Objective
We sought to identify first-trimester maternal serum biomarkers for the prediction of late-onset preeclampsia (PE) using metabolomic analysis.
Study Design
In a case-control study, nuclear magnetic resonance–based metabolomic analysis was performed on first-trimester maternal serum between 11 +0 -13 +6 weeks of gestation. There were 30 cases of late-onset PE, ie, requiring delivery ≥37 weeks, and 59 unaffected controls. The concentrations of 40 metabolites were compared between the 2 groups. We also compared 30 early-onset cases to the late-onset group.
Results
A total of 14 metabolites were significantly elevated and 3 significantly reduced in first-trimester serum of late-onset PE patients. A complex model consisting of multiple metabolites and maternal demographic characteristics had a 76.6% sensitivity at 100% specificity for PE detection. A simplified model using fewer predictors yielded 60% sensitivity at 96.6% specificity. Strong separation of late- vs early-onset PE groups was achieved.
Conclusion
Significant differences in the first-trimester metabolites were noted in women who went on to developed late-onset PE and between early- and late-onset PE.
Metabolomics, a relatively recent addition to the “omics” family, involves the high-throughput characterization and interpretation of the small-molecule metabolites (<1500 d) produced by cells, tissues, and organisms. To date, >8000 human metabolites from >80 chemical classes have been identified or catalogued. As technology improves it is expected that this number could grow by a factor of ≥10. Because of the wide chemical diversity of metabolites, their tight coupling with environmental interactions (food, drugs, gut microbiota), and their huge phenotypic-dependent concentration variations (≥10 6 ), metabolomics offers a powerful, quantitative route to describe the actual phenotype of cells, tissues, or organisms in both normal and diseased states. Recently, significant advances have occurred both in metabolite identification techniques and computational techniques for analyzing the large volume of data generated by metabolomic studies. There is currently tremendous interest in the use of metabolomics for the characterization and early diagnosis of complex diseases.
See Journal Club, page 87
Preeclampsia (PE) is a common obstetric disorder characterized by hypertension and proteinuria during pregnancy. It is a cause of significant morbidities, affecting the health of both the mother and fetus. However, its causes and pathophysiology largely remain a mystery. It now appears that PE is at least 2 fairly distinct disorders, an early-onset and a late-onset form. The early-onset variety typically occurs <34-35 weeks of pregnancy, and is associated with significant fetal morbidities. The pathophysiology is thought to be failure of trophoblast invasion of the maternal spiral arteriole resulting in maintenance of high maternal vascular resistance. This is consistent with the high frequency of placental underperfusion reported in this disorder.
The late-onset form is considered to be more of a maternal constitutional disorder due to underlying maternal microvascular disorders such as hypertension or a genetic predisposition in which poor trophoblast invasion is thought to play a less significant role. Late-onset PE is significantly more common and while it often has a mild course can be associated with significant clinical morbidities. It is therefore important to investigate its pathogenesis and if possible to develop biomarker predictors of this disorder.
Studies have now confirmed the clinical feasibility of first-trimester screening for early-, late-, and intermediate-onset varieties of PE using demographic, clinical, biomarker, and uterine artery Doppler information. Recently, the National Collaborating Center for Women’s and Children’s Health in the United Kingdom issued clinical guidelines for routine early prenatal screening for PE based on maternal demographic, historical, and clinical characteristics. It is possible that, in the future, combining clinical with biomarker predictors could further enhance screening accuracy. Our primary objective was to evaluate the use of metabolomics to identify first-trimester biomarkers of late-onset PE. Secondarily, we evaluated the diagnostic accuracy of these markers for late-onset PE prediction. Finally, we evaluated the capability of metabolomics for distinguishing late- from early-onset PE.
Materials and Methods
Study population
This study is part of an ongoing prospective study being conducted by the Fetal Medicine Foundation, London, United Kingdom, for the first-trimester prediction of important fetal and obstetric disorders. Institutional review board project #02-03-033 approval was obtained on March 14, 2003. The details of patient evaluation and study methods have been extensively described in a prior report of metabolomic prediction of early-onset PE. A routine population of British women was prospectively screened from March 2003 through September 2009 and they all gave written consent to participate in the study, which was approved by the King’s College Hospital Research Ethics Committee. Briefly, women were recruited at 11 +0 −13 +6 weeks’ gestation. Maternal characteristics and medical history were documented and first-trimester ultrasound, including crown-rump length (CRL) and uterine artery Doppler pulsatility index (PI), was measured. Data collection was planned before laboratory testing. The lower of the left and right uterine artery Doppler PI value was used for PE prediction. Maternal serum samples were also obtained and stored at −80°C for subsequent laboratory analysis. The long-term objective of the project is to develop and evaluate new markers and existing biomarkers of PE. Apart from the previously published study on early-onset PE these cases represent the first metabolomic analysis of PE from this study population.
