Background
Inflammation is a proximate mediator of preterm birth and fetal injury. During inflammation several microRNAs (22 nucleotide noncoding ribonucleic acid (RNA) molecules) are up-regulated in response to cytokines such as interleukin-1β. MicroRNAs, in most cases, fine-tune gene expression, including both up-regulation and down-regulation of their target genes. However, the role of pro- and antiinflammatory microRNAs in this process is poorly understood.
Objective
The principal goal of the work was to examine the inflammatory genomic profile of human decidual cells challenged with a proinflammatory cytokine known to be present in the setting of preterm parturition. We determined the coding (messenger RNA) and noncoding (microRNA) sequences to construct a network of interacting genes during inflammation using an in vitro model of decidual stromal cells.
Study Design
The effects of interleukin-1β exposure on mature microRNA expression were tested in human decidual cell cultures using the multiplexed NanoString platform, whereas the global inflammatory transcriptional response was measured using oligonucleotide microarrays. Differential expression of select transcripts was confirmed by quantitative real time–polymerase chain reaction. Bioinformatics tools were used to infer transcription factor activation and regulatory interactions.
Results
Interleukin-1β elicited up- and down-regulation of 350 and 78 nonredundant transcripts (false discovery rate < 0.1), respectively, including induction of numerous cytokines, chemokines, and other inflammatory mediators. Whereas this transcriptional response included marked changes in several microRNA gene loci, the pool of fully processed, mature microRNA was comparatively stable following a cytokine challenge. Of a total of 6 mature microRNAs identified as being differentially expressed by NanoString profiling, 2 (miR-146a and miR-155) were validated by quantitative real time–polymerase chain reaction. Using complementary bioinformatics approaches, activation of several inflammatory transcription factors could be inferred downstream of interleukin-1β based on the overall transcriptional response. Further analysis revealed that miR-146a and miR-155 both target genes involved in inflammatory signaling, including Toll-like receptor and mitogen-activated protein kinase pathways.
Conclusion
Stimulation of decidual cells with interleukin-1β alters the expression of microRNAs that function to temper proinflammatory signaling. In this setting, some microRNAs may be involved in tissue-level inflammation during the bulk of gestation and assist in pregnancy maintenance.
The uterine decidua, situated at the interface between the maternal decidua and the fetal compartments, plays a pivotal role in the events leading to both term and preterm parturition in humans. Decidual activation by cytokines and physical stretch drives the molecular cascades that prompt the onset of labor at term, and disruption of decidual integrity by infection or bleeding causes localized inflammation that is associated with preterm birth (PTB). Classic studies by Gustavil and colleagues demonstrated a pivotal role of the decidua in the production of prostaglandin F 2 α upon the instillation of hypertonic saline into the intraamniotic cavity for termination of pregnancy.
Term and preterm labors are associated with increased expression of proinflammatory cytokines and chemokines at the fetal-maternal interface that summon the infiltration of leukocytes into the decidual microenvironment.
Human labor is an inflammatory process, although debate persists over whether term and preterm parturitions are similar or different biological entities. Inflammation in the setting of parturition occur systemically and locally. High-dimensional profiling indicates that molecular signatures accompanying labor differ, depending on clinical context.
Choriodecidual chemokine expression patterns differ in idiopathic (ie, noninfectious etiology) compared with PTB in the presence of histologically verified chorioamnionitis. Such results underscore previous findings and foreshadow the evolving concept that PTB is a complex syndrome with distinct phenotypes and underlying pathogenesis that can best be appreciated by considering them in separate contexts.
Cataloging by gene expression profiling has been the mainstay of molecular methods in research efforts into PTB. With the advent of sophisticated bioinformatics tools, it is now possible to use systems biology approaches to study gene networks in complex, physiologically relevant contexts (ie, gene regulatory network [GRN] analysis). GRN analysis is a computational method that identifies relationships among genes up-regulated or down-regulated in response to a given stimulus.
Noncoding ribonucleic acids (RNAs), including microRNAs (miRNAs), provide an important level of molecular fine-tuning of biological and pathobiological processes. These miRNAs are small noncoding RNAs (∼ 22 nucleotides in length) that, in general, dampen gene expression. In addition, some miRNAs target mRNAs whose gene products exert inhibitory activity, and in this case, these miRNAs may actually enhance expression of small subsets of genes. More than 2500 unique mature human miRNAs have been identified, each with the ability to regulate the expression of potentially hundreds of genes.
The miRNAs represent one of the most abundant and conserved classes of regulatory molecules that influence global gene regulation. Mature miRNAs (generated following the posttranscriptional processing of precursor miRNAs) block protein translation or promote mRNA degradation by imperfect Watson-Crick base pairing. The miRNAs arise from larger transcripts located within structural genes (intragenic miRNAs) or from independently transcribed units (intergenic miRNAs) that are processed from primary transcripts into functional miRNAs.
A few miRNA profiling studies have used clinical samples in the context of parturition to report on which miRNAs are expressed in gestational tissues in the setting of normal or infection-mediated PTB. However, the relationship between miRNAs and the mRNAs they regulate is ill defined and has not been studied in depth in the context of preterm labor. It is crucial that we bridge the gap in our understanding of the role of miRNAs in inflammatory gene regulation to more effectively identify new biomarker and/or therapeutic strategies to combat preterm parturition.
We conducted a profiling investigation of miRNAs and their cognate mRNA targets in human decidual stromal cells to construct gene regulatory networks to begin defining the inflammatory pathways involved in preterm labor. We also considered these mRNA-miRNA relationships in the context of the transcription factors (TFs) that govern both gene expression programs to build a framework for future hypothesis testing by clinical and laboratory experimentation. These studies have important implications for translating gene expression signatures into a more coherent molecular picture of inflammation-mediated pathways involved in term and preterm parturition.
Materials and Methods
Study design
The overarching design of the present study was the evaluation of putative inflammatory gene targets by miRNAs expressed in decidual cells in response to cytokine challenge. In addition, we sought to determine whether progesterone could reverse the proinflammatory effects of cytokines and mRNA and miRNA gene expression.
Decidual stromal cell culture and treatments
All tissues were obtained following written informed consent, and the study was approved by the Institutional Review Boards (IRB) at Yale University and The Ohio State University. Cells were initially isolated at Yale under IRB approval and then transferred to Ohio State, requiring additional local IRB approval.
After written IRB approval and informed consent, fibroblasts were prepared from the decidua parietalis of reflected fetal membranes obtained from 3 uncomplicated pregnancies after elective repeat cesarean delivery as previously described. All specimens were obtained prior to the onset of labor. At confluence, decidual cells were primed for 13 days in medium supplemented with 10 –8 M 17β-estradiol (Sigma-Aldrich, St Louis, MO) and 10 –7 M medroxyprogesterone acetate (Sigma-Aldrich) to stimulate in vitro decidualization (see Supplemental Methods for further details).
On day 1, cultures were treated in a defined medium with PBS containing 0.1% bovine serum albumin (vehicle control) or with 1 ng/mL of human recombinant interleukin-1β (IL-1β; R&D Systems, Minneapolis, MN) for 6 hours. IL-1β is a cytokine shown previously to be produced in decidual cells. Cells from 3 different patients were used for the studies.
RNA isolation and quantitative real-time–reverse transcriptase analysis
Total RNA was extracted with TRIzol (Invitrogen, Carlsbad, CA), and after chloroform and centrifugation, the aqueous phase was precipitated overnight at –20°C with an equal volume of 70% ethanol and then applied to a miRNeasy spin column (QIAGEN, Valencia, CA) and processed according to the manufacturer’s protocol. This included on-column deoxyribonuclease digestion using the RNase-Free deoxyribonuclease kit (QIAGEN) to remove contaminating genomic DNA. RNA was quantified by absorbance at 260/280 nm using a NanoDrop spectrophotometer (Thermo Scientific, Hudson, NH). Methodological details of the quantitative real time–polymerase chain reaction (qRT-PCR) studies can be found in the Supplemental Methods.
For all reactions, fold changes were calculated using the comparative cycle threshold method described by Schmittgen and Livak using large ribosomal protein (RPLP0; Applied Biosystems, Foster City, CA) as the internal control gene run in parallel assays. Statistical analysis was performed using the Mann-Whitney statistical test, after determining that gene amplification data did not exhibit a Gaussian distribution.
