite values.
Principal component analysis (PCA) is a multivariate analysis technique and was used to find the most useful principal components for distinguishing groups of interest in the dataset. The first principal component has the largest possible variance to discriminate each group, and the second principal component that is calculated orthogonal to the first principal component has the second highest variance possible.
Partial least squares discriminant analysis (PLS-DA) rotates around the different principal components to find the optimal combination for discriminating the case from the control group. Permutation analysis uses random resampling of cases and controls to determine the probability that the observed and control groups is a result of chance. A total of 2000 resamplings were performed and calculated. A P value that represents the probability of a chance finding is generated. A variable importance in projection (VIP) plot, which is a visual representation of the significance or importance of the particular metabolite in discriminating the groups of interest, is provided.
Metabolite concentrations in CHD vs controls were compared. Logistic regression analyses were performed with outcomes (CHD or normal) as the dependent variable and metabolites as the independent or determinant variable to develop a predictive algorithm for CHD detection. Metabolites with a significant correlation with CHD status on univariate analysis were initially entered into the model development. Other variables including NT, fetal CRL, and maternal demographics and medical status were combined with metabolite concentrations and run in selected logistic regression analyses. Finally, logistic regression analyses including NT and the preceding metabolomic and maternal markers were performed.
Paired sensitivity and false-positive rates (1 – specificity) at different risk thresholds were calculated. A receiver-operator characteristic (ROC) curve is plotted with sensitivity values on the Y-axis and the corresponding false-positive ratio (1 – specificity) on the X-axis. The area under the ROC curve (AUC) indicates the accuracy of a test for correctly distinguishing one group such as CHD pregnancies from normal (control), where AUC = 1 indicates a perfectly discriminating test. The 95% confidence interval (CI) and P values for the AUC curves were calculated. Permutation testing was also performed to determine the probability that the AUC obtained was due to chance.
Results
Metabolomic analyses using 2 analytical techniques, NMR and direct injection (DI)/LC-MS/MS, were performed for 27 cases of CHD and 59 normal matched controls. Neither case nor control fetuses had any known or suspected chromosomal or syndromic abnormalities. Table 1 gives the breakdown of the different types of CHD. Table 2 compares maternal pregnancy and other demographic characteristics between study and control groups. No significant difference was observed. A total of 150 metabolites were identified and quantified using the DI/LC-MS/MS technique. By using NMR spectroscopy, a total of 38 metabolites were quantified. There were 174 distinct metabolites measured by the 2 platforms.
Heart defect | n |
---|---|
AVSD/DORV | 1 |
AVSD/DORV/PA | 1 |
DORV/PS | 2 |
DORV/TOF | 2 |
DORV/PA | 1 |
TGA | 3 |
TGA-corrected VSD | 1 |
TGA/PS | 1 |
TOF | 9 |
TOF/MS | 1 |
TOF/PA | 5 |
Parameter | CHD | Control | P value |
---|---|---|---|
n | 27 | 59 | |
Mean maternal age (y), mean (SD) a | 29.2 (6.5) | 30.0 (5.2) | NS |
Ethnicity, n (%) b | |||
Caucasian | 23 (82.1) | 47 (79.7) | NS |
African descent | 3 (10.7) | 10 (16.9) | |
Asian/other | 2 (7.1) | 2 (3.4) | |
Nulliparous, n (%) c | NS | ||
Multiparous | 12 (42.9) | 23 (39.0) | |
Nulliparous | 16 (57.1) | 36 (61.0) | |
BMI, mean (SD) a | 24.1 (4.2) | 24.4 (3.5) | NS |
GA-CRL (wks), mean (SD) a | 12.7 (0.7) | 12.7 (0.6) | NS |
By using a univariate analysis, a total of 118 metabolites from the DI/LC-MS/MS assay were found to have significant concentration differences in maternal serum in CHD vs normal controls on paired comparisons. The mean (SD) concentrations, Wilcoxon-Mann-Whitney test, and P values for each of those significant metabolites along with fold change and direction of change in CHD cases relative to controls are provided in Table 3 .
