Background
The analysis of circulating microparticles in pregnancy is of revolutionary potential because it represents an in vivo biopsy of active gestational tissues.
Objective
We hypothesized that circulating microparticle signaling will differ in pregnancies that experience spontaneous preterm birth from those delivering at term and that these differences will be evident many weeks in advance of clinical presentation.
Study Design
Utilizing plasma specimens obtained between 10 and 12 weeks’ gestation as part of a prospectively collected birth cohort in which pregnancy outcomes are independently validated by 2 board-certified maternal-fetal medicine physicians, 25 singleton cases of spontaneous preterm birth ≤ 34 weeks were matched by maternal age, race, and gestational age of sampling (±2 weeks) with 50 uncomplicated term deliveries. Circulating microparticles from these first-trimester specimens were isolated and analyzed by multiple reaction monitoring mass spectrometry for potential protein biomarkers following previous studies. Markers with robust univariate performance in correlating spontaneous preterm birth were further evaluated for their biological relevance via a combined functional profiling/pathway analysis and for multivariate performance.
Results
Among the 132 proteins evaluated, 62 demonstrated robust power of detecting spontaneous preterm birth in a bootstrap receiver-operating characteristic curve analysis at a false discovery rate of < 20% estimated via label permutation. Differential dependency network analysis identified spontaneous preterm birth-associated coexpression patterns linked to biological processes of inflammation, wound healing, and the coagulation cascade. Linear modeling of spontaneous preterm birth using a multiplex of the candidate biomarkers with a fixed sensitivity of 80% exhibited a specificity of 83% with median area under the curve of 0.89. These results indicate a strong potential of multivariate model development for informative risk stratification.
Conclusion
This project has identified functional proteomic factors with associated biological processes that are already unique in their expression profiles at 10-12 weeks among women who go on to deliver spontaneously ≤ 34 weeks. These changes, with further validation, will allow the stratification of patients at risk of spontaneous preterm birth before clinical presentation.
Preterm birth is a leading cause of neonatal morbidity and death in children younger than 5 years of age, with deliveries at the earlier gestational ages exhibiting a dramatically increased risk. Compared with infants born after 38 weeks, the composite rate of neonatal morbidity doubles for each earlier gestational week of delivery.
In 2005, the cumulative annual expense of preterm birth within the Unites States was estimated to be in excess of $26.2 billion. Approximately two thirds of preterm births are spontaneous, meaning they are not associated with medical intervention, in nature. Yet despite the compelling nature of this condition, there has been little recent advancement in our understanding of the etiology of spontaneous preterm birth (SPTB). Although there is an increasing consensus that SPTB represents a syndrome rather than a single pathologic entity, it has been both ethically and physically difficult to study the pathophysiology of the uteroplacental interface.
The evolving field of circulating microparticle (CMP) biology may offer a solution to these difficulties because these particles present a sampling of the uteroplacental environment. Additionally, studying the contents of these particles holds the promise of identifying novel blood-based, and possibly clinically useful, biomarkers.
Microparticles are membrane-bound nanovesicles that range in size from 50 to 300 nm and shed by a wide variety of cell types. Microparticle nomenclature varies, but typically microparticles between 50 and 100 nm are called exosomes, and those > 100 nm are termed microvesicles, and other terms, such as microaggregates, are often used in literature. Unless otherwise stated, we will use the term microparticle as a general reference to all of these species.
Increasingly, microparticles are recognized as important means of intercellular communication in physiological, pathophysiological, and apoptotic circumstances. Although the contents of different types of microparticles vary with cell type and their expression, they can include nuclear, cytosolic, and membrane proteins as well as lipids and messenger and microribonucleic acids. Their contents hold information regarding the state of the cell type origin at the time of microparticle expression; thus, they represent a unique window in real time into the activities of cells, tissues, and organs that might otherwise be remote to sampling.
