Clinicians care for individual patients, each of whom has certain unique problems. Clinicians base their decisions on a panoply of factors that include features of the acute and chronic medical problems of the patients as well as features of both their personalities (e.g., How likely are they to comply with a certain therapeutic regimen? Do they resist conventional medical care?) and their private lives (e.g., Can they afford a certain medication? Are they planning to travel out of the country in the next few days?).
In contrast, epidemiologists study groups of people. They draw conclusions from studies of large numbers of patients and usually base conclusions on probabilities and biostatistical analyses of average (mean) outcomes.
Fortunately, these two approaches—the individualistic approach of the clinician and the probabilistic approach of the classic epidemiologist—are not incompatible. Indeed, each can illuminate the other. So it is that a chapter on epidemiology and biostatistics is included in a clinical textbook of pediatric infectious diseases. The goal of this chapter is not to make the reader an expert in these fields—courses and textbooks are designed for that purpose. Instead, the goal is to summarize how selected key aspects of epidemiology and biostatistics can be applied to understand studies that will improve our ability to evaluate and treat children with infectious diseases. Readers concerned with the epidemiology of a particular infection or syndrome should consult the appropriate chapter.
Epidemiologic Studies
Design of Studies
Overview and Definitions
Epidemiologic studies generally are designed to be either descriptive or analytic. In descriptive studies, the goal is to describe a population (e.g., the clinical manifestations and prognosis of children with tuberculosis). In analytic studies, the goal is to assess associations between two or more variables (e.g., whether children who receive an experimental vaccine are less likely to develop the infection that the vaccine is intended to prevent than are controls). Indeed, analytic studies usually are designed to assess whether a causal association exists among the variables (e.g., Is a vaccine efficacious? Do children who attend daycare centers have a higher risk of acquiring infections caused by certain bacteria or viruses?). Of course, categorizing a study so easily may not be possible. In a study that is primarily descriptive, causal associations within subgroups may be assessed. For instance, in a study of the clinical epidemiology of tuberculosis in children, the investigators may compare the mortality rates of younger children with those of older children or the mortality rates of children with tuberculous meningitis with those of children who have infection at other sites.
Most studies, whether descriptive or analytic, seek to reach conclusions about the particular group that is being assessed (e.g., children with tuberculosis). However, because studying all persons with the condition of interest is not possible, investigators inevitably study a sample of persons and hope that the conclusions drawn from studying the sample apply to the entire population of interest (the target population ). The validity of a study refers to the extent to which the results are true (accurate). The extent to which the conclusions drawn from the study sample (e.g., children with tuberculosis at a certain hospital) are valid for the target population (e.g., all children with tuberculosis in the same city) is a measure of the study’s internal validity. The extent to which the conclusions drawn from the study sample are valid for a less restrictively defined, larger population (e.g., all children with tuberculosis in the United States) is a measure of the study’s external validity, which also is called its generalizability. To ensure that a study is both valid and generalizable, investigators must take steps to protect against a variety of potential biases that may distort the results of a study.
Elements of an Analytic Study
Epidemiologists refer to the major elements of an analytic study as the exposure and the outcome. The exposure is the factor that the investigator hypothesizes is related causally to the outcome of interest. In this context, the term exposure is not used in the classic sense of potential contact with an infectious agent; rather, it is any factor (e.g., living at a certain altitude, race, receipt of either a vaccine or a medication, smoking cigarettes) that may be associated causally with the outcome. The outcome is the effect (e.g., cancer) that putatively is related causally to the exposure. The putative association may be one in which the exposure either causes or prevents the outcome of interest.
Before the specific type of study is defined, several additional elements of a study must be considered. One is how the sample for the study is selected. In general, investigators select a sample of the population either on the basis of exposure (e.g., patients who either received or did not receive a vaccine or who either attend or do not attend group daycare) or on outcome (e.g., patients who either had or did not have pneumococcal bacteremia or patients who either died or survived). Another key element is the timing of the study in relation to the timing of exposure and outcome. In studies in which the timing is historical, both the exposure and the outcome occurred before the study was initiated (e.g., a case-control study of a vaccine’s efficacy in which cases with disease are identified from historical logbooks and antecedent receipt of the vaccine is determined from medical records). Timing may be concurrent— that is, both the exposure and the outcome occur after the study is initiated (e.g., a randomized clinical trial of the efficacy of a new antibiotic). Timing also may be mixed, a mixture of historical and concurrent (e.g., a study of the current IQ of children who previously had aseptic meningitis).
A final important element of a study is its direction. Direction is used to describe the order in which outcome and exposure are assessed. It may be done in a forward direction, from exposure to outcome (e.g., in a clinical trial of a vaccine’s efficacy, the exposure [receipt or nonreceipt of the experimental vaccine] occurs first, and the occurrence of the outcome [the infection that the vaccine is designed to prevent] is determined subsequently). Alternatively, a study’s direction may be backward, from outcome to exposure (e.g., in a case-control study, the outcome [e.g., pneumococcal bacteremia] is determined first, and exposure [e.g., previous receipt of pneumococcal vaccine] is determined subsequently). Alternatively, exposure and outcome may be determined simultaneously (e.g., a survey in which determination of whether subjects have sickle-cell disease and whether they are taking penicillin occurs at the same time). A study’s direction can have important implications for inferences about causal associations; for example, even though a strong statistical association may exist between sickle-cell disease and the use of penicillin, concluding that the penicillin caused the sickle-cell disease would be erroneous. Although such an inference obviously is not plausible in this instance, the dangers of making erroneous inferences about causality are very real when exposures and outcomes that are less well understood are studied, and these types of errors have occurred many times in the history of medical research.
Although the terms prospective and retrospective are used widely, substantial variability exists in how they are applied. The term prospective is used to describe studies in which selection of the sample is based on the exposure, in which the timing is concurrent, or in which the direction of the study is forward (from exposure to outcome). The term retrospective is used to describe studies in which selection of the sample is based on the outcome, in which the timing is historical, or in which the direction of the study is backward (from outcome to exposure). Referring to the specific elements of the study is preferable to using the terms prospective and retrospective, which are applied so imprecisely.