Metabolite (biochemical name) | Mean (SD) | Fold change | CHD/control | P value a | |
---|---|---|---|---|---|
CHD | Control | ||||
Number of cases | 27 | 59 | — | — | — |
C0 (carnitine) | 16.798 (14.323) | 24.408 (5.444) | –1.45 | Down | .0168 |
C2 (acetylcarnitine) | 2.5022 (2.2864) | 4.4217 (1.7936) | –1.77 | Down | .0008 |
C3 (propionylcarnitine) | 0.2075 (0.1802) | 0.2999 (0.081) | –1.45 | Down | .0177 |
C3:1 (propenoylcarnitine) | 0.0253 (0.00163) | 0.0317 (0.0061) | –1.26 | Down | .0363 |
C3-OH (hydroxypropionylcarnitine) | 0.0918 (0.0644) | 0.1834 (0.0256) | –2 | Down | .0000 |
C4 (butyrylcarnitine) | 0.1378 (0.119) | 0.2169 (0.0736) | –1.57 | Down | .0025 |
C5 (valerylcarnitine) | 0.0845 (0.0722) | 0.1179 (0.0241) | –1.39 | Down | .0070 |
C5-M-DC (methylglutarylcarnitine) | 0.049 (0.0327) | 0.1123 (0.0447) | –2.29 | Down | .0000 |
C5-OH(C3-DC-M) (hydroxyvalerylcarnitine (methylmalonylcarnitine)) | 0.0602 (0.0527) | 0.1732 (0.0719) | –2.88 | Down | .0000 |
C5:1-DC (glutaconylcarnitine) | 0.0725 (0.1405) | 0.0262 (0.005) | 2.76 | Up | .0130 |
C6:1 (hexenoylcarnitine) | 0.0241 (0.0125) | 0.0293 (0.0049) | –1.22 | Down | .0050 |
C8 (octanoylcarnitine) | 0.0943 (0.04369) | 0.1304 (0.0496) | –1.38 | Down | .0018 |
C9 (nonaylcarnitine) | 0.0353 (0.0207) | 0.059 (0.017) | –1.67 | Down | .0000 |
C10 (decanoylcarnitine) | 0.1267 (0.0743) | 0.2449 (0.1076) | –1.93 | Down | .0000 |
C10:1 (decenoylcarnitine) | 0.204 (0.0677) | 0.2306 (0.0504) | –1.13 | Down | .0045 |
C10:2 (decadienylcarnitine) | 0.0317 (0.0179) | 0.0498 (0.0156) | –1.57 | Down | .0001 |
C12 (dodecanoylcarnitine) | 0.045 (0.0197) | 0.0651 (0.0228) | –1.45 | Down | .0003 |
C14 (tetradecanoylcarnitine) | 0.0337 (0.0126) | 0.0403 (0.0065) | –1.19 | Down | .0003 |
C14:1 (tetradecenoylcarnitine) | 0.1014 (0.0757) | 0.1978 (0.0404) | –1.95 | Down | .0000 |
C14:2 (tetradecadienylcarnitine) | 0.0135 (0.0071) | 0.0217 (0.0087) | –1.6 | Down | .0001 |
C14:2-OH (hydroxytetradecadienylcarnitine) | 0.0105 (0.0063) | 0.0125 (0.0042) | –1.2 | Down | .0039 |
C16 (hexadecanoylcarnitine) | 0.046 (0.0375) | 0.0767 (0.0217) | –1.67 | Down | .0000 |
C16:2 (hexadecadienylcarnitine) | 0.011 (0.0057) | 0.0133 (0.0024) | –1.21 | Down | .0068 |
C18 (octadecanoylcarnitine) | 0.0258 (0.018) | 0.0371 (0.0085) | –1.44 | Down | .0005 |
C18:1 (octadecenoylcarnitine) | 0.0477 (0.043) | 0.0835 (0.037) | –1.75 | Down | .0000 |
C18:2 (octadecadienylcarnitine) | 0.0208 (0.0151) | 0.0321 (0.0112) | –1.54 | Down | .0011 |