Microarray profiling and data analysis
Prior to processing, the quality and purity of total RNA were evaluated using the Agilent 2100 Bioanalyzer RNA 6000 Nano Kit and on the ND1000 NanoDrop platform (Agilent Technologies, Palo Alto, CA). Total RNA (250 ng per sample) was processed using the Ambion WT expression kit (Ambion, Austin, TX) and labeled with the Affymetrix GeneChip whole transcript sense target labeling assay (Affymetrix, Santa Clara, CA), followed by hybridization to the Affymetrix Human Gene 2.0 ST array according to the manufacturers’ protocols. Hybridized arrays were scanned on an Affymetrix GeneChip Scanner 3000 7G and analyzed using Affymetrix software. Details of the data processing and analysis for gene expression data may be found in the Supplemental Methods section. These data have been deposited in the National Center for Biotechnology Information’s Gene Expression Omnibus.
Mature miRNA profiling
Multiplexed miRNA expression was profiled using the nCounter platform (Human version 2 miRNA expression assay; NanoString Technologies, Seattle, WA). The nCounter system provides a means to capture and count individual mRNAs of any kind (ie, coding and noncoding RNAs). It is a direct method that does not require enzymatic amplification and is ideally suited for low abundance transcripts.
For each reaction, total RNA (100 ng) was used as input for the nCounter miRNA sample preparation reactions according to the manufacturer’s instructions. Differential expression analysis of the resulting counts data was performed using the edgeR (version 3.4.0) Bioconductor package. Complete details of the reactions and analytical methods are found in Supplemental Methods.
The pheatmap (version 0.7.7) R package was used for hierarchical cluster analysis using Euclidean distance and complete linkage and for heat map generation. Following the identification of mature miRNAs by NanoString profiling, we confirmed 10 of the most highly up-regulated miRs by qRT-PCR using biological replicates from a separate set of decidual cell cultures (see Supplemental Materials ).
Bioinformatics analysis
Details of the methods used for mature miRNA target prediction, transcriptional factor binding motif (TFBM) enrichment analysis, pathway enrichment analysis, and prediction of TF activation based on global expression patterns are provided in the Supplemental Methods.
Results
Differential expression of inflammatory genes in response to cytokine and progesterone
Microarray profiling of cultured decidual cells challenged with IL-1β for 6 hours revealed an up-regulation of 448 and a down-regulation of 116 transcript clusters (350 and 78 nonredundant transcripts, respectively) ( Figure 1 A and Supplemental Table 1 ).

Among highly up-regulated transcripts were numerous proinflammatory cytokines (eg, IL-1A, IL-6, and tumor necrosis factor); leukoattractants (including 8 chemokine ligand chemokines and 6 cysteine-cysteine chemokines); interferon-inducible genes (eg, MX1 , GBP1 , OAS2 , and IFI44 ); inducible prostaglandin-synthesizing enzymes (eg, prostaglandin-endoperoxide synthase 2 and prostaglandin E synthase); and matrix metalloproteinases (e.g., MMP1, MMP3, and MMP12). The up-regulation of 8 of these transcripts was confirmed by qRT-PCR ( Supplemental Figure 1 ).
The IL-1β–induced transcripts were enriched for several Kyoto Encyclopedia of Genes and Genomes pathways, including cytokine-cytokine receptor interaction, apoptosis, and Janus kinase–signal transducer and activator of transcription (STAT) signaling, whereas down-regulated transcripts were enriched for pathways relating to Wnt signaling and actin cytoskeleton dynamics ( Table 1 ).
Group | KEGG accession number | KEGG pathway name | Pathway description | Adjusted P value | Genes |
---|---|---|---|---|---|
Up-regulated transcripts | hsa04060 | Cytokine-cytokine receptor interaction | Pertains to intercellular signaling proteins/glycoproteins serving as critical regulators of host defense, cell growth and differentiation, cell death, angiogenesis, development, and homeostatic processes | < .0001 | CD40 , LIF , IL23A , HGF , TNFRSF11B , INHBA , CXCL1 , CSF3 , CSF2 , CXCL5 , CXCL3 , CSF1 , CXCL2 , CXCL6 , IL7R , CCL20 , IL6 , IL8 , CCL11 , TNFSF15 , IL15 , CXCL11 , TNFSF18 , IL17RB , CXCL10 , IL11 , IL15RA , IL1B , IL1A , CCL1 , CCL2 , CCL8 , CX3CL1 , CCL5 , TNFSF10 , IFNGR2 , IFNAR2 , TNFRSF9 , TNFSF13B , TNF , TNFRSF1B , TSLP |
hsa04210 | Apoptosis | Refers to the process of controlled cell death triggered by intrinsic and extrinsic signaling events. | .0001 | NFKB1 , NFKB2 , IL1B , IL1A , IRAK2 , PIK3CD , TNF , NFKBIA , IRAK3 , PPP3CC , CFLAR , BIRC3 , BIRC2, TNFSF10 | |
hsa04620 | Toll-like receptor signaling pathway | Signaling pathway involved in the recognition of and cellular response to pathogen-associated molecular patterns | .0006 | CXCL11 , CXCL10 , MAP3K8 , IL1B , PIK3CD , CD40 , NFKBIA , CCL5 , NFKB1 , NFKB2 , IFNAR2 , TNF , IL6 , IL8 | |
hsa04630 | JAK-STAT signaling pathway | Signaling cascade important for the cellular response to a wide variety of cytokines and growth factors | .0007 | STAT5A , IL15 , IL11 , IL15RA , SOCS3 , PIK3CD , LIF , IL23A , IFNGR2 , IL13RA2 , IFNAR2 , CSF3 , CSF2 , IL7R , STAT4 , IL6 , TSLP | |
hsa05222 | Small-cell lung cancer | Includes molecular mechanisms often deregulated in small-cell lung cancers | .0014 | PTGS2 , NFKB1 , NFKB2 , PIK3CD , CDK6 , LAMC2 , TRAF1 , NFKBIA , LAMB3 , BIRC3 , BIRC2 , LAMA3 | |
hsa04660 | T-cell receptor signaling pathway | Signaling cascades involved in T-lymphocyte proliferation/ differentiation and cytokine production | .0081 | NFKB1 , NFKB2 , MAP3K8 , PIK3CD , CSF2 , TNF , NFKBIE , NFKBIA , PPP3CC , NFATC2 , NFATC1 | |
hsa04920 | Adipocytokine signaling pathway | Signaling pathways important in adipocyte signaling, lipid homeostasis, and inflammation-associated insulin resistance | .0144 | NFKB1 , NFKB2 , SOCS3 , TNF , NFKBIE , NFKBIA , TNFRSF1B , ACSL4 , ACSL5 | |
hsa04640 | Hematopoietic cell lineage | Signaling pathways regulating the differentiation and development of leukocyte, erythrocyte, and megakaryocyte cell lineages | .0144 | IL11 , IL1B , IL1A , CSF3 , CSF2, TNF , CSF1 , IL7R , IL6, TFRC | |
hsa04662 | B cell receptor signaling pathway | Signaling events contributing to B-lymphocyte proliferation/ differentiation and antibody production | .0230 | NFKB1 , NFKB2 , PIK3CD , NFKBIE , NFKBIA , PPP3CC , NFATC2 , NFATC1 | |
Down-regulated transcripts | hsa05210 | Colorectal cancer | Includes molecular mechanisms commonly deregulated in colorectal cancers | .0093 | PIK3R1 , FZD2 , FZD7 |
hsa04310 | Wnt signaling pathway | Refers to pathways mediating cellular responses to Wnt-protein morphogens (ligands of the Frizzled family receptors), including transcription of Wnt target genes, cell shape plasticity, and control of intracellular calcium levels | .0065 | PRICKLE1 , FZD2 , FZD7 , DKK1 | |
hsa04810 | Regulation of actin cytoskeleton | Includes general pathways involved in the dynamic control of the actin cytoskeleton as it contributes to cell polarity and motility | .0213 | FGF9 , TMSB4X , CHRM2 , PIK3R1 | |
hsa04080 | Neuroactive ligand-receptor interaction | Generally refers to interactions between soluble ligands and cellular receptors present in (but not limited to) neuronal tissues | .0378 | SSTR1 , HTR2B , ADRA2A , CHRM2 | |
hsa05217 | Basal cell carcinoma | Includes molecular mechanisms deregulated in basal cell carcinomas | .0340 | FZD2 , FZD7 |
Ingenuity Pathway Analysis Upstream Regulator analysis predicted the activation of 57 and inhibition of 22 TFs ( Supplemental Table 2 and Supplemental Figure 2 ) in this setting. Consistent with proinflammatory signaling, activation was inferred for the nuclear factor-κB (NF-κB) complex, interferon regulatory factors, activator protein 1 subunits, and STAT complexes, among others.