Types of Studies
Epidemiologic studies may be classified as either experimental or observational. In experimental studies, the exposure is assigned by the investigators (e.g., in a clinical trial of an experimental vaccine, the investigator assigns subjects to receive either the experimental vaccine or a placebo). In observational studies, the exposure occurs naturally (e.g., rainfall in a study that assesses the effect of the amount of rainfall on rates of mosquito-borne infections), is selected by the subjects or their parents (e.g., attendance at group daycare in a study that assesses the frequency of infectious illnesses in children who attend and others who do not attend group daycare), or is assigned in the course of regular medical care (e.g., receipt of an approved vaccine in a case-control study of the effectiveness of the vaccine). Observational studies also are called surveys.
Experimental Studies
The paradigm for an experimental study is the randomized clinical trial, which is a special type of longitudinal cohort study in which the exposure is assigned randomly to the subjects by the investigators. In randomized trials, the direction of the study always is forward, selection (or categorization) of subjects always is based on exposure (i.e., exposure is determined at the time of randomization), and timing always is concurrent (both exposure and outcome are determined during the real period of the study). Randomized trials are the sine qua non for evaluating the effect of new agents designed either to prevent (e.g., vaccines, prophylactic drugs) or to treat (e.g., antimicrobial agents) diseases. By randomly allocating subjects to either receive or not receive the agent that is being tested, potential bias is minimized. Theoretically, if the size of the sample is adequate, the only difference between the groups is whether they received the experimental agent. Consequently, if the study is conducted properly, inferring that statistically significant differences in outcomes between the groups were related causally to the experimental agent is reasonable. For this reason, in most instances, the efficacy of new therapeutic agents or new vaccines must be demonstrated in clinical trials before the U.S. Food and Drug Administration (FDA) will approve them (although in some instances new products are approved if criteria for safety and for some surrogate end point, such as a serologic correlate of immunity, are met).
The advantages and disadvantages of randomized trials are summarized in Box 6.1 . Although clinical trials are the gold standard for investigators who wish to design a scientifically valid study, they do have numerous limitations. A major problem is that clinical trials usually are expensive. Subjects need to be selected, enrolled, and monitored longitudinally to detect the outcomes. When the outcome is a disease that is relatively rare (e.g., pneumococcal bacteremia), large samples are necessary to provide adequate statistical power. Because sponsors of clinical trials usually want to test a new drug or an experimental vaccine under conditions that will maximize the chance that it will be found to be efficacious, patients with comorbid conditions (e.g., sickle-cell disease, asplenia, metastatic cancer) that might negatively affect their response to the new agent often are excluded from the study. If the subjects who are excluded are an important part of the target population for the new agent (e.g., the patients with comorbid conditions are likely to be at high risk for serious complications of the disease and thus potentially could benefit greatly from an effective new intervention), the generalizability of the results of the clinical trial may be impaired.
Advantages
-
Gold standard for scientific validity
-
Randomization ensures unbiased allocation of the exposure (e.g., a new drug or an experimental vaccine)
-
Blinding ensures unbiased assessment of outcomes
Disadvantages
-
Poor statistical power for rare diseases
-
Requires large samples
-
Requires longitudinal follow-up
-
Logistically complex and expensive
-
Impaired generalizability when the study population differs from the ultimate target population
-
Ethical issues
-
Requires informed consent
-
Difficult to use to evaluate approved (presumably efficacious) products
Most randomized clinical trials are conducted in a double-blind manner; that is, neither the investigators nor the subjects know whether they received the experimental intervention (e.g., a new drug) or the comparison agent (e.g., either a placebo or an agent used in the standard manner). Double-blinding helps ensure lack of bias in ascertainment of the outcome because neither the subject nor the investigator can be influenced either to (or not to) seek medical care or undergo diagnostic tests on the basis of which of the interventions was received.
Randomized clinical trials may pose difficult ethical problems because the new (and potentially efficacious) agent is not given to the controls. By the time that clinical trials are begun, usually some evidence suggests that the agent is efficacious (e.g., preliminary studies in a limited group of subjects showing that a new vaccine induces antibodies). Accordingly some patients and patient advocates might suggest that withholding a potentially efficacious therapeutic agent or a vaccine from persons at risk is not ethical; consequently, it may be difficult to have persons agree to be potential controls in studies of a promising new therapy.
Problems also may arise when approval of a product for the target population is based on studies that are conducted in a different population. For example, polyvalent pneumococcal polysaccharide vaccine was approved in the United States for the elderly and for adults with chronic conditions, such as chronic obstructive pulmonary disease and congestive heart failure, that put them at increased risk of acquiring serious pneumococcal infections. However, the data on which approval was based were derived from clinical trials conducted among young gold miners in South Africa who were at risk largely not because of their age or underlying illnesses but because of the conditions in which they lived and worked. Reports of vaccine failure and poor antibody response to the vaccine in the target population in the United States led to questions about the vaccine’s efficacy. However, once the vaccine was approved, conducting a randomized clinical trial in the target population was difficult ethically because it would mean withholding, on a random basis, an approved (and presumably efficacious) vaccine from patients at risk. Consequently all but one of the post-licensure studies of this vaccine’s efficacy were observational studies.
Conducting an experimental study in which the exposure is not assigned randomly is possible. For example, one might conduct an experimental cohort study in which volunteers receive a certain intervention (e.g., a new drug) while controls receive no intervention. Both groups would be monitored forward in time while undergoing surveillance for the outcome event. Although such a study incorporates some of the features of a randomized clinical trial, because the intervention is not assigned randomly, such studies are subject to significant biases.
There are also situations in which special kinds of clinical trials are needed. For example, cluster randomized clinical trials are now common. In this type of experimental study, the unit of randomization is not the individual but rather a group or “cluster” (e.g., randomization by community). This can be important in trials of, for example, experimental vaccines or other interventions that may induce herd immunity, so that unimmunized individuals in a community in which many individuals received the vaccine may be at lower risk than unimmunized individuals in the general population. However, this type of study also presents special challenges in its design and statistical analysis. Another special kind of clinical trial is a noninferiority or an equivalence trial , designed to show that the effectiveness of a new agent is not inferior to a standard agent (by a specified amount) or that its effect is equivalent (with specified parameters). These kinds of trials also present special problems in design and analysis.
Observational Studies
Cohort studies.