LysoPC a C16:0 (lysophosphatidylcholine acyl C16:0) | 72.189 (66.133) | 142.065 (39.638) | –1.97 | Down | .0000 |
LysoPC a C16:1 (lysophosphatidylcholine acyl C16:1) | 1.8565 (1.8622) | 2.6836 (1.0076) | –1.45 | Down | .0155 |
LysoPC a C17:0 (lysophosphatidylcholine acyl C17:0) | 1.8811 (1.9996) | 2.6388 (0.8126) | –1.4 | Down | .0044 |
LysoPC a C18:0 (lysophosphatidylcholine acyl C18:0) | 19.323 (17.977) | 36.487 (11.673) | –1.89 | Down | .0001 |
LysoPC a C18:1 (lysophosphatidylcholine acyl C18:1) | 13.380 (12.740) | 27.946 (9.468) | –2.09 | Down | .0000 |
LysoPC a C18:2 (lysophosphatidylcholine acyl C18:2) | 17.582 (17.132) | 36.345 (14.315) | –2.07 | Down | .0000 |
LysoPC a C20:3 (lysophosphatidylcholine acyl C20:3) | 1.6375 (1.5461) | 2.7411 (1.1319) | –1.67 | Down | .0011 |
LysoPC a C20:4 (lysophosphatidylcholine acyl C20:4) | 4.2194 (3.7183) | 7.9856 (2.2556) | –1.89 | Down | .0000 |
PC aa C28:1 (phosphatidylcholine diacyl C28:1) | 2.3011 (1.9755) | 3.4444 (1.1203) | –1.5 | Down | .0168 |
PC aa C30:0 (phosphatidylcholine diacyl C30:0) | 3.5667 (3.29) | 6.0819 (2.7436) | –1.71 | Down | .0015 |
PC aa C30:2 (phosphatidylcholine diacyl C30:2) | 0.2731 (0.3429) | 0.5917 (0.1918) | –2.17 | Down | .0000 |
PC aa C32:0 (phosphatidylcholine diacyl C32:0) | 11.830 (10.249) | 21.468 (6.561) | –1.81 | Down | .0003 |
PC aa C32:1 (phosphatidylcholine diacyl C32:1) | 12.399 (12.890) | 23.352 (12.85) | –1.88 | Down | .0003 |
PC aa C32:2 (phosphatidylcholine diacyl C32:2) | 3.395 (3.2336) | 6.5874 (2.6463) | –1.94 | Down | .0001 |
PC aa C32:3 (phosphatidylcholine diacyl C32:3) | 0.5258 (0.4654) | 0.8819 (0.2227) | –1.68 | Down | .0014 |
PC aa C34:1 (phosphatidylcholine diacyl C34:1) | 168.650 (151.577) | 316.441 (96.259) | –1.88 | Down | .0001 |
PC aa C34:2 (phosphatidylcholine diacyl C34:2) | 284.991 (253.246) | 512.414 (109.171) | –1.8 | Down | .0002 |
PC aa C34:3 (phosphatidylcholine diacyl C34:3) | 14.688 (13.192) | 26.705 (10.794) | –1.82 | Down | .0006 |
PC aa C34:4 (phosphatidylcholine diacyl C34:4) | 1.580 (1.4083) | 2.9268 (1.229) | –1.85 | Down | .0003 |
PC aa C36:1 (phosphatidylcholine diacyl C36:1) | 34.645 (31.864) | 65.276 (21.905) | –1.88 | Down | .0000 |
PC aa C36:2 (phosphatidylcholine diacyl C36:2) | 155.673 (140.706) | 279.018 (74.520) | –1.79 | Down | .0001 |
PC aa C36:3 (phosphatidylcholine diacyl C36:3) | 106.650 (95.964) | 188.801 (66.714) | –1.77 | Down | .0009 |
PC aa C36:4 (phosphatidylcholine diacyl C36:4) | 139.129 (121.517) | 260.540 (70.103) | –1.87 | Down | .0000 |