A total of 30 singleton pregnancies that subsequently developed late-onset PE requiring delivery >34 weeks formed the study group and were matched with 60 unaffected controls. These cases were not previously used in any prior publication. The late-onset PE cases were selected at random from our database of available stored samples. Each case of late-onset PE was matched with 2 controls who delivered a phenotypically normal neonate with appropriate birth weight for gestational age at term and did not develop any hypertensive disorder of pregnancy and who had blood collected within 3 days of assessment of the late-onset PE case. There was no evident source of bias in the selection of cases or controls. The definition of PE used was that proposed by the International Society for the Study of Hypertension in Pregnancy, namely systolic pressure ≥140 mm Hg or diastolic pressure ≥90 mm Hg on ≥2 occasions 4 hours apart >20 weeks of gestation, in women who were previously normotensive. Proteinuria was defined as a total of 300 mg in a 24-hour urine collection or 2 readings of at least 2 + proteinuria on a midstream or catheterized urine specimen in the absence of a 24-hour urine collection must also have been present in addition to the hypertension. Proteinuria must also have been present in addition to the hypertension for the diagnosis of PE. No HELLP syndrome or gestational hypertension cases were included.
Nuclear magnetic resonance (NMR) spectrometry was used for metabolite identification in the specimen samples. Sample preparation and NMR spectroscopy methods were performed as detailed previously using a 500-MHz Varian Inova NMR spectrometer (Varian Inc, Palo Alto, CA). Forty metabolites in each serum sample were identified and quantified in each case and control sample using commercial (NMR Suite 7.1; Chenomx Inc, Edmonton, Alberta, Canada) spectral fitting software containing an NMR spectral reference library of >200 compounds. On initial metabolomic analysis, readers were blinded to patient status.
Statistical analysis
Recommended statistical procedures for metabolomic analysis were followed. Log scaling was used for normalization of all metabolomic data. Principal component analysis (PCA) and partial least squares discriminant analysis (PLS-DA) were performed to identify patterns. PCA is an unsupervised classification technique for transforming a complex collection of data points such that the important properties of the sample can be more simply displayed along the X- and Y-axes. Two clusters on the PCA plot indicate that there are significant metabolite differences between the normal and control groups.
PLS-DA is used to enhance the separation between the groups by summarizing the data into a few latent variables that maximize covariance between the response and the predictors. To minimize the possibility that the observed separation on PLS-DA is due to chance, permutation testing was performed. This involved repeated (2000 times) data sampling, with different random labeling. A significant P value indicates that the separation observed between groups is very unlikely to be due to chance. The MetaboAnalyst computer program was used to perform (PCA and PLS-DA) analyses. A variable importance in projection (VIP) plot is a plot ranking the metabolites based on their importance in discriminating study from control groups. Metabolites with the highest values on X-and Y-axes are the most powerful group discriminators.
In comparing the concentrations of metabolites between groups, outlier testing was performed using Dixon Q test. The Dixon Q test is used for identification of outliers in the dataset and replaces that value with the one closest to it. Replacement of outliers helps to meet the assumption of normal distribution and equal variance between groups. Only a single value (for valine) was adjusted in this fashion, however. Kolmogorov-Smirnov and Shapiro-Wilk tests of normal distribution were performed. Metabolite concentrations in late-onset PE vs controls were compared using the 2-tailed t test. Mann-Whitney U test was used in comparing metabolite concentrations between groups that were not normally distributed. Other independent variables including fetal CRL, uterine artery Doppler, PI, and maternal age, parity, weight, ethnicity, smoking, and medical disorders were included in the genetic computing analyses along with the metabolite concentrations for PE prediction.