The direction of cohort studies always is forward, and the selection (or categorization) of subjects is always based on exposure; however, the timing may be concurrent, historical, or mixed. Thus, a cohort study may identify a cohort of subjects at a point (or at several points) in time in the recent or remote past, categorize them regarding their status with respect to the exposure (e.g., receipt of either a vaccine or a drug), and then monitor them forward in time for the occurrence of the outcome event (e.g., an infection or an unpleasant complication) until a certain point in time, which could be in the past, present, or future. As in a clinical trial, subjects must not have the outcome at the time of enrollment in the study.
Cohort studies share many of the disadvantages of randomized clinical trials (see Box 6.1 ) but have the additional disadvantage that, because they are not experimental, they are subject to many potential biases. On the other hand, cohort studies have many practical advantages (e.g., the timing can be historical, so conducting a 30-year follow-up study in just months is possible), and, because they are observational studies, they usually have fewer potential ethical problems than does a randomized trial. In addition, for rare exposures, a critical factor is to be able to base selection of subjects on exposure.
Case-control studies.
In both clinical trials and observational cohort studies, the selection of subjects is based on their exposures, and they are monitored in a forward direction until the outcome is determined. In case-control studies, the process is reversed. Subjects are selected on the basis of outcomes. The cases have the outcome, usually a disease; the controls do not have the outcome. The direction of the study is backward—the previous exposure is ascertained after the subjects are selected. The timing of case-control studies usually is historical, but it may be mixed; the timing of the exposure always is historical, but the timing of the outcome may be either historical or concurrent. For example an investigator may conduct a case-control study of a vaccine’s effectiveness in which persons who are infected (cases) are identified concurrently through active surveillance of a microbiology laboratory (incident cases). Because case-control studies are nonexperimental and the exposure (and, sometimes, the outcome) occurred in the past, the potential for bias is great both in selection of the sample and in ascertainment of both the exposure and the outcome. The advantages and disadvantages of case-control studies are shown in Box 6.2 . Use of “sham” outcomes and/or exposures in addition to the true outcomes or exposures of interest has been suggested as a means of assessing the internal validity of case-control studies.
Advantages
-
Statistically powerful method to assess outcomes that are rare or delayed
-
Logistically easier and more efficient than large experimental or observational cohort studies
-
No longitudinal follow-up, so it can be completed relatively quickly and inexpensively
-
Ethically acceptable because it is an observational study
Disadvantage
-
Subject to many potential biases
Cross-sectional studies.
Unlike cohort studies (both experimental and observational), in which timing is forward from exposure to outcome, and case-control studies, in which timing is backward from outcome to exposure, in cross-sectional studies, outcome and exposure are determined at the same time. In cohort studies, causal inference is made from cause to effect, whereas in case-control studies, causal inference is made from effect to cause. In cross-sectional studies, determining whether the exposure preceded the outcome may not be possible. Consequently making valid causal inferences from a cross-sectional study also may not be possible.
Selection of subjects for a cross-sectional study may be based on outcome, exposure, or neither. However, because cross-sectional studies include only persons with prevalent outcomes, they are particularly problematic for studies of infectious diseases because patients whose illnesses (outcomes) either resolved or resulted in death before the study is conducted are not counted as having the outcome. Consequently, cross-sectional studies are more suitable to the study of chronic conditions and rarely are used in studies of infectious diseases.
Analysis of Epidemiologic Studies
The results of epidemiologic studies with dichotomous exposures and outcomes often are displayed in a 2 × 2 contingency table. However the way that the results are analyzed statistically depends on the type of study.
Cohort Studies
Analysis of longitudinal cohort studies (both experimental trials and observational studies) is shown in Table 6.1 . The measure of association between the exposure and the outcome is the relative risk (sometimes called the risk ratio ), which is an expression of the magnitude of this association in the study sample and represents an estimate of the association in the population from which the study sample was drawn. A relative risk of 1 indicates that no association exists between the exposure and the outcome, a relative risk greater than 1 indicates that the exposure is associated with an increased risk of the outcome, and a relative risk less than 1 indicates that the exposure is associated with a decreased risk of the outcome. The attributable risk (the risk of the outcome that is attributable to the exposure) is calculated as the risk in exposed subjects minus the risk in unexposed subjects. Of course, testing the statistical significance of any association is necessary because the relative risk could be greater than 1 or less than 1 by chance (see later sections on stochastic statistics and confidence intervals).
Outcome Present | Outcome Absent | Total | |
---|---|---|---|
Exposed | a | b | a + b |
Unexposed | c | d | c + d |
Total | a + c | b + d |
These analyses assume that no attrition exists among the subjects in the study. However, because of death, migration out of the study area, and loss to follow-up, as well as variation in the time of enrollment (enrollment in either a clinical trial or an observational cohort study may occur over a prolonged period or, indeed, throughout a study), variation virtually always exists among subjects in the duration of time that they are at risk for the outcome. If the average time at risk among the exposed and the unexposed subjects is equal, the analysis shown in Table 6.1 is likely to be valid. However, if a great deal of irregular attrition or new enrollment occurs during the study, the denominator is expressed better as person-time at risk (e.g., person-months, person-years) rather than as the number of persons in the group. When this method is used, the rate then is called the incidence density rate and the index of comparison is called the incidence density ratio.
In studies of infectious diseases, the outcome event often is the occurrence of an infection. Frequently, persons who become infected recover and may remain at risk for the outcome event again. Nonetheless, a subject usually should be censored (i.e., removed from the study) once the outcome event occurs. The fact that the outcome occurred may indicate that the subject has an increased risk for the outcome, independent of the exposure that is being assessed. For example, consider a study of the protective efficacy of a conjugate pneumococcal vaccine in which one of the subjects (whether the subject is a vaccinee or a control does not matter) experiences three or four episodes of invasive infection caused by pneumococci (perhaps because the subject has a previously unrecognized underlying condition, such as an acquired immunodeficiency syndrome or a congenital immunoglobulin deficiency). The estimate of the vaccine’s efficacy would be distorted substantially if each of the outcome events was counted. Generally, in a primary analysis, only the initial event should be counted. Another reason to censor the subject is that, in some instances, the outcome event may be fatal. If some subjects die of the outcome (and, therefore, must be censored), not censoring subjects with the outcome who survive is, in effect, allowing prognosis of the infection to affect the estimate of the vaccine’s efficacy on the risk of the outcome event.
