Introduction
The science of medicine requires knowledge of more than just the effects of a disease process on the individual. A grasp of the epidemiology of a disease is necessary for clinicians to achieve a more complete understanding of the afflictions of their patients. In empirical samples, an n of 1 may not prove to be a valid data point. This is where the science of epidemiology steps in. This chapter covers the basics of epidemiology and issues that often challenge researchers in the area of family violence. The chapters that follow in this section will then more fully explore what is known about the epidemiology of family violence.
Defined by Merriam-Webster as “A branch of medical science that deals with the incidence, distribution, and control of disease in a population,” or, “The sum of the factors controlling the presence or absence of a disease or pathogen,” epidemiology can aid the clinician with a more complete understanding of a person’s disease, and answers many different types of questions about diseases. (See Table 1-1 .) The epidemiology of a disease covers a broad range of information that can be helpful in determining who is at risk for a given condition. When epidemiological data are gathered or interpreted incorrectly, those data can be misleading to clinicians. It is important to remember that a risk factor for a condition is different than a risk factor for an outcome. For example, high blood pressure is a risk factor for heart disease; low blood pressure during a heart attack is a risk factor for an adverse outcome.
Issue | Question |
---|---|
Abnormality | Is this child abused or neglected? |
Diagnosis | How accurate are the tests used to diagnose abuse or neglect? |
Frequency | How often are children abused or neglected? |
Risk | What are the risk factors for abuse or neglect? |
Prognosis | What are the outcomes and consequences for abuse or neglect? |
Treatment | How does treating (or diagnosing) child abuse or neglect change the course of the condition? |
Prevention | Does an intervention prevent abuse or neglect? Does early detection and intervention change the course? |
Cause | What leads to abuse or neglect? Or what are the mechanisms of the injuries? |
Cost | How much does it cost to care for abused or neglected children? How much does it cost to miss a case of severe physical abuse? |
For clinicians who care for victims of family violence, it is useful to know which families are at most risk and what factors are most amenable to intervention and prevention. Given that it is often difficult or impossible to obtain the history from an impartial witness when presented with injuries in a child that may be the result of abuse, we often use other factors to determine the need for further action. Some of these factors are determined by our knowledge of the literature; others are based on our anecdotal experience over our careers.
Terminology
Research has shown that the medical literature is steadily becoming more complicated and even out of reach for some medical readers. With a rapidly expanding body of increasingly difficult medical literature, it is essential that the fundamentals of epidemiology be understood. A command of the terminology used in epidemiology is crucial to an understanding of the medical literature.
The common epidemiological terms incidence and prevalence have worked their way into the general vernacular, perhaps without an understanding of their meanings. Incidence is the proportion of a group without a condition that will go on to develop the condition in a specific period of time. For example, the incidence of abusive head trauma would be the number of new cases in a year among the population at risk for abusive head trauma. Prevalence is defined as the proportion of a group who has the condition either at one point in time or during a period of time. For example, the prevalence of sexual abuse among women is said to be 25% or higher but most abuse would not have occurred in the past year.
It should be obvious that the method of data collection will strongly influence the determination of the incidence or prevalence of a disease. When physicians rely on their own personal experience to informally assess the prevalence of a disease, their anecdotal experience will be based on the peculiarities of their practices. It follows, then, that clinicians will be influenced to be alert to a given condition based on their perception of the prevalence of the disease in their population. For example, if a clinician tells a colleague, “I don’t screen for domestic violence in my patient population because it doesn’t occur often in my area,” the clinician’s perception of the prevalence of domestic violence (DV) among his patients influences his clinical decision not to screen for DV. This results in circularity of reasoning: there is little risk of DV in my patient population, so I do not need to screen for DV. I do not screen for DV in my patient population, so I do not identify DV. For this reason, clinicians must be especially aware of the hazards of relying on their anecdotal experiences. They should also carefully read the methodology sections of the research they rely on to determine the measured prevalence or incidence of a disease.
