Objective
Prelabor cesareans in women without a prior cesarean is an important quality measure, yet one that is seldom tracked. We estimated patient-level risks and calculated how sensitive hospital rankings on this proposed quality metric were to risk adjustment.
Study Design
This retrospective cohort study linked Californian patient data from the Agency for Healthcare Research and Quality with hospital-level operational and financial data. Using the outcome of primary prelabor cesarean, we estimated patient-level logistic regressions in progressively more detailed models. We assessed incremental fit and discrimination, and aggregated the predicted patient-level event probabilities to construct hospital-level rankings.
Results
Of 408,355 deliveries by women without prior cesareans at 254 hospitals, 11.0% were prelabor cesareans. Including age, ethnicity, race, insurance, weekend and unscheduled admission, and 12 well-known patient risk factors yielded a model c-statistic of 0.83. Further maternal comorbidities, and hospital and obstetric unit characteristics only marginally improved fit. Risk adjusting hospital rankings led to a median absolute change in rank of 44 places compared to rankings based on observed rates. Of the 48 (49) hospitals identified as in the best (worst) quintile on observed rates, only 23 (18) were so identified by the risk-adjusted model.
Conclusion
Models predict primary prelabor cesareans with good discrimination. Systematic hospital-level variation in patient risk factors requires risk adjustment to avoid considerably different classification of hospitals by outcome performance. An opportunity exists to define this metric and report such risk-adjusted outcomes to stakeholders.
Cesareans represent the most common major surgical procedure in the United States. The rise in utilization, variation in rates, and concerns about unnecessary procedures are driving national debate among providers, payers, regulators, and patients.
Unnecessary procedures pose potential immediate medical harms to both mother and child, negatively impact maternal attachment and breast-feeding rates, lead to potential harms in future pregnancies, and do not prevent pelvic floor dysfunction.
Current US rates of cesarean are high and more than double the consensus 10-15% optimal rate defined by the World Health Organization, and still far above the objective of 23.9% for low-risk nulliparous pregnancies set by Healthy People 2020. Especially when performed without a woman having begun to labor, many cesareans may not be medically indicated, although they can be ethically performed on an informed patient’s request.
Preventing unnecessary first cesarean delivery is a valid national goal for quality improvement in maternity care. Well-known research has long identified indications for primary prelabor cesareans using administrative data yet this quality measure is not widely calculated or reported.
To support this and the related goal of public reporting of meaningful quality metrics, we used risk-adjustment techniques to estimate patient- and hospital-level prelabor cesarean rates among women without prior cesarean in a large sample of California hospitals. We then explored the extent to which systematic variation across hospitals in hospital, maternal, fetal, and placental risk factors affected hospital rankings on this quality measure.
Materials and Methods
We conducted a cross-sectional study using data from all inpatient admissions to Californian state-regulated hospitals in 2010 in the State Inpatient Database datasets of the Agency for Healthcare Research and Quality (AHRQ) Healthcare Cost and Utilization Project. Our patient-level analytic dataset was constructed as follows ( Appendix ; Supplementary Table 1 and Figure 1 ). We included patient admissions with any instances of International Classification of Diseases, Ninth Revision, Clinical Modification ( ICD-9-CM ) codes of outcomes of delivery. We excluded patients with nondelivery codes. We excluded patients with a history of cesarean, missing age due to masking, or aged <14 years. Our analytic dataset thus comprised 408,355 records ( Figure 1 ). This study qualified for expedited review under 45 Code of Federal Regulations 46.110 (b)(2) and was approved by the Institutional Review Board of the University of Southern California.

We followed the algorithm of Gregory et al closely. We identified cesareans by ICD-9-CM procedural codes (74, 74.0, 74.1, 74.2, 74.4, 74.9, or 74.99) in any 1 of 21 procedure fields and/or a diagnostic-related group code for cesarean (370, 765, 371, or 766). To identify the presence of labor during this admission, we coded for the presence of vaginal delivery, cephalic version from breech (652.1X), disproportion (653.XX), obstructed labor (660.XX), abnormality of forces of labor (661.XX), long labor (662.XX), failed induction of labor (659.0X and 659.1X), fetal distress (656.3), and cord prolapse (663.0). The coded presence of a cesarean in the absence of coded labor was defined as the outcome of interest. In sensitivity analyses, we classified deliveries in which a diagnosis of nonreassuring fetal heart rate (FHR) ( ICD-9-CM diagnostic code 659.71) had been made as occurring after labor, removing them from the outcome of interest.