A complementary analysis revealed an overrepresentation of TFBMs corresponding to several TFs predicted to be activated (including response elements for NF-κB, interferon regulatory factors 1/2, STAT1/3, activator protein 1) among the promoters of IL-1β–induced genes ( Supplemental Table 3 ). In contrast, overrepresented TFBMs within the promoters of down-regulated genes did not correspond with the TFs predicted to be inhibited.
Expression of miRNAs in response to cytokine challenge and progesterone
Within the microarray data set, transcript clusters associated with 15 miRNAs and 4 long, noncoding RNAs (lncRNAs) were up-regulated by at least ±1.5-fold (false discovery rate < 0.1), whereas those corresponding to 9 miRNAs and 3 lncRNAs were down-regulated ( Figure 1 B and Table 2 ).
Transcript cluster identification | Gene symbol(s) | Accession number(s) a | Description | Linear fold change | ANOVA P value | FDR |
---|---|---|---|---|---|---|
16800630 | MIR147B | MI0005544 | MiRNA 147b | 10.86 | < .001 | 0.006 |
16991760 | MIR3142 | MI0014166 | MiRNA 3142 | 9.74 | < .001 | 0.016 |
16991757 | MIR146A | MI0000477 | MiRNA 146a | 8.41 | < .001 | 0.004 |
16921827 | MIR155 | MI0000681 | MiRNA 155, MIR155 host gene (nonprotein coding) | 5.13 | < .001 | 0.019 |
16772285 | LINC00944 | Long intergenic nonprotein coding RNA 944 | 4.04 | .001 | 0.042 | |
16685359 | LINC01137 | Long intergenic nonprotein coding RNA 473 | 3.23 | < .001 | 0.017 | |
17025564 | LINC00473 | Long intergenic nonprotein coding RNA 1137 | 2.87 | < .001 | 0.014 | |
16659238 | MIR4632 | MI0017259 | MiRNA 4632 | 2.34 | .001 | 0.033 |
16788780 | MIR154 | MI0000480 | MiRNA 154 | 1.92 | .006 | 0.094 |
16821396 | MIR3182 | MI0014224 | MiRNA 3182 | 1.72 | < .001 | 0.010 |
16713309 | MIR4683 | MI0017315 | MiRNA 4683 | 1.70 | < .001 | 0.003 |
16788749 | MIR1185-1 | MI0003844 | MiRNA 1185-1 | 1.67 | .006 | 0.089 |
16914859 | MIR645 | MI0003660 | MiRNA 645 | 1.66 | .005 | 0.080 |
16937152 | LINC00312 | Long intergenic nonprotein coding RNA 312 | 1.64 | .004 | 0.069 | |
16788762 | MIR889 | MI0005540 | MiRNA 889 | 1.63 | < .001 | 0.026 |
16846915 | MIR3614 | MI0016004 | MiRNA 3614 | 1.57 | < .001 | 0.015 |
16788715 | MIR299 | MI0000744 | MiRNA 299 | 1.56 | .001 | 0.027 |
16864393 | MIR4751 | MI0017390 | MiRNA 4751 | 1.52 | < .001 | 0.020 |
16905434 | MIR1246 | MI0006381 | MiRNA 1246 | 1.50 | .003 | 0.060 |
16709201 | MIR4680 | MI0017312 | MiRNA 4680 | –1.52 | .001 | 0.040 |
17114970 | MIR452, MIR224 | MI0001733, MI0000301 | MiRNA 452, miRNA 224 | –1.59 | .001 | 0.032 |
16775763 | MIR622 | MI0003636 | MiRNA 622 | –1.59 | < .001 | 0.025 |
17056160 | MIR196B | MI0001150 | MiRNA 196b | –1.72 | < .001 | 0.011 |
16660497 | LINC00339 | Long intergenic nonprotein coding RNA 339 | –1.73 | .002 | 0.053 | |
16921627 | LINC00478 | Long intergenic nonprotein coding RNA 478 | –2.09 | .007 | 0.098 | |
16990949 | MIR143, MIR145 | MI0000459, MI0000461 | MiRNA 143, miRNA 145, MIR143 host gene (nonprotein coding) | –2.14 | .005 | 0.083 |
17114326 | MIR424 | MI0001446 | MiRNA 424 | –2.20 | < .001 | 0.022 |
17114334 | MIR503 | MI0003188 | MiRNA 503 | –2.47 | .001 | 0.028 |
16965142 | LINC01085 | Long intergenic nonprotein coding RNA 1085 | –2.80 | < .001 | 0.017 |
a Refers to miRBase ( http://www.mirbase.org/ ).
To determine whether changes in miRNA transcript expression also affected mature miRNA abundance, we surveyed mature miRNA expression using the NanoString platform. Based on the absolute value of the expression data, miR-21, miR-143, miR-145, and miR-4454 as well as several members of the let-7, miR-10, miR-15, miR-29, and miR-125 families were among the top 50 most highly expressed mature miRNAs in untreated term decidual cells ( Supplemental Table 4 ). Half of these miRNAs were previously reported as being abundant in human endometrial stromal cells decidualized in vitro, whereas members of the miR-181, miR-183, and miR-200 families, which undergo down-regulation following decidualization of endometrial stromal cells, were among the least abundant miRNAs (data not shown).
Following an IL-1β challenge, a signature of 6 mature miRNAs differentiated unstimulated from stimulated decidual cells ( Figure 1 C and Table 3 ). Only 3 mature miRNAs (miR-143-3p, miR-145-5p, and miR-146a-5p) had corresponding differentially expressed primary/precursor miRNA transcript clusters by microarray, and in each instance, the mature form changed less than the associated primary/precursor transcript. Thus, in pairs of miRNAs represented on both platforms, we observed poor correlation (Pearson’s r = 0.189, Spearman’s ρ = 0.028) between the fold changes estimated using NanoString compared with those estimated by microarray ( Figure 1 D).
Mature miRNA | Accession number a | Linear fold change | P value | FDR |
---|---|---|---|---|
miR-146a-5p | MIMAT0000449 | 4.71 | < .001 | < 0.001 |
miR-525-5p | MIMAT0002838 | 1.74 | < .001 | < 0.001 |
miR-143-3p | MIMAT0000435 | –1.15 | < .001 | < 0.001 |
miR-145-5p | MIMAT0000437 | –1.16 | < .001 | < 0.010 |
miR-924 | MIMAT0004974 | –1.73 | < .001 | 0.015 |
miR-4454 | MIMAT0018976 | –1.96 | < .001 | 0.097 |
a Refers to miRBase ( http://www.mirbase.org/ ).
To confirm the miRNA profiling studies, we performed qRT-PCR assays using probe sets designed to detect either the primary or mature forms of 5 miRNAs ( Figure 2 ). Both intergenic miRNAs that are processed from primary transcripts of the MIR143/145 transcript cluster were down-regulated by ∼ 2.5-fold following cytokine treatment ( Figure 2 , A and B), but neither of the corresponding mature miRNAs were differentially expressed by qRT-PCR ( Figure 2 , F and G). Both the primary and mature forms of miR-146a and miR-155 were up-regulated by qRT-PCR following IL-1β exposure ( Figure 2 , C and H), whereas no significant changes in the primary and mature forms of miR-4454 were detected ( Figure 2 , E and J).

Analysis of gene expression networks in response to cytokine and progesterone
To better understand the pathways potentially dysregulated in response to altered mature miRNA expression, we queried the DIANA miRPath server using miR-146a, miR-155, miR-525, and miR-924 as inputs. These 4 miRNAs were predicted to influence multiple signaling pathways ( Table 4 ), including the Toll-like receptor and mitogen-activated protein kinase pathways. A more extensive analysis revealed 1697 known/predicted targets for these miRNAs (∼ 241 targets per miRNA).
