After reading this chapter you should be able to:
- •
apply evidence-based medicine to clinical practice
- •
interpret a research paper or systematic review appropriately
Evidence-based paediatrics requires the paediatrician to use the current best evidence in the assessment of clinical conditions and treatment options and to apply that to the specific needs of the individual young patient. Consequently, an understanding of the scientific evidence that supports clinical practice is one of the many important skills for the paediatrician to master, in particular the ability to read and understand both the strengths and the limitations of published studies and so identify the most appropriate management plan.
It is appropriate to develop an open and critical approach to any published work and to develop a structured review of any an article. The essence of scientific publication has always been to seek input and opinion from colleagues with knowledge and understanding of the study topic and to allow any conclusions to be supported or challenged.
A structured review used in the assessment of published research
- •
the relevance of the research question to current clinical practice
- •
clear definitions provided for study groups and parameters
- •
study topics stated clearly at the start of the trial
- •
changes to study protocol are presented and implications considered
- •
study population relevant to current clinical practice
- •
study population correctly identified, recruited and recruitment bias avoided
- •
presence of appropriate controls
- •
sample size correctly calculated to answer the initial question
- •
appropriate outcomes measured rather than surrogate markers
- •
appropriate statistical tests used
- •
appropriate conclusions drawn
Study design
Publications of studies follow a standard format and present the background to the issue of interest, the methodology to be used, the results obtained, the outcomes discussed and the references used.
A recognition of the numerous formats that are used in obtaining data in a published study is an important first step in understanding the strengths of the conclusions drawn. The ‘Hierarchy of Evidence’ ranks study design formats and their potential for bias or systematic error.
Level | Type of evidence |
---|---|
I | Systematic reviews and metaanalyses of RCTs with definitive results |
II | RCTs with definitive results (confidence intervals that do not overlap) |
III | RCTs with nondefinitive results (clinically significant effect but with confidence intervals overlapping the threshold for this effect) |
IV | Cohort studies |
V | Case-control studies |
VI | Cross sectional surveys |
VII | Case reports |
Systematic Reviews are a collation and summary of all evidence in a particular area and include metaanalyses where results from different studies are reanalysed together. They provide an improvement in the assessment of a topic of interest by increasing the size of the populations studied and thereby provide a greater precision and generalisability than single studies. They also use predefined and transparent criteria in the collection and analysis of the combined information. The quality—that is the risk bias—of included studies is evaluated, with particular assessment of the methods of subject selection, randomisation and blinding.
Randomised control trial (RCT). Subjects are randomly assigned to one of two groups (experimental versus control) with the only expected difference being the outcome studied, and consequently these types of studies provide the most reliable evidence on the effectiveness of interventions. In a blind RCT study, the participants in the clinical trial do not know if they are receiving the placebo or the real treatment, whilst in a double-blind study, neither the participants nor the researchers are aware of which group of individuals are to receive the placebo and which are to receive the experimental treatment. This aims to minimise the placebo effect and bias.
Crossover study. This is a variation of the RCT where all subjects receive all treatment options consecutively but the order in which these treatments are offered is randomised. There may even be multiple phases in the study with differing treatment options, and in such studies each patient will act as their own control. Crossover studies are useful when recruitment to a trial may leave some participants not being offered a new trial medication and so making recruitment difficult.
Cohort studies are used to study incidence, causes and prognosis. They are usually prospective in nature and follow individuals with a particular condition or who receive a particular treatment and they are compared with another group of people who are not affected by the condition or who did not receive the treatment. Longitudinal studies are required for questions about prognosis.
Case-control studies can be considered retrospective. They begin with the outcomes and do not follow people over time. Researchers choose individuals with a particular characteristic (the cases) and compare features of interest with a control group of individuals who do not have the characteristic. This type of study is required for questions around causation.
Lower-level designs include cross-sectional studies where a defined population is observed at a single point in time or at certain time intervals, case reports and expert opinions.