Accuracy and reliability in diagnosing conditions is a concern for all clinicians. If a patient’s signs and symptoms are misdiagnosed as a condition attributable to abuse or neglect, there are adverse consequences to the family and the child, such as further unnecessary diagnostic testing or a child protective services investigation of the family. On the other hand, if a child is abused or neglected and their presenting condition is erroneously identified as being attributable to something else, then the child is underdiagnosed and the necessary cascade of events that should take place to assess and protect the child (and potentially other siblings in the home) does not take place, leaving the child in a potentially dangerous environment.
There are four possible outcomes that result when a diagnosis of abuse or neglect is considered: (1) The patient is correctly identified as abused or neglected, a true positive diagnosis; (2) the patient is correctly identified as not abused or neglected, a true negative diagnosis; (3) the patient is not abused or neglected, but is diagnosed as having been so, a false positive diagnosis; and, (4) the patient is abused or neglected and is not identified as having been so, a false negative diagnosis ( Figure 1-1 ). To determine the accuracy of the diagnosis, one must know what the “gold standard” is. In other words, what is the most accurate way of knowing whether a given patient has a given disease at a given point in time? For many diseases, the gold standard is an autopsy. Since most patients will not have an autopsy to confirm a diagnosis, we are forced to rely on other tests to arrive at a diagnosis. We then weigh our results against what the results could be if we were able to perform the gold standard study.

Sensitivity and specificity are measures of diagnostic accuracy. The sensitivity of a test is defined as its ability to accurately identify the true positives. Increasing the sensitivity likely also results in generating more false positives as an unintended consequence. Very sensitive tests, therefore, are most helpful when the results are negative. Ruling out a condition with a sensitive test can assure the clinician (and the family) that the condition in question most likely does not exist. However, there will be patients who will test positive with sensitive tests that do not actually have the condition. These patients will possibly require further intervention or, in the case of suspected child abuse, further investigation. The specificity of a test is defined as its ability to accurately classify true negatives as persons without the disease in question. If a patient is screened with a highly specific test and the results are positive, it can be stated with certainty that the patient does indeed have the condition (i.e., it is unlikely that the positive test results could be attributed to another condition). Specific tests are more useful when the test is positive, as the condition has been ruled in by the positive results.
Balancing the specificity and the sensitivity of a test is challenging. A test with low sensitivity could miss true positives and result in a child left in a potentially abusive situation, whereas tests with low specificity can result in false positives, which may subject a family to intrusive investigations and a child to unnecessary testing. When the testing is being performed for a disease or condition where the consequences for missing the diagnosis are severe, it is important to choose the most sensitive test, sacrificing specificity if necessary.
By definition, the specificity and sensitivity of a given test in a given patient require information as to whether the patient truly has or does not have the condition. Because this information is not known when the clinician actually orders the test, it becomes necessary to understand the predictive value of the test for this patient. Two types of probability are used to describe the predictive value of a test: the positive predictive value (PPV) and the negative predictive value (NPV). The positive predictive value of a test is the likelihood that a patient has the condition when the test results indicate that they have the condition. The negative predictive value of a test is the likelihood that patient does not have the condition when the test results indicate that they do not have the condition.
The predictive value of a test is different than the test sensitivity and specificity. Sensitivity and specificity are characteristics of the test; the predictive value is a characteristic of the test and the prevalence of the disease in the patients being tested. Consider the situation where a condition has a very low prevalence in a given population; for example, HIV infections in U.S. children. In such a situation, if a test is highly sensitive and the results are positive, it is still most likely to be a false positive. If a highly sensitive test for HIV is performed in a child following a sexual assault, the pretest probability (the prevalence) is low. Therefore, the predictive value (or the posttest probability) of a positive HIV test remains low in this population. If the results are positive, there is a good chance it will be a false positive and will therefore require further testing.
In another example, consider the negative predictive value of the skeletal survey for rib fractures in the potentially abused infant. If an infant presents with a head injury, multiple bruises and a limb fracture and then receives a skeletal survey, the pretest probability is high that other fractures such as rib fractures will be found. Rib fractures are prevalent in abused infants. If no fractures are identified on the skeletal survey, it is quite possible this is a false negative result, despite x-rays being a sensitive test for rib fractures. For this reason, the negative predictive value of this study to correctly identify rib fractures is low and further testing is necessary to confirm the absence of this type of fracture if other data or history prompts concerns about physical abuse.