KEGG accession number | KEGG pathway name | Pathway description | P value | Targeted genes |
---|---|---|---|---|
hsa04620 | Toll-like receptor signaling pathway | Signaling pathway involved in the recognition of and cellular response to pathogen-associated molecular patterns. | < 0.0001 | CCL5, CD80, FOS, IFNA6, IFNA7, IKBKE, IRAK1, IRF5, MAPK10, PIK3CA, PIK3R1, RELA, TAB2, TBK1, TLR6, TLR7, TRAF3, TRAF6 |
hsa04666 | Fc gamma R-mediated phagocytosis | Refers to components of the phagocytic pathway activated upon binding of antibodies to Fc receptors; this often occurs following opsonization of foreign extracellular materials as a host-defense mechanism. | < 0.0001 | AMPH, ARF6, ARPC5, CDC42, DOCK2, HCK, INPP5D, MYO10, PIK3CA, PIK3R1, PIP5K1B, PLA2G4F, PLD2, PRKCE, RPS6KB1, VAV3 |
hsa04722 | Neurotrophin signaling pathway | Pertains to signaling pathways activated by a family of trophic factors involved in differentiation and survival of neural cells. | < 0.0001 | BAX, BDNF, BDNF, CAMK2D, CDC42, FASLG, GSK3B, IRAK1, KRAS, MAPK10, NRAS, NTRK3, NTRK3, PIK3CA, PIK3R1, RAP1B, RELA, RPS6KA3, SORT1, SOS1, TRAF6 |
hsa04012 | ErbB signaling pathway | Signaling pathways activated through ErbB receptor tyrosine kinases, which regulate diverse cellular responses including proliferation, differentiation, motility, and survival. | < 0.0001 | ABL2, CAMK2D, ERBB2, ERBB4, GSK3B, KRAS, MAPK10, NRAS, PAK2, PAK7, PIK3CA, PIK3R1, RPS6KB1, SOS1 |
hsa05213 | Endometrial cancer | Includes pathways implicated in type-I and type-II endometrial carcinomas. | < 0.0001 | APC, CTNNA3, ERBB2, GSK3B, ILK, KRAS, NRAS, PIK3CA, PIK3R1, SOS1, TCF4 |
hsa04010 | MAPK signaling pathway | Refers to processes regulating the mitogen-activated protein kinase (MAPK) cascade, which directs various cellular functions including proliferation, differentiation, migration, and inflammation. | < 0.0001 | BDNF, CACNB3, CACNB4, CDC42, DUSP14, FASLG, FGF7, FGF9, FOS, HSPA1A, IL1A, KRAS, MAP3K13, MAP3K2, MAP4K3, MAPK10, NRAS, PAK2, PDGFRA, PLA2G4F, PTPRQ, RAP1B, RAPGEF2, RASA2, RASGRP4, RELA, RPS6KA3, RPS6KA4, SOS1, TAB2, TAOK1, TRAF6 |
hsa05200 | Pathways in cancer | Refers to multiple signaling cascades de-regulated in human cancers including those promoting proliferation, tissue invasion, metastasis, angiogenesis, evasion of apoptosis, and resistance to anti-growth signals. | 0.0001 | APC, ARNT, BAX, CDC42, COL4A3, CSF1R, CTNNA3, DAPK1, E2F2, ERBB2, ETS1, FASLG, FGF7, FGF9, FOS, FZD4, FZD5, GSK3B, HHIP, IGF1R, KITLG, KRAS, MAPK10, NRAS, PDGFRA, PGF, PIAS2, PIK3CA, PIK3R1, PTCH1, RARB, RB1, RELA, SKP2, SMAD4, SOS1, SUFU, TCEB1, TCF4, TRAF3, TRAF6 |
hsa00900 | Terpenoid backbone biosynthesis | Refers to metabolic pathways involved in the synthesis of terpenoids (isoprenoids), which give rise to steroid, sterol, and carotenoid precursor molecules; the HMG-CoA reductase pathway is an example. | 0.0001 | ACAT1, FNTA, HMGCS1, PCYOX1, PDSS2, RCE1 |
hsa05211 | Renal cell carcinoma | Includes pathways commonly de-regulated in renal cell carcinomas. | 0.0002 | ARNT, CDC42, ETS1, KRAS, NRAS, PAK2, PAK7, PGF, PIK3CA, PIK3R1, RAP1B, SOS1, TCEB1 |
hsa05160 | Hepatitis C | Comprises several host cell immunity pathways that are subverted during hepatitis C virus infection. | 0.0002 | DDX58, GSK3B, IFNA6, IFNA7, IKBKE, KRAS, MAPK10, NRAS, PIK3CA, PIK3R1, PPP2CA, PPP2R1A, RELA, SCARB1, SOS1, TBK1, TRAF3, TRAF6 |
Because the destabilization of target mRNAs is thought to be a major mechanism through which miRNAs reduce target protein expression, we compared these target predictions with our microarray expression data. Interestingly, only 38 of the known or predicted mRNA targets (2.2%) exhibited differential expression by microarray, and of these, only 32% exhibited anticorrelated expression with their corresponding miRNAs. Network diagrams of experimentally validated miRNA-mRNA interactions, in addition to predicted TF-mRNA interactions, are shown in Figure 3 .

Results
Differential expression of inflammatory genes in response to cytokine and progesterone
Microarray profiling of cultured decidual cells challenged with IL-1β for 6 hours revealed an up-regulation of 448 and a down-regulation of 116 transcript clusters (350 and 78 nonredundant transcripts, respectively) ( Figure 1 A and Supplemental Table 1 ).

Among highly up-regulated transcripts were numerous proinflammatory cytokines (eg, IL-1A, IL-6, and tumor necrosis factor); leukoattractants (including 8 chemokine ligand chemokines and 6 cysteine-cysteine chemokines); interferon-inducible genes (eg, MX1 , GBP1 , OAS2 , and IFI44 ); inducible prostaglandin-synthesizing enzymes (eg, prostaglandin-endoperoxide synthase 2 and prostaglandin E synthase); and matrix metalloproteinases (e.g., MMP1, MMP3, and MMP12). The up-regulation of 8 of these transcripts was confirmed by qRT-PCR ( Supplemental Figure 1 ).
The IL-1β–induced transcripts were enriched for several Kyoto Encyclopedia of Genes and Genomes pathways, including cytokine-cytokine receptor interaction, apoptosis, and Janus kinase–signal transducer and activator of transcription (STAT) signaling, whereas down-regulated transcripts were enriched for pathways relating to Wnt signaling and actin cytoskeleton dynamics ( Table 1 ).
