Epidemiology of Adverse Drug Effects
Louis Vernacchio
Allen A. Mitchell
Introduction
The practice of rational drug therapy requires weighing the benefits expected from the use of a given drug against its risks. Unfortunately, this ideal circumstance is rarely achieved because no matter how much we know about a drug’s benefit, we often have an inadequate understanding about the frequency and severity of its adverse effects.
Historically, the lack of knowledge about adverse drug effects has been especially acute in pediatrics because of a relative scarcity of data from children. In many cases, drugs approved for adults have become widely used in children without careful consideration of potential adverse effects that may occur in the pediatric population. The growth and development that characterize children make them vulnerable to adverse outcomes unique to childhood, and often, to specific stages of childhood. The possibility of such alterations of growth, cognitive development, or specific organ development may not be appreciated in adult experience with a given drug but can have significant impact on children. Examples are numerous and include inhibition of linear growth by corticosteroids (1), tooth enamel dysplasia caused by tetracycline antibiotics (2), the association between erythromycin use and pyloric stenosis in young infants (3), and concern (still controversial) about deleterious effects of fluoroquinolones on cartilage development in prepubescent children (4). In addition to adverse outcomes that are unique to childhood, consideration must be given to the fact that children may metabolize certain drugs differently than adults, potentially leading to an increased risk of adverse effects. Chloramphenicol-induced “gray baby syndrome,” in which delayed hepatic metabolism in infants leads to toxicity, is a classic example of this phenomenon (5,6).
That adverse drug reactions (ADRs) were indeed common and sometimes serious in the pediatric population was first demonstrated by systematic studies of ADRs in children that began in the 1970s (7,8,9,10,11,12,13). Since then, a substantial body of international literature on pediatric ADRs has confirmed and expanded these observations. In this chapter, we will focus on an epidemiologic approach to the study of adverse drug effects in children, reviewing both theoretical considerations and practical study approaches. We will attempt to emphasize aspects that are unique or especially relevant to pediatrics, using historical and current examples to illustrate the key points.
Theoretical Considerations
Whether from the clinical or epidemiologic perspective, three broad areas of information must be considered in the study of adverse drug effects: the drug of interest (the “exposure”), the adverse reaction to the drug (the “outcome”), and the nature of the patients who experience these effects (the “population”).
Exposure
Although the concept of exposure is straightforward, the definitions of what constitutes a drug and what constitutes an exposure are not always apparent. For example, few would quarrel with including in the definition oral and parenteral exposure to an antibiotic, but does drug exposure also include such common substances as intravenous fluids, vitamins, and oxygen? Furthermore, does the route of exposure (e.g., topical or by inhalation) affect the definition? These distinctions have important implications. As one example, because of varying definitions, three studies describing drug use in the newborn intensive care nursery (two from the same population) differed with respect to the number of drugs used in the patients, ranging from and average of 3.4 to 10.4 (13,14,15). Variations in the definition of what constitutes a drug exposure will clearly affect not only estimates of drug use but also the observed rates of ADRs in a given population.
Those who care for children must also consider another kind of drug exposure—the so-called inactive ingredients. These agents are included in a variety of medications to increase stability, solubility, shelf life, and the like. Although it is recognized that certain ingredients, such as sulfites and tartrazine, can produce adverse effects in sensitive
individuals, observations from neonatal intensive care units have revealed that patients may suffer serious and even fatal reactions from such “inactive” ingredients as benzyl alcohol and propylene glycol (16,17). Unfortunately, agents added to active pharmaceuticals vary over time and manufacturers, and pharmacists, nurses, and physicians alike are usually unaware of the number and nature of “inactive” ingredients they are administering to patients (18). Obviously, lack of awareness about such exposures limits the likelihood of detecting their adverse effects.
individuals, observations from neonatal intensive care units have revealed that patients may suffer serious and even fatal reactions from such “inactive” ingredients as benzyl alcohol and propylene glycol (16,17). Unfortunately, agents added to active pharmaceuticals vary over time and manufacturers, and pharmacists, nurses, and physicians alike are usually unaware of the number and nature of “inactive” ingredients they are administering to patients (18). Obviously, lack of awareness about such exposures limits the likelihood of detecting their adverse effects.