Clinicians must understand the prevalence of a condition in their patient population to accurately determine the predictive value of a test. As previously discussed, the prevalence of a condition can be difficult to accurately assess in clinicians’ personal patient populations. Prevalence of abuse can be estimated by starting with the population prevalence and then considering risk factors such as persistent crying, demographics factors such as young maternal age, and the specific details of a clinical situation. By relying upon published epidemiological studies, clinicians can hone their skill at estimating the pretest probability of a given condition and therefore better assess the posttest probability when the results are received.
Still, the medical literature must be viewed through a cautious lens. Often, literature will describe risk factors associated with a given condition. Quantifying the effect of a risk factor on the likelihood of a condition can be done in different statistical fashions. One term is attributable risk (AR). The AR is the incidence of a condition in patients with a specific risk factor minus the incidence of the condition in patients without that risk factor. AR estimates should be reserved for situations in which the factors might be considered causal and not simply as confounders. For example, smoking is a causal risk factor associated with lung cancers but male gender is not thought to be causal and is a marker for increased rates of smoking (a confounder).
The relative risk (RR), also known as the risk ratio, describes the risk (probability) of developing a condition when a risk factor is present versus the risk of developing the same condition without the risk factor. One might ask, “What is the relative risk for an infant being fatally abused if there is an unrelated male caregiver in the home?” To answer this question, one would need to know the probability of an infant being fatally abused with and without an unrelated male caregiver. Relative risks are the statistic most often encountered in cohort studies (i.e., longitudinal studies of a defined group of individuals).
Odds ratios (OR) are distinct from RR, but are sometimes used interchangeably when the disease or outcome being looked at is rare. ORs are used in retrospective studies and case-control studies as measures of effect describing the strength of a relationship. They describe the ratio of the odds of having the exposure if you have the disease compared with the odds of having been exposed if you don’t have the disease or outcome. If an OR is greater than 1, the first group has a higher odds of the condition relative to the second group; if the OR is less than 1, the first group has a lower odds of the condition relative to the second group. It is apparent that the OR would be more appropriate in a case-control study or a retrospective study when you consider the fact that the condition (e.g., some type of abuse) is already present in the sample and the researcher is interested in knowing how often a risk factor is present in the group that is abused versus the group that is not. To use RR, the condition is not yet present, the risk factor is, and the researcher is watching to see which subjects develop the condition.
There are situations where a public health policy makes sense, even if it addresses a risk factor which is relatively weak, if that risk factor affects many people in a community. A risk factor with a small relative risk, but which is widely prevalent may play a larger role in the development of a condition than a risk factor that has a high relative risk but is rare in the population. The statistical measure that describes this impact is the population attributable risk (PAR). Public health initiatives related to lead abatement in urban population centers, for example, are targeting a known population attributable risk. In the field of child abuse research, the work on social capital as a modifiable risk factor is an example of attempting to address a PAR. By increasing the social capital in a neighborhood, the risk of abuse for the children in the neighborhood can be reduced more broadly than by attempting to address the risk factors in an individual home. Therefore, while the relative risk is smaller to the individual children in the neighborhood, the widely prevalent risk of decreased social capital will affect more children placing them at increased risk of being abused. This is the so called “prevention paradox. ”
Epidemiological Studies in Child Abuse
Countless studies address risk factors, prevalence, and incidence of child abuse and neglect in the extant body of medical literature. A few of these studies are widely referenced as sources for our understanding of the epidemiology of child abuse and neglect. The National Child Abuse and Neglect Data System (NCANDS) and the National Incidence Studies (NIS) are two of the most commonly cited studies. These studies both address rates of various forms of child abuse, but gather information in very different ways. Data collected in the NCANDS are compiled by child protection agencies across the United States. With an enormous data set consisting of millions of data points, numerous questions can be addressed but there are limitations that must be considered. Children represented in the NCANDS are only those who came to the attention of local authorities. It is unknown how many others are abused or neglected but aren’t identified in this sample. Although many epidemiological questions can be answered by examining the case details and risk factors of children who are reported for suspicions of abuse or neglect, the sample is inherently biased, and these biases can influence our understanding of the problem.