Genetic programming is a branch of evolutionary computing while genetic computing is a branch of genetic programming. The advantage of genetic computing lies in its ability to handle nonnormally distributed outcome measures and the large volume of data generated from “omics” laboratory analyses and it has previously been successfully used for metabolomic analysis. Genetic computing generates rules by which an optimal number of variables can be selected from a large number of exploratory variables for the optimal prediction of the outcomes of interest. The Gmax computer program, version 11.09.23 (Genetic Computing Consultants Limited, London, UK) was used for genetic computing analysis.
In addition, using a limited number of independent biochemical and demographic predictor variables, logistic regression was used to generate probability equations for the prediction of PE. Based on the regression equations generated, individual probability for developing late-onset PE was calculated. Receiver operating characteristics curves with sensitivity plotted against false-positive rates (1-specificity) were generated along with the 95% confidence interval and/or P values for the area under the curve. For the primary analysis, power analysis indicated that a minimum of 17 cases was needed in each group to have 80% power for a 2-sided P < .0001.
After the primary analysis, a normal group of 60 control cases previously reported for the early-onset PE metabolomic study derived from the same patient population was subsequently added to the current study group of 30 cases and 59 control patients to permit sufficient power to perform the regression analyses. Finally, we used PCA and PLS-DA analyses to determine whether late-onset PE could be differentiated from early-onset PE by directly comparing the 30 early-onset PE cases reported in a prior publication with the 30 late-onset PE cases in the current study.
Results
Results for a total of 30 cases and 59 (of 60) controls used for the primary analysis are reported, as insufficient volume of serum was available for metabolomic analysis in one of the control samples. Table 1 compares the maternal age, weight, race, and gestational age at blood collection was determined by and represented by CRL measurements, between late-onset PE and normal cases. There was a significant difference in maternal race with a lower percentage of whites and higher percentage