A high proportion of adverse pregnancy outcomes have their pathophysiological origins at the uteroplacental interface in early pregnancy. The ability to understand protein signaling and the state of associated tissue and cell populations may be predictive of impending complications. Our present analysis will demonstrate the ability to capture informative, microparticle-related, protein signaling at the end of the first trimester (mean, 11 weeks). We will further demonstrate that this signaling discriminates between pregnancies delivering at gestational ages marked by considerable neonatal morbidity (≤ 34 weeks) compared with those delivering at term.
Materials and Methods
Clinical specimen collection
Maternal K 2 -EDTA plasma samples (10-12 weeks’ gestation) were obtained and stored at –80°C at Brigham and Women’s Hospital (BWH) (Boston, MA) between 2009 and 2014 as part of the prospectively collected LIFECODES birth cohort.
Eligibility criteria included patients who were ≥ 18 years of age, initiated their prenatal care at ≤ 15 weeks of gestation, and planned on delivering at the BWH. Exclusion criteria included preexisting medical disorders (preexisting diabetes, gestational diabetes, autoimmune disorders, current cancer diagnosis, human immunodeficiency virus, and hepatitis) and fetal anomalies and was restricted to singleton gestations.
Gestational age of pregnancy was confirmed by ultrasound scanning ≤ 12 weeks’ gestation. If consistent with the last menstrual period dating, the last menstrual period was used to determine the due date. If not consistent, then the due date was set by the earliest available ultrasound. Full-term birth was defined as ≥37 weeks of gestation, and preterm birth for the purposes of this investigation was defined as SPTB ≤ 34 weeks. The lower limit of the gestational age was set at 23 weeks.
All cases were independently reviewed and validated by 2 board-certified maternal-fetal medicine physicians. When disagreement in pregnancy outcome or characteristic arose, the case was re-reviewed and a consensus conference held to determine the final characterization. Twenty-five singleton cases of SPTB ≤ 34 weeks (n = 8 preterm labor, n = 1 2 premature rupture of membranes, n = 5 cervical insufficiency) were matched to 2 control term deliveries by maternal age, race, and gestational age of sampling (±2 weeks).
The protocol was approved by institutional review board at BWH, and written informed consent was obtained from all participating women.
CMP enrichment
Plasma samples were shipped on dry ice to the David H. Murdock Research Institute (Kannapolis, NC) and randomized by NX Prenatal Inc to blind laboratory personnel performing sample processing and testing to case/control status. CMPs were enriched by size exclusion chromatography and isocratically eluted using the NeXosome elution reagent (in-house proprietary reagent). Briefly, PD-10 columns (GE Healthcare Life Sciences, Indianapolis, IN) were packed with 10 mL of 2% agarose bead standard (pore size, 50–150 um) from ABT (Miami, FL), washed with elution reagent, and stored at 4°C for a minimum of 24 hours and no longer than 3 days prior to use.
On the day of use, the columns were again washed and 1 mL of thawed neat plasma sample was applied to the column. The circulating microparticles were captured in the column void volume, partially resolved from the high abundant protein peak. The samples were processed in batches of 15–20 across 4 days to minimize variability between processing individual samples. One aliquot of the pooled CMP column fraction from each clinical specimen, containing 200 μg of total protein (determined by bicinchoninic assay) was transferred to a 2 mL microcentrifuge tube and shipped on dry ice to Biognosys (Zurich, Switzerland) for proteomic analysis.
Liquid chromatography–mass spectrometry
Quantitative proteomic liquid chromatography–mass spectrometry analysis was performed by Biognosys AG. Briefly, for each sample a total of 20 μg of protein was lyophilized and then denatured with 8 M urea, reduced using dithiothreitol, alkylated with Biognosys alkylation solution, and digested overnight with trypsin (Promega, Madison, WI) as previously described. Resulting sample peptides were dried using a SpeedVac system and redissolved in 45 μL of Biognosys LC solvent and mixed with Biognosys PlasmaDive (extended version 2.0) stable isotope-labeled reference peptide mix containing the Biognosys iRT kit.