The NIS is a survey that occurs about every 10 years as a form of active surveillance. The goal of this study is to more accurately identify the number of child abuse and neglect cases that occur in a community but that may not come to the attention of child protection authorities. By using community sentinels (i.e., trained observers to report abuse), cases can be identified at a broader population level. With probability sampling techniques, the NIS attempts to demonstrate the impact of abuse nationally through a more affordable research methodology than would be possible if the entire population were studied.
Direct sampling of the population is another methodology used to assess the rate or prevalence of abuse. Examples of this methodology include the CarolinaSAFE study, the National Gallup Poll, and the Juvenile Victimization Questionnaire. In these types of studies, families are contacted and questioned about specific acts of child maltreatment. In some studies parents are questioned, and in others the children are questioned. Interestingly, despite the obvious concern that a parent would be reluctant to report acts of violence against, or inappropriate contact with, their children, these studies have repeatedly found this not to be the case.
Although the studies discussed above are essential tools for researchers, clinicians, and policy makers, there are numerous inherent challenges that researchers and epidemiologists face when designing studies on child abuse and neglect. All of the studies mentioned have some limitations.
Problems in Conducting Research in Child Abuse and Neglect
Data Collection Issues
Studies can collect data in a number of ways: actively or passively, using primary data sources or official reports, anonymously, or through face-to-face interviews. The NCANDS study is an example of passive data collection using official reports. Because this sample includes only cases that are brought to the attention of authorities, undoubtedly, the prevalence of child abuse is underestimated. Another methodological problem with this dataset is that individual states reporting data do not use uniform definitions. Because the determination of whether to substantiate a case of child abuse or neglect is made by the agency investigating the allegation, there is inherently a subjective component. Criteria to substantiate cases (i.e., meeting the legal burden of proof in a given state) can change over time and be subject to secular or legal trends.
Just as the variations in state legal definitions and the subjective nature of the substantiation of allegations affect the reported prevalence of abuse in studies such as the NCANDS, the reported prevalence and incidence of abuse in other types of research are directly impacted by the researchers’ definitions. Circularity is an issue that can pose a particular problem in child abuse research. If a researcher defines a case of abusive head trauma (AHT) as a child who has (1) sustained a traumatic brain injury, (2) has a child protection team consult, and (3) this consult determines that the child is a victim of AHT, there is an inherent circularity that the reader must acknowledge when interpreting the findings of the study. Concluding that intracranial hemorrhage is a sign of abusive head trauma when the clinical team only suspected abusive head trauma because of the presence of intracranial hemorrhage is an example of circular reasoning. Early research on child abuse often contained circular reasoning that made it difficult for the results to be taken at face value. That does not mean these studies are without value; it does mean that the field must strive to achieve more stringent (i.e., less circular) case definition criteria when conducting research. Numerous studies of AHT are now using elaborate protocols for case definitions to avoid previous issues with circularity. ,
Data sources can introduce bias into a study. For example, random digit dial surveys rely on subjects answering survey questions honestly and as accurately as possible. In the CarolinaSAFE study, 1435 households were called in North Carolina and South Carolina and questioned about the disciplinary practices, and potentially abusive practices, used by adults in the household against a randomly selected child under 18 years of age. Although this methodology allows a unique perspective on possible abuse, especially abuse that may never come to the attention of authorities or community sentinels, it is subject to both recall bias and desirability bias.
Researchers conducting this type of research often will use a time frame they want the subject to use when answering questions: “In the last 6 months, how often did you hit your child somewhere other than on the bottom?” This type of question is subject to a recall bias. For rare events, the recall may be accurate. If a parent rarely ever uses physical discipline, the one time it was used in the last 6 months might have been for an especially egregious infraction on the part of the child and the event will stand out in the mind of the parent. If parents routinely use physical discipline and whip their children with belts for every perceived wrong, it may be difficult to accurately recollect the number of times. However, in a case such as this, the upper level choice (e.g., more than 10 times) may adequately encompass the extent of the events.