PC aa C36:5 (phosphatidylcholine diacyl C36:5) | 20.626 (20.914) | 42.042 (23.098) | –2.04 | Down | .0001 |
PC aa C36:6 (phosphatidylcholine diacyl C36:6) | 1.2059 (1.1361) | 2.1289 (0.9321) | –1.77 | Down | .0015 |
PC aa C38:0 (phosphatidylcholine diacyl C38:0) | 3.0315 (2.754) | 5.089 (1.5576) | –1.68 | Down | .0013 |
PC aa C38:1 (phosphatidylcholine diacyl C38:1) | 1.0299 (1.2723) | 1.4582 (0.58) | –1.42 | Down | .0026 |
PC aa C38:3 (phosphatidylcholine diacyl C38:3) | 39.351 (35.404) | 67.848 (22.399) | –1.72 | Down | .0015 |
PC aa C38:4 (phosphatidylcholine diacyl C38:4) | 76.611 (64.780) | 141.130 (37.687) | –1.84 | Down | .0001 |
PC aa C38:5 (phosphatidylcholine diacyl C38:5) | 40.448 (34.594) | 76.975 (21.930) | –1.9 | Down | .0001 |
PC aa C38:6 (phosphatidylcholine diacyl C38:6) | 90.567 (84.144) | 178.280 (54.427) | –1.97 | Down | .0000 |
PC aa C40:1 (phosphatidylcholine diacyl C40:1) | 0.5856 (0.3525) | 0.6059 (0.1541) | –1.03 | Down | .0255 |
PC aa C40:4 (phosphatidylcholine diacyl C40:4) | 2.9053 (2.5455) | 4.9576 (1.7367) | –1.71 | Down | .0017 |
PC aa C40:5 (phosphatidylcholine diacyl C40:5) | 7.8919 (6.8919) | 14.0773 (4.5583) | –1.78 | Down | .0005 |
PC aa C40:6 (phosphatidylcholine diacyl C40:6) | 28.029 (25.525) | 54.476 (16.859) | –1.94 | Down | .0000 |
PC aa C42:0 (phosphatidylcholine diacyl C42:0) | 0.6763 (0.5907) | 1.2374 (0.3778) | –1.83 | Down | .0000 |
PC aa C42:1 (phosphatidylcholine diacyl C42:1) | 0.3311 (0.3002) | 0.5457 (0.1542) | –1.65 | Down | .0009 |
PC aa C42:2 (phosphatidylcholine diacyl C42:2) | 0.2295 (0.2297) | 0.3178 (0.1022) | –1.38 | Down | .0130 |
PC aa C42:4 (phosphatidylcholine diacyl C42:4) | 0.2195 (0.2094) | 0.2922 (0.08) | –1.33 | Down | .0200 |
PC aa C42:5 (phosphatidylcholine diacyl C42:5) | 0.5168 (0.4713) | 0.8146 (0.2572) | –1.58 | Down | .0052 |
PC aa C42:6 (phosphatidylcholine diacyl C42:6) | 0.9336 (0.4475) | 1.1482 (0.298) | –1.23 | Down | .0123 |
PC ae C32:1 (phosphatidylcholine acly-alkyl C32:1) | 2.2309 (1.9781) | 3.8034 (1.1018) | –1.7 | Down | .0009 |
PC ae C32:2 (phosphatidylcholine acly-alkyl C32:2) | 0.6953 (0.632) | 1.0491 (0.2574) | –1.51 | Down | .0085 |
PC ae C34:0 (phosphatidylcholine acly-alkyl C34:0) | 1.237 (1.1026) | 1.9551 (0.6817) | –1.58 | Down | .0045 |
PC ae C34:1 (phosphatidylcholine acly-alkyl C34:1) | 7.9483 (7.1334) | 14.6214 (4.8614) | –1.84 | Down | .0002 |
PC ae C34:2 (phosphatidylcholine acly-alkyl C34:2) | 9.0328 (8.2812) | 15.6214 (5.1054) | –1.73 | Down | .0004 |