of blacks in the late-onset PE group as well as greater body weight in the PE group ( Table 1 ). In Table 2 we assessed the pairwise differences between individual metabolite concentrations for controls and late-onset PE patients. A total of 17 metabolites were present in significantly different concentrations in late-onset PE vs control groups. Fourteen of these 17 metabolite concentrations were increased in the late-onset PE group while 3 were reduced compared to normals. The P values determined via a Student t test prove that for 17 of the metabolites, the individual concentration differences are significant (meaningful and reproducible) at levels determined by at P < .05. The fold changes are also presented in Table 2 . Some separation and discrimination is achieved between the cases of late-onset PE and the controls from the PCA analysis of the NMR data is shown in Figure 1 . The separation, however, did not appear dramatic. The PLS-DA analysis resulted in a detectable separation between late-onset PE group (in green ) compared to normal cases (in red ) ( Figure 2 ). Permutation testing revealed that the observed separation of the late-onset PE from the normal group was highly unlikely to be due to chance ( P < .0005). Figure 3 displays the VIP plot. Glycerol, carnitine, methylhistidine, and acetone appeared to be the most important metabolites for distinguishing late-onset PE from normal cases, based on VIP analysis. A heat map is shown on the right of the VIP plot. Red indicates that a particular metabolite concentration is increased in late-onset PE cases while green indicates reduced concentration compared to normal controls.
Parameter | Late-onset preeclampsia | Control | P value |
---|---|---|---|
No. of cases | 30 | 59 | — |
Maternal age, y, mean (SD) | 31.2 (6.4) | 30.8 (5.6) | .81 |
Racial origin, n (%) | .02 | ||
White | 14 (46.7) | 44 (74.6) | |
Black | 14 (46.7) | 14 (23.7) | |
Asian | 0 (0) | 1 (1.7) | |
Mixed | 2 (6.7) | 0 (0) | |
Nullipara, n (%) | 12 (40) | 31 (52.5) | .37 |
Weight, kg, mean (SD) | 74.9 (15.7) | 67.7 (12.2) | .03 |
Crown-rump length, mm, mean (SD) | 62.0 (9.1) | 62.7 (7.6) | .69 |
Uterine pulsatility index, MoM, mean (SD) | 1.07 (0.35) | 0.98 (0.31) | .22 |
Metabolite | Late-onset PE, mean (SD) (concentration in μmol/L) | Controls, mean (SD) (concentration in μmol/L) | P value | Fold change |
---|---|---|---|---|
No. of cases | 30 | 59 | — | — |