Desirability bias is the bias introduced when the subject knows what the answer “should be,” or what the researcher would like the answer to be. Most people recognize the social taboos associated with shaking a baby as a form of “discipline” and it would be expected that the parents would not self-report this behavior to avoid being perceived as a “bad parent.” Although it cannot be determined if some parents chose not to report this behavior because of desirability bias, the fact remains that some parents in the CarolinaSAFE study did report this alarming behavior. Desirability bias also plays a role when screening for violence of any type in a clinical setting. When a mother is asked if she experiences violence in the home, there are numerous reasons for her to withhold the truth if she is indeed a victim of interpersonal violence, including not wanting to appear to be a victim, fear of her abuser, or fear that the physician will report her to child protective services.
Some biases are introduced when subjects are enrolled into a study. A selection bias is a bias that can occur when subjects are chosen for a study in a way that reduces the likelihood that they are a representative sample of the larger population. For example, a researcher is interested in prevalence of retinal hemorrhages among all children less than 6 months of age who come to the emergency department (ED) for any reason. If the researcher’s protocol requires that the potential subject must have both parents present to be enrolled in the study, a significant percentage will not be able to be enrolled. Are the patients who arrive at the ED with only one parent significantly different than those that arrive with two parents? Does this introduce a possible bias in the design? Another example is the situation where an institutional review board (IRB) requires that a researcher include language in the informed consent document that states, “If retinal hemorrhages are identified in your child, there is a chance this finding could be related to inflicted trauma, also known as child abuse. If this is the case, we are required by law to report our concerns to child protective services.” Would this statement influence the likelihood that a family would consent to the study? It could be that parents who knew their child had sustained inflicted trauma would decline participation because they would be likely identified and reported if hemorrhages were found. On the other hand, it could be the parents did not abuse their child but might be afraid that hemorrhages would be identified (a false positive) and they would be reported and subjected to an unwarranted investigation, so they choose not to participate. Either or both of these situations would influence the researcher’s ability to accurately determine how often retinal hemorrhages are present in infants seeking care in an ED.
Ecological fallacies occur when a characteristic of a group is attributed to an individual person, implying a causal association with an outcome. For example, if there was a high rate of neglect in a particular census tract, an ecological fallacy would be to suggest that an individual living in that census tract is highly likely to neglect a child. Living in the census tract does not mean one will be neglectful, but there are likely some characteristics of the population in the area that increases the occurrence of neglect. Stereotypes are an example of an ecological fallacy that can negatively influence the ability of a clinician or researcher to accurately diagnose or classify abuse or neglect. Although lower socioeconomic status (SES) is a known risk factor associated with many forms of abuse or neglect, not all poor families abuse their children. However, rates of abuse may be high in areas with high concentrations of low SES families. Failing to consider this association (i.e., a higher concentration of low SES families is associated with a higher rate of abuse in the population) could result in drawing incorrect conclusions about residents of these areas.
Researchers who recognize the potential pitfalls that ecological fallacies pose can address them through wise methodology. A stratified sampling strategy allows the researcher to control for variables by maintaining homogenous subsets of the sample. For example, early research on child abuse suggested that certain types of abuse were more common among minority populations. By generalizing in this fashion, the research fails to take into account that there are other features shared among members of a minority population besides their race or ethnicity. It is not uncommon that SES is correlated with minority status. If the research were conducted to control for the SES of the various racial groups, it might be that SES was a stronger determinant of risk of abuse than was race. This in fact is the case.