A recently recognized area of concern in the pediatric population is the potential for adverse effects from “natural” products including herbs, megavitamins, and other dietary supplements. The prevalence of use of such products among children in the United States is unclear, with estimates for recent use ranging from as low as 2% to as high as 45%, depending on the population studied and the methodology used (19,20,21,22,23,24). What is not debatable is that herbal and other “natural” products have the potential for serious adverse effects in children. For example, a number of cases of sudden cardiac death and stroke have been linked to ephedra use, an herbal drug present in many diet aids, “energy” drinks, and other products specifically marketed to children (25). Less serious ADRs, such as allergic reactions and erythema nodosum linked to echinacea, have importance both because of their inherent effects and because they could be mistakenly attributed to the child’s illness or to “conventional” treatments that are used concurrently (26,27,28).
Certainly, in terms of surveillance for potential adverse effects, a broad variety of substances and routes of administration should be considered in the definition of drug exposures. This may be of particular concern in pediatrics. The association between excessive inhaled oxygen and retinopathy in premature infants is but one dramatic example in pediatrics of the potential for a serious ADR from an intervention that, at first glance, many may not even be classified as a drug exposure (29,30).
Outcome
What outcomes are considered to be adverse drug effects? Much of the debate (and confusion) centers around the definition of the term “adverse drug reaction.” The World Health Organization, for example, defines ADR as a response to a drug that is noxious and unintended and that occurs at doses used in humans for prophylaxis, diagnosis, or therapy (31). Although the World Health Organization definition does not exclude events that may be associated with a patient’s disease state, others may exclude such events. Most definitions include noxious or pathological signs and symptoms, but do they also consider, for example, abnormalities in laboratory values in the absence of symptoms? Would asymptomatic hyperkalemia be considered an adverse reaction to potassium supplements? Many question whether certain signs and symptoms should be included as adverse reactions, particularly where they may be common, trivial, or unavoidable consequences of a drug’s pharmacologic action (e.g., drowsiness with antihistamines, mild diarrhea with ampicillin, and leukopenia with cytotoxic drugs). Some would consider as ADRs only those outcomes requiring a change in drug therapy. Because few studies use a common definition of an ADR, one must be wary about making comparisons among different studies. As was the case for exposures, we prefer a broad definition of ADRs (as long as one does not give undue importance to the “total ADR rate” derived from such a definition), since it facilitates study of the broad spectrum of drug-related events.
Another source of confusion results from failure to make clear distinctions between effects of drugs that become manifest after short-term use and effects that become apparent only after long-term use. Acute effects following short-term exposure often involve allergic or hypersensitivity reactions (e.g., serum sickness with cefaclor), idiosyncratic responses (e.g., extrapyramidal signs due to phenothiazines), or extensions of a drug’s known pharmacology (e.g., arrhythmias due to digitalis). Effects following long-term administration can occur in a precipitate manner (e.g., gastrointestinal bleeding from aspirin) or can have a more insidious onset (e.g., cataracts with corticosteroids); some effects may involve a latent interval, becoming apparent long after the drug exposure has ceased (e.g., adenocarcinoma of the vagina in adolescent females exposed in utero to diethylstilbestrol). A classification based on the temporal relation between duration of therapy and onset of adverse effect is particularly useful in considering various strategies for evaluating the full spectrum of ADRs.
Few ADRs represent unique clinical events. As a result, signs or symptoms that might be attributed to drug therapy by one observer might as easily be attributed to the patient’s underlying disease state by another. For example, a rash occurring when amoxicillin is used to treat acute otitis media may be attributed either to the amoxicillin or to an underlying viral infection; lethargy in a patient with recurrent seizures may be attributed to the anticonvulsant used to control the seizures or to a postictal state. It is important to recognize that even healthy individuals who are receiving no medications will frequently report symptoms that are commonly considered side effects of drugs, such as fatigue, headache, and rash (32).
