Background
Gestational diabetes mellitus (GDM) is one of most common complications of pregnancy, with incidence rates varying by maternal age, race/ethnicity, obesity, parity, and family history. Given its increasing prevalence in recent decades, covariant environmental and sociodemographic factors may be additional determinants of GDM occurrence.
Objective
We hypothesized that environmental risk factors, in particular measures of the food environment, may be a diabetes contributor. We employed geospatial modeling in a populous US county to characterize the association of the relative availability of fast food restaurants and supermarkets to GDM.
Study Design
Utilizing a perinatal database with >4900 encoded antenatal and outcome variables inclusive of ZIP code data, 8912 consecutive pregnancies were analyzed for correlations between GDM and food environment based on countywide food permit registration data. Linkage between pregnancies and food environment was achieved on the basis of validated 5-digit ZIP code data. The prevalence of supermarkets and fast food restaurants per 100,000 inhabitants for each ZIP code were gathered from publicly available food permit sources. To independently authenticate our findings with objective data, we measured hemoglobin A1c levels as a function of geospatial distribution of food environment in a matched subset (n = 80).
Results
Residence in neighborhoods with a high prevalence of fast food restaurants (fourth quartile) was significantly associated with an increased risk of developing GDM (relative to first quartile: adjusted odds ratio, 1.63; 95% confidence interval, 1.21–2.19). In multivariate analysis, this association held true after controlling for potential confounders ( P = .002). Measurement of hemoglobin A1c levels in a matched subset were significantly increased in association with residence in a ZIP code with a higher fast food/supermarket ratio (n = 80, r = 0.251 P < .05).
Conclusion
As demonstrated by geospatial analysis, a relationship of food environment and risk for gestational diabetes was identified.
Introduction
Gestational diabetes mellitus (GDM), defined as glucose intolerance first detected during pregnancy, is a prevalent pregnancy comorbidity and strong predictor of type 2 diabetes mellitus (DM) in both the mother and her offspring. The incidence of GDM among women in the United States has increased in recent decades with higher prevalence in Hispanic and African American populations. While there are a number of recognized risk factors, the occurrence of GDM increases by virtue of maternal age, obesity (body mass index [BMI] >30), race/ethnicity, smoking, and familial history. In addition, early initial studies have shown that potentially modifiable independent factors such as physical activity, diet, and low socioeconomic status are associated with the development of gestational diabetes. With respect to diet and of interest to our work reported herein, the influence of distance to food establishments on the diet quality of gravidae (independent of diabetes) has been described. In the nonpregnant population, there is early and initial evidence suggesting an association between food environment and diabetes later in life among nonpregnant subjects. However, to date no one has linked food availability in pregnancy with the occurrence of GDM.
To analyze the potential influence of the food environment on GDM, we performed geospatial modeling in Harris County, Texas (the third most populous US county), using subject ZIP code data. Geospatial modeling utilizes multimodal databases to assess the prevalence of environmental factors in specific geographic areas with the cooccurrence of disease. When integrated with robust perinatal databases, geospatial modeling serves as an approach to investigate and evaluate previously not considered risk factors, as a study on smoking during pregnancy and antenatal care within spatial clusters has shown. In our study, we focused on the risk factors of population density, and availability of healthy and unhealthy food, and examined their potential relationship with GDM.
The objective of our study was to employ geospatial modeling to characterize the association of the relative availability of food choices (supermarkets and fast food restaurants) with GDM and elevated hemoglobin A1c (HbA1c) values.