Group | KEGG accession number | KEGG pathway name | Pathway description | Adjusted P value | Genes |
---|---|---|---|---|---|
Up-regulated transcripts | hsa04060 | Cytokine-cytokine receptor interaction | Pertains to intercellular signaling proteins/glycoproteins serving as critical regulators of host defense, cell growth and differentiation, cell death, angiogenesis, development, and homeostatic processes | < .0001 | CD40 , LIF , IL23A , HGF , TNFRSF11B , INHBA , CXCL1 , CSF3 , CSF2 , CXCL5 , CXCL3 , CSF1 , CXCL2 , CXCL6 , IL7R , CCL20 , IL6 , IL8 , CCL11 , TNFSF15 , IL15 , CXCL11 , TNFSF18 , IL17RB , CXCL10 , IL11 , IL15RA , IL1B , IL1A , CCL1 , CCL2 , CCL8 , CX3CL1 , CCL5 , TNFSF10 , IFNGR2 , IFNAR2 , TNFRSF9 , TNFSF13B , TNF , TNFRSF1B , TSLP |
hsa04210 | Apoptosis | Refers to the process of controlled cell death triggered by intrinsic and extrinsic signaling events. | .0001 | NFKB1 , NFKB2 , IL1B , IL1A , IRAK2 , PIK3CD , TNF , NFKBIA , IRAK3 , PPP3CC , CFLAR , BIRC3 , BIRC2, TNFSF10 | |
hsa04620 | Toll-like receptor signaling pathway | Signaling pathway involved in the recognition of and cellular response to pathogen-associated molecular patterns | .0006 | CXCL11 , CXCL10 , MAP3K8 , IL1B , PIK3CD , CD40 , NFKBIA , CCL5 , NFKB1 , NFKB2 , IFNAR2 , TNF , IL6 , IL8 | |
hsa04630 | JAK-STAT signaling pathway | Signaling cascade important for the cellular response to a wide variety of cytokines and growth factors | .0007 | STAT5A , IL15 , IL11 , IL15RA , SOCS3 , PIK3CD , LIF , IL23A , IFNGR2 , IL13RA2 , IFNAR2 , CSF3 , CSF2 , IL7R , STAT4 , IL6 , TSLP | |
hsa05222 | Small-cell lung cancer | Includes molecular mechanisms often deregulated in small-cell lung cancers | .0014 | PTGS2 , NFKB1 , NFKB2 , PIK3CD , CDK6 , LAMC2 , TRAF1 , NFKBIA , LAMB3 , BIRC3 , BIRC2 , LAMA3 | |
hsa04660 | T-cell receptor signaling pathway | Signaling cascades involved in T-lymphocyte proliferation/ differentiation and cytokine production | .0081 | NFKB1 , NFKB2 , MAP3K8 , PIK3CD , CSF2 , TNF , NFKBIE , NFKBIA , PPP3CC , NFATC2 , NFATC1 | |
hsa04920 | Adipocytokine signaling pathway | Signaling pathways important in adipocyte signaling, lipid homeostasis, and inflammation-associated insulin resistance | .0144 | NFKB1 , NFKB2 , SOCS3 , TNF , NFKBIE , NFKBIA , TNFRSF1B , ACSL4 , ACSL5 | |
hsa04640 | Hematopoietic cell lineage | Signaling pathways regulating the differentiation and development of leukocyte, erythrocyte, and megakaryocyte cell lineages | .0144 | IL11 , IL1B , IL1A , CSF3 , CSF2, TNF , CSF1 , IL7R , IL6, TFRC | |
hsa04662 | B cell receptor signaling pathway | Signaling events contributing to B-lymphocyte proliferation/ differentiation and antibody production | .0230 | NFKB1 , NFKB2 , PIK3CD , NFKBIE , NFKBIA , PPP3CC , NFATC2 , NFATC1 | |
Down-regulated transcripts | hsa05210 | Colorectal cancer | Includes molecular mechanisms commonly deregulated in colorectal cancers | .0093 | PIK3R1 , FZD2 , FZD7 |
hsa04310 | Wnt signaling pathway | Refers to pathways mediating cellular responses to Wnt-protein morphogens (ligands of the Frizzled family receptors), including transcription of Wnt target genes, cell shape plasticity, and control of intracellular calcium levels | .0065 | PRICKLE1 , FZD2 , FZD7 , DKK1 | |
hsa04810 | Regulation of actin cytoskeleton | Includes general pathways involved in the dynamic control of the actin cytoskeleton as it contributes to cell polarity and motility | .0213 | FGF9 , TMSB4X , CHRM2 , PIK3R1 | |
hsa04080 | Neuroactive ligand-receptor interaction | Generally refers to interactions between soluble ligands and cellular receptors present in (but not limited to) neuronal tissues | .0378 | SSTR1 , HTR2B , ADRA2A , CHRM2 | |
hsa05217 | Basal cell carcinoma | Includes molecular mechanisms deregulated in basal cell carcinomas | .0340 | FZD2 , FZD7 |
Ingenuity Pathway Analysis Upstream Regulator analysis predicted the activation of 57 and inhibition of 22 TFs ( Supplemental Table 2 and Supplemental Figure 2 ) in this setting. Consistent with proinflammatory signaling, activation was inferred for the nuclear factor-κB (NF-κB) complex, interferon regulatory factors, activator protein 1 subunits, and STAT complexes, among others.
A complementary analysis revealed an overrepresentation of TFBMs corresponding to several TFs predicted to be activated (including response elements for NF-κB, interferon regulatory factors 1/2, STAT1/3, activator protein 1) among the promoters of IL-1β–induced genes ( Supplemental Table 3 ). In contrast, overrepresented TFBMs within the promoters of down-regulated genes did not correspond with the TFs predicted to be inhibited.
Expression of miRNAs in response to cytokine challenge and progesterone
Within the microarray data set, transcript clusters associated with 15 miRNAs and 4 long, noncoding RNAs (lncRNAs) were up-regulated by at least ±1.5-fold (false discovery rate < 0.1), whereas those corresponding to 9 miRNAs and 3 lncRNAs were down-regulated ( Figure 1 B and Table 2 ).
Transcript cluster identification | Gene symbol(s) | Accession number(s) a | Description | Linear fold change | ANOVA P value | FDR |
---|---|---|---|---|---|---|
16800630 | MIR147B | MI0005544 | MiRNA 147b | 10.86 | < .001 | 0.006 |
16991760 | MIR3142 | MI0014166 | MiRNA 3142 | 9.74 | < .001 | 0.016 |
16991757 | MIR146A | MI0000477 | MiRNA 146a | 8.41 | < .001 | 0.004 |
16921827 | MIR155 | MI0000681 | MiRNA 155, MIR155 host gene (nonprotein coding) | 5.13 | < .001 | 0.019 |
16772285 | LINC00944 | Long intergenic nonprotein coding RNA 944 | 4.04 | .001 | 0.042 | |
16685359 | LINC01137 | Long intergenic nonprotein coding RNA 473 | 3.23 | < .001 | 0.017 | |
17025564 | LINC00473 | Long intergenic nonprotein coding RNA 1137 | 2.87 | < .001 | 0.014 | |
16659238 | MIR4632 | MI0017259 | MiRNA 4632 | 2.34 | .001 | 0.033 |
16788780 | MIR154 | MI0000480 | MiRNA 154 | 1.92 | .006 | 0.094 |
16821396 | MIR3182 | MI0014224 | MiRNA 3182 | 1.72 | < .001 | 0.010 |
16713309 | MIR4683 | MI0017315 | MiRNA 4683 | 1.70 | < .001 | 0.003 |
16788749 | MIR1185-1 | MI0003844 | MiRNA 1185-1 | 1.67 | .006 | 0.089 |
16914859 | MIR645 | MI0003660 | MiRNA 645 | 1.66 | .005 | 0.080 |
16937152 | LINC00312 | Long intergenic nonprotein coding RNA 312 | 1.64 | .004 | 0.069 | |
16788762 | MIR889 | MI0005540 | MiRNA 889 | 1.63 | < .001 | 0.026 |
16846915 | MIR3614 | MI0016004 | MiRNA 3614 | 1.57 | < .001 | 0.015 |
16788715 | MIR299 | MI0000744 | MiRNA 299 | 1.56 | .001 | 0.027 |
16864393 | MIR4751 | MI0017390 | MiRNA 4751 | 1.52 | < .001 | 0.020 |
16905434 | MIR1246 | MI0006381 | MiRNA 1246 | 1.50 | .003 | 0.060 |
16709201 | MIR4680 | MI0017312 | MiRNA 4680 | –1.52 | .001 | 0.040 |
17114970 | MIR452, MIR224 | MI0001733, MI0000301 | MiRNA 452, miRNA 224 | –1.59 | .001 | 0.032 |
16775763 | MIR622 | MI0003636 | MiRNA 622 | –1.59 | < .001 | 0.025 |
17056160 | MIR196B | MI0001150 | MiRNA 196b | –1.72 | < .001 | 0.011 |
16660497 | LINC00339 | Long intergenic nonprotein coding RNA 339 | –1.73 | .002 | 0.053 | |
16921627 | LINC00478 | Long intergenic nonprotein coding RNA 478 | –2.09 | .007 | 0.098 | |
16990949 | MIR143, MIR145 | MI0000459, MI0000461 | MiRNA 143, miRNA 145, MIR143 host gene (nonprotein coding) | –2.14 | .005 | 0.083 |
17114326 | MIR424 | MI0001446 | MiRNA 424 | –2.20 | < .001 | 0.022 |
17114334 | MIR503 | MI0003188 | MiRNA 503 | –2.47 | .001 | 0.028 |
16965142 | LINC01085 | Long intergenic nonprotein coding RNA 1085 | –2.80 | < .001 | 0.017 |
a Refers to miRBase ( http://www.mirbase.org/ ).
To determine whether changes in miRNA transcript expression also affected mature miRNA abundance, we surveyed mature miRNA expression using the NanoString platform. Based on the absolute value of the expression data, miR-21, miR-143, miR-145, and miR-4454 as well as several members of the let-7, miR-10, miR-15, miR-29, and miR-125 families were among the top 50 most highly expressed mature miRNAs in untreated term decidual cells ( Supplemental Table 4 ). Half of these miRNAs were previously reported as being abundant in human endometrial stromal cells decidualized in vitro, whereas members of the miR-181, miR-183, and miR-200 families, which undergo down-regulation following decidualization of endometrial stromal cells, were among the least abundant miRNAs (data not shown).