PC ae C34:3 (phosphatidylcholine acly-alkyl C34:3) | 6.5576 (6.1098) | 11.1413 (3.0949) | –1.7 | Down | .0003 |
PC ae C36:0 (phosphatidylcholine acly-alkyl C36:0) | 1.0045 (1.1707) | 1.0445 (0.3054) | –1.04 | Down | .0195 |
PC ae C36:1 (phosphatidylcholine acly-alkyl C36:1) | 7.0628 (6.4818) | 11.3303 (3.5905) | –1.6 | Down | .0048 |
PC ae C36:2 (phosphatidylcholine acly-alkyl C36:2) | 12.106 (10.981) | 20.838 (6.545) | –1.72 | Down | .0004 |
PC ae C36:3 (phosphatidylcholine acly-alkyl C36:3) | 6.3834 (5.9313) | 11.0311 (3.8796) | –1.73 | Down | .0005 |
PC ae C36:4 (phosphatidylcholine acly-alkyl C36:4) | 12.696 (10.967) | 21.900 (6.566) | –1.72 | Down | .0008 |
PC ae C36:5 (phosphatidylcholine acly-alkyl C36:5) | 8.0333 (6.9636) | 14.273 (3.6587) | –1.78 | Down | .0001 |
PC ae C38:0 (phosphatidylcholine acly-alkyl C38:0) | 2.4102 (2.0677) | 3.9207 (1.302) | –1.63 | Down | .0034 |
PC ae C38:1 (phosphatidylcholine acly-alkyl C38:1) | 0.8754 (1.2408) | 0.8763 (0.4078) | –1.00 | Down | .0114 |
PC ae C38:2 (phosphatidylcholine acly-alkyl C38:2) | 1.9462 (1.9687) | 3.0116 (0.9756) | –1.55 | Down | .0013 |
PC ae C38:3 (phosphatidylcholine acly-alkyl C38:3) | 3.9018 (3.5774) | 6.0499 (2.145) | –1.55 | Down | .0100 |
PC ae C38:4 (phosphatidylcholine acly-alkyl C38:4) | 9.647 (8.2858) | 17.6932 (5.2507) | –1.83 | Down | .0001 |
PC ae C38:5 (phosphatidylcholine acly-alkyl C38:5) | 11.949 (10.363) | 22.833 (6.419) | –1.91 | Down | .0000 |
PC ae C38:6 (phosphatidylcholine acly-alkyl C38:6) | 6.044 (5.4155) | 11.0441 (3.144) | –1.83 | Down | .0002 |
PC ae C40:1 (phosphatidylcholine acly-alkyl C40:1) | 1.0876 (1.0198) | 1.7633 (0.55) | –1.62 | Down | .0011 |
PC ae C40:2 (phosphatidylcholine acly-alkyl C40:2) | 1.6831 (1.5317) | 2.6479 (0.753) | –1.57 | Down | .0062 |
PC ae C40:3 (phosphatidylcholine acly-alkyl C40:3) | 1.2038 (1.1703) | 1.6982 (0.5214) | –1.41 | Down | .0155 |
PC ae C40:4 (phosphatidylcholine acly-alkyl C40:4) | 1.886 (1.6743) | 3.2221 (1.0074) | –1.71 | Down | .0017 |
PC ae C40:5 (phosphatidylcholine acly-alkyl C40:5) | 3.1709 (2.7955) | 5.6119 (1.6168) | –1.77 | Down | .0004 |
PC ae C40:6 (phosphatidylcholine acly-alkyl C40:6) | 4.8457 (4.4043) | 9.0374 (2.6279) | –1.87 | Down | .0000 |
PC ae C42:0 (phosphatidylcholine acly-alkyl C42:0) | 0.8517 (0.4519) | 1.0654 (0.2901) | –1.25 | Down | .0048 |
PC ae C42:1 (phosphatidylcholine acly-alkyl C42:1) | 0.3911 (0.3516) | 0.5233 (0.162) | –1.34 | Down | .0237 |