In the late 1970s, professionals dealing with child abuse and neglect attempted to describe the ubiquitous nature of child maltreatment. It was stated that abuse affects all religions, races, communities, and economic levels. This led researcher Leroy Pelton to address the so-called Myth of Classlessness. Dr. Pelton demonstrated a real increased rate of abuse and neglect among lower SES groups. It has been suggested that cognitive biases can lead to differences in recognition and reporting of abuse and this explains the higher rates among poorer populations. However, this would not explain the apparent dose-effect of poverty on the rates of abuse. Data cited clearly show that rates of abuse increase as SES decreases. Dr. Pelton explained that when public awareness increased about child abuse and reporting, there was not a concomitant increase in the rate of reports on higher SES families. “We have no grounds for proclaiming that if middle-class and upper-class households were more open to public scrutiny, we would find proportionately as many abuse and neglect cases among them. Undiscovered evidence is no evidence at all. ” Although it is important to recognize that rates of abuse among the poor are higher, it does not suggest that being poor causes parents to harm or neglect children in their care. The myth of classlessness serves to minimize the unique stresses that poverty places on families and the more dangerous environments that children are exposed to when raised in poverty. This minimization results in a diversion from what could be a focused risk reduction effort, the reduction of the number of children living in poverty.
Ethical Issues
There are significant ethical considerations in research on child abuse and neglect. One overarching issue facing researchers is mandated reporting of suspected child maltreatment, which is required by law in all 50 U.S. states. Many potential subjects are aware of this law and might be reluctant to disclose information that could result in abuse being reported to the authorities. This could result in measurement bias, a bias directly related to the desirability bias.
With the rise of IRBs governing research, there has arisen an issue unique to child abuse research—should prospective subjects be warned of the possibility of a report being made if abuse or neglect is suspected? With no consensus existing and no standard approach to this issue, some IRBs require researchers to warn potential subjects (or, as is often the case in child abuse research, the proxy signing the consent), while other IRBs remain silent on the issue, assuming that mandated reporting is a known factor that exists regardless of the research being conducted. It is arguable even that reporting suspected abuse or neglect is not a risk to the patient, and rather, it could bring benefit by protecting the child from further harm. Further, it should be noted that the principle of informed consent being given by a proxy (in the case of children, often a parent) is founded on the concept of the subject’s best interest. In the case of a parent as an abuser, there is an inherent conflict of interest that could preclude that parent from offering informed consent when the “risk” of reporting is disclosed. Although currently no answers have been reached by the research and bioethical community, this very topic is being actively addressed internationally to arrive at some approach that can balance the rights of the child (and family) and the need for quality research on child abuse and neglect.
Difficulties Identifying Child Abuse and Neglect
Both researchers and clinicians are faced with the issue of accurately identifying cases of abuse or neglect. Just as researchers must be cognizant of biases that adversely affect their studies, clinicians should also be aware of biases that adversely affect their ability to diagnose abuse or neglect accurately. These biases are referred to as cognitive biases or cognitive errors. Errors in the correct diagnosis of abuse can directly lead to inaccuracies in the reported epidemiology of abuse. Take, for example, the NCANDS data. Official reports of abuse are often based on the diagnosis of abuse by a medical professional. If medical professionals are systematically misdiagnosing (either over- or under-diagnosing) abuse, the official reported numbers will not be accurate, leading to a skewed understanding of the prevalence of abuse. Several types of cognitive bias are particularly relevant to the recognition of child maltreatment. For example, selection bias can influence whether a sample accurately reflects the underlying population. When selection bias affects a clinician in a clinical encounter, it can influence who is evaluated for a given condition. If a clinician feels that a family is a “nice family” and is therefore at low risk for abusing their children, injuries in their children will not be evaluated in the same way they would be if the child were from a “bad family.” This selection bias will tend to create a self-fulfilling prophecy. If clinicians only search for abuse in “bad families,” they will find examples in troubled families because they are looking for it. By failing to consider abuse in “nice families,” they will fail to identify abuse and will feel justified in their continued practice of relying on their subjective sense of “good” versus “bad” families.
Confirmation bias can also appear in a clinician’s evaluation for abuse. When abuse is in the differential diagnosis, we ask questions and order tests to strengthen our certainty of the diagnosis. We will tend to incorporate positive findings that support our theory and disregard information that does not fit into our cognitive framework for abuse. Similarly, when the patient with injuries that could be due to abuse is seen through the filter of a “good family,” we will be subject to a measurement bias and will look for alternate explanations for the injury and disregard information that would lead us to believe that a “good family” could have harmed their child.