Materials and Methods
Subjects and specimens
This study population is part of an ongoing perinatal database and biobank (PeriBank) at Baylor College of Medicine. Institutional review board approval for this study was given by Baylor College of Medicine (H-26364). Subjects were recruited by trained PeriBank study personnel who approach eligible gravidae at the time of admission to labor and delivery. After consent was obtained, >4900 variables of clinical information were directly extracted from the electronic medical record and accompanying prenatal records alongside directed subject questioning. A subset of charts are routinely audited by a board-certified maternal-fetal medicine physician scientist (K.M.A.) to ensure quality of the data. For this study, extracted and analyzed clinical data include socioeconomic background (income and education), patient history (diseases, vaccination record, current and past smoking status, substance abuse), and residential information coded to the patient’s 5-digit ZIP code by trimester of pregnancy for where they live and where they work. Routine screening for GDM was performed between 24-28 weeks of pregnancy with a 50-g oral glucose challenge test (cutoff value of 140 mg/dL), followed by a 100-g oral glucose tolerance test. Blood samples were collected under a uniform protocol and stored under high integrity conditions at –80°C. Over the interval of this study, PeriBank enrolled 90.8% of all gravidae delivering at the Baylor College of Medicine–affiliated hospital, Ben Taub General Hospital (Harris Health System, Houston, TX).
Inclusion criteria
We included all singleton pregnancies enrolled from August 2011 through December 2014 (n = 10,208). The exclusion criteria were lack of validated ZIP code information (n = 648), residential ZIP code outside of Harris County, Texas (n = 533), hospitalization without delivery (n = 32), and type 1 DM (n = 83). Data extraction was performed for key aspects of maternal demographic and pregnancy history as described above.
Data sources for geospatial analysis
Publicly available data sources including the 2011 and 2012 US Census were utilized to determine the population density (number of food establishments per 100,000 inhabitants) and the availability of food establishments (here defined as the entity of fast food restaurants, supermarkets, and other grocery stores) on ZIP code basis: ZIP Code Business Patterns were utilized to assess economic activity of every registered establishment within ZIP code areas of Harris County, Texas. The US Census Bureau databank does not include specific information on the sales volume nor affiliation to a certain chain. Food establishments were coded by the North American Industry Classification System. We used the code 445110 for “supermarket and other grocery (except convenience stores) stores” extraction, simply referred to as “supermarkets” throughout this article. Additionally code 445120 was used for extraction of information on “convenience stores” and code 722513 was used for the extraction of numbers of “limited service restaurants” being referred to as “fast food restaurants” throughout the text. The terminus “food environment” refers to food establishments within a defined residential area.
Harris County, Texas, which includes several smaller cities besides Houston (eg, Bellaire, Pasadena, Spring, and Katy), has 230 annotated ZIP codes, of which only 132 reflect residential areas (remainder are PO box or company ZIP codes). The proportion of food establishments to the population within each ZIP code was calculated to obtain density specification per 100,000 inhabitants.
Assumptions that ZIP code data may not reflect sole exposure to food environment is justified, as inhabitants cross ZIP code area borders for shopping and dining, in transit to and from work, and for lack of supermarkets in several less populous ZIP codes. To overcome this potential limitation, we employed the technique of creating buffers (or buffer zones) around every single ZIP code in accordance with previously validated means. A buffer zone was defined as the entity of multiple neighboring ZIP codes that entirely surrounded 1 central ZIP code of interest. The range of ZIP codes in a buffer was 3-11. These buffer zones were generated by manual curation, based on current ZIP code maps of Harris County, Texas. In succession we approximated the density of food establishments within the corresponding neighborhood (here defined as central ZIP code plus buffer zone) of a single ZIP code where the patients most likely conducted their grocery shopping and dining out during pregnancy. Density of food establishments in each neighborhood was calculated using a weighted mean approach (sum of food establishments in central ZIP code plus buffer zone/sum of inhabitants within the central ZIP code plus sum of inhabitants within the buffer zone). The buffer approach is an established method of defining neighborhoods and creating a basis for calculating density of establishments within reach of residence. Nevertheless there is no compulsory criteria available regarding appropriate buffer determination in the current literature. For each pregnancy ZIP code and neighborhood food establishment density was assigned by first-trimester residential ZIP code. If not available, second- or third-trimester ZIP code information was utilized instead.