Methods
Statistical and sample size
Statistical power refers to the ability to identify an effect that is present in a population using a test based on a sample from that population. It is directly linked to sample size and too few patients may mean there is not enough power to detect a difference of interest. Complex and formal power calculations need to be undertaken prior to the start of a study to ensure that it has sufficient statistical power to determine any differences between groups.
incidence | an outcome that occurs infrequently requires many more patients in order to detect a difference |
population variance | the greater the variance (standard deviation) in the population then the more patients are needed to demonstrate a significant difference |
treatment effect size | a small difference in any effect between groups or treatments needs a higher number of subjects to allow identification of those differences. the opposite is true |
alpha type-I error | refers to the probability of finding a difference when a difference does not exist. The most common alpha cut-off used is 5% (often seen as p ≤ 0.05), indicating a 5% chance that a significant difference is actually due to chance and is not a true difference |
beta type-II error | refers to the probability of not detecting a difference when one actually exists. Beta is directly related to study power. Most research uses a beta cutoff of 20% (0.2), indicating a 20% chance that a significant difference is missed |
Bias
The interpretation or appraisal of outcomes requires consideration as to whether the outcome is truly due to the treatment or intervention or whether other explanations are possible. Systematic errors may be introduced into sampling or testing and lead to various types of bias.
Research bias can compromise the validity of the interpretation of outcomes, leading to the exclusion of a true effect or the acceptance of a false one. Bias can be divided into:
- •
selection bias
- •
information bias
- •
confounding variables
Selection bias is linked to the recruitment of patients; a cohort of individuals deciding not to participate in the study could lead to under- or overestimation of a particular effect or risk. Patients lost to follow-up, self-selection into a study and lack of randomisation are further examples.