We recorded 39 patient characteristics using AHRQ data on demographics, admission, and comorbidities ( Table 1 ). We coded for age, Hispanic ethnicity, race, primary insurance or payer status, the quartile of state income to which the median household income in the patient ZIP code belonged, whether the admission was scheduled, or occurred on a weekend. We coded for 12 well-known maternal, fetal, and placental risk factors previously shown to be associated with prelabor cesareans. We also coded for a further 13 maternal comorbidities associated with higher-risk pregnancies.
Demographics | Labored, vaginal or cesarean | Prelabor cesarean | P value a |
---|---|---|---|
(n = 363,624) | (n = 44,731) | ||
Age, y | 27.7 | 29.6 | < .0001 |
Race, % b | < .0001 | ||
White | 58.2 | 57.2 | |
Unknown race | 23.9 | 22.5 | |
Asian-Pacific Islander | 11.8 | 12.3 | |
Black | 5.8 | 7.6 | |
Ethnicity, % b | |||
Hispanic | 46.2 | 39.4 | < .0001 |
Prelabor cesarean risk factors, % c | |||
Malpresentation | 2.3 | 32.0 | < .0001 |
Preterm gestation | 5.1 | 17.8 | < .0001 |
Other hypertension | 5.6 | 11.2 | < .0001 |
Maternal soft-tissue disorder | 1.8 | 9.2 | < .0001 |
Multiple gestation | 0.6 | 8.9 | < .0001 |
Antepartum hemorrhage | 0.9 | 8.3 | < .0001 |
Macrosomia | 1.8 | 8.2 | < .0001 |
Hypertension | 0.8 | 5.4 | < .0001 |
High head | 1.2 | 3.7 | < .0001 |
Herpes | 1.5 | 3.3 | < .0001 |
Uterine scar | 0.0 | 1.1 | < .0001 |
Fetal congenital abnormality | 0.1 | 0.4 | < .0001 |
Pregnancy risk factors, % d | |||
Diabetes | 6.9 | < .0001 | |
Oligohydramnios | 2.6 | < .0001 | |
Intrauterine growth disorder | 1.2 | < .0001 | |
Mental illness | 2.7 | < .0001 | |
Asthma | 2.6 | < .0001 | |
Thyroid disease | 1.8 | < .0001 | |
Chorioamnionitis | 2.6 | .486 | |
Rhesus disease | 1.6 | 1.7 | .189 |
Heart disease | 0.4 | 0.8 | < .0001 |
Kidney disease | 0.2 | 0.3 | < .0001 |
Liver disease | 0.1 | 0.2 | < .0001 |
Substance abuse disorder | 0.1 | 0.2 | < .0001 |
Cerebrovascular disease | <0.1 | 0.1 | < .0001 |
Peripartum diagnosis, % | |||
Fetal heart rate abnormality e | 13.4 | 31.5 | < .0001 |
a Values from χ 2 tests for variables with categorical values and Kruskal-Wallis tests for those with continuous values
b Ethnicity is independent of race
d As in Henry et al and Gregory et al
e Diagnostic International Classification of Diseases, Ninth Revision, Clinical Modification code 659.71.
We associated hospitals in the 2010 patient-level data with 2 sources of hospital-level data from the Californian Office of Statewide Health Planning and Development ( Table 2 ). California Automated Licensing Information and Tracking System data were available from the close of the 2010 calendar year. Using Automated Licensing Information and Tracking System data, we recorded hospital ownership type, number of beds and bassinets, teaching hospital status, total number of yearly discharges, and the 24-hour on-premise availability of selected physician types and services.