Correlation of gestational HbA1c levels with food environmental factors
Maternal HbA1c and total hemoglobin levels were measured in 80 serum specimens from maternal term blood samples. Specifically, HbA1c was uniformly measured in extracted specimens obtained from PeriBank using commercially available human glycated HbA1c enzyme-linked immunosorbent assay kits (CSG-E08139h, CUSABIO, Wuhan, China) following the manufacturers’ protocols. Per measurement, 50 μL of serum was used. Percentage of HbA1c was calculated according to the Diabetes Control and Complications Trial using the following formula: HbA1c [%] = HbA1c [mmol/mol Hgb]/10.929 + 2.15.
Statistical analysis
Clinical and demographic variables for all pregnancies were extracted from the PeriBank database. Subsequent handling of the data as well as statistical testing was performed using software (SPSS, Version 22, IBM Corp, Armonk, NY). Descriptive statistics (frequency, median, and interquartile range [IQR]) were used to characterize the demographic, clinical, and environmental variables of the data set. Since all continuous variables were found to be nonnormally distributed on Kolmogorov-Smirnov tests, nonparametric statistical tests were utilized for bivariate analyses. Associations between gestational diabetes occurrence and food establishment density were tested using Spearman rank correlation. Prior to entering into the regression models, nonnormally distributed variables were log-transformed. Multiple logistic regression analysis was performed to assess the associations between gestational diabetes and food establishment density, adjusting for race/ethnicity, education, gravidity, income, maternal age, and BMI. In addition, odds ratios (OR) for GDM occurrence were calculated with stratified environmental variables on the basis of median and quartiles (above vs below median, fourth vs first quartile). Adjusted OR (aOR) were calculated by correcting for the same variables as described above. Correlation between HbA1c level and food environment was assessed using Spearman rank correlation, followed by multilinear regression analysis. Across all statistical analysis, P < .05 was accepted as significant. Values are given as count (percentage) or median (IQR), except as indicated otherwise.
Materials and Methods
Subjects and specimens
This study population is part of an ongoing perinatal database and biobank (PeriBank) at Baylor College of Medicine. Institutional review board approval for this study was given by Baylor College of Medicine (H-26364). Subjects were recruited by trained PeriBank study personnel who approach eligible gravidae at the time of admission to labor and delivery. After consent was obtained, >4900 variables of clinical information were directly extracted from the electronic medical record and accompanying prenatal records alongside directed subject questioning. A subset of charts are routinely audited by a board-certified maternal-fetal medicine physician scientist (K.M.A.) to ensure quality of the data. For this study, extracted and analyzed clinical data include socioeconomic background (income and education), patient history (diseases, vaccination record, current and past smoking status, substance abuse), and residential information coded to the patient’s 5-digit ZIP code by trimester of pregnancy for where they live and where they work. Routine screening for GDM was performed between 24-28 weeks of pregnancy with a 50-g oral glucose challenge test (cutoff value of 140 mg/dL), followed by a 100-g oral glucose tolerance test. Blood samples were collected under a uniform protocol and stored under high integrity conditions at –80°C. Over the interval of this study, PeriBank enrolled 90.8% of all gravidae delivering at the Baylor College of Medicine–affiliated hospital, Ben Taub General Hospital (Harris Health System, Houston, TX).
Inclusion criteria
We included all singleton pregnancies enrolled from August 2011 through December 2014 (n = 10,208). The exclusion criteria were lack of validated ZIP code information (n = 648), residential ZIP code outside of Harris County, Texas (n = 533), hospitalization without delivery (n = 32), and type 1 DM (n = 83). Data extraction was performed for key aspects of maternal demographic and pregnancy history as described above.
Data sources for geospatial analysis
Publicly available data sources including the 2011 and 2012 US Census were utilized to determine the population density (number of food establishments per 100,000 inhabitants) and the availability of food establishments (here defined as the entity of fast food restaurants, supermarkets, and other grocery stores) on ZIP code basis: ZIP Code Business Patterns were utilized to assess economic activity of every registered establishment within ZIP code areas of Harris County, Texas. The US Census Bureau databank does not include specific information on the sales volume nor affiliation to a certain chain. Food establishments were coded by the North American Industry Classification System. We used the code 445110 for “supermarket and other grocery (except convenience stores) stores” extraction, simply referred to as “supermarkets” throughout this article. Additionally code 445120 was used for extraction of information on “convenience stores” and code 722513 was used for the extraction of numbers of “limited service restaurants” being referred to as “fast food restaurants” throughout the text. The terminus “food environment” refers to food establishments within a defined residential area.