Reports in the mid-1970s described the difficulties inherent in attempts to establish valid and reproducible systems for implicating a particular drug in a specific adverse event (33,34). Since then, a number of researchers devised various algorithms to formally assess causality in suspected ADRs (35,36,37). Unfortunately, these approaches rely heavily on current information about a drug and its side effects, and they are therefore unlikely to facilitate discovery of new, previously unrecognized ADRs; in fact, by relying on current information about a drug’s effects, some schemes may tend to discourage such discovery. Furthermore, effective use of the algorithms requires the availability of information (such as the results of withdrawal and rechallenge) that is often unavailable in the usual context of clinical practice. Critical assessment of specific algorithms has suggested that they have limited utility (38,39), and it is unlikely that these approaches will have major value in furthering our understanding of diverse ADRs. On the other hand, algorithms do identify the kinds of questions that must be answered to assess causality, and for this reason they can indeed prove helpful by highlighting appropriate questions in the clinician’s
assessment of a particular adverse event in a particular patient.
assessment of a particular adverse event in a particular patient.
Population
The third area of information needed for the study of ADRs concerns the population of patients being treated. Such information provides critical insight into both the nature of the ADRs and risk factors for their occurrence. For example, age is especially important in pediatrics as it may affect the risk of particular reactions; it is uninformative, for example, to describe the risk of sulfonamide-induced kernicterus in the entire pediatric age range, since this particular outcome is limited to newborn infants. The diseases for which patients receive drugs also affect the risk of experiencing an ADR; patients with cancer are far more likely to receive highly toxic drugs, and therefore have life-threatening adverse reactions than are other patients with less serious illnesses.
In addition to demographic factors, proper assessment of drug-effect relationships invariably requires a detailed understanding of the drug and outcome under consideration. For example, in a study of aspirin and Reye syndrome, one would be interested in whether the preceding illnesses (e.g., influenza) that prompted the aspirin use might distinguish those adversely affected by the exposure from those who were not. In a study of valproate-associated hepatotoxicity, one would wish to know whether valproate-treated epileptic patients differed from those who were treated with alternate drugs; for example, if valproate were given as a drug of “last resort,” it may preferentially be administered to those who suffered ADRs on other anticonvulsants and who, therefore, may be at increased risk for ADRs on valproate.
Estimating Rates of Adverse Drug Reactions
One of the most important aspects of ADRs is the frequency, or rate, with which they occur. In epidemiologic terms, rate refers to the number of people with the outcome of interest (the numerator) divided by the population at risk (the denominator), over a specified time. When applied to the study of ADRs, the numerator is the number of patients with reactions to a given drug, and the denominator is the number of patients exposed to that drug. The importance of the denominator is often overlooked; if penicillin-induced anaphylaxis is observed in 10 patients, the rate of this event cannot be determined unless the number of patients exposed to penicillin is also known. In statements reflecting rates of ADRs, the time reference is often implied and commonly refers to the period of drug exposure (e.g., the number of cases of anaphylaxis occurring during the course of penicillin therapy). However, for reactions that may occur after drug exposure has ceased, one must be particularly cautious that the time periods are appropriate in both the numerator and the denominator.
Finally, recall that the definition of the denominator refers to the population at risk for the reaction. Thus, although all penicillin-exposed patients might constitute an appropriate denominator in a consideration of anaphylaxis, since all exposed patients are at risk for anaphylaxis, the denominator for consideration of cyclophosphamide-induced azoospermia would not be all patients but only postpubescent males.
Statistical Considerations
When a certain adverse event is observed in an at-risk population, a critical question arises as to whether that event should be attributed to the drug. In some settings, such as those involving acute events such as anaphylaxis, attribution may be straightforward. However, attribution is not straightforward for most adverse events, so the first step in answering this question is to determine the likelihood that the observed association between the drug exposure and adverse outcome is not due to chance. There are a number of statistical methods to help answer this critical question, and we will use a hypothetical example to illustrate their use.