Using a different method to adjust for unequal durations of follow-up in a study may be necessary, particularly when a long latent period exists between exposure and outcome (a condition that often is met when the study involves the effect of a potential carcinogen). In such instances, simply to sum the total person-times of exposure may be misleading because the outcome is more likely to occur many years after exposure. For example, although the total person-time at risk would be the same (1000 person-years), the risk of an outcome developing clearly may be substantially different for 500 persons, each of whom is monitored for 2 years after exposure, than it may be for 40 persons, each of whom is monitored for 25 years after exposure. In such situations, the analyses must be adjusted for variation in the length of follow-up, which can be done with the use of survival analysis (also known as life-table analysis ) . The two basic methods of survival analysis, the actuarial method and the Kaplan-Meier (product-limit) method, are described in detail elsewhere.
Case-Control and Cross-Sectional Studies
Analysis of a case-control study (with dichotomous outcomes and exposures) is shown in Table 6.2 . Because selection of subjects is based on their outcomes, one cannot calculate the risk of development of the outcome, as one would in a longitudinal study (such as a clinical trial). Instead, one calculates the proportion of each group (cases and controls) that is exposed. The measure of association in a case-control study is the odds ratio. The odds of some occurrence is the probability that it will occur divided by the probability that it will not occur. In a case-control study, we are interested in the odds of exposure. The odds ratio is the ratio of the odds of exposure among cases (a/c) divided by the odds of exposure among controls (b/d). For rare events, the odds ratio closely approximates the relative risk of exposure that would be found in a longitudinal study of the same association. Use of a method known as risk set sampling allows odds ratios from case-control studies to approximate a risk ratio even if the outcome is not rare. Cross-sectional studies are analyzed either like a case-control study (if the selection of subjects was based on their outcomes) or like a cohort study (if the selection of subjects was based on their exposures).
Cases (Outcome Present) | Controls (Outcome Absent) | |
---|---|---|
Exposed | a | b |
Unexposed | c | d |
Summary Statistics
Perhaps the most basic use of statistical analysis is to summarize data. This type of statistical analysis often is called either summary or descriptive statistics. The specific summary measures that are used depend in part on whether the variables being summarized are continuous (variables with equal distance between intervals) or categorical (variables with two or more discrete categories). Age, weight, height, temperature, concentration of creatinine in serum, and the number of hours spent in group daycare all are examples of continuous variables (sometimes called dimensional or quantitative variables). Race, sex, country of residence, and whether a patient is being ventilated artificially are examples of categorical variables (sometimes called discrete variables). Of course, continuous variables can be analyzed categorically (e.g., age can be divided into either a dichotomous variable [<45 years or ≥45 years] or a polychotomous variable [20 to 44 years, 45 to 59 years, and ≥60 years]).
Continuous Variables
A frequency distribution is classification of the values of a sample of continuous variables into successive categories (e.g., for a sample of different ages, one might place each value into different 5-year [birth to 4 years, 5 to 9 years, etc.], 3-year [birth to 2 years, 3 to 5 years, etc.], or 1-year [0 year, 1 year, 2 years, 3 years, etc.] categories) and expressed as the frequency of values within each category.
A sample of values of a continuous variable also can be summarized mathematically by describing its central tendency, shape, and spread. The central tendency can be expressed as a mean (or average ) , a median (the middle value of the sample), or a mode (the most frequent value in the sample). The mean value is calculated by summing all the individual values in the sample and dividing by the number of individual values. Thus, for a sample that is composed of subjects aged 1, 1, 2, 2, 2, 3, 3, 4, 10, and 12 years, the mean age is 4 years (40/10), the median age (the age for which half the subjects are older than and half are younger than its value—if the number of subjects is even, the median is the mean of the two middle values) is 2.5 years [(2 + 3)/2], and the mode is 2 years. These summary measures provide only a limited view of the data. For example, the mean of a distribution can be shifted in the direction of extreme outlying values; in the example, the mean is 4 years even though 70% of the subjects are younger than 4 years. Likewise, from the median value alone, one would not know whether outlying values exist. Consequently, describing the spread of a distribution is important.
The spread of a sample may be expressed by its range and by its standard deviation. The range of a sample is the interval between the highest and lowest value in its distribution. In the previous example, the range is from 1 to 12 years. Another useful way of mathematically summarizing the spread of a distribution is to express the interval between each individual value and the mean value of the sample. To summarize these values, one cannot simply sum all the differences because the sum always equals zero (the sum of the positive differences equals the sum of the negative differences). To avoid this problem, the standard deviation of a sample is used. This parameter is calculated by taking the square root of the variance of the sample. The variance is the sum of the squares of the differences from the mean of each individual value divided by the number of degrees of freedom of the sample (which is equal to the number of values in the sample minus one, or N − 1). The sum of the squares is divided by the number of degrees of freedom of the sample ( N − 1) rather than by N (the actual number in the sample) because this calculation is thought to represent more accurately the true variance of the population from which a sample is taken.
To calculate the standard deviation of the sample in the example, one must determine the variance, which is the sum of the squares of the difference of each individual value from the mean of the sample divided by the degrees of freedom (number of values minus one):
which equals 132 ÷ 9, or 14.6667 years. The standard deviation is simply the square root of the variance, which is 3.8297 years.
In the medical literature, investigators often use the standard error of the mean (which is the square root of the variance divided by the square root of the number in the sample) to express the spread of the frequency distribution of a sample. However, the standard error actually is designed to be a measure of the standard deviation of the means of repeated samples from a single source population. Because the standard error always is smaller than the standard deviation of a single sample, it gives the erroneous impression that the spread of a sample is smaller than it actually is. A large sample with a large spread (and a large standard deviation) may have a small standard error. The standard error should not be used to describe the spread of a single sample.
The general shape of the frequency distribution can be inferred from the parameters given earlier. The characteristics of the most important distribution in statistics, the familiar bell-shaped curve of the normal distribution, are shown in Fig. 6.1 and are discussed in a later section about diagnostic tests. The peak value of any distribution is the mode. A distribution may be bimodal, trimodal, and so on if it has two or more modes. A frequency distribution may be asymmetric, or skewed. If the mean is greater than the median (as in the example), the distribution is skewed to the right; conversely, if the mean is less than the median, the distribution is skewed to the left.