Harris County, Texas, which includes several smaller cities besides Houston (eg, Bellaire, Pasadena, Spring, and Katy), has 230 annotated ZIP codes, of which only 132 reflect residential areas (remainder are PO box or company ZIP codes). The proportion of food establishments to the population within each ZIP code was calculated to obtain density specification per 100,000 inhabitants.
Assumptions that ZIP code data may not reflect sole exposure to food environment is justified, as inhabitants cross ZIP code area borders for shopping and dining, in transit to and from work, and for lack of supermarkets in several less populous ZIP codes. To overcome this potential limitation, we employed the technique of creating buffers (or buffer zones) around every single ZIP code in accordance with previously validated means. A buffer zone was defined as the entity of multiple neighboring ZIP codes that entirely surrounded 1 central ZIP code of interest. The range of ZIP codes in a buffer was 3-11. These buffer zones were generated by manual curation, based on current ZIP code maps of Harris County, Texas. In succession we approximated the density of food establishments within the corresponding neighborhood (here defined as central ZIP code plus buffer zone) of a single ZIP code where the patients most likely conducted their grocery shopping and dining out during pregnancy. Density of food establishments in each neighborhood was calculated using a weighted mean approach (sum of food establishments in central ZIP code plus buffer zone/sum of inhabitants within the central ZIP code plus sum of inhabitants within the buffer zone). The buffer approach is an established method of defining neighborhoods and creating a basis for calculating density of establishments within reach of residence. Nevertheless there is no compulsory criteria available regarding appropriate buffer determination in the current literature. For each pregnancy ZIP code and neighborhood food establishment density was assigned by first-trimester residential ZIP code. If not available, second- or third-trimester ZIP code information was utilized instead.
Correlation of gestational HbA1c levels with food environmental factors
Maternal HbA1c and total hemoglobin levels were measured in 80 serum specimens from maternal term blood samples. Specifically, HbA1c was uniformly measured in extracted specimens obtained from PeriBank using commercially available human glycated HbA1c enzyme-linked immunosorbent assay kits (CSG-E08139h, CUSABIO, Wuhan, China) following the manufacturers’ protocols. Per measurement, 50 μL of serum was used. Percentage of HbA1c was calculated according to the Diabetes Control and Complications Trial using the following formula: HbA1c [%] = HbA1c [mmol/mol Hgb]/10.929 + 2.15.
Statistical analysis
Clinical and demographic variables for all pregnancies were extracted from the PeriBank database. Subsequent handling of the data as well as statistical testing was performed using software (SPSS, Version 22, IBM Corp, Armonk, NY). Descriptive statistics (frequency, median, and interquartile range [IQR]) were used to characterize the demographic, clinical, and environmental variables of the data set. Since all continuous variables were found to be nonnormally distributed on Kolmogorov-Smirnov tests, nonparametric statistical tests were utilized for bivariate analyses. Associations between gestational diabetes occurrence and food establishment density were tested using Spearman rank correlation. Prior to entering into the regression models, nonnormally distributed variables were log-transformed. Multiple logistic regression analysis was performed to assess the associations between gestational diabetes and food establishment density, adjusting for race/ethnicity, education, gravidity, income, maternal age, and BMI. In addition, odds ratios (OR) for GDM occurrence were calculated with stratified environmental variables on the basis of median and quartiles (above vs below median, fourth vs first quartile). Adjusted OR (aOR) were calculated by correcting for the same variables as described above. Correlation between HbA1c level and food environment was assessed using Spearman rank correlation, followed by multilinear regression analysis. Across all statistical analysis, P < .05 was accepted as significant. Values are given as count (percentage) or median (IQR), except as indicated otherwise.