Consider a situation in which 50 children with viral upper respiratory infections are treated with echinacea, and 15 of these patients develop a rash within days following initiation of the drug therapy. The rash rate is then 15/50, or 30%. We cannot conclude, however, that this rash rate is related to the echinacea until we compare it with the rate that would be expected among similar patients who did not receive echinacea. So, assume that among a comparable group of 50 children with viral upper respiratory infections who did not receive echinacea, 5 children developed rashes over a comparable period—a rate of 10%. The observed difference in rates (30% vs. 10%) may reflect rashes caused by the drug exposure or may merely represent a chance occurrence. Statistical testing identifies the probability of chance occurrences, and we usually state that a difference is statistically significant when the probability that the observation is due to chance is 5% or less (i.e., p < .05); that is, we are 95% confident that the observation is due to factors other than chance. It is important to keep in mind, however, that statistical testing is based solely on probability assessments to which we attach arbitrary cut-points; it does not provide certainty regarding the role of chance. Thus, if the echinacea–rash association is “significant” at p = .05, there remains a 5% probability that the observed difference in rate is due to chance. This type of error is called a “type I” or “alpha” error; it is equivalent to the “false-positive” conclusion in the evaluation of diagnostic tests. The magnitude of the alpha (false positive) error is equal to the p value that one uses to define “statistically significant” and, as noted above, is usually set at 5% (though there is no magic to that number). In our example, the likelihood that the observed difference (15/50 vs. 5/50) is due to chance is actually less than 5% (in fact, at p = .012, it is 1.2%). Thus, if we accept a 5% risk of making a wrong call, we can state that in this study the higher rate of rash among the echinacea-exposed children is unlikely to be due to chance (and therefore likely due to other factors, including possibly a cause–effect relationship).
Another, and often preferable, way to express the risk of an ADR in an exposed group of patients compared with an unexposed group is with the relative risk (RR) and associated confidence interval. In the context of ADRs, RR is defined as the rate of the adverse event in the exposed individuals divided by the rate in the unexposed. In the
above example, the risk in the exposed group is 30% and that in the unexposed is 10%, yielding a RR of 3.0. In other words, rash occurred three times more commonly in the exposed individuals than in those not exposed. (If rash occurred with equal frequency in both groups, the RR would equal 1.0.) When using RR, the potential role of chance is expressed through calculation of the 95% confidence interval. In the current example, the 95% confidence interval ranges from a RR of 1.2 (the lower bound) to 7.6 (the upper bound). That the lower 95% confidence interval excludes a risk of 1.0 reflects that we are more than 95% certain that the observed association is not likely due to chance (the same information expressed by the p value of <.05); however, the confidence interval provides the additional information that we are 95% confident that the true RR lies between 1.2 and 7.6, and indeed is statistically more likely to be in the middle of that range and less likely to be near the extremes. Confidence intervals provide the additional benefit of statistical perspective. For a given risk estimate (e.g., RR = 3.0), p will be less than .05 whether the lower bound is 1.1 or 2.9, since both exclude 1.0; however, the values of the respective lower bounds should prompt the observer to recognize the more tentative nature of the former estimate.
above example, the risk in the exposed group is 30% and that in the unexposed is 10%, yielding a RR of 3.0. In other words, rash occurred three times more commonly in the exposed individuals than in those not exposed. (If rash occurred with equal frequency in both groups, the RR would equal 1.0.) When using RR, the potential role of chance is expressed through calculation of the 95% confidence interval. In the current example, the 95% confidence interval ranges from a RR of 1.2 (the lower bound) to 7.6 (the upper bound). That the lower 95% confidence interval excludes a risk of 1.0 reflects that we are more than 95% certain that the observed association is not likely due to chance (the same information expressed by the p value of <.05); however, the confidence interval provides the additional information that we are 95% confident that the true RR lies between 1.2 and 7.6, and indeed is statistically more likely to be in the middle of that range and less likely to be near the extremes. Confidence intervals provide the additional benefit of statistical perspective. For a given risk estimate (e.g., RR = 3.0), p will be less than .05 whether the lower bound is 1.1 or 2.9, since both exclude 1.0; however, the values of the respective lower bounds should prompt the observer to recognize the more tentative nature of the former estimate.