An investigator may want to summarize the relationship between two different continuous variables, which can be done with the use of linear regression, in which a straight line is constructed to represent the relationship between a continuous dependent variable ( y ) and a continuous independent variable ( x ). An equation ( y = a + bx ) can be created that represents the best “fit” to describe the relationship between the actual values of y and x, in which a (the intercept ) is the value of y when x equals zero and b (the regression coefficient ) is the slope of the line. Such an equation allows us to summarize the relationship between these variables and to extrapolate the value of each variable for conditions that may not have been observed in the sample. For example, one can calculate the incremental value of y for each interval change in the value of x.
Categorical Variables
The measure generally used to summarize categorical data is called a rate or a proportion. Rates consist of a numerator and a denominator. The numerator represents the actual number of persons in the sample who have the characteristic of interest (e.g., those with fever, those with tuberculosis, those who received a vaccine), and the denominator is the total number of persons in the sample. The persons in the numerator must be included in the group that the denominator represents, and the persons in the denominator must be able to have the characteristic that the numerator represents. For example, if one were interested in the rate of testicular cancer in the population, women should not be included in the denominator because they cannot have testicular cancer. Some experts believe that rates, by definition, should include a time factor (e.g., incident cases per person-months or per year). However, the term is used widely to refer to a proportion of a sample, as described earlier.
One of the most important rates that is calculated in epidemiologic studies is the incidence rate or attack rate, which is the number of persons in whom a certain outcome develops divided by the number of persons in whom the outcome could have developed during a specified period. For example, a comparison may be made of the incidence rates of an infection over time in two groups in which the interventions given to prevent an infection differed (e.g., vaccine, placebo).
Another important rate, the prevalence rate, is the number of persons in whom a certain outcome develops divided by the total number of persons in the group at a specific point in time. In calculating the incidence rate, persons who at zero time (before the study begins) already have the outcome (e.g., prevalent cases) are excluded from both the numerator and the denominator. At time zero, the numerator of the incidence rate is zero. Thus, if one were interested in the incidence rate of lung cancer, persons who had lung cancer at the beginning of the study period would be excluded. The prevalence rate, by contrast, is measured at one point in time, and the numerator includes all persons with the outcome at that time.
Certain features of many infectious diseases affect both the prevalence rate and the incidence rate as measures of the occurrence of disease. The prevalence rate of any condition is related to its chronicity and the mortality rate associated with it; conditions that are common and chronic but are not associated necessarily with immediate mortality (e.g., atherosclerotic heart disease) have a relatively high prevalence rate. On the other hand, the prevalence rates are relatively low for conditions that either have a low mortality rate and resolve completely (e.g., many infectious illnesses) or have a high short-term mortality rate. To get a reliable estimate of the importance of such conditions, one must determine their incidence rates.
To describe variations in occurrence of infections over limited periods within a single population, such as a city or a state, a simple numerical frequency (e.g., number of cases of infection) often is used—daily or weekly (during an epidemic), monthly (to reflect seasonal patterns), or annually (to compare successive years). Comparisons among different populations or subgroups within a population or at widely separated times in the same population require use of the incidence rate, or attack rate.
For infections transmitted by contact, the frequency with which infection or disease occurs among exposed susceptible individuals provides a measure of the infectivity of the agent. This frequency, called the secondary attack rate, is defined as the number of contacts who become infected (or who develop disease) within the maximal incubation period of the infectious agent divided by the number of susceptible persons in the exposed group or population in question. The secondary attack rate is applied most usefully only to closed groups, households, or classrooms, where exposure safely can be presumed for all members. The first, or primary, case is the presumed source of exposure; other initial cases that occur within the minimal incubation period or since presumed exposure of the primary cases are called co-primary cases . In calculating the secondary attack rate, primary and co-primary cases are excluded from the numerator and the denominator. Cases that occur subsequently within the maximal incubation period constitute the secondary cases. Cases that develop later are excluded because it is presumed they occurred from either a different exposure or from tertiary spread. The exclusion of immune individuals from the denominator usually is feasible only for diseases sufficiently characteristic clinically that the history serves to identify them (e.g., measles, chickenpox). Moreover, one may not want to exclude immune individuals (who may not be easily identifiable) in calculating primary or secondary attack rates. Although immune individuals are not readily identifiable in the case of common respiratory diseases, the secondary attack rate based on all exposed members of the group still may be a useful measure. Its utility decreases, however, when the period of communicability of the primary case (as with Mycoplasma pneumoniae infections) is longer than the incubation period because distinguishing between secondary and tertiary cases becomes difficult.
Finally the occurrence of death caused by a specific disease typically is expressed in two different ways. One, the cause-specific mortality rate, is defined as the number of deaths from the disease during a specified period of time divided by the total number of persons in the population. It is a measure of the consequences of the disease in the population. The effect of the disease among infected individuals is reflected by the case-fatality rate, which is defined as the number of deaths due to the infection in a specified period of time divided by the number of persons infected during the same period.
Bias
Bias occurs when the estimate of the association between the exposure and the outcome in the sample differs systematically from the true value in the population. Bias in the estimate of the association is different from bias in the measurement of individual variables. The latter bias (which may occur, for example, if an instrument such as a thermometer is calibrated incorrectly) may or may not affect the estimate of association in an epidemiologic study. Biased measurements usually affect the exposed and the unexposed groups equally. By contrast, analytic bias is the effect of differential error (nonrandom error) on the assessment of the relationship between exposure and outcome. Analytic bias can occur because of information bias (as a result of differential error in ascertainment of either the exposure or the outcome), sample distortion bias (because the joint distribution of the exposure and the outcome in the sample chosen for the study is not representative of the distribution of these factors in the target population), and confounding bias (because the joint distribution of one or more variables that independently are related both to the exposure and to the outcome is unequal in the groups that are being compared). Ensuring that bias does not affect a study is critical to its validity. Furthermore, although using statistical methods to adjust for certain sources of bias (particularly for confounding bias) in analysis of the results of a study sometimes is possible, adjustment for bias after the fact may be impossible.