Then 1 μg of total protein was injected to an in-house packed C18 column (75 μm inner diameter and 10 cm column length; New Objective, Woburn, MA); the column material was Magic AQ, 3 μm particle size, 200 Å pore size from Michrom, Auburn, CA) on a Easy nLC nano–liquid chromatography system (Thermo Scientific, Waltham, MA).
Liquid chromatography–multiple reaction monitoring (LC-MRM) assays were measured on a Thermo Scientific TSQ Vantage triple-quadrupole mass spectrometer equipped with a standard nanoelectrospray source. The liquid chromatography gradient for LC-MRM was 5-35% solvent B (97% acetonitrile in water with 0.1% formic acid) for 30 minutes followed by 35-100% solvent B for 2 minutes and 100% solvent B for 8 minutes (total gradient length was 40 minutes).
For quantification of the peptides across samples, the TSQ Vantage (Thermo Scientific) was operated in scheduled multiple reaction monitoring mode with an acquisition window length of 3.25 minutes. The liquid chromatography eluent was electrosprayed at 1.9 kV and Q1 was operated at unit resolution (0.7 Da). Signal processing and data analysis were carried out using SpectroDive Biognosys’ proprietary software for multiplexed multiple reaction monitoring data analysis. A Q-value filter of 1% was applied. Protein concentration was determined based on the normalized 1 μg of protein injected to the liquid chromatography–mass spectrometry.
Statistical analysis
To select informative analytes that differentiate SPTB from term deliveries, the processed protein quantitation data were first subjected to univariate receiver-operating characteristic (ROC) curve analysis. Bootstrap resampling against nulls from sample label permutation was used to control the false-discovery rate (FDR). Briefly, for each protein, an ROC analysis was repeated on bootstrap samples from the original data, and the mean and SD of the area under the curve (AUCs) was estimated. The bootstrap procedure was then applied on the same data again but with sample SPTB status labels randomly permutated.
The permutation analysis provided the null results to control the FDR and adjust for multiple comparison during the selection of potential protein biomarkers. The Differential Dependency Network (DDN) bioinformatic tool was then applied to extract SPTB phenotype-dependent high-order coexpression patterns among the proteins. An additional bioinformatic tool, BiNGO, was used to identify gene ontology (GO) categories that were overrepresented in the DDN subnetworks to suggest plausible functional links between the observed proteomic dysregulations and SPTB. Finally, to assess the complementary values among the selected proteins and the range of their potential clinically relevant performance, multivariate linear models were derived and evaluated using bootstrap resampling.
Results
The demographic and clinical characteristics of the sample set are presented in Table 1 . Maternal age, race, body mass index, use of public insurance, smoking during pregnancy, and gestational age at enrollment were similar in both groups. Maternal educational levels were higher in the controls, and a greater proportion of the SPTB cases were primaparious and had a prior history of PTB.
Characteristic | SPTB (n = 25) n, %, or mean (SD) | Controls (n = 50) n, %, or mean (SD) | P value a |
---|---|---|---|
Maternal age, y | 32.8 (7.3) | 31.6 (5.8) | .44 |
Race | .10 | ||
White | 8 (32.0%) | 23 (46.0%) | |
African-American | 3 (12.0%) | 5 (10.0%) | |
Hispanic | 8 (32.0%) | 18 (36.0%) | |
Asian | 3 (12.0%) | 2 (4.0%) | |
Other | 3 (12.0%) | 2 (4.0%) | |
Maternal BMI, kg/m 2 | 29.3 (6.9) | 27.3 (7.4) | .17 |
Maternal education | .004 | ||
Less than high school | 3 (12.0%) | 0 (0.0%) | |
High school/equivalent | 1 (4.0%) | 0 (0.0%) | |
More than high school | 21 (84.0%) | 50 (100.0%) | |
On public insurance | 14 (28.0%) | 10 (40.0%) | .31 |
Primiparous | 13 (52.0%) | 14 (28.0%) | .04 |
Smoked during pregnancy | 4 (8.0%) | 1 (4.0%) | .66 |
Prior history of preterm birth | 7 (28.0%) | 3 (6.0%) | .01 |
Enrollment gestational age | 11.7 (3.0) | 11.6 (3.0) | .99 |
Gestational age at delivery | 31.9 (2.9) | 39.4 (0.9) | < .001 |
a P values were calculated with a Wilcoxon rank sum test, χ 2 test, Fisher exact test, or ANOVA where appropriate.