PC ae C42:2 (phosphatidylcholine acly-alkyl C42:2) | 0.5345 (0.5106) | 0.8615 (0.2624) | –1.61 | Down | .0015 |
PC ae C42:3 (phosphatidylcholine acly-alkyl C42:3) | 0.8538 (0.817) | 1.3914 (0.4665) | –1.63 | Down | .0019 |
PC ae C42:4 (phosphatidylcholine acly-alkyl C42:4) | 1.0104 (0.9584) | 1.6802 (0.6029) | –1.66 | Down | .0027 |
PC ae C42:5 (phosphatidylcholine acly-alkyl C42:5) | 2.2948 (1.8271) | 3.9456 (1.2567) | –1.72 | Down | .0002 |
PC ae C44:3 (phosphatidylcholine acly-alkyl C44:3) | 0.1417 (0.1267) | 0.203 (0.0655) | –1.43 | Down | .0021 |
PC ae C44:4 (phosphatidylcholine acly-alkyl C44:4) | 0.4641 (0.421) | 0.7322 (0.2906) | –1.58 | Down | .0020 |
PC ae C44:5 (phosphatidylcholine acly-alkyl C44:5) | 2.0303 (1.7939) | 3.7191 (1.3549) | –1.83 | Down | .0001 |
PC ae C44:6 (phosphatidylcholine acly-alkyl C44:6) | 1.3903 (1.2122) | 2.5122 (0.742) | –1.81 | Down | .0001 |
SM (OH) C14:1 (hydroxysphingomyeline C14:1) | 4.7973 (4.3326) | 7.9934 (2.0106) | –1.67 | Down | .0009 |
SM (OH) C16:1 (hydroxysphingomyeline C16:1) | 2.6565 (2.2818) | 4.4412 (0.9153) | –1.67 | Down | .0012 |
SM (OH) C22:1 (hydroxysphingomyeline C22:1) | 9.7724 (8.4566) | 16.7107 (4.144) | –1.71 | Down | .0021 |
SM (OH) C22:2 (hydroxysphingomyeline C22:2) | 9.1052 (7.7116) | 15.5155 (3.1796) | –1.7 | Down | .0009 |
SM (OH) C24:1 (hydroxysphingomyeline C24:1) | 1.0876 (0.9485) | 1.7359 (0.44) | –1.6 | Down | .0092 |
SM C16:0 (sphingomyeline C16:0) | 79.934 (68.457) | 140.997 (28.289) | –1.76 | Down | .0002 |
SM C16:1 (sphingomyeline C16:1) | 11.455 (10.081) | 20.316 (4.306) | –1.77 | Down | .0002 |
SM C18:0 (sphingomyeline C18:0) | 18.125 (15.986) | 31.102 (6.468) | –1.72 | Down | .0003 |
SM C18:1 (sphingomyeline C18:1) | 8.2855 (7.2093) | 14.7 (3.1262) | –1.77 | Down | .0002 |
SM C20:2 (sphingomyeline C20:2) | 0.8174 (0.8328) | 1.7089 (0.4626) | –2.09 | Down | .0000 |
SM C22:3 (sphingomyeline C22:3) | 4.5683 (5.4572) | 13.3436 (3.8124) | –2.92 | Down | .0000 |
SM C24:0 (sphingomyeline C24:0) | 16.752 (14.327) | 29.457 (7.463) | –1.76 | Down | .0008 |
SM C24:1 (sphingomyeline C24:1) | 43.053 (37.370) | 79.007 (16.719) | –1.84 | Down | .0000 |
SM C26:0 (sphingomyeline C26:0) | 0.1904 (0.2071) | 0.2502 (0.0652) | –1.31 | Down | .0074 |
SM C26:1 (sphingomyeline C26:1) | 0.3279 (0.2907) | 0.5388 (0.145) | –1.64 | Down | .0057 |
In Table 4 , a similar comparison of metabolite concentrations was performed for only NMR-based metabolomics. Significant differences were noted in 5 metabolites using the NMR platform.