Following an IL-1β challenge, a signature of 6 mature miRNAs differentiated unstimulated from stimulated decidual cells ( Figure 1 C and Table 3 ). Only 3 mature miRNAs (miR-143-3p, miR-145-5p, and miR-146a-5p) had corresponding differentially expressed primary/precursor miRNA transcript clusters by microarray, and in each instance, the mature form changed less than the associated primary/precursor transcript. Thus, in pairs of miRNAs represented on both platforms, we observed poor correlation (Pearson’s r = 0.189, Spearman’s ρ = 0.028) between the fold changes estimated using NanoString compared with those estimated by microarray ( Figure 1 D).
Mature miRNA | Accession number a | Linear fold change | P value | FDR |
---|---|---|---|---|
miR-146a-5p | MIMAT0000449 | 4.71 | < .001 | < 0.001 |
miR-525-5p | MIMAT0002838 | 1.74 | < .001 | < 0.001 |
miR-143-3p | MIMAT0000435 | –1.15 | < .001 | < 0.001 |
miR-145-5p | MIMAT0000437 | –1.16 | < .001 | < 0.010 |
miR-924 | MIMAT0004974 | –1.73 | < .001 | 0.015 |
miR-4454 | MIMAT0018976 | –1.96 | < .001 | 0.097 |
a Refers to miRBase ( http://www.mirbase.org/ ).
To confirm the miRNA profiling studies, we performed qRT-PCR assays using probe sets designed to detect either the primary or mature forms of 5 miRNAs ( Figure 2 ). Both intergenic miRNAs that are processed from primary transcripts of the MIR143/145 transcript cluster were down-regulated by ∼ 2.5-fold following cytokine treatment ( Figure 2 , A and B), but neither of the corresponding mature miRNAs were differentially expressed by qRT-PCR ( Figure 2 , F and G). Both the primary and mature forms of miR-146a and miR-155 were up-regulated by qRT-PCR following IL-1β exposure ( Figure 2 , C and H), whereas no significant changes in the primary and mature forms of miR-4454 were detected ( Figure 2 , E and J).

Analysis of gene expression networks in response to cytokine and progesterone
To better understand the pathways potentially dysregulated in response to altered mature miRNA expression, we queried the DIANA miRPath server using miR-146a, miR-155, miR-525, and miR-924 as inputs. These 4 miRNAs were predicted to influence multiple signaling pathways ( Table 4 ), including the Toll-like receptor and mitogen-activated protein kinase pathways. A more extensive analysis revealed 1697 known/predicted targets for these miRNAs (∼ 241 targets per miRNA).
KEGG accession number | KEGG pathway name | Pathway description | P value | Targeted genes |
---|---|---|---|---|
hsa04620 | Toll-like receptor signaling pathway | Signaling pathway involved in the recognition of and cellular response to pathogen-associated molecular patterns. | < 0.0001 | CCL5, CD80, FOS, IFNA6, IFNA7, IKBKE, IRAK1, IRF5, MAPK10, PIK3CA, PIK3R1, RELA, TAB2, TBK1, TLR6, TLR7, TRAF3, TRAF6 |
hsa04666 | Fc gamma R-mediated phagocytosis | Refers to components of the phagocytic pathway activated upon binding of antibodies to Fc receptors; this often occurs following opsonization of foreign extracellular materials as a host-defense mechanism. | < 0.0001 | AMPH, ARF6, ARPC5, CDC42, DOCK2, HCK, INPP5D, MYO10, PIK3CA, PIK3R1, PIP5K1B, PLA2G4F, PLD2, PRKCE, RPS6KB1, VAV3 |
hsa04722 | Neurotrophin signaling pathway | Pertains to signaling pathways activated by a family of trophic factors involved in differentiation and survival of neural cells. | < 0.0001 | BAX, BDNF, BDNF, CAMK2D, CDC42, FASLG, GSK3B, IRAK1, KRAS, MAPK10, NRAS, NTRK3, NTRK3, PIK3CA, PIK3R1, RAP1B, RELA, RPS6KA3, SORT1, SOS1, TRAF6 |
hsa04012 | ErbB signaling pathway | Signaling pathways activated through ErbB receptor tyrosine kinases, which regulate diverse cellular responses including proliferation, differentiation, motility, and survival. | < 0.0001 | ABL2, CAMK2D, ERBB2, ERBB4, GSK3B, KRAS, MAPK10, NRAS, PAK2, PAK7, PIK3CA, PIK3R1, RPS6KB1, SOS1 |
hsa05213 | Endometrial cancer | Includes pathways implicated in type-I and type-II endometrial carcinomas. | < 0.0001 | APC, CTNNA3, ERBB2, GSK3B, ILK, KRAS, NRAS, PIK3CA, PIK3R1, SOS1, TCF4 |
hsa04010 | MAPK signaling pathway | Refers to processes regulating the mitogen-activated protein kinase (MAPK) cascade, which directs various cellular functions including proliferation, differentiation, migration, and inflammation. | < 0.0001 | BDNF, CACNB3, CACNB4, CDC42, DUSP14, FASLG, FGF7, FGF9, FOS, HSPA1A, IL1A, KRAS, MAP3K13, MAP3K2, MAP4K3, MAPK10, NRAS, PAK2, PDGFRA, PLA2G4F, PTPRQ, RAP1B, RAPGEF2, RASA2, RASGRP4, RELA, RPS6KA3, RPS6KA4, SOS1, TAB2, TAOK1, TRAF6 |
hsa05200 | Pathways in cancer | Refers to multiple signaling cascades de-regulated in human cancers including those promoting proliferation, tissue invasion, metastasis, angiogenesis, evasion of apoptosis, and resistance to anti-growth signals. | 0.0001 | APC, ARNT, BAX, CDC42, COL4A3, CSF1R, CTNNA3, DAPK1, E2F2, ERBB2, ETS1, FASLG, FGF7, FGF9, FOS, FZD4, FZD5, GSK3B, HHIP, IGF1R, KITLG, KRAS, MAPK10, NRAS, PDGFRA, PGF, PIAS2, PIK3CA, PIK3R1, PTCH1, RARB, RB1, RELA, SKP2, SMAD4, SOS1, SUFU, TCEB1, TCF4, TRAF3, TRAF6 |
hsa00900 | Terpenoid backbone biosynthesis | Refers to metabolic pathways involved in the synthesis of terpenoids (isoprenoids), which give rise to steroid, sterol, and carotenoid precursor molecules; the HMG-CoA reductase pathway is an example. | 0.0001 | ACAT1, FNTA, HMGCS1, PCYOX1, PDSS2, RCE1 |
hsa05211 | Renal cell carcinoma | Includes pathways commonly de-regulated in renal cell carcinomas. | 0.0002 | ARNT, CDC42, ETS1, KRAS, NRAS, PAK2, PAK7, PGF, PIK3CA, PIK3R1, RAP1B, SOS1, TCEB1 |
hsa05160 | Hepatitis C | Comprises several host cell immunity pathways that are subverted during hepatitis C virus infection. | 0.0002 | DDX58, GSK3B, IFNA6, IFNA7, IKBKE, KRAS, MAPK10, NRAS, PIK3CA, PIK3R1, PPP2CA, PPP2R1A, RELA, SCARB1, SOS1, TBK1, TRAF3, TRAF6 |
Because the destabilization of target mRNAs is thought to be a major mechanism through which miRNAs reduce target protein expression, we compared these target predictions with our microarray expression data. Interestingly, only 38 of the known or predicted mRNA targets (2.2%) exhibited differential expression by microarray, and of these, only 32% exhibited anticorrelated expression with their corresponding miRNAs. Network diagrams of experimentally validated miRNA-mRNA interactions, in addition to predicted TF-mRNA interactions, are shown in Figure 3 .

Comment
Principal findings of the study
In this model of uterine decidual stroma depleted of immune and epithelial cells, we demonstrated that IL-1β induced a panoply of proinflammatory genes. Some of the more highly expressed genes induced by IL-1β were coding sequences for chemokines, cytokines, prostaglandin-synthesizing enzymes, microsomal prostaglandin E synthase, transcriptional regulators, and matrix metalloproteinase 1 ( Supplemental Figure 1 ). These results are highly consistent with our previous work in amnion stromal cells, a cell type with similarities to decidua stromal cells. Many of these genes have previously been implicated individually in models of preterm parturition.