Glycerol | 800.7 (541.7) | 312 (296.8) | < .001 | 2.4 |
1-methylhistidine | 70.3 (40.0) | 38.9 (20.3) | < .001 | 1.7 |
Valine | 142.5 (50.6) | 121.6 (43.3) | < .05 | 1.1 |
Carnitine | 46.8 (24.8) | 27.8 (20.0) | .001 | 1.7 |
Acetone | 22.1 (11.4) | 14.9 (8.5) | .003 | 1.6 |
Trimethylamine | 6.03 (2.0) | 7.6 (3.3) | .005 | 0.88 |
Isopropanol | 10.7 (4.6) | 7.7 (4.8) | .006 | 1.4 |
Pyruvate | 83.1 (45.8) | 62.1 (24.1) | .006 | 1.3 |
Hydroxyisovalerate_3 | 6.5 (3.3) | 4.7 (2.5) | .008 | 1.4 |
Acetamide | 11.9 (7.8) | 16.1 (6.4) | .008 | 0.73 |
Glucose | 4312.9 (1783.0) | 3362.4 (765.9) | .008 | 1.2 |
Dimethylamine | 3.2 (1.7) | 4.4 (2.1) | .012 | 0.74 |
Hydroxybutyrate_2 | 28.0 (14.4) | 21.2 (7.5) | .02 | 1.3 |
Creatinine | 63.2 (16.5) | 55.1 (14.7) | .021 | 1.1 |
Creatine | 41.5 (5.9) | 33.4 (15.6) | .024 | 1.2 |
Citrate | 85.9 (26.9) | 74.1 (23.5) | .028 | 1.3 |
Hydroxybutyrate_3 | 49.9 (46.7) | 29.7 (19.1) | .038 | 1.4 |
Leucine | 114.5 (98.5) | 87.1 (61.9) | .112 | 1.2 |
Acetate | 80.6 (101.5) | 49.1 (52.5) | .12 | 1.6 |
Betaine | 33.3 (23.6) | 21.6 (9.4) | .14 | 1.5 |
Glutamine | 253.1 (131.1) | 218.5 (66.9) | .182 | 1.2 |
Ethanol | 67.7 (42.6) | 56.1 (37.2) | .19 | 1.2 |
Ornithine | 36.8 (17.4) | 42.3 (22.5) | .24 | 0.87 |
Acetoacetate | 18.9 (9.8) | 16.5 (9.6) | .27 | 1.1 |
Alanine | 366.8 (204.8) | 323.8 (151.2) | .27 | 1.1 |
Lactate | 1213.1 (564.7) | 1100.9 (689.3) | .44 | 1.1 |
Methionine | 24.7 (7.4) | 23.6 (6.5) | .48 | 1.0 |
Threonine | 157.2 (60.2) | 166.2 (62.5) | .5 | 0.94 |
Propylene glycol | 11.1 (5.0) | 11.8 (4.9) | .51 | 0.93 |
Formate | 27.0 (13.8) | 29.0 (17.8) | .6 | 1.02 |
Tyrosine | 65.1 (23.7) | 62.3 (21.7) | .6 | 1.04 |
Proline | 172.2 (57.7) | 165.7 (56.4) | .61 | 1.04 |
Serine | 148.4 (103.4) | 158.6 (92.2) | .635 | 0.93 |
Arginine | 136.3 (55.5) | 131.2 (35.9) | .65 | 1.04 |
Asparagine | 31.3 (11.3) | 32.4 (13.7) | .71 | 0.96 |
Phenylalanine | 78.0 (45.9) | 80.9 (45.4) | .78 | 0.96 |
Glycine | 238.4 (129.3) | 244.0 (115.7) | .84 | 0.97 |
Choline | 172.3 (341.5) | 185.7 (351.6) | .87 | 0.93 |
Succinate | 13.2 (13.8) | 13.4 (12.9) | .9 | 0.83 |
Malonate | 23.1 (14.3) | 23.1 (8.7) | .97 | 0.98 |
Isobutyrate | 7.6 (2.5) | 7.6 (3.2) | 1 | 1.0 |

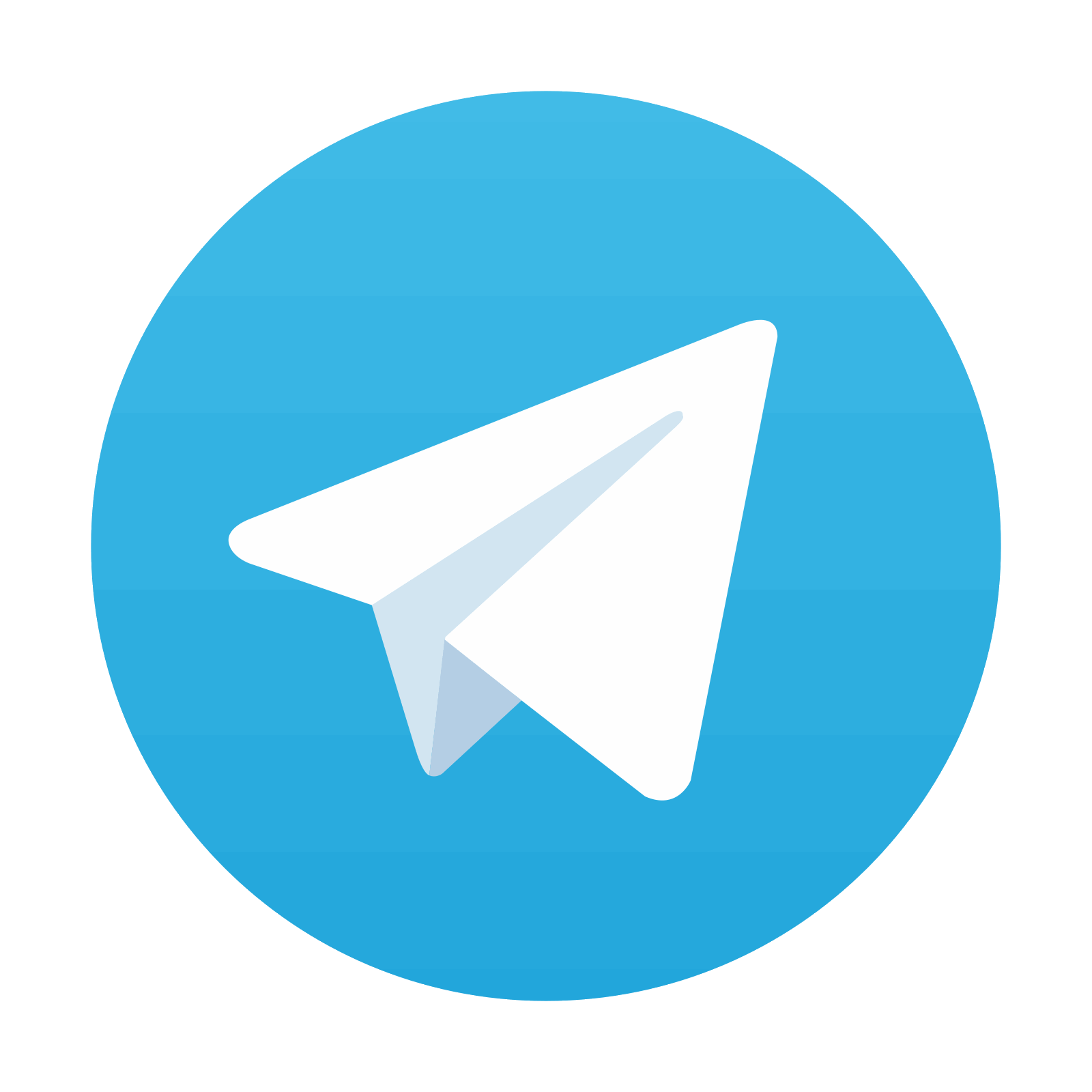
Stay updated, free articles. Join our Telegram channel

Full access? Get Clinical Tree
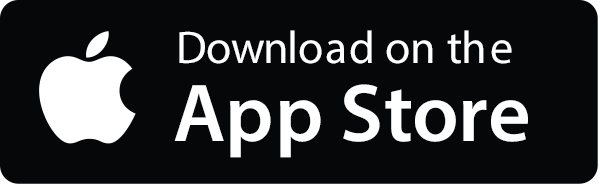

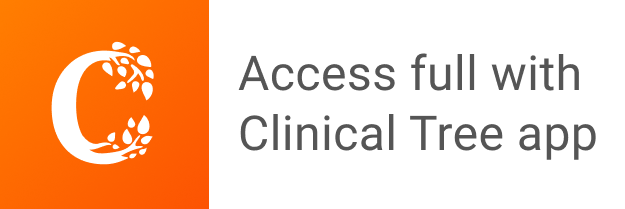