Metabolite | Mean (SD) | Fold change | CHD/control | P value a | |
---|---|---|---|---|---|
CHD | Control | ||||
Number of cases | 27 | 59 | — | — | — |
2-Hydroxybutyrate | 17.13 (7.48) | 16.69 (6.25) | 1.03 | Up | .8963 |
3-Hydroxybutyrate | 33.63 (42.57) | 30.15 (37.69) | 1.12 | Up | .4208 |
Acetamide | 6.56 (3.47) | 7.78 (5.04) | –1.19 | Down | .5828 |
Acetate | 25.69 (7.99) | 29.85 (8.26) | –1.16 | Down | .0207 b |
Acetoacetate | 14.33 (10.92) | 12.64 (8.21) | 1.13 | Up | .6822 |
Acetone | 15 (4.34) | 18.46 (5.83) | –1.23 | Down | .0039 b |
Alanine | 284.62 (65.96) | 267.32 (53.39) | 1.06 | Up | .1440 |
Betaine | 21.99 (7.84) | 20.05 (8.39) | 1.1 | Up | .2056 |
Carnitine | 21.08 (4.88) | 19.98 (4.82) | 1.06 | Up | .1894 |
Choline | 7.68 (3.02) | 7.49 (2.69) | 1.03 | Up | .8123 |
Citrate | 59.29 (11.58) | 61.85 (13.38) | –1.04 | Down | .4510 |
Creatine | 25.5 (11.41) | 23.84 (11.5) | 1.07 | Up | .3449 |
Creatinine | 36.96 (8.1) | 35.54 (9.46) | 1.04 | Up | .1879 |
Dimethyl sulfone | 5.06 (3.56) | 4.78 (2.73) | 1.06 | Up | .9703 |
Ethanol | 46.68 (25.73) | 32.21 (16.46) | 1.45 | Up | .013 b |
Glucose | 3241.19 (898.95) | 3171.93 (754.22) | 1.02 | Up | .7376 |
Glutamate | 52.21 (14.83) | 56.4 (12.69) | –1.08 | Down | .1147 |
Glutamine | 311.3 (55.65) | 310.98 (52.92) | 1 | Up | 1.0000 |
Glycerol | 124.62 (45.58) | 133.9(34.38) | –1.07 | Down | .1614 |