An example of information bias is detection bias, which occurs when there is differential detection of the outcome in the exposed and the unexposed groups. For example, numerous studies in which clinical scales to assess whether children with fever have a serious illness have been developed. Often, however, the “serious illness” may include certain abnormal laboratory test results (e.g., an abnormal radiograph of the chest or a low concentration of sodium in serum). Because children with high scores on these scales were more likely to undergo diagnostic tests, detection bias may have occurred because similar abnormalities (e.g., a “silent” pneumonia or a decreased concentration of sodium in serum) might go undetected in the “unexposed” group (children with lower scores on the clinical scale), a much smaller proportion of whom underwent a diagnostic test to detect a possible outcome. The best way to avoid information bias is to use standardized, consistent methods to ascertain both the exposure and the outcome and to blind subjects and investigators to ensure that no differential ascertainment of either the outcome (in longitudinal studies) or the exposure (in case-control studies) occurs.
Sample distortion bias occurs when the sample in a study is not representative of the target population. However, a nonrepresentative sample does not lead to bias necessarily; bias in assessment of the association between exposure and outcome occurs only if differential distribution of the exposure (or differential risk of the outcome) occurs in the subjects who are selected for the study versus the overall target population. This distribution can be the result of selection bias. For example, consider a case-control study of the effectiveness of an approved vaccine in which the control subjects (the uninfected patients) were chosen from private practices in affluent suburbs, whereas the case subjects (the infected patients) were any patients in whom a serious infection developed and who were hospitalized. The association between antecedent vaccination and infection probably is biased because the controls are not a representative sample of the population from which the cases emerged and because the proportion of them likely to have been vaccinated is higher than in the group of all uninfected persons in the population. Another possible source of sample distortion bias is differential loss to follow-up in a longitudinal study. The potential for sample distortion bias can be minimized by maximizing the probability that a representative sample will be selected, ideally by random sampling (or some other kind of unbiased sampling), and by minimizing loss to follow-up in longitudinal studies.
Confounding bias occurs when the association between exposure and outcome is distorted by a variable (a confounder ) that is distributed unequally between the groups, is associated independently with both the exposure and the outcome, and is not in the causal pathway from exposure to outcome. One example of confounding bias is susceptibility bias, which occurs when the risk of the subjects (to development of the outcome) differs in the exposed and unexposed groups, independent of the exposure. For example, imagine a longitudinal study of the protective efficacy of a vaccine against Haemophilus influenzae type b in infants. Ensuring that an equal proportion of subjects in the vaccinated and the unvaccinated groups attended group daycare is important because attendance at group daycare is associated with an increased risk of developing infection with H. influenzae type b. If a substantially higher proportion of vaccinees than of controls attended group daycare, their attendance might confound assessment of the vaccine’s efficacy and result in a biased estimate of the effect of the vaccine (lower than the true effect). By contrast, if a disproportionate number of controls attended group daycare, the estimate of the vaccine’s efficacy might be biased in the opposite direction (it would be erroneously high because controls would have a disproportionately higher risk of acquiring infection with H. influenzae type b than would vaccinees).
In the previous example, adjusting for the effect of the confounder might be possible by either stratification or multivariable analysis (see later discussion). However, identifying confounders may not always be possible. Random allocation of subjects is the best strategy to protect against confounding, especially if there is uncertainty about the ability to identify all important potential confounders.
Causes of Disease
Historical Perspectives
Although the previously discussed principles apply to all epidemiologic studies, in studies of infectious diseases three additional factors contribute uniquely to who is and who is not affected: (1) the cause is a specific external agent (the infecting organism), (2) transmission of the organism to the host is required, and (3) certain host factors, such as immunity to infection or disease or genetic determinants of susceptibility or resistance to infection may affect both risk of and outcome of infection. Recognition of these factors (the infecting agent, transmission, and immunity) evolved gradually over many years.
The discipline of epidemiology evolved from the study of great epidemic diseases such as plague, cholera, and smallpox. The periodic waves of these diseases, which were associated with high mortality rates, stimulated the first serious efforts to explain the occurrence of disease on the basis of factors other than supernatural or divine forces.
Fundamental to such explanations was the concept of contagion . This factor long had been implicit in attitudes toward victims of leprosy, as exemplified by such early Christian practices as conducting antemortem funerals for lepers, who then were given a bell and cup and forbidden further human contact or, more drastically, were buried alive or burned at the stake. The English physician Thomas Sydenham (1624–89) introduced laudanum (derived from opium) as a painkiller, recognized the efficacy of Peruvian bark (quinine) in malaria, and revived the Hippocratic idea of “epidemic constitutions” (of atmospheric nature), which, by grafting onto existing illness, gave all concurrent illnesses the character reflecting the then prevailing “constitution.” These views persisted in colonial America, where they were expounded by such eminent individuals as Noah Webster (Webster’s Dictionary) and Dr. Benjamin Rush of Philadelphia.
Nonetheless, by the mid-18th century, the theory of contagion had gained acceptance for particular diseases, including measles, syphilis, and smallpox. The theory is alleged to have been exploited in an early act of biologic warfare: Massachusetts colonists reportedly presented the blankets of smallpox victims as gifts to the Indians, who then suffered a decimating epidemic.
The true origin of the concept of immunity is uncertain, but it was applied first in relation to smallpox. Variolation (inoculation of people with material from pox lesions that was expected to induce modified, but immunizing, disease) was practiced in the 11th or 12th century in China and in the early 18th century in both England and the American colonies. Also popular in rural England at this time was the theory that cowpox, a minor disease acquired from afflicted cattle, induced immunity to smallpox. This theory was verified by Edward Jenner (reported in 1798) and resulted, years later, in general acceptance of cowpox vaccine (vaccinia) to protect against smallpox.
The germ theory of disease was stated explicitly in 1855 by John Snow, an English anesthesiologist who took up cholera epidemiology as an avocation. Snow argued that the causative agent of cholera was a living cell that multiplied with great rapidity but was too small to be seen under the microscopes then in use. Louis Pasteur (1822–95) formally validated the germ theory by showing that the microorganisms responsible for fermentation were not generated spontaneously but came from the air. On this basis, Joseph Lord Lister revolutionized surgery by using carbolic acid to combat atmospheric germs and minimize “putrification” in surgical procedures.
