Objective
The objective of the study was to perform first-trimester maternal serum metabolomic analysis and compare the results in aneuploid vs Down syndrome (DS) pregnancies.
Study Design
This was a case-control study of pregnancies between 11+0 and 13+6 weeks. There were 30 DS cases and 60 controls in which first-trimester maternal serum was analyzed. Nuclear magnetic resonance-based metabolomic analysis was performed for DS prediction.
Results
Concentrations of 11 metabolites were significantly different in the serum of DS pregnancies. The combination of 3-hydroxyisovalerate, 3-hydroxybuterate, and maternal age had a 51.9% sensitivity at 1.9% false-positive rate for DS detection. One multimarker algorithm had 70% sensitivity at 1.7% false-positive rate. Novel markers such as 3-hydroxybutyrate, involved in brain growth and myelination, and 2-hydroxybutyrate, involved in the defense against oxidative stress, were found to be abnormal.
Conclusion
The study reports novel metabolomic markers for the first-trimester prediction of fetal DS. Metabolomics provided insights into the cellular dysfunction in DS.
Metabolites are low-molecular-weight organic and inorganic chemicals that are the substrates, intermediates, and byproducts of enzyme-mediated biochemical reactions in the cell. The molecules include but are not limited to carbohydrates, amino acids, peptides, nucleic acids, organic acids, vitamins, lipids, and other biological species. Metabolomics refers to the systematic identification and quantification of these small (less than 1500 Da) molecules.
For Editors’ Commentary, see Contents
See related editorial, page 339
Advances in the development of analytic platforms such as nuclear magnetic resonance (NMR) and high-performance liquid chromatography–mass spectrometry and in statistical programs for analysis of the large volume of data generated in metabolomic studies have fueled the rapidly growing interest in this field.
Metabolites form the building blocks of genes, ribonucleic acid transcripts, and proteins. For these and other reasons, metabolomics is thought to have the potential to provide more fundamental and global information than genomics, proteomics, and transcriptomics and to more precisely reflect the phenotype of the cell. The human serum metabolome has now been extensively characterized. A total of 4229 confirmed and highly probable compounds have recently been reported.
One practical application of metabolomics has been in the development of biomarkers for disparate and complex disorders such as schizophrenia, meningitis, and colon cancer. To date, there has been only very limited use of metabolomics in obstetrics.
Routine screening for Down syndrome (DS) in pregnancy has been the standard of clinical care in the United States and other developed countries. The identification of new biomarkers for DS screening continues to be an area of intense research interest.
The purpose of this study was to determine whether metabolomic markers in maternal blood can distinguish DS from normal pregnancies during the first trimester. We also performed a preliminary evaluation of the diagnostic accuracy of some of these markers.
Materials and Methods
This was a case-control study drawn from a large prospective study to identify potential biomarkers of pregnancy complications in women attending for their routine first hospital visit in pregnancy at King’s College Hospital (London, UK) from March 2003 to February 2009. In this visit, which is held at 11 +0 -13 +6 weeks of gestation, all women have combined screening for aneuploidies. Maternal demographic characteristics, ultrasononographic measurements, and biochemical results were recorded in a computer database. Karyotype results and details on pregnancy outcomes were added into the database as soon as they became available. All cases of trisomy 21 had a fetal karyotype confirmed following a chorionic villus sampling. The controls were selected from women delivering live-born neonates at full term with a normal karyotype or a normal phenotypic examination.
Written informed consent was obtained from the women agreeing to participate in the study, which was approved by the King’s College Hospital Ethics Committee. Maternal venous blood collected in plain BD vacutainer tubes (Becton Dickinson UK Limited, Oxfordshire, UK) was processed within 15 minutes of blood collection and centrifuged at 3000 rpm for 10 minutes to separate serum from packed cells. The maternal serum obtained after centrifugation was then divided into 0.5 mL aliquots and stored at –80°C until subsequent analysis. None of the samples were previously thawed and refrozen.
An aliquot of their serum were used for the measurement of free β-human chorionic gonadotropin (hCG) and pregnancy associated plasma protein A (PAPP-A). The serum samples were transported to the laboratory (Edmonton, Canada) for metabolomic testing by air courier on dry ice and maintained in a frozen state.
The case-control study population consisted of 30 cases with fetal trisomy 21 and 60 euploid controls. Each case of fetal trisomy 21 was randomly chosen from archival specimens and was matched with 2 euploid controls with blood collected within 1 week of each other. None of the samples in the case-control study were previously thawed and refrozen or used in any pilot study.