Table 58.1 2.0, 3.0, and 4.0 for Frequencies of Adverse Events in Unexposed Subjects of 1/50 to 1/10,000 | ||||||||||||||||||||||||||||
---|---|---|---|---|---|---|---|---|---|---|---|---|---|---|---|---|---|---|---|---|---|---|---|---|---|---|---|---|
|
It is useful to consider the situation in which no statistically significant difference between an exposed and unexposed group is found. Suppose our study revealed five patients with rashes among the 50 echinacea-exposed patients and five among the 50 unexposed. In this case, the RR is 1.0 and statistical testing would of course lead us to conclude that there is no evidence of a difference in rash rates between the two groups. Were these findings to be reported, it would not be unusual to see a conclusion such as the following: “Because there was no increased rate of adverse reactions among echinacea recipients, this drug appears safe.” The frequency with which such statements are made reflects the failure to appreciate the very important distinction between “lack of evidence” of an association between a drug and adverse effect (i.e., no meaningful information one way or another) and “evidence of no association.” In the current example, even though no association was observed, one cannot confidently rule out its existence. In fact, a study of only 50 exposed and 50 unexposed subjects would be too small to confidently rule out even fairly large increases in risk. The possibility of failing to observe an association when one actually exists is known as a “type II” or “beta” error and is comparable to the “false-negative” conclusion in the evaluation of diagnostic tests. Generally, beta is set at 0.2, meaning we are willing to accept a 20% chance of failing to detect a true association. Stated as “power” (1-beta), such a study would have an 80% chance of detecting an association where one does indeed exist.
Assuming an alpha of 0.05 and a beta of 0.2, how many exposed patients would one have to observe in order to detect various rates of ADRs? Table 58.1 presents the number of drug users needed to detect relative risks ranging from 2.0 to 4.0 for adverse events that occur among unexposed patients with varying frequencies. Thus, if the rate of a given adverse effect in the unexposed patients is 1 in 50, and if a drug is associated with a fourfold increase in risk, the increase can be detected in a sample of about 200 exposed patients. On the other hand, if the baseline rate of an adverse effect is 1 in 10,000, then nearly 44,000 exposed patients would have to be studied to detect a relative risk of the same magnitude (RR = 4.0); if the exposed patients have only a twofold increased risk of the adverse effect (RR = 2.0), its detection will require studying more than 230,000 patients exposed to the drug.
Finally, a word about relative versus absolute risk is in order. Statistical testing may reveal that an observed association between a drug exposure and given ADR is unlikely to be due to chance, and even if we assume that the observation is valid and causal (see following sections), it does not automatically follow that the increase in risk is clinically important. Suppose, for example, that in 100,000 children with viral upper respiratory infections treated with echinacea, a rash occurred in 100 whereas in a comparable sample of 100,000 children not treated with echinacea, a rash occurred in 25. The relative risk for exposed children is 4.0 with a confidence interval of 2.6 to 6.2, a highly statistically significant result. However, the absolute risk is only 1 per 1,000 in the exposed children compared with 0.25 per 1,000 in the unexposed, a difference in absolute risk of 0.75 per 1,000. Unless the rash in question were life-threatening, most would consider the absolute increase in risk to be clinically unimportant, regardless of the level of statistical significance.