Cytokine stimulation of noncoding RNAs in decidual cells: discordance in precursor and mature miRNAs
Microarray profiling additionally revealed that IL-1β altered the expression of a host of regulatory noncoding RNAs, including miRNA and lncRNA loci. However, in contrast to the sharp changes in the expression of miRNA genetic loci (ie, primary and precursor miRNA transcripts), the pool of mature miRNAs profiled by NanoString was relatively stable following cytokine stimulation. Strikingly, there was poor overall correlation between the transcriptional response of primary/precursor transcripts and their corresponding mature species under these conditions.
These findings were recapitulated using qRT-PCR: coordinate expression between the primary and mature forms was observed for only 3 of the 5 miRNAs selected for validation. Biogenesis of miRNA is a highly complex, multistep process, and such discordance might simply reflect the kinetics of the required processing reactions and/or the relative stability of each RNA species. Previous studies have found similar discordance, and the current results highlight a need to distinguish between precursor and mature miRNA signatures during profiling because they can represent nonredundant miRNA expression signatures.
Network and pathway analysis defines an inflammatory gene signature containing cytokine-driven mRNAs and miRNAs
Of the miRNAs exhibiting differential expression in response to cytokine challenge, it is noteworthy that both miR-146 and miR-155 were up-regulated. These results are consistent with the responses of these miRNAs to proinflammatory stimuli in other reports.
Additionally, both miR-146a and miR-155 have been implicated in the control of inflammation in several biological settings. In light of emerging evidence suggesting that certain miRNAs participate in regulatory loops that modulate the activity of TFs governing their own expression, it is worth noting that both of these miRNAs may be induced by NF-κB and have targets that, when inhibited, suppress NF-κB signaling.
The miRNAs, miR-143 and miR-145, have been previously shown to be up-regulated in amnion mesenchymal and epithelial cells, and the level of miR-143 in cells from reflected amnion was decreased after the onset of labor. Loss of miR-143 in the setting of inflammation may permit the heightened synthesis of prostaglandins that drive parturition events (ie, myometrial contractility and cervical ripening). Thus, miRNAs govern the overall expression of the inflammation cascade by dampening proinflammatory genes and, if unregulated, could lead to irreparable tissue damage.
It is likely regulatory RNAs prohibit modest local inflammation elicited by temporary loss of tissue homeostasis, as might occur in pregnancy. Thus, proinflammatory signals (ie, cytokines, chemokines, eicosanoid-synthesizing enzymes, and matrix metalloproteinases) in the decidua may be countered by miRNAs (such as miR-146a and miR-155) that are driven by the same transcription factors that incite the inflammatory response. In this context, therapeutic targeting of NF-κB, per se, could inadvertently thwart the dampening effects of the regulatory RNA segment of the inflammatory gene network. On the other hand, development of miRNA mimetics to globally dampen proinflammatory signaling may represent a novel therapeutic approach to halting inflammation, as may occur in the context of preterm parturition.
GRN modeling is an important adjunct to pathway analysis when interpreting transcriptomics data. In principle, GRN modeling may be used to infer activation or repression of signaling pathways based on global gene expression signatures, even when the genes of such pathways do not exhibit differential expression. In practice, however, such models have certain limitations.
Improving the accuracy of GRN modeling is important to human parturition research, given the difficulties in the direct detection of signaling events as they occur in utero. The development of methods to infer tissue-level signaling events antecedent to labor using gene expression signatures obtained after delivery could help to clarify molecular pathways that differentiate PTB subtypes and potentially reveal novel therapeutic targets. Realizing this goal will require improvements in bioinformatics tools for GRN inference as well as the development of PTB-specific databases cataloging high-dimensional data sets from clinical samples, animal models, and tissue culture experiments.
Potential clinical implications of the study
Understanding the contribution of miRNAs to physiological pregnancy maintenance, term labor, and PTB is at an early stage. Our present results suggest that decidual miRNAs differentially regulated in response to cytokine challenge have target genes that are themselves part of the proinflammatory transcriptional cascade. This implies that these miRNAs have evolved as part of a greater regulatory network that serves to limit (at least up to a certain threshold) and/or resolve inflammation in utero, and may ultimately be exploitable as therapeutic agents.
Future studies directed at defining the role of miRNAs in inflammation within intrauterine tissues are warranted and may be assisted using systems biology approaches that incorporate information obtained from studies utilizing both in vitro and animal models. This will include the functional analysis of highly expressed miRNAs to confirm the nature of their molecular targets.
The ultimate goal of this line of investigation is to provide improved clarity in coding and noncoding gene expression in the setting of inflammation and the role of progesterone in regulating proinflammatory gene networks. This research may provide new molecular targets for intervention in preventing or managing preterm labor.
Limitations and future directions
The current study was conducted as a proof of principle to assess the utility of gene network analysis to predict mRNA and miRNA transcripts that participate in term and preterm parturition. Admittedly, the sample sizes for these experiments were limited in number, and we are building additional specimens into the investigations moving forward. In addition, after identifying key miRNAs, we will perform functional analyses of these tune-tuning noncoding RNAs to confirm their authentic targets.
Acknowledgments
This work is presented in partial fulfillment of the Fellowship in Maternal-Fetal Medicine at The Ohio State University by Dr Sherrine Ibrahim and was supported by the Ohio State University Perinatal Research and Development Fund and the March of Dimes Prematurity Research Center Ohio Collaborative (grants 22-FY13-543 and 22-FY14-470). Portions of this work were presented at the 2013 annual meetings of the Society for Gynecologic Investigation. The authors gratefully acknowledge Dr Hansjuerg Alder and the staff of the Ohio State University Comprehensive Cancer Center Nucleic Acid Shared Resource for technical assistance and the nursing staff of the Labor and Delivery Units at Yale University Medical Center and the Wexner Medical Center and for assistance in collecting specimens.
Supplemental Methods
Decidual stromal cell isolation and culture
Decidual fibroblasts were prepared from placentas delivered prior to labor onset following term cesarean delivery. Briefly, the decidua was scraped from the maternal surface of the chorion, minced, and incubated with 25 μg/mL of collagenase (200 U/mg; Worthington Biochemical, Lakewood, NJ) and 6.25 U/mL of deoxyribonuclease (Sigma-Aldrich, St Louis, MO). Cell clusters in the final digested tissue were further dissociated by passage through a 23-gauge needle.
The cells were enriched by centrifugation through discontinuous Percoll gradients and then cultivated over 3 passages until > 99% free of CD45 + leukocytes in phenol red-free Dulbecco’s modified Eagle medium/Ham’s F-12 (50:50; Life Technologies, Carlsbad, CA) supplemented with 10% charcoal-stripped calf serum, 100 U/mL penicillin, 100 μg/mL streptomycin, and 0.25 μg/mL amphotericin B.
At confluence, decidual cells were primed for 13 days in basal medium supplemented with charcoal-stripped calf serum containing 10 –8 M 17β-estradiol (17β-E 2 ; Sigma-Aldrich) and 10 –7 M medroxyprogesterone acetate (Sigma-Aldrich). The cultures were rinsed with phosphate-buffered saline (pH 7.2) and switched to a defined medium consisting of serum-free basal medium with ITS+ Premix (insulin, transferrin, selenium; BD Biosciences, Franklin Lakes, NJ), 5 μM FeSO 4 , 0.5 μM ZnSO 4 , 1 nM CuSO 4 (Sigma-Aldrich), 50 μg/mL ascorbic acid (Sigma-Aldrich), and 50 ng/mL human recombinant epidermal growth factor (BD Biosciences) with 17β-estradiol and medroxyprogesterone acetate overnight prior to the experiments. The cultures were then treated with phosphate-buffered saline containing 0.1% bovine serum albumin (vehicle control) or with 1 ng/mL of human recombinant interleukin-1β, (R&D Systems, Minneapolis, MN) for 6 hours. Cells from 3 different patients were used for the studies.
Quantitative real-time-reverse transcriptase (qRT-PCR) analysis
To verify the expression patterns for select messenger ribonucleic acids (mRNAs), reverse transcription was performed using 1 μg of total RNA, oligo(dT)12-18 primers, and Superscript III reverse transcriptase (Invitrogen, Carlsbad, CA).