Characteristics | Labored, vaginal or cesarean | Prelabor cesarean | P value a |
---|---|---|---|
(n = 363,624) | (n = 44,731) | ||
Hospital beds, total b | 320.3 | 341.5 | .0001 |
Perinatal | 33.4 | 34.7 | .0001 |
Pediatric | 13.5 | 14.6 | .0001 |
NICU | 19.9 | 21.5 | .0001 |
Ownership b | < .0001 | ||
Nonprofit | 69.6 | 69.5 | |
Investors | 14.2 | 14.6 | |
City, district, or county | 14.1 | 12.9 | |
State | 2.2 | 3.0 | |
Original license date, mean | May 1962 | September 1961 | .0001 |
Hospital discharges, total b | 15,431 | 16,472 | .0001 |
Teaching hospital, % b | 11.8 | 12.4 | < .0001 |
24-h on-premise availability, % b | |||
Physician | 85.3 | 85.9 | .002 |
Operating room | 61.7 | 65.7 | < .0001 |
Laboratory services | 86.3 | 87.0 | < .0001 |
Anesthesiologist | 63.1 | 67.0 | < .0001 |
ED anesthesia | 60.4 | 63.6 | < .0001 |
ED operating room | 57.8 | 61.1 | < .0001 |
BC/BE professional staff, total | |||
Anesthesiologists, hospital based | 19.5 | 21.3 | .0001 |
Obstetricians, hospital based | 12.1 | 12.2 | .76 |
Obstetricians, off-hospital | 21.1 | 24.5 | .0001 |
Fellows and residents, total | |||
Anesthesiology | 1.9 | 2.4 | .0001 |
Obstetrics and gynecology | 2.3 | 2.7 | .0001 |
Educational programs, % | |||
Nurse anesthetists | 10.9 | 11.2 | .10 |
Nurse practitioners | 14.6 | 14.7 | .56 |
Nurse midwives | 5.2 | 3.9 | < .0001 |
a Values from χ 2 tests for variables with categorical values and Kruskal-Wallis tests for those with continuous values
b At year end 2010 based on state government Automated Licensing Information and Tracking System data, all other data come from “Year 35” state government regulatory reports and have varying fiscal year end dates of June 30, 2009; Sept. 30, 2009; Dec. 31, 2009; and Jan. 31, 2010 all data are patient-weighted means by category.
Additionally, within 4 months of the end of their respective fiscal year end, licensed hospitals in California submit detailed operational and financial data. Due to differences in hospital reporting dates and fiscal years, these data were variously reported on June 30, 2009; Sept. 30, 2009; Dec. 31, 2009; and Jan. 31, 2010. We used these data to record the original license date of the hospital, detailed hospital-level financials and key financial ratios, overall hospital payer mix, professional staffing with attending and trainee anesthesiologists and obstetricians, presence of particular nurse educational programs, and obstetric productivity and operations data.
For patient characteristics we present means, and for hospital characteristics we present patient-weighted means. We used χ 2 tests for differences in categorical variables and Kruskal-Wallis equality of population tests for differences in continuous variables between those women without a prior cesarean who labored, and those who instead received a prelabor cesarean.
We constructed a series of progressively more saturated logistic regression models on the outcome of interest. For each model, we recorded the generalized R 2 and the c-statistic, or discrimination of the model. The c-statistic can be interpreted as the probability that the model correctly identifies the 1 patient who actually experienced the outcome, from a randomly chosen pair of patients comprising 1 who did and 1 who did not.
Recent research has nevertheless shown that models with only small improvements in c-statistic can nevertheless drive large changes in patient risk classification. To provide information beyond changes in the c-statistic, we calculated the estimated Integrated Discrimination Improvement. We did not test model calibration in this large-scale study, as the use of the Hosmer-Lemeshow calibration test has been shown to be inappropriate in datasets >50,000 records.
Lastly, we used the predicted probabilities of the event to generate hospital-level predicted rates of primary prelabor cesarean. We constructed observed/expected ratios and used these to rank hospitals. We contrasted these rankings with rankings of the same hospitals by the observed, unadjusted rate, and constructed hospital reclassification tables. We used SAS software (version 9.2; SAS Institute, Cary, NC) for data management while statistical analyses were performed using STATA (version 12.1; StataCorp LP, College Station, TX).