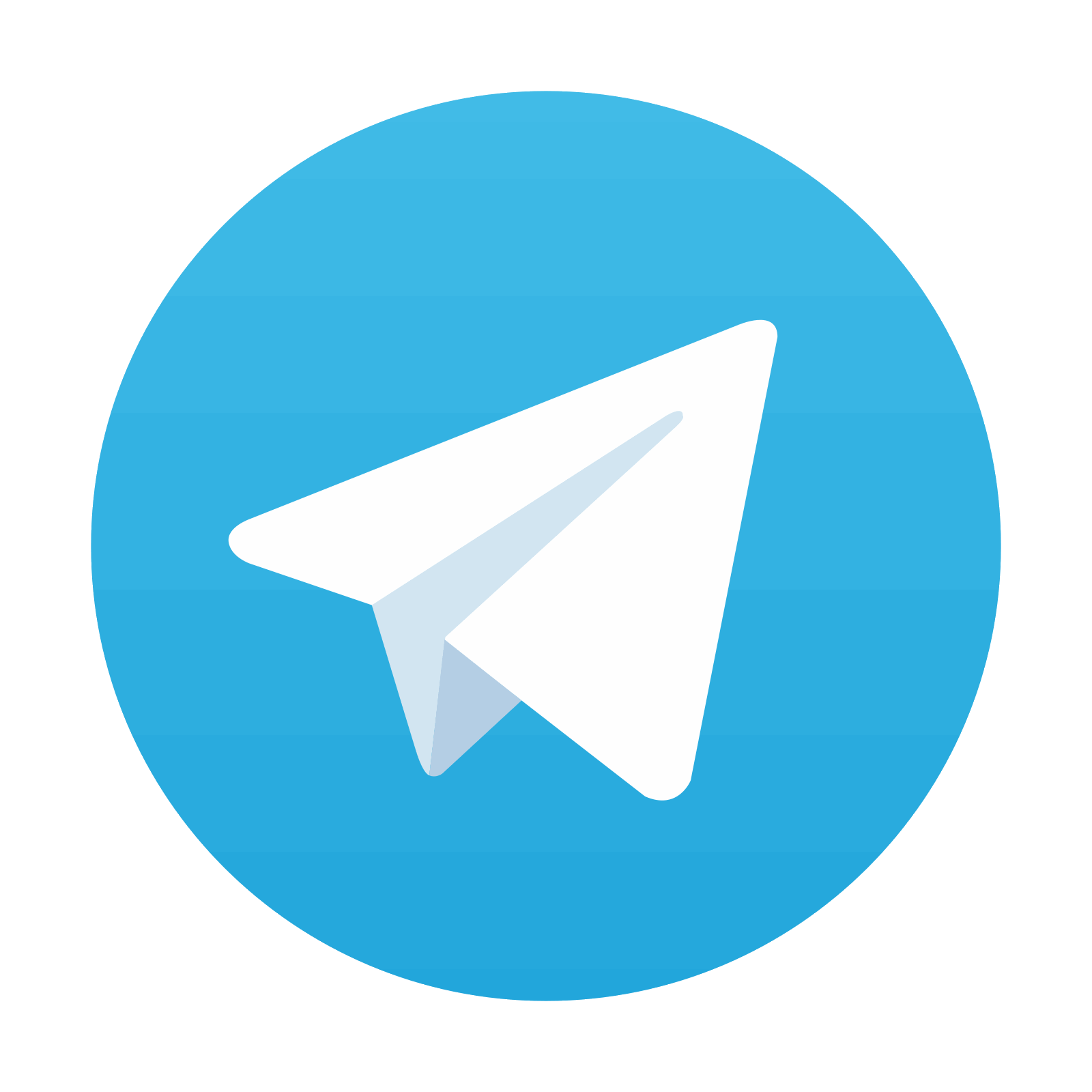
Stay updated, free articles. Join our Telegram channel

Full access? Get Clinical Tree
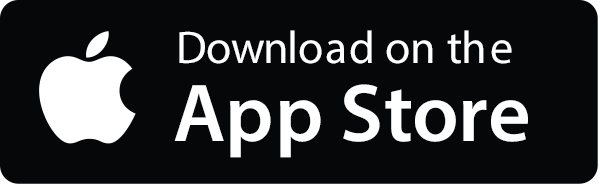