In Pasteur’s wake, bacteria were cultured with great frequency from ill individuals and often were identified erroneously as causal agents. Robert Koch (1843–1910), who first isolated the bacterial causes of tuberculosis and cholera, also was the first to introduce scientific rigor into the proof of primary causation. His famed “postulates,” to be satisfied before a causal relationship between a bacterium and a disease could be accepted, required that (1) the presence of the agent be shown in every case by its recovery in pure culture; (2) the agent not be found in cases of other disease; (3) the agent, when isolated, be capable of reproducing the disease in experimental animals; and (4) the agent be recovered in pure culture from such experimental disease.
Koch’s postulates since have been modified, largely to meet problems posed by viruses. As obligate intracellular parasites, viruses cannot be “cultivated in pure culture.” In addition, they often are host specific and do not produce disease in an animal model. Other considerations that were invoked as elements of proof included the significance of recovery of the agent from diseased tissues, the demonstration of an increase in titer of specific antibody in temporal relation to the disease, and, most conclusive, the specific preventive effect of vaccines containing the viral antigen. One further situation not recognized by Koch is that infections with true pathogens do not always cause disease. We now recognize pathogenicity (defined as the proportion of infections that result in disease) as an important characteristic of infectious disease agents.
General Concepts
Causation of infectious diseases is defined in terms of the primary cause and contributing factors (or secondary causes). The former is the specific microorganism (the agent of disease) without which the particular disease cannot occur. Contributing factors affect the likelihood that infection will occur and help determine that disease will result, given infection. Identification of the causative agent may lead to the development of effective means for providing specific protective immunization (e.g., diphtheria and tetanus toxoids, vaccines against polio and measles). Finding the cause of a disease also may lead to other means of control. An example is the discovery of Legionella pneumophila as the cause of Legionnaires’ disease. The discovery of the organism led to the understanding of how the disease is transmitted—via aerosols from cooling towers, which serve as reservoirs for the bacteria. Disinfection of these towers has helped to control Legionnaires’ disease.
Infection and disease are not synonymous, although infection is necessary for an infectious disease to occur. Infection denotes replication of organisms in the tissues of a host, usually with an associated immune response; infection may be either subclinical (asymptomatic), or, if it results in development of overt clinical manifestations, it is denoted as disease. There are many examples of infections, such as poliomyelitis, other enteroviral infections, and mumps, that may be either asymptomatic or the cause of serious disease in the host. When disease occurs, it may vary in severity among infected individuals. Some infections produce full-blown disease in all infected susceptible individuals; measles is an example. Simple colonization, in contrast to infection and disease, is a state in which the organism parasitizes the host at a given site, replicates, and may persist, but it does not proceed further to cause infection or disease or to induce an immune response in the host. The carrier state, in which the organism persists over time and may be transmitted to others, may occur after colonization, infection, or disease. Examples of organisms that behave in this way include group A streptococcus and Neisseria meningitidis .
Many factors, largely related to the host and to the conditions of exposure, determine whether colonization occurs and whether the subsequent processes of infection and disease occur. These contributing or risk factors in the host are extensive and varied and may include but are not limited to age, sex, race, immune status, genetic constitution, and general state of health, including underlying diseases. Similarly, contributing factors unrelated to the host may include climate, the presence of vectors, quality of sanitation, intimacy of exposure, and socioeconomic conditions. These contributing factors vary among infectious diseases and are discussed in chapters about specific infectious agents in this textbook.
Factors Related to the Infectious Agent
What characteristics of infectious agents are significant epidemiologically? Factors associated with the occurrence of disease include factors related to perpetuation of the infectious agent, factors that govern the type of contact required to infect humans, and factors that determine the occurrence of disease. Also important are characteristics useful in classification and specific identification of agents. Some important characteristics are intrinsic, in that they can be described after appropriate direct examination of the agent. Host-related characteristics can be described only on the basis of the behavior of the agents in the host.
Intrinsic Properties
Precise classification and identification of agents are basic to the specific recognition of infections and related disease. Both depend on intrinsic properties, including morphology (which alone provides the basis for identifying most higher organisms), chemical composition (the type of nucleic acid being important in viral classification), and antigenic character, which is related to chemical composition. The last is central to specific identification of agent isolates and antibodies induced by infection. Requirements for growth or replication provide keys to the identification of some bacteria (e.g., fermentation of different sugars) and many viruses that replicate optimally or only in cultures of certain types of cells incubated at specified temperatures. For example, rhinoviruses replicate best in human diploid cells incubated at 33°C.
In recent years, we have gained the ability to rapidly detect and classify many infectious agents by identification of their genomes. The use of whole-genome analysis, molecular analysis of gene fragments, and various methods of polymerase chain reaction (PCR) has resulted in reclassification of some microorganisms and of rapid identification of pathogens, including microorganisms that cannot be cultivated by conventional means. Developments in this field are shifting the focus of clinical microbiology laboratories from morphologic and biochemical testing to molecular testing.
Several intrinsic properties relate to transmission and long-term survival of infectious agents. Persistence outside the host depends on requirements for replication (viruses replicate only within the cells of their host, whereas bacteria often can grow in food or milk) and on viability under natural conditions of temperature, moisture, and radiation. The ability of agents to persist determines whether transmission requires direct contact, as with influenzaviruses, or can involve indirect mechanisms operating over longer periods. Examples include polioviruses, typhoid bacilli, and the bacteria that cause Legionnaires’ disease.
The spectrum of animals and arthropods that an agent can infect (the host range) helps determine the possibilities for the transmission of and the reservoirs for that agent. The broader the range, the greater the possibilities. Agents that are transmitted by arthropod vectors include St. Louis encephalitis virus and Borrelia burgdorferi, the cause of Lyme disease. The former can be transmitted by a wide variety of mosquitoes, whereas the latter is transmitted by a limited number of species of ticks. Among agents that do not require a vector, many infect only humans (diphtheria bacillus, the meningococcus, and measles virus), whereas others have multiple natural hosts (rabies virus, most Salmonella bacteria).