The 132 proteins evaluated via targeted MRM were individually assessed for ability to differentiate SPTB from term deliveries. By requiring that the mean bootstrap AUCs for each candidate protein be significantly greater than the null (> mean + SD of mean bootstrap AUCs estimated with label permutation; see Figure 1 ) and excluding proteins with large bootstrap AUCs variances, 62 of the 132 proteins demonstrated robust power for the detection of SPTB.
In contrast, using the same criteria with sample label permutation, only 12 proteins would have been selected. The estimated FDR for protein selection was therefore < 20% (12 of 62). These 62 proteins were considered potential candidates for further multivariate analysis. Individually, 25 of the 62 proteins had a value of P < .10 and an AUC > 0.618 for differentiating SPTB from term controls ( Table 2 ).
Protein | P value | AUC |
---|---|---|
AACT | .003 | 0.715 |
KLKB1 | .013 | 0.678 |
APOM | .015 | 0.674 |
ITIH4 | .024 | 0.662 |
IC1 | .034 | 0.651 |
KNG1 | .035 | 0.650 |
TRY3 | .048 | 0.644 |
CO9 | .051 | 0.639 |
F13B | .058 | 0.635 |
APOL1 | .060 | 0.634 |
LCAT | .062 | 0.633 |
PGRP2 | .067 | 0.631 |
THBG | .072 | 0.628 |
FBLN1 | .073 | 0.628 |
ITIH2 | .073 | 0.628 |
CD5L | .075 | 0.627 |
CBPN | .077 | 0.626 |
VTDB | .082 | 0.624 |
AMBP | .087 | 0.622 |
CO8A | .087 | 0.622 |
ITIH1 | .089 | 0.622 |
TTHY | .095 | 0.619 |
F13A | .097 | 0.619 |
APOA1 | .100 | 0.618 |
HPT | .100 | 0.618 |
Differential dependency network analysis among the 62 selected proteins identified a number of SPTB phenotype-associated coexpression patterns ( Figure 2 ). A number of GO categories, such as inflammation, wound healing, the coagulation cascade, and steroid metabolism, were overrepresented among the DDN analysis coexpression subnetworks. Table 3 provides a listing of the top discriminating pairwise correlations ( P < .001-.069). There were a total of 20 unique proteins that formed the DDN subnetworks. Several of the pairwise correlations (CBPN-TRFE, CPN2-TRFE, A1AG1-MBL2) were markers for inclusion in the term controls rather than the SPTB cases, which suggest a protection against SPTB ( Table 3 ).