Results
A total of 408,355 women met the study inclusion and exclusion restrictions ( Figure 1 ). Of these women without prior cesarean, 44,731 women (10.95%) experienced a prelabor cesarean. They were significantly more likely to be older, of black race, from a ZIP code with higher household income, scheduled, and privately insured ( Supplementary Table 2 ). Hispanic ethnicity was significantly less likely among women receiving a prelabor cesarean as was weekend admission and Medi-Cal (California’s Medicaid program) insurance.
Of the 12 maternal, fetal, and placental conditions known to be associated with prelabor cesarean ( Table 1 ), 71.4% of those experiencing the outcome had ≥1, compared to 18.4% ( P < .0001) who delivered vaginally or labored and then received a cesarean. Of 13 other maternal risk factors, 35.6% receiving a prelabor cesarean had ≥1 conditions, while 21.0% did not ( P < .0001).
Among the total 254 hospitals in the study sample there were significant differences in key patient-weighted mean characteristics ( Table 2 ). Among patients who experienced a prelabor cesarean, their hospitals were significantly more likely to be larger, owned by investors or the state, or be a teaching hospital ( Supplementary Table 3 ), however the hospitals of such patients had significantly lower return on assets despite slightly lower rates of charity and uncompensated services, and a payer mix that was generally comparable.
Among patients experiencing the outcome, their hospital was more likely to have anesthesia and obstetric service available when needed, while the presence of nurse education programs such as certified registered nurse anesthetist was not significantly different. Obstetric standard service units were significantly higher in the hospitals of those patients (9835 vs 9098 adjusted patient days per year, P < .001). There were no meaningful differences in productivity or expenses per service unit (total expenses per adjusted obstetric patient day $1299 vs $1302).
Our model-building strategy was to progressively saturate an initial patient-level null model containing age alone ( Supplementary Table 4 ) with initially very low explanatory power and relatively low discrimination of 0.58.
Adding race, ethnicity, insurance status, scheduled, weekend admission, and the 12 prelabor cesarean risk factors increased the amount of explained variance to 26% and the c-statistic to a very substantial 0.83, with concomitant increase in integrated discrimination to 27.7%. Following this model, further addition of patient and hospital characteristics including county of hospital location yielded only very small improvements in explanatory power (to 0.28), c-statistic (to 0.85), and integrated discrimination (to 29.6%).
We report the estimated coefficients of our selected model (model XIII, Supplementary Table 5 ) and used the predictions of event probability from this model in ranking analyses. This model contained all but hospital operational and financial data, which were missing for all Kaiser Permanente hospitals.
Across 254 hospitals in the patient-level dataset, the unweighted observed mean prelabor cesarean rate was 11.1% (SD 8.6%; median 9.9%) as a fraction of hospital deliveries in women without prior cesarean. In subsequent ranking analyses we excluded 3 hospitals with <100 deliveries in 2010, and 10 hospitals with considerable missing data for the hospital operational covariates. With the exclusion of these outliers the unweighted mean prelabor cesarean rate for the 241 hospitals fell slightly to 10.4% (SD 4.0%) but the median was preserved.
We aggregated the patient-level predictions of the outcome from our selected model across these 241 hospitals, and computed the ratio of observed to expected rates. We contrast the distribution of actual prelabor cesarean rates against the more closely fitting normal-like distribution of the risk-adjusted rate in Figure 2 .

We used these distributions of unadjusted and risk-adjusted prelabor cesarean rates to construct rankings of each of the 241 hospitals along each dimension. The best quintile (ie, with the lowest rate) based on observed, unadjusted rate had a mean of 5.8%, the worst quintile 16.4%. By risk-adjusted rate, the best quintile mean was 7.9%, the worst was 14.6%.