Elaboration of exotoxins is an intrinsic attribute of many bacteria and in many instances contributes in varying degrees to the pathogenesis of disease and indirectly to immunity. Another factor is susceptibility to chemotherapeutic agents or antibiotics. Successful treatment may shorten the period of communicability, as in group A streptococcal and Bordetella pertussis infections, but it may lead to relaxed vigilance for infection; syphilis, gonorrhea, and HIV (since the development of highly active anti-retroviral therapy) are notable examples.
The instability of some intrinsic characteristics as a result of the emergence of genetically different populations because of mutations, selective pressure, transfer of genes or plasmids between bacteria, or genetic recombination can be important. One example is the resistance to chemotherapeutic or antibiotic agents that may result from selective pressure (the probable explanation for the rapid acquisition of multiple antibiotic resistance by gonococci and in human immunodeficiency virus [HIV] or plasmid transfer of resistance to antibiotics among enteric bacteria). Antibiotic resistance is of increasing importance, as exemplified by the appearance of multidrug-resistant Mycobacterium tuberculosis, penicillin-resistant pneumococci, methicillin-resistant staphylococci, and carbapenem-resistant Klebsiella pneumoniae .
Change in antigenic character can diminish the effectiveness of immunity and complicate specific recognition of infection. Influenza A virus is the classic example, with periodic major changes (shift) occurring in either or both crucial surface antigens, hemagglutinin and neuraminidase, associated with pandemic disease and progressive minor changes in hemagglutinin in the interpandemic period. Finally, the emergence of new diseases, such as St. Louis encephalitis, which first affected humans in Paris, Illinois, in 1932, or the appearance of a known disease in a new reservoir, possibly exemplified by the emergence of West Nile virus in North America in the early 2000s, can be the result of adaptation of the agent to a new host.
Epidemiologic Properties Relating to the Host
Some epidemiologically important properties of infectious agents can be defined only with reference to specific hosts. Such properties include infectivity, pathogenicity, virulence, and immunogenicity.
Infectivity.
Infectivity (ability to invade and multiply in a host) is measured conceptually in terms of the minimal number of infective particles required to establish an infection. This number, which can vary from one host to another and within the same host depending on the portal of entry, host age, and, in some cases, medications, can be determined only experimentally. Except for benign agents with which challenge of human volunteers is permissible, the infectivity of agents for humans must be inferred from the facility with which they spread in populations or, more directly, from the frequency with which infection develops in exposed susceptible individuals within a reasonable incubation period (secondary attack rate). Some researchers have used prisoners to study infectivity of Salmonella and Shigella, but the ethics of such studies has now been questioned. Measles, varicella, and polioviruses are highly infective because they require few infective particles to cause infection; rubella, mumps, and rhinoviruses have intermediate infectivity; and salmonellae and tubercle bacilli have low infectivity. Infectivity and pathogenicity may vary among strains of the same organism. The infectivity of group A streptococci is related directly to the amount of M protein in the cell wall, and strains of Staphylococcus aureus that appear identical in the laboratory may differ strikingly in infectivity and virulence. Additionally, some evidence indicates that strains of influenza A may vary in infectivity and virulence independent of preexisting immunity in the host. Contemporary studies show that variation in certain genes that are not ordinarily expressed in the clinical diagnostic laboratory are responsible for these variations in infectivity.
Pathogenicity.
Pathogenicity (ability to induce disease) is measured in terms of the proportion of infections that result in disease. It ordinarily can be determined readily by studies of the incidence and outcome of naturally occurring infections in humans. This proportion may be affected by the size of the infecting dose and numerous host factors, including age. Highly pathogenic agents include typhoid bacilli, rabies, measles, varicella, and rhinoviruses. Agents of intermediate pathogenicity include rubella, mumps, and adenoviruses; polioviruses and the tubercle bacillus have low pathogenicity.
Virulence.
Virulence may sometimes be a synonym for pathogenicity but is defined more usefully as a measure of the severity of the disease that does occur. Various criteria may be used: days confined to bed, hospitalization rate, serious sequelae that require life support such as dialysis and mechanical ventilation and others such as persisting paralysis, and death. The measure of virulence is the number of severe cases over the total number of cases, which, when death is the criterion, becomes the familiar case-fatality rate. With this as our measure, the viral agents previously mentioned fall into a different gradient from that based on pathogenicity. Rabies virus (with a case-fatality rate of nearly 100%) qualifies as highly virulent, and poliovirus (with a case-fatality rate of 7% to 10% for paralytic disease) can be classed as moderately virulent. Measles, with an occasional death from encephalitis or pneumonia, is far down the scale but is still ahead of mumps, varicella, nonfetal rubella, and rhinoviruses, for which the case-fatality rates are very low. Outcomes of severity short of death such as days of intensive care utilization or of requirement for supplemental oxygen can be used in a similar fashion.
Immunogenicity.
Immunogenicity (ability to induce specific immunity) is measured best functionally in terms of the degree and duration of resistance conferred by infection. Although agents may differ with respect to the immunogenicity of their intrinsic “protective antigens,” more important factors are the sites of primary infection and disease and the amount of antigen formed during infection to stimulate a host response. Superficial sites, such as the respiratory mucosa, are guarded chiefly by secretory antibody, which is poorly persistent; agents such as rhinoviruses, which replicate only at such sites, are ineffective stimulants of the systemic immune response. The amounts of the respective toxins released during clinical tetanus and diphtheria usually do not induce satisfactory immunity. In contrast, systemic viral infections, such as with measles and yellow fever viruses, induce solid and long-lasting immunity.
Factors Related to Relationship Between Infectious Agent and Host
The infected host provides a shelter in which the agent can multiply and from which it may spread. Key questions involve how long the agent can persist in the host and over what period and by what avenues it can escape. The time relationships and descriptive terms of different phases of infection are suggested schematically in Fig. 6.2 .

Full access? Get Clinical Tree
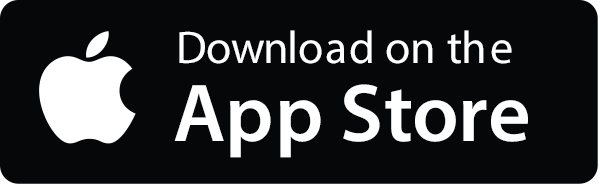