Results
Study population
This is a nested cohort analysis of clinical and environmental data of 8912 gravidae with validated ZIP code data enrolled during the defined study period (August 2011 through December 2014). Demographic data are summarized in Table 1 . The median age of our study population was 29.3 (IQR 24.3-33.9) years with the majority being white Hispanic (68%), followed by white, non-Hispanic (20%), African American (9%), Asian (3%), Hawaiian/Pacific Islander (0.2%), and Native American (0.1%). The majority of our patients earned <$34,999 per year and only a minority earned above a familial low-income level ($35,000-74,000: 2.8% and >$75,000: 1.4%). The majority of subjects (58.6%) had not completed a high-school degree, were multigravida (median gravidity of 3), and were overweight in early pregnancy (median 27.3 kg/m 2 , 23.6-31.6) with sufficient or excessive weight gain (24.9% and 46.6%, respectively). Consistent with national and regional estimates in our cohort, 12.9% of subjects carried a diagnosis of GDM, while 3.6% of our patients had preexisting type 2 DM. Delivery routes include spontaneous vaginal (72.3%), cesarean (26.3%), and operative vaginal (1.2%) ( Table 2 ). With respect to newborn data of interest, 7.3% were macrosomic.
Demographics | Total Median (IQR) or % | GDM Median (IQR) or % | No GDM Median (IQR) or % | GDM including type 2 diabetes mellitus Median (IQR) or % | Type 2 diabetes mellitus (no GDM) Median (IQR) or % | GDM vs no GDM χ 2 or Kruskal-Wallis/Mann-Whitney U test | GDM including type 2 diabetes mellitus vs no GDM χ 2 or Kruskal-Wallis/Mann-Whitney U test |