Our reclassification analysis examined agreement between centile membership according to whether unadjusted or risk-adjusted rankings were used. The median absolute change across the 2 rankings was 44 places (interquartile range, 15–95). We found substantial disagreement between the 2 rankings ( Table 3 ). Of the 48 hospitals ranked in the top quintile by observed rates, only 23 were so ranked by risk-adjusted rates. Similarly, of the 49 hospitals ranked in the worst quintile by observed rates, only 18 were so ranked by risk-adjusted rates.
Rank based on actual observed prelabor cesarean rate a | Risk-adjusted rank based on risk-adjusted model b | Total | ||
---|---|---|---|---|
Best 20% | Middle 60% | Worst 20% | ||
Best 20% | 23 | 21 | 4 | 48 |
Middle 60% | 22 | 95 | 27 | 144 |
Worst 20% | 3 | 28 | 18 | 49 |
Total c | 48 | 144 | 49 | 241 |
a Hospital rank calculated on observed rate of prelabor cesarean rate without patient or hospital risk adjustment
b Hospital rank based on ratio of observed rate to rate predicted from estimated event probabilities from model XIII ( Supplemental Tables 1-6 ). We prespecified this risk model as more detailed models had substantial missing data due to Kaiser Permanent hospitals not reporting financials. Model controlled for patient demographic, prelabor cesarean risk factors, pregnancy risk factors, and hospital characteristics ( Tables 1 and 2 )
c Of 254 hospitals with patients who met study inclusion and exclusion restrictions ( Figure 1 ), 13 hospitals were excluded from this ranking analysis. Three delivered <100 women without prior cesarean, 6 hospitals lacked consistent data on 24-h professional service availability, and 4 hospitals lacked consistent data on obstetric operations, load, and complexity.
A total of 105 hospitals changed classes (eg, from best 20% to middle 60%), of which 7 hospitals reversed their rankings from best to worst quintile or vice versa. We repeated this analysis using top 5%, middle 90%, and bottom 5% classes (data not shown), and found a far lower degree of disagreement. A total of 26 hospitals changed classes, and none changed completely from best to worst or vice versa.
In sensitivity analyses we addressed the question of whether coding for nonreassuring FHR was a prelabor marker or a marker of labor. We re-ran the analysis above, but classified deliveries in which FHR abnormalities had been diagnosed as not prelabor. The discrimination of the prelabor cesarean patient-level risk model did not deteriorate but improved, with an increased c-index of 0.91 (data not shown). In Supplementary Table 6 , we show that this definition of quality was still characterized by substantial reclassification; with a comparable median absolute change in rank of 47 places between unadjusted and risk-adjusted outcomes. Finally, we examined the outcome of prelabor cesarean associated with a diagnosis of nonreassuring FHR as has recently been suggested and found broadly similar distribution of this outcome across hospitals, compared to the other quality measures above (data not shown).
Results
A total of 408,355 women met the study inclusion and exclusion restrictions ( Figure 1 ). Of these women without prior cesarean, 44,731 women (10.95%) experienced a prelabor cesarean. They were significantly more likely to be older, of black race, from a ZIP code with higher household income, scheduled, and privately insured ( Supplementary Table 2 ). Hispanic ethnicity was significantly less likely among women receiving a prelabor cesarean as was weekend admission and Medi-Cal (California’s Medicaid program) insurance.
Of the 12 maternal, fetal, and placental conditions known to be associated with prelabor cesarean ( Table 1 ), 71.4% of those experiencing the outcome had ≥1, compared to 18.4% ( P < .0001) who delivered vaginally or labored and then received a cesarean. Of 13 other maternal risk factors, 35.6% receiving a prelabor cesarean had ≥1 conditions, while 21.0% did not ( P < .0001).
Among the total 254 hospitals in the study sample there were significant differences in key patient-weighted mean characteristics ( Table 2 ). Among patients who experienced a prelabor cesarean, their hospitals were significantly more likely to be larger, owned by investors or the state, or be a teaching hospital ( Supplementary Table 3 ), however the hospitals of such patients had significantly lower return on assets despite slightly lower rates of charity and uncompensated services, and a payer mix that was generally comparable.