Glycine | 141.82 (37.97) | 135.06 (40.06) | 1.05 | Up | .2040 |
Isobutyrate | 4.59 (1.89) | 4.65 (2.06) | –1.01 | Down | .9888 |
Isoleucine | 43.24 (16.72) | 40.9 (11.7) | 1.06 | Up | .6652 |
Lactate | 1236.89 (410.48) | 1270.97 (604.28) | –1.03 | Down | .7341 |
Leucine | 73.74 (23.04) | 69.55 (16.39) | 1.06 | Up | .7028 |
Lysine | 103.04 (27.01) | 97.44 (29.48) | 1.06 | Up | .2073 |
Malonate | 11.84 (2.88) | 11.11 (3) | 1.07 | Up | .1754 |
Methionine | 16.34 (4.74) | 15.3 (4.66) | 1.07 | Up | .2317 |
Ornithine | 25.15 (7.57) | 22.37 (8.47) | 1.12 | Up | .1365 |
Phenylalanine | 44.46 (12.81) | 43.9 (15.78) | 1.01 | Up | .5058 |
Proline | 111.43 (37.73) | 106 (33.59) | 1.05 | Up | .5484 |
Propylene glycol | 8.84 (2.78) | 8.25 (2.09) | 1.07 | Up | .4047 |
Pyruvate | 63.92 (19.24) | 54.52 (25.5) | 1.17 | Up | .0155 b |
Serine | 75.47 (22.9) | 77.55 (23.11) | –1.03 | Down | .9370 |
Succinate | 3.35 (1.78) | 3.31 (1.13) | 1.01 | Up | .5506 |
Threonine | 112.57 (25.66) | 107.31 (26.21) | 1.05 | Up | .3449 |
Tyrosine | 42.94 (11.38) | 45.63 (19.15) | –1.06 | Down | .9296 |
Valine | 147.88 (37.36) | 143.24 (30.09) | 1.03 | Up | .5148 |
3-Methylhistidine | 26.93 (13.26) | 23.74 (15.16) | 1.13 | Up | .0185 b |
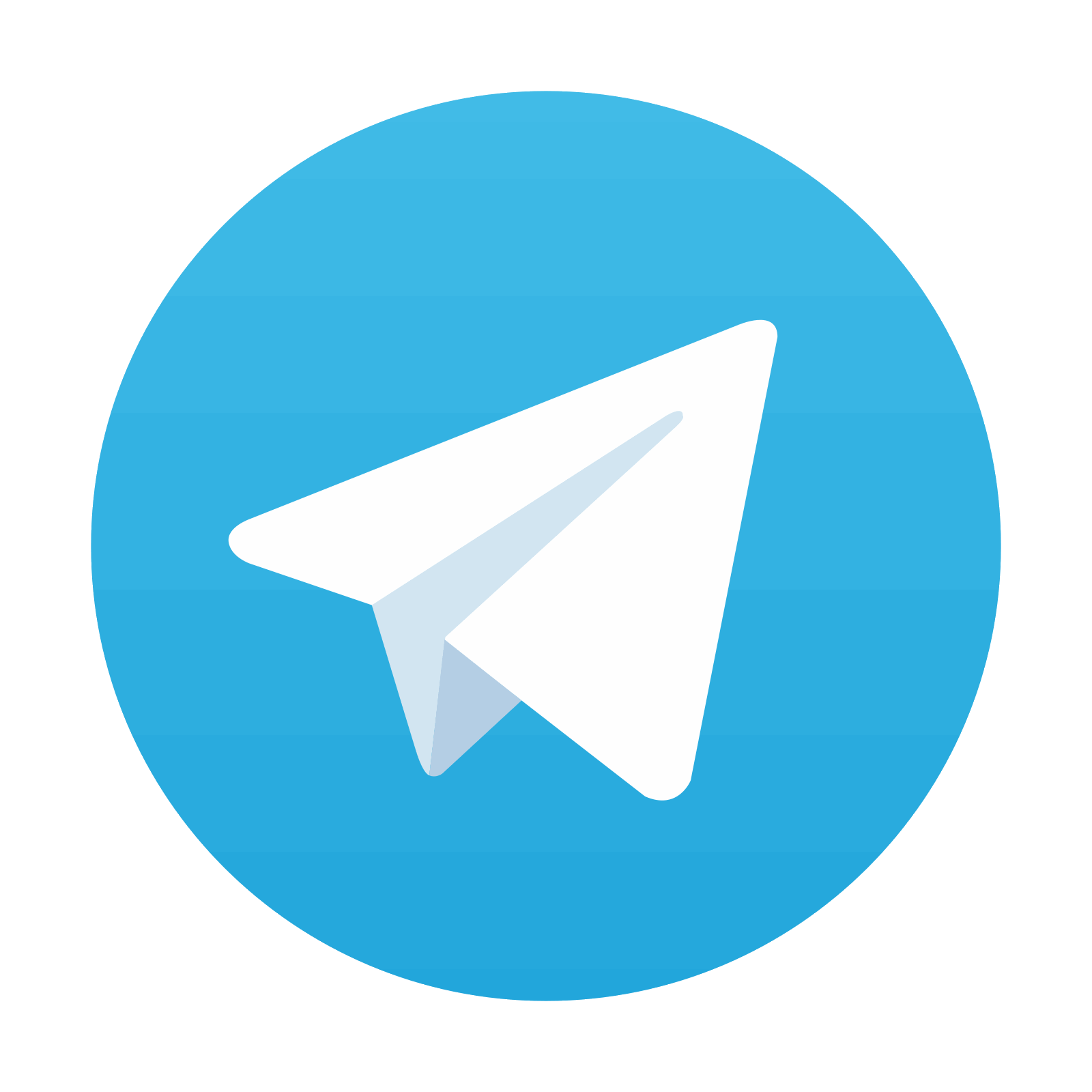
Stay updated, free articles. Join our Telegram channel

Full access? Get Clinical Tree
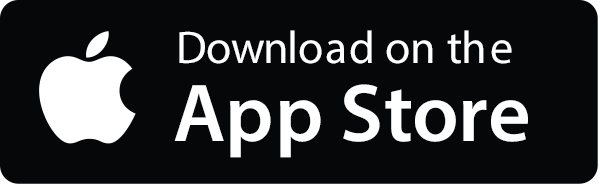

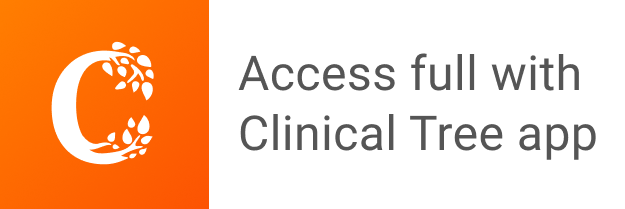