NMR spectroscopy was the analytic platform used for metabolomic analysis. NMR is very quantitative but is not a particularly sensitive approach for metabolomics. Typically only 35-45 compounds can be identified or selected from a serum NMR spectrum on routine analysis. We have previously described in detail the sample preparation, spectroscopic methods, and statistical analyses used. A 500 MHz Varian Inova NMR spectrometer was used to collect the spectra of all serum samples. Overall, 40 serum metabolites were identified, quantified, and compared in each DS and normal case using the NMR Chenomx NMR Suite 7.1 (Edmonton, Canada), a commercial software package for spectral identification and quantification of metabolites. It is from this set of 40 detectable metabolites that the DS metabolite signature was determined. Standard statistical techniques recommended for metabolomic analysis including normalization, principal component analysis (PCA), and partial least squares discriminant analysis (PLS-DA) were used.
Data normalization is important to reduce systematic bias or technical variation and to improve statistical robustness. This was accomplished using log normalization. PCA is a multivariate technique that identifies the uncorrelated variables or metabolites (principal components) that account for the largest variance or difference between the study and control groups. The metabolites are ordered based on their contribution to the variance between the 2 groups. In the PCA plot, the first principal component is represented on the X-axis and the second most important on the Y-axis (with the third principal component on the Z-axis in a 3-dimensional plot). The minimum number of principal components that when combined together accounts for the highest percentage of the total variance between groups is sought. Clustering of the data points on the PCA plot provides visual evidence that the study and control groups can be discriminated by measuring the principal components.
PLS-DA was used to further enhance the discrimination between study and control groups. With PLS-DA analysis, the principal components are rotated to find the metabolites most responsible for separating cases from controls PLS-DA therefore improves separation between the groups. PLS-DA is typically performed after separation is demonstrated on PCA. There is a risk in PLS-DA analysis of observing separation between controls and study group that might not be statistically significant. To minimize this risk, permutation testing is performed with random relabeling of the metabolomic data and rerunning of the PLS-DA analysis. After 2000 repetitions of the relabeling process, the P values of the probability that the observed separation might be due to chance were calculated. PCA and PLS-DA analyses were performed with a MetaboAnalyst computer program.
A variable importance in projection (VIP) plot was also constructed. This is a graph of the relative contributions of individual metabolites to the variance between the 2 groups. The higher the VIP value (X- and Y-axis), the greater is the individual contribution of that metabolite to group separation.
Statistical analysis
Mean and SD metabolite concentrations were compared between groups. Logistic regression analysis was performed using a limited number of metabolites only and metabolites plus maternal age for the prediction of DS. Based on these regression equations, receiver operating characteristic (ROC) curves plotting sensitivity against false-positive rate values for DS prediction were generated. Correlation analysis between the most important metabolites based on VIP and maternal age and ethnicity, which are commonly used to standardize DS biomarkers, were performed. Kolmogorov Smirnov and Shapiro-Wilk tests of normal distribution were performed. Power analysis indicated that a minimum of 17 cases and 34 controls were needed in each group to have 80% power for a 2-sided P < .05. The statistical significance was set at P < .05.
Apart from standard statistical analyses, genetic programming (GP) was utilized to identify significant metabolite and other predictors of DS. The diagnostic accuracies of various marker combinations were evaluated with GP. These included demographic and clinical parameters (maternal age, ethnicity, weight, parity, tobacco use, and the presence of medical disorders along with the metabolites). Evolutionary algorithms, including genetic algorithms, and their use in metabolomic analyses have been the subjects of prior reviews. Genetic computing is a branch of GP. We had previously published an explanation of this approach. Briefly, GP uses the principles of evolutionary genetics, namely selection, recombination, and mutation. Rules are generated by which the optimal combinations of predictive markers are identified. GP does not suffer from the limitations of conventional statistical analysis (eg, requiring normality of the data distribution and handling the absence of data points) and is equally useful for evaluating extremely large data collections or a small volume of data and has been claimed to be superior to standard statistical analyses. GP analysis was performed by The GMAX computer program, version 11.9.23 ( www.Thegmax.com ).
Results
Results were obtained for 30 DS cases and 60 controls. The demographic and clinical characteristics of DS cases and controls are shown in Table 1 . As expected, DS mothers were significantly older than control mothers. In addition, there were racial differences between the 2 groups. In Table 2 , the serum metabolite concentrations are compared between cases and controls. A total of 11 metabolites had significantly different concentrations between the DS and normal groups, whereas the differences were of borderline significance in the case of 2 metabolites. Figure 1 , A and B, show 2- and 3-dimensional PCA plots. The contributions of the principal components to the variance between DS and normal groups are shown on the X- and Y- axes on the 2-dimensional PCA plot (and Z-axis in the case of the 3-dimensional plot). There is visual evidence of separation between the 2 groups. The corresponding PLS-DA plots are shown in Figure 2 , A and B.