The concept of anchoring is important in a discussion of cognitive biases. With anchoring, we attach great significance to a piece of information and build from there. This focus can derail a clinical evaluation and result in overdiagnosing or underdiagnosing of abuse. As an example, consider a mother presenting to the ED with her 18-month-old child who is limping and refusing to bear weight on her leg. The mother reports this has been going on for 3 days and has progressed to the point where the child wants to be carried everywhere. The mother is young, unemployed, and has two other children under the age of 5 years at home. She appears tired and disheveled. While waiting for the physician, the nurse overhears the mother yelling at someone on her cell phone and using very explicit language. The physician examining the child is told about the overheard phone call before the examination. When examining the patient, the physician feels the mother is rude and abrupt with him. Given the social information up to this point, the physician believes this patient has a high likelihood of an abusive injury. Anchoring on these facts and the clinician’s subjective assessment of the mother’s appearance and behavior, he begins to question her specifically about discipline, other caregivers, and previous reports to child protective services. As the exchange escalates, the mother becomes extremely frustrated and attempts to explain that the child has been sick recently and has had low grade fevers. Because this information does not fit in with a diagnosis of abuse, the physician does not incorporate it into the overall clinical picture. He interprets her increasing hostility as a sign that she has harmed her child, again adding weight to his cognitive anchor. He now will make clinical and diagnostic decisions based on where he has arrived as a result of his anchor: an evaluation for child abuse. Consider the alternative scenario: a mother comes in with the same child but is neatly dressed and calm. She is young but married and employed. Her husband is at home with her other two small children. When the physician arrives to examine the child, he is struck by how articulately the mother describes the child’s symptoms and clinical course. He begins to develop a diagnostic strategy to determine whether this is toxic synovitis. Both of these cognitive anchors drive the interactions and influence the diagnosis. What if the first child had toxic synovitis, but was incorrectly labeled as abused and the second child was abused and incorrectly labeled as toxic synovitis? Cognitive anchors must be consciously recognized to be sure one does not miss important diagnostic information.
Implicit biases or stereotypes are another example of potential cognitive pitfalls. It is clear that a patient’s race plays a significant role in the type and quality of medical care received. The first three rounds of the NIS have failed to show a difference in the rate of abuse by race. Despite this lack of evidence, researchers have shown that minority children, particularly African-American children, are more likely to be medically evaluated for the possibility of abusive injuries and are more likely to be reported to CPS, regardless of whether their injuries are likely accidental or inflicted. A recent study clearly demonstrated that in children with identical injuries, minority children were significantly more likely to be reported to CPS than their white counterparts. There is evidence that abusive head trauma will be missed more frequently in white children. It is unknown whether minority children have greater errors of “overdiagnosis,” but a detection bias is strongly suggested by the work of Lane et al. Clinicians need to center their judgments about the presence or absence of child abuse on risk factors other than race since race has no known contribution to the a priori risk.
Strength of the Evidence
With the field of literature rapidly expanding, we are developing an increasing understanding of the public health impact of child abuse and neglect. We also are recognizing the need to accurately define and measure child maltreatment to identify how to prevent it. As researchers and clinicians become increasingly aware of cognitive pitfalls, we become better able to address them, strengthening our work along the way. It would be wrong to “throw the baby out with the bath water” and walk away from earlier studies because of the flaws we now recognize. The early literature is the foundation on which to build sound hypotheses that we can then test in a more rigorous fashion. Research, like the clinical diagnostic process, is an iterative process.
Future Directions
As we hone our abilities to effectively measure abuse and neglect, we will need to continue to sample diverse populations, both nationally and internationally, to more fully understand who is at risk and how we can modify that risk. We also need to begin to explore how to teach clinicians to be “better thinkers.” We all rely on cognitive shortcuts without realizing it. Problems arise when we do not assess what we as a field know and apply that to what we as individual clinicians do in our clinical practice.