Information bias occurs when patients are misclassified, for example, when individuals with a particular disease or exposure are classified as nondiseased or nonexposed due to an inaccuracy of diagnostic tests. Such a problem will invalidate outcomes .
Confounders are variables that influence both the dependent variable and independent variable, causing a spurious association. Confounding variables are those that may compete with the exposure of interest (e.g. treatment) in explaining the outcome of a study. For example, in a study about whether a lack of exercise (independent variable) leads to weight gain (dependent variable) in children, the number of calories consumed would be a confounding variable. Identifying possible confounders early on and controlling for them will assist in eliminating bias.
- •
well-designed research protocols
- •
randomised selection of patients
- •
random allocation to groups
- •
blind or double blind
- •
balance confounding variable across groups
- •
maximising follow up
- •
analysing randomised controlled trial data on ‘intention to treat’ principle
Data analysis
Statistical tests
The most commonly used statistical tests are shown along with the appropriate conditions under which they should be used. They can generally split into:
- •
tests of difference—comparing means
- •
tests of relationships—strength of correlations between variables
It is important to understand the basis and appropriateness of tests used in analysing the collected data.
Tests of difference | ||
---|---|---|
Tests used to: | Parametric data | Non-parametric data |
compare two paired (related) groups | t-test (paired) | Wilcoxon signed rank |
compare three or more unmatched (independent) groups | ANOVA | Kruskal–Wallis |
compare three or more matched (related) groups/conditions | ANOVA (repeated measures) | Friedman’s ANOVA |
investigate relationship between two variables | Pearson’s correlation | Spearman’s rank |
Tests of relationship | ||
---|---|---|
Tests should | Parametric data | Non-parametric data |
investigate relationship between two variables | Pearson’s correlation | Spearman’s rank |
predict a value from other measured variables (relationships between) | Logistic regression (category variable) or Linear regression (continuous variable) | Nonparametric regression (less used) |
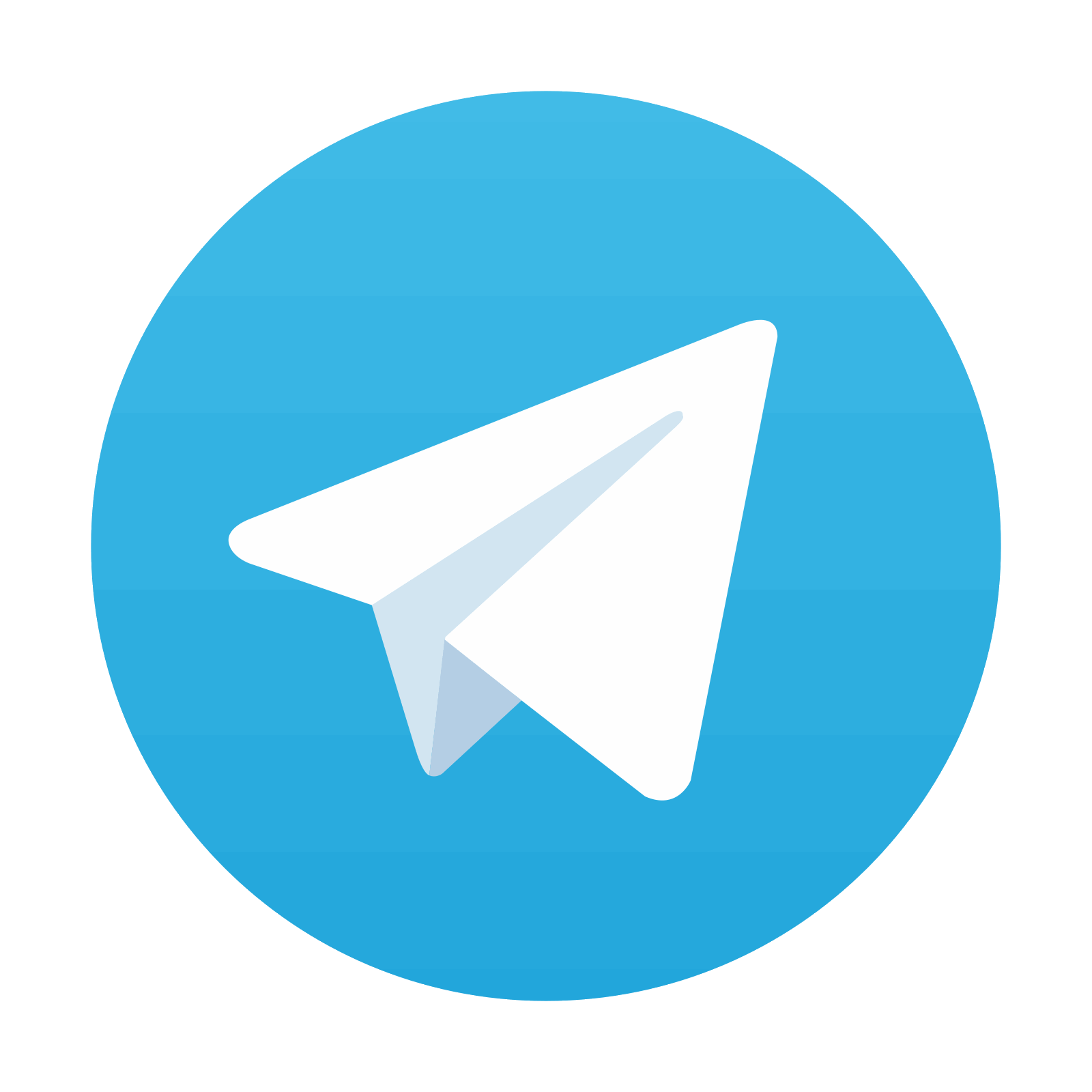
Stay updated, free articles. Join our Telegram channel

Full access? Get Clinical Tree
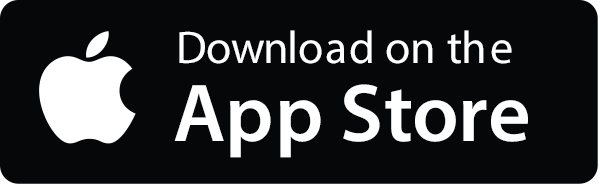

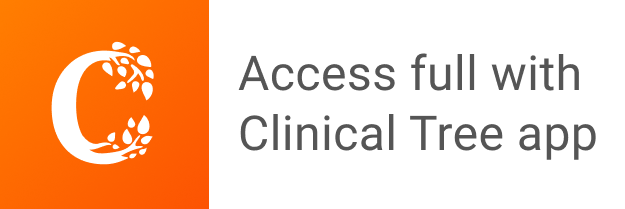