---|---|---|---|---|---|---|---|
No. | 8912 | 1152 | 7442 | 1308 | 162 | 8594 | 8750 |
Age, y | 29.29 (24.29–33.92) | 32.57 (28.54–36.34) | 28.48 (23.69–33.12) | 32.73 (28.63–36.48) | 33.49 | P < .001 | P < .001 |
Gravidity | 3 (2–4) | 3 (2–5) | 3 (2–4) | 3 (2–5) | 3 (2–5) | P < .001 | P < .001 |
BMI (first trimester) | 27.29 (23.64–31.61) | 30.07 (26.58–34) | 26.6 (23.21–30.71) | 30.27 (26.72–34.15) | 30.7 (27.51–35.93) | P < .001 | P < .001 |
Ethnicity | |||||||
Hispanic | 80.2% | 86.6% | 79.0% | 86.5% | 86.4% | P < .001 | P < .001 |
Non-Hispanic | 19.8% | 13.4% | 21.0% | 13.5% | 13.6% | ||
Education | |||||||
<12th Grade | 58.6% | 62.6% | 57.6% | 62.4% | 71.8% | P = .005 | P = .003 |
High school/GED | 27.5% | 26.2% | 27.8% | 26.6% | 20.9% | ||
Some college | 6.4% | 6.3% | 6.5% | 6.1% | 4.5% | ||
College degree | 5.6% | 4.2% | 6.0% | 3.9% | 1.8% | ||
Masters degree | 1.1% | 0.7% | 1.1% | 1.0% | 0.9% | ||
Doctoral/professional | 0.8% | 0.1% | 0.9% | 0.1% | 0% | ||
Income | |||||||
<$34,999 | 97.2% | 96.9% | 95.5% | 97.0% | 97.8% | P = .028 | P = .019 |
$35,000–74,999 | 2.8% | 2.5% | 3.1% | 2.5% | 2.2% | ||
≥$75,000 | 1.4% | 0.5% | 1.8% | 0.5% | 0% |
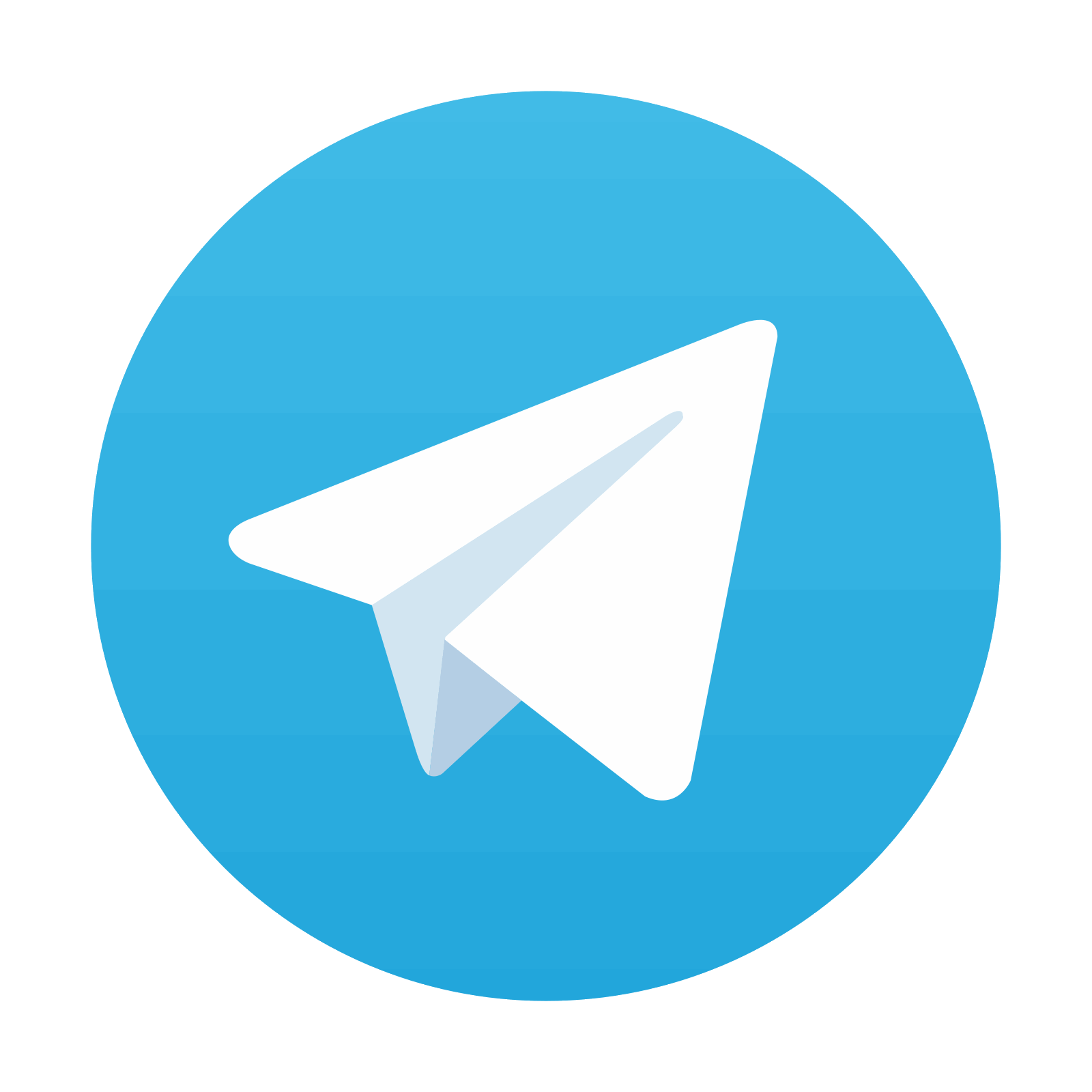
Stay updated, free articles. Join our Telegram channel

Full access? Get Clinical Tree
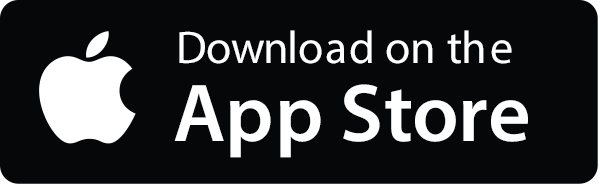