Parameter | Down syndrome | Euploid control | P value |
---|---|---|---|
Number of cases | 30 | 60 | — |
Maternal age (y), mean (SD) | 37.2 (3.9) | 30.5 (7.1) | < .01 |
Racial origin, n (%) | .012 | ||
White | 27 (90) | 33 (55.9) | |
Black | 2 (6.7) | 19 (32.2) | |
Asian | 1 (3.3) | 4 (6.8) | |
Mixed | 0 (0) | 3 (5.1) | |
Nullipara, n (%) | 8 (26.7) | 25 (42.4) | .11 |
Weight (kg), mean (SD) | 66.3 (14.9) | 67.0 (15.0) | .83 |
Medical disorder present | 6 (20) | 5 (8.5) | .11 |
Crown-rump length (mm), mean (SD) | 67.2 (7.4) | 64.8 (8.7) | .19 |
Metabolite | Down syndrome Mean (SD) | Euploid controls Mean (SD) | P value |
---|---|---|---|
Number of cases | 30 | 60 | — |
2-hydroxybutyrate | 23.1 (14.1) | 15.0 (7.4) | .008 |
3-hydroxybutyrate | 62.7 (85.0) | 21.0 (19.4) | .018 |
2-hydroxyisovalerate | 6.9 (4.0) | 4.1 (2.8) | .002 |
Acetamide | 9.2 (5.5) | 6.4 (4.9) | .028 |
Acetate | 27.5 (17.6) | 30.1 (22.6) | .576 |
Acetoacetate | 23.5 (24.3) | 13.9 (7.3) | .054 |
Acetone | 15.6 (8.7) | 10.5 (3.3) | .007 |
Alanine | 247.2 (89.1) | 231.9 (87.3) | .46 |
Arginine | 125.6 (37.3) | 132.7 (50.7) | .516 |
Asparagine | 27.3 (9.6) | 25.6 (10.3) | .48 |
Betaine | 25.4 (5.5) | 27.0 (8.9) | .411 |
Carnitine | 25.5 (9.7) | 19.5 (8.3) | .004 |
Choline | 31.6 (113.2) | 52.7 (143.9) | .507 |
Citrate | 57.2 (18.7) | 51.9 (16.5) | .208 |
Creatine | 37.4 (13.3) | 34.4 (11.8) | .308 |
Creatinine | 50.1 (12.6) | 48.0 (11.9) | .466 |
Dimethylamine | 33.6 (19.0) | 49.1 (30.6) | .019 |
Ethanol | 14.4 (10.4) | 14.1 (8.4) | .859 |
Formate | 3479.9 (628.4) | 3496.6 (620.1) | .90 |
Glucose | 245.5 (96.0) | 217.3 (73.8) | .148 |
Glutamine | 150.4 (128.0) | 174.3 (216.4) | .60 |
Glycerol | 205.7 (57.44) | 203.1 (79.9) | .88 |
Glycine | 5.1 (2.5) | 4.1 (1.7) | .056 |
Isobutyrate | 38.0 (14.0) | 35.2 (15.4) | .434 |
Isopropanol | 4.7 (4.2) | 6.1 (5.3) | .229 |
Lactate | 1172.1 (683.4) | 884.7 (369.2) | < .05 |
Leucine | 67.3 (34.6) | 60.0 (46.9) | .87 |
Malonate | 13.7 (7.1) | 15.8 (9.7) | .336 |
Methionine | 25.0 (6.9) | 32.6 (11.5) | < .001 |
Ornithine | 30.5 (12.1) | 26.0 (10.8) | .10 |
Phenylalanine | 55.4 (27.0) | 46.2 (30.8) | .191 |
Proline | 142.2 (60.4) | 134.0 (52.4) | .53 |
Propyl-glycol | 7.0 (2.9) | 6.6 (1.9) | .49 |
Pyruvate | 77.9 (32.4) | 61.0 (26.4) | .014 |
Serine | 135.3 (45.2) | 148.4 (52.2) | .29 |
Succinate | 5.3 (5.6) | 5.6 (12.5) | .91 |
Threonine | 123.3 (31.8) | 119.3 (45.2) | .68 |
Tyrosine | 50.5 (15.4) | 54.1 (20.7) | .436 |
Valine | 117.1 (33.4) | 114.8 (34.9) | .774 |
L-methylthistidine | 40.4 (12.3) | 32.7 (13.7) | .016 |


Figure 3 is a VIP plot that indicates that 3-hydroxybutyrate, 3-hydroxyisovalerate, and 2-hydroxybutyrate are the most discriminating metabolites. On the right of the VIP plot is a heat map. In the trisomy 21 column, red indicates that the concentration of the particular metabolite was increased in the DS group compared with normal, whereas green indicates reduced metabolite concentration. As shown in Figure 3 , a total of 2000 permutations were performed and the separation observed between the groups was highly statistically significant ( P = .0025). Using standard regression models based on a limited number of metabolites only as shown in Table 3 , 48.1% DS detection rate was achieved at a 3.1% false-positive rate. Furthermore, a specificity rate of 100% (0% false-positive rate) and a detection rate of 40.7% was observed with this particular algorithm.