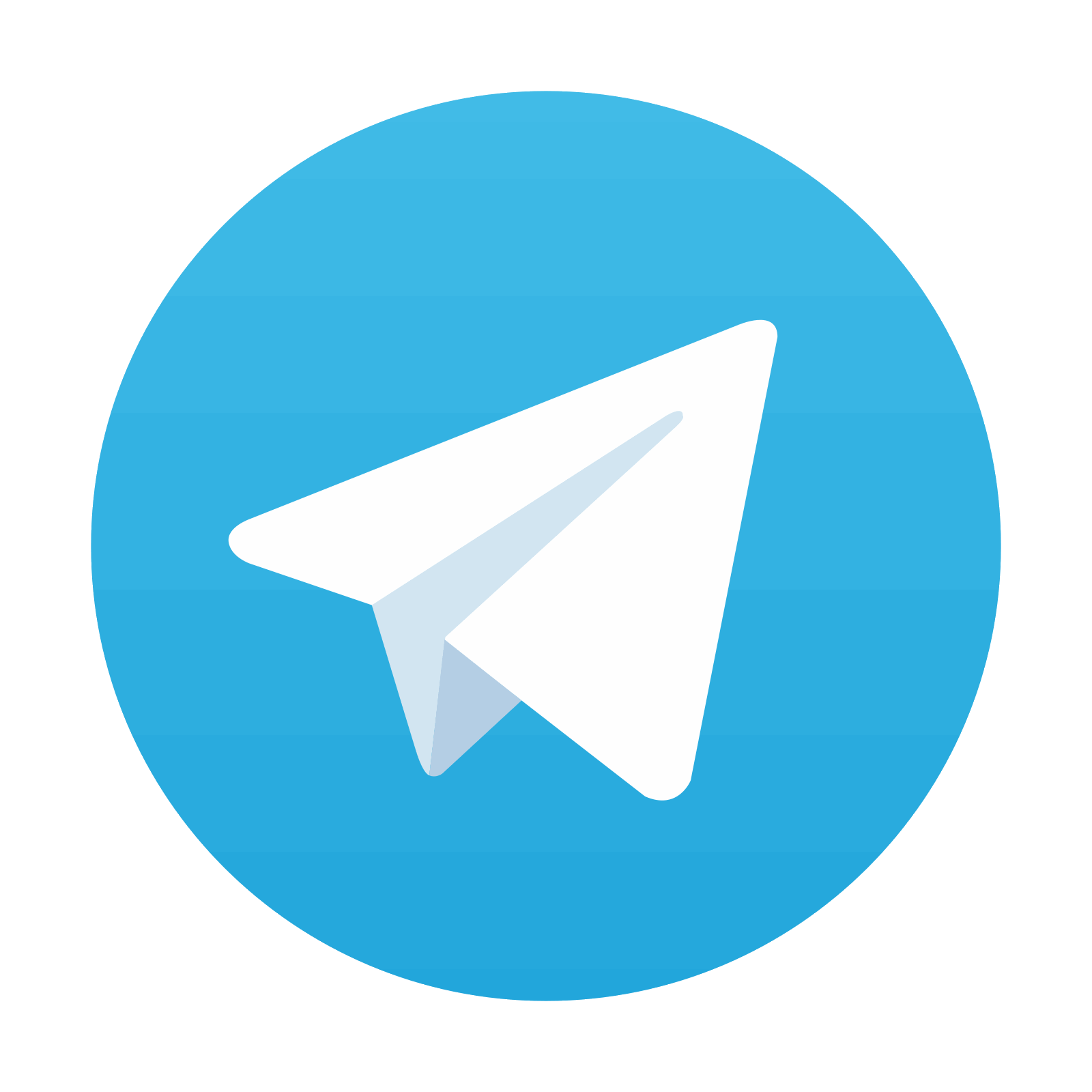
Stay updated, free articles. Join our Telegram channel

Full access? Get Clinical Tree
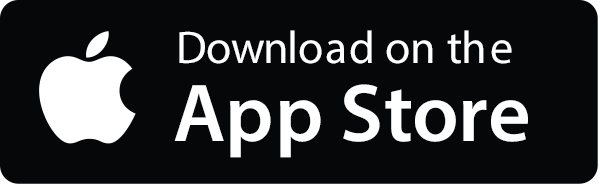