The following TaqMan gene expression assays (Applied Biosystems, Foster City, CA) were used for qRT-PCR: Hs00153133_m1 ( PTGS2 ); Hs00985639_m1 ( IL6 ); Hs00153283_m1 ( NFKBIA ); Hs00601975_m1 ( CXCL2 ); Hs00174128_m1 ( TNF ); Hs00174103_m1 ( IL8 ); Hs00899658_m1 ( MMP1 ); and Hs00610420_m1 ( PTGES ). Each 20 μL reaction consisted of 5 μL complementary deoxyribonucleic acid (250 ng), 1 μL of TaqMan primer/probe mixture, and 10 μL LightCycler 480 Probes master mix (Roche Applied Science, Mannheim, Germany), and 4 μL of nuclease-free water. Amplifications were performed on the LightCycler 480 System (Roche Applied Science).
For primary miRNAs (pri-miRNAs), reverse transcription was performed using the high-capacity complementary deoxyribonucleic acid reverse transcription kit (Applied Biosystems), according to the manufacturer’s instructions. Each reaction comprised 10 μL of master mix (10× reverse transcription buffer, deoxynucleotide triphosphate mix, reverse transcription random primers, Multiscribe reverse transcription enzyme, ribonuclease inhibitor, and nuclease-free water) and 1 μg of RNA (in 10 μL).
For qRT-PCR, TaqMan gene expression master mix and TaqMan Pri-miRNA assays (Applied Biosystems) were used. The assays were: Hs03303166_pri ( MIR143 ); Hs03303169_pri ( MIR145 ); Hs03303259_pri ( MIR146A ); Hs03303349_pri ( MIR155 ); and Hs04258223_pri ( MIR445 ). For mature miRNAs, reverse transcription was performed with 10 ng of total RNA using the TaqMan microRNA reverse transcription kit (Applied Biosystems), per the manufacturer’s recommendations. Each reaction received 7 μL of master mix, 5 μL of RNA (10 ng), and 3 μL of the reverse transcription primer appropriate for each target miRNA. TaqMan gene expression master mix and TaqMan microRNA assays (Applied Biosystems) were used for qRT-PCR. The following assays were used: 002249 (hsa-miR-143-3p); 002278 (hsa-miR-145-5p); 000468 (hsa-miR-146a-5p); 002623 (hsa-miR-155-5p); and 461830_mat (hsa-miR-4454).
All reactions were performed on the LightCycler 480 system (Roche Applied Science) using the cycling conditions recommended by either the TaqMan Pri-miRNA assays protocol (for pri-miRNAs) or the TaqMan small RNA assays protocol (for mature miRNAs).
Microarray profiling and data analysis
Total RNA (250 ng per sample) was processed using the Ambion WT expression kit (Austin, TX) and labeled with Affymetrix GeneChip whole-transcript sense target labeling assay (Affymetrix, Santa Clara, CA), followed by hybridization to the Affymetrix Human Gene 2.0 ST array according to the manufacturers’ protocols. The Affimetrix GeneChip Human Gene 2.0 ST whole-transcript array platform containing probes measuring 53,617 transcript clusters (including 40,716 reference sequence transcripts comprised of mRNAs, precursor miRNAs, and long intergenic noncoding RNAs) was used for transcriptome profiling.
Following hybridization and scanning using Affymetrix GeneChip Scanner 3000 7G, the feature intensity (CEL) files were preprocessed using the Affymetrix Expression Console software version 1.3 by executing the default gene Robust Multichip Analysis and sketch quantile normalization algorithms to generate summarized expression values (CHP files).
The analysis included several quality control assessments to confirm fidelity of the individual hybridizations. Gene level differential expression analysis was subsequently performed using the Affymetrix Transcriptome Analysis Console software version 1.0 using the default analytical pipeline including the following algorithms: Tukey’s biweight averaging, unpaired single-factor analysis of variance, and the Benjamini-Hochberg step-up false discovery rate (FDR)-controlling procedure. For the default analysis, a linear fold-change threshold of ± 2 and an FDR of 0.1 were used.
Mature microRNA profiling and analysis
The nCounter platform (human version 2 miRNA expression assay; NanoString Technologies, Seattle, WA) was used for mature miRNA profiling. Total RNA (100 ng) was used as input for nCounter miRNA sample preparation reactions, performed according to the manufacturer’s instructions. Specific DNA tags were ligated to the 3′ end of each mature miRNA in a multiplexed ligation reaction using reverse-complementary bridge oligonucleotides to direct the ligation of each miRNA to its designated tag. The resulting material was hybridized with a panel of miRNA:tag-specific nCounter capture and barcoded reporter probes.
Hybridization reactions were performed according to the manufacturer’s instructions at 64°C for a minimum of 18 hours. Hybridized probes were purified using the nCounter Prep Station and analyzed using the nCounter digital analyzer to count individual fluorescent barcodes and quantify target RNA molecules present in each sample. For each assay, a high-density scan (600 fields of view) was performed.
Technical normalization was performed using nSolver Analysis Software version 1.1, according to the recommendations of the manufacturer (NanoString Technologies). Probes with low levels of expression (defined as less than the mean plus 2 SD of counts assigned to negative control probes, which was 22.4 in our data set) were omitted from subsequent analyses.
Differential expression analysis of the NanoString data was performed using the edgeR (version 3.4.0) Bioconductor package, as implemented within the R statistical programming language. Trimmed mean of M-values normalization was used together with the generalized linear model approach coupled with a paired-sample design matrix (matching was based on decidual cell preparations from each of the individual donors). Differential expression was determined using the glmLRT function, which performs generalized linear model likelihood ratio tests. For FDR control, the method of Benjamini and Hochberg was used.
MicroRNA target analysis
Known or predicted miRNA targets were obtained using a combination of the multiMiR (version 1.0.1) Bioconductor package, the Ingenuity Pathway Analysis microRNA target filter algorithm (QIAGEN, Valencia, CA) and manual searches of available target prediction algorithms. All experimentally validated targets from the Ingenuity Expert Findings, TarBase, miRTarBase, and/or miRecords databases were retained. We additionally accepted potential targets present consistently among 3 or more of the following 8 databases: DIANA microT-CDS, EIMMo, MicroCosm, miRanda, miRDB, PicTar, PITA, and TargetScan.
Pathway analysis and regulatory network modeling
Pathway enrichment analysis for differentially expressed transcripts based on microarray profiling data was performed using Enrichr web-based application. The DNA Intelligent Analysis (DIANA) miRPath version 2.0 analysis web server was used to predict pathway dysregulation in response to fluctuating miRNA expression. To identify transcription factors activated or inhibited in response to proinflammatory stimulation, differentially expressed microarray transcripts were evaluated using the Ingenuity Pathway Analysis Upstream Regulator analysis tool ( http://pages.ingenuity.com/IngenuityUpstreamRegulatorAnalysisWhitepaper.html ). These data were filtered based on molecule type to include ligand-dependent nuclear receptors and transcriptional regulators.
For the mRNA expression data, transcriptional factor binding motif enrichment analysis was performed in a manner similar to the one that we previously described, with modifications. National Center for Biotechnology Information reference sequence transcript accession numbers for unique, differentially expressed probes from our microarray analysis were curated by conversion from Entrez Gene IDs and/or Human Genome Organisation gene symbols using the g:Profiler tool set.
Multiple probes mapping to identical genes were consolidated using unique gene symbols. Genes with multiple reference sequences were resolved by using the accession number for the longest or most frequently appearing transcript. FASTA sequences corresponding to the promoter regions of individual mRNA transcripts (–1500 to +500 bp relative to each transcriptional start site) were abstracted from the NCBI36/hg18 human genome assembly using the University of California Santa Cruz Genome Browser ( http://genome.ucsc.edu/cgi-bin/hgGateway ). These sequences were then analyzed for overrepresented transcriptional factor binding motifs using the Transcription Factor Affinity Prediction web tool with JASPAR core vertebrate matrix models, a background model of human promoters, and Benjamini-Hochberg multiple testing correction. All network interaction diagrams were rendered using the Gephi network analysis and visualization software package version 0.8.2.
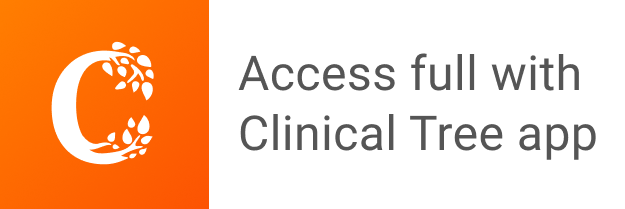