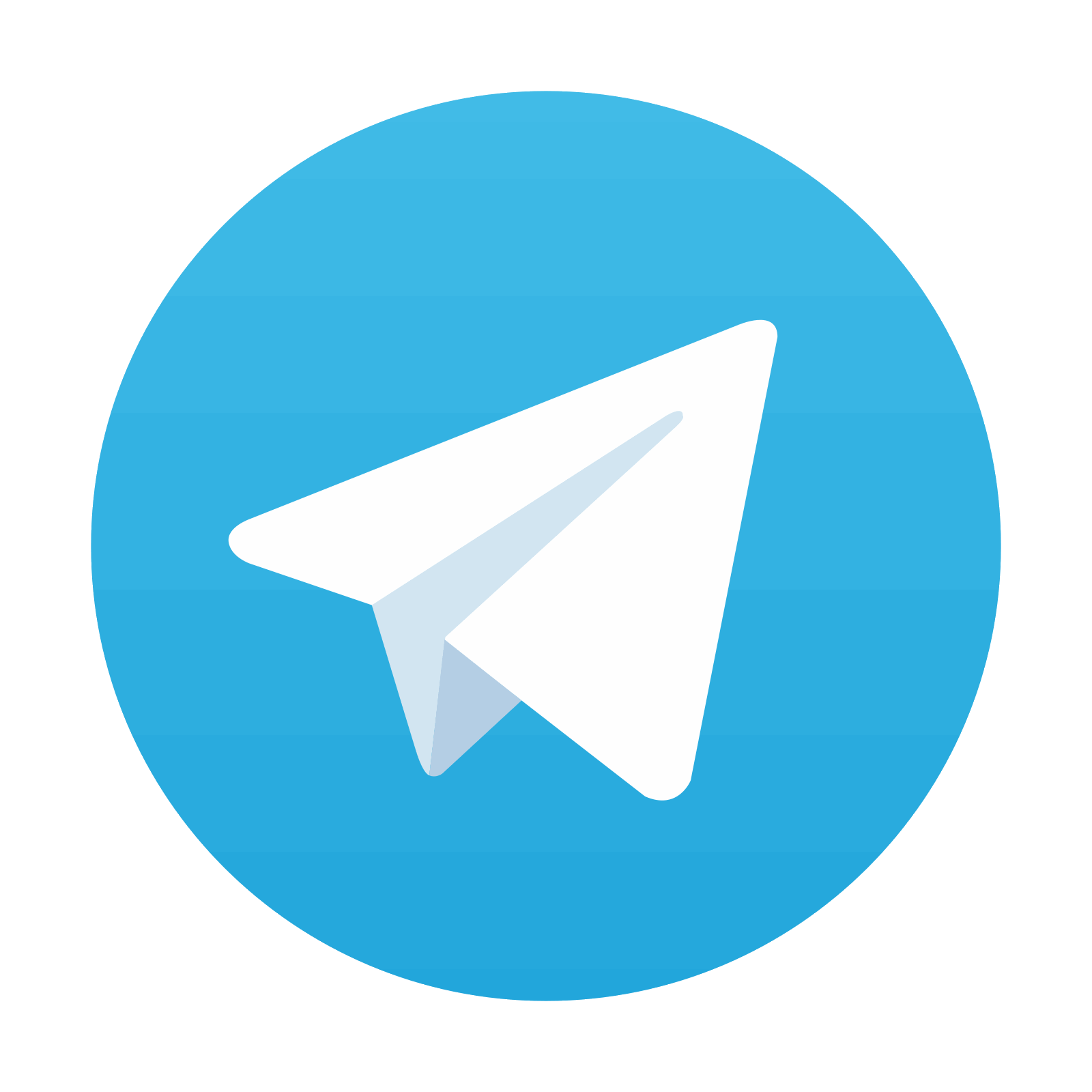
Stay updated, free articles. Join our Telegram channel

Full access? Get Clinical Tree
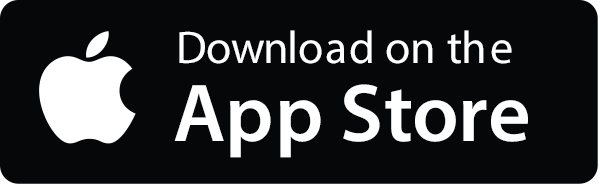