Among patients experiencing the outcome, their hospital was more likely to have anesthesia and obstetric service available when needed, while the presence of nurse education programs such as certified registered nurse anesthetist was not significantly different. Obstetric standard service units were significantly higher in the hospitals of those patients (9835 vs 9098 adjusted patient days per year, P < .001). There were no meaningful differences in productivity or expenses per service unit (total expenses per adjusted obstetric patient day $1299 vs $1302).
Our model-building strategy was to progressively saturate an initial patient-level null model containing age alone ( Supplementary Table 4 ) with initially very low explanatory power and relatively low discrimination of 0.58.
Adding race, ethnicity, insurance status, scheduled, weekend admission, and the 12 prelabor cesarean risk factors increased the amount of explained variance to 26% and the c-statistic to a very substantial 0.83, with concomitant increase in integrated discrimination to 27.7%. Following this model, further addition of patient and hospital characteristics including county of hospital location yielded only very small improvements in explanatory power (to 0.28), c-statistic (to 0.85), and integrated discrimination (to 29.6%).
We report the estimated coefficients of our selected model (model XIII, Supplementary Table 5 ) and used the predictions of event probability from this model in ranking analyses. This model contained all but hospital operational and financial data, which were missing for all Kaiser Permanente hospitals.
Across 254 hospitals in the patient-level dataset, the unweighted observed mean prelabor cesarean rate was 11.1% (SD 8.6%; median 9.9%) as a fraction of hospital deliveries in women without prior cesarean. In subsequent ranking analyses we excluded 3 hospitals with <100 deliveries in 2010, and 10 hospitals with considerable missing data for the hospital operational covariates. With the exclusion of these outliers the unweighted mean prelabor cesarean rate for the 241 hospitals fell slightly to 10.4% (SD 4.0%) but the median was preserved.
We aggregated the patient-level predictions of the outcome from our selected model across these 241 hospitals, and computed the ratio of observed to expected rates. We contrast the distribution of actual prelabor cesarean rates against the more closely fitting normal-like distribution of the risk-adjusted rate in Figure 2 .

We used these distributions of unadjusted and risk-adjusted prelabor cesarean rates to construct rankings of each of the 241 hospitals along each dimension. The best quintile (ie, with the lowest rate) based on observed, unadjusted rate had a mean of 5.8%, the worst quintile 16.4%. By risk-adjusted rate, the best quintile mean was 7.9%, the worst was 14.6%.
Our reclassification analysis examined agreement between centile membership according to whether unadjusted or risk-adjusted rankings were used. The median absolute change across the 2 rankings was 44 places (interquartile range, 15–95). We found substantial disagreement between the 2 rankings ( Table 3 ). Of the 48 hospitals ranked in the top quintile by observed rates, only 23 were so ranked by risk-adjusted rates. Similarly, of the 49 hospitals ranked in the worst quintile by observed rates, only 18 were so ranked by risk-adjusted rates.