Node (protein) 1 | Node (protein) 2 | Coexpressed in phenotype | P value |
---|---|---|---|
A2AP | SEPP1 | SPTB | < .001 |
CBPN | TRFE | TERM | < .001 |
CPN2 | TRFE | TERM | < .001 |
HEMO | THBG | SPTB | .002 |
A2MG | F13B | SPTB | .003 |
IC1 | TRFE | SPTB | .003 |
KAIN | MBL2 | SPTB | .004 |
A2GL | LCAT | SPTB | .005 |
A2MG | CO6 | SPTB | .005 |
CHLE | SEPP1 | SPTB | .009 |
MBL2 | PGRP2 | SPTB | .022 |
KLKB1 | SEPP1 | SPTB | .045 |
A1AG1 | MBL2 | TERM | .064 |
PGRP2 | SEPP1 | SPTB | .066 |
A1AG1 | FBLN1 | SPTB | .069 |
Based on the available sample size and to avoid overtraining, only linear models were evaluated to assess the clinically relevant performance, and the variables were limited to all possible combinations of 2 or 3 proteins of the 20 proteins in Table 3 (1330 models). Each model was derived and evaluated using 200 bootstrap resampled data to estimate the median (90% confidence interval [CI]) and specificity for ROC AUCs with a fixed sensitivity of 80%. The top 20 models in terms of the lower bound of 90% CI of AUCs and specificities are listed in Tables 4 and 5 , respectively. Given the limitations imposed by the sample size, the model could not be tested on an independent sample set. To compensate for this, the CIs for the panel’s performances in the training data set were estimated through iterative bootstrap analysis.
Panel | Specificity at 80% sensitivity Median (90% CI) | AUC Median (90% CI) |
---|---|---|
A2MG HEMO MBL2 | 0.830 (0.654–0.935) | 0.892 (0.829–0.949) |
HEMO IC1 KLKB1 | 0.842 (0.666–0.927) | 0.892 (0.824–0.942) |
A2MG HEMO KLKB1 | 0.812 (0.634–0.933) | 0.879 (0.819–0.945) |
A1AG1 A2MG HEMO | 0.824 (0.666–0.940) | 0.887 (0.815–0.943) |
A1AG1 A2MG CO6 | 0.800 (0.630–0.922) | 0.876 (0.814–0.932) |
F13B HEMO KLKB1 | 0.808 (0.643–0.907) | 0.878 (0.810–0.931) |
IC1 KLKB1 TRFE | 0.837 (0.680–0.939) | 0.882 (0.808–0.943) |
HEMO IC1 LCAT | 0.825 (0.653–0.932) | 0.879 (0.808–0.938) |
KLKB1 LCAT TRFE | 0.830 (0.683–0.935) | 0.870 (0.807–0.943) |
A1AG1 KLKB1 TRFE | 0.804 (0.630–0.919) | 0.876 (0.806–0.935) |
A1AG1 HEMO KLKB1 | 0.808 (0.659–0.918) | 0.872 (0.805–0.931) |
A2MG KLKB1 TRFE | 0.811 (0.632–0.932) | 0.878 (0.804–0.937) |
CPN2 HEMO KLKB1 | 0.804 (0.630–0.922) | 0.871 (0.803–0.936) |
A2GL A2MG HEMO | 0.796 (0.543–0.923) | 0.872 (0.803–0.933) |
HEMO KLKB1 PGRP2 | 0.800 (0.637–0.939) | 0.873 (0.801–0.932) |
HEMO KLKB1 LCAT | 0.816 (0.674–0.940) | 0.874 (0.801–0.944) |
A2AP KLKB1 TRFE | 0.821 (0.666–0.927) | 0.865 (0.800–0.947) |
KLKB1 LCAT PGRP2 | 0.808 (0.667–0.918) | 0.872 (0.798–0.939) |
A2MG LCAT TRFE | 0.823 (0.619–0.928) | 0.871 (0.798–0.934) |
A1AG1 HEMO IC1 | 0.802 (0.500–0.898) | 0.861 (0.797–0.921) |
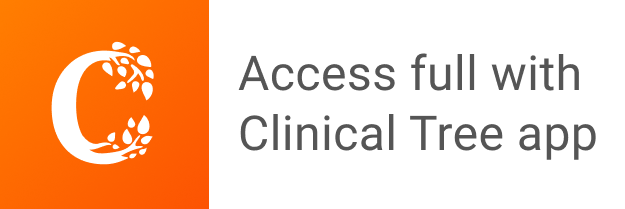