Rank based on actual observed prelabor cesarean rate a | Risk-adjusted rank based on risk-adjusted model b | Total | ||
---|---|---|---|---|
Best 20% | Middle 60% | Worst 20% | ||
Best 20% | 23 | 21 | 4 | 48 |
Middle 60% | 22 | 95 | 27 | 144 |
Worst 20% | 3 | 28 | 18 | 49 |
Total c | 48 | 144 | 49 | 241 |
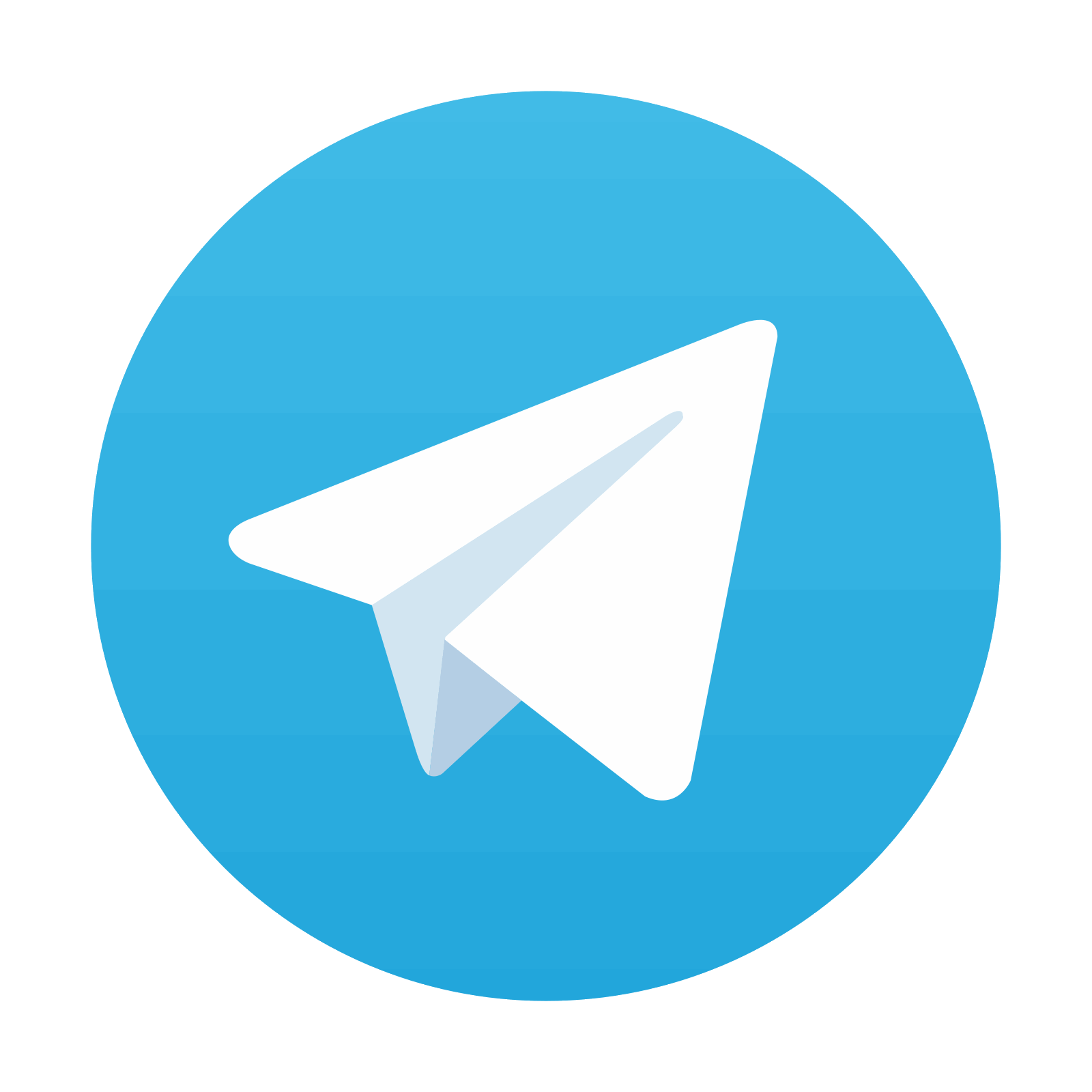
Stay updated, free articles. Join our Telegram channel

Full access? Get Clinical Tree
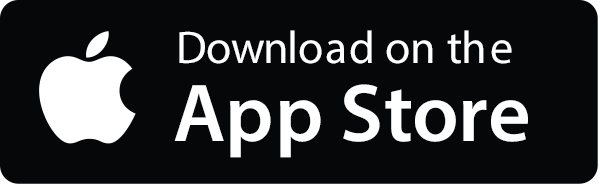

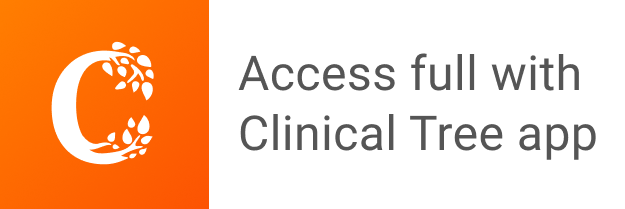