The article below summarizes a roundtable discussion of a study published in this issue of the Journal in light of its methodology, relevance to practice, and implications for future research. Article discussed:
Treloar SA, Bell TA, Nagle CM, et al. Early menstrual characteristics associated with subsequent diagnosis of endometriosis. Am J Obstet Gynecol 2010;202:534.e1-6.
The full discussion appears at www.AJOG.org pages e1-4.
Discussion Questions
- ■
What was this study’s primary hypothesis?
- ■
What assumptions did the authors make?
- ■
What approach did the authors take to test their hypothesis?
- ■
Were the study populations appropriate?
- ■
Were the statistical analyses suitable?
- ■
What were the key findings?
- ■
How might these findings be further explored?
Endometriosis is a common disease associated with pelvic pain, dysmenorrhea and dyspareunia. It is diagnosed when endometrial tissue is discovered outside the uterine cavity, but its presentation and symptoms vary. Several theories on the pathogenesis of endometriosis exist, including Sampson’s theory that endometrial tissue flows back through the fallopian tubes during menses and implants in the pelvis. In this month’s meeting of the Journal Club, discussion centered on a case-control study by Treloar and colleagues, who investigated connections between early menstrual characteristics and later diagnosis of endometriosis—a relationship that would support Sampson’s theory.
See related article, page 534
Finding the perfect match
During the discussion of this study, participants touched on matching techniques for case-control studies. Two general approaches are available: individual matching and frequency matching. In individual matching, controls and cases are matched on 1 or more characteristics. In frequency matching, the proportion of participants with a particular trait is the same in the group of cases and in the group of controls. With either technique, the main goal is to reduce confounding by matching qualities that are related to the diagnosis and exposure but are not of interest in the study. Most often these characteristics include age, race, and/or sex.
The main benefit of matching, as mentioned in our discussion, is the potential for improved statistical efficiency since the confounding factor is equally distributed between both study groups. This equal distribution should provide investigators with tighter or more precise confidence intervals around their calculated odds ratios. Nevertheless, investigators need to be mindful of the number and type of characteristics chosen, as overmatching is possible, making it difficult to distinguish differences between cases and controls. Then, too, the matching process can introduce bias, because once unmatched controls are eliminated, the remaining controls may not be representative of the population as a whole.
In this study, cases and controls were frequency-matched on age and region of residence. Cases were chosen randomly from a cohort of women initially recruited for a genetic study of endometriosis. Controls were randomly selected from twin pairs in the Australian Twin Registry; eligible subjects had never reported a diagnosis of endometriosis. With the information provided by the authors, it is not immediately evident why matching was performed on region of residence. As mentioned, matching should be performed using attributes that are related to both the diagnosis and exposure. While one can infer that region of residence might influence exposure and a subsequent diagnosis of endometriosis, readers should keep points like this in mind when interpreting study results. Overall, the authors were conservative, choosing only 2 characteristics to match, so we may be somewhat reassured that overmatching was not an issue. Journal Club members were not concerned that matching introduced its own bias.
One model fits all?
Another important issue raised during the discussion was the appropriate choice of analytic methods in a matched study design. The available options for analyzing data from individually matched datasets include stratified analysis, McNemar analysis, and logistic regression. Logistic regression allows for control of variables other than the matched variables, an important advantage. When it is used with matched data, as it was in this study, authors should note whether unconditional or conditional logistic regression was used, as certain criteria are required for each.
The choice depends on the number of parameters considered in the model relative to the sample size. Unconditional logistic regression can be used in analyses where the number of parameters is small relative to the number of subjects, whereas conditional logistic regression is recommended for analyses in which the number of parameters is large relative to the number of subjects. For example, individual matching increases the number of parameters, requiring that conditional logistic regression be used. If unconditional logistic regression were used for analysis of individually matched data, the odds ratio might be overestimated.
Conversely, in frequency matching, the number of matching variables is small compared to the total sample size, so unconditional logistic regression is suitable, as long as the matching variables are included in the model. Treloar and colleagues used frequency matching and unconditional logistic regression for their data analysis. They included age and state of residence as covariates in their model, so their analytic approach appears to be sound.
Future directions
Despite a reliable analytic approach, any case-control study can be subject to bias. Moreover, when cases and controls are chosen from 2 different populations, as was the case in this study, neither matching nor analytic technique can completely make up for the differences between groups. A prospective cohort study within 1 population might theoretically be the next best step, but in fact, such work would be difficult if not impossible to carry out. Endometriosis is thought to develop over a long period, and surgery is required for diagnosis, meaning participants would have to undergo surgery to rule disease in or out. Yet, performing surgery on asymptomatic patients is not feasible.
An alternative approach to studying the relationship between menstrual cycle characteristics and the diagnosis of endometriosis might be a nested case-control study within 1 existing cohort of women. In such a study, cases are chosen based on the diagnosis of interest; endometriosis, in this case. Controls would then be selected randomly from among women who are in the same cohort but have not been diagnosed with endometriosis at the time the 2 groups are selected; possibly, the controls could develop the disease of interest over time. Choosing both sets from the same cohort would ensure that the controls and cases were representative of the same population. Issues such as sample bias and overmatching could be avoided, and use of simpler analytic techniques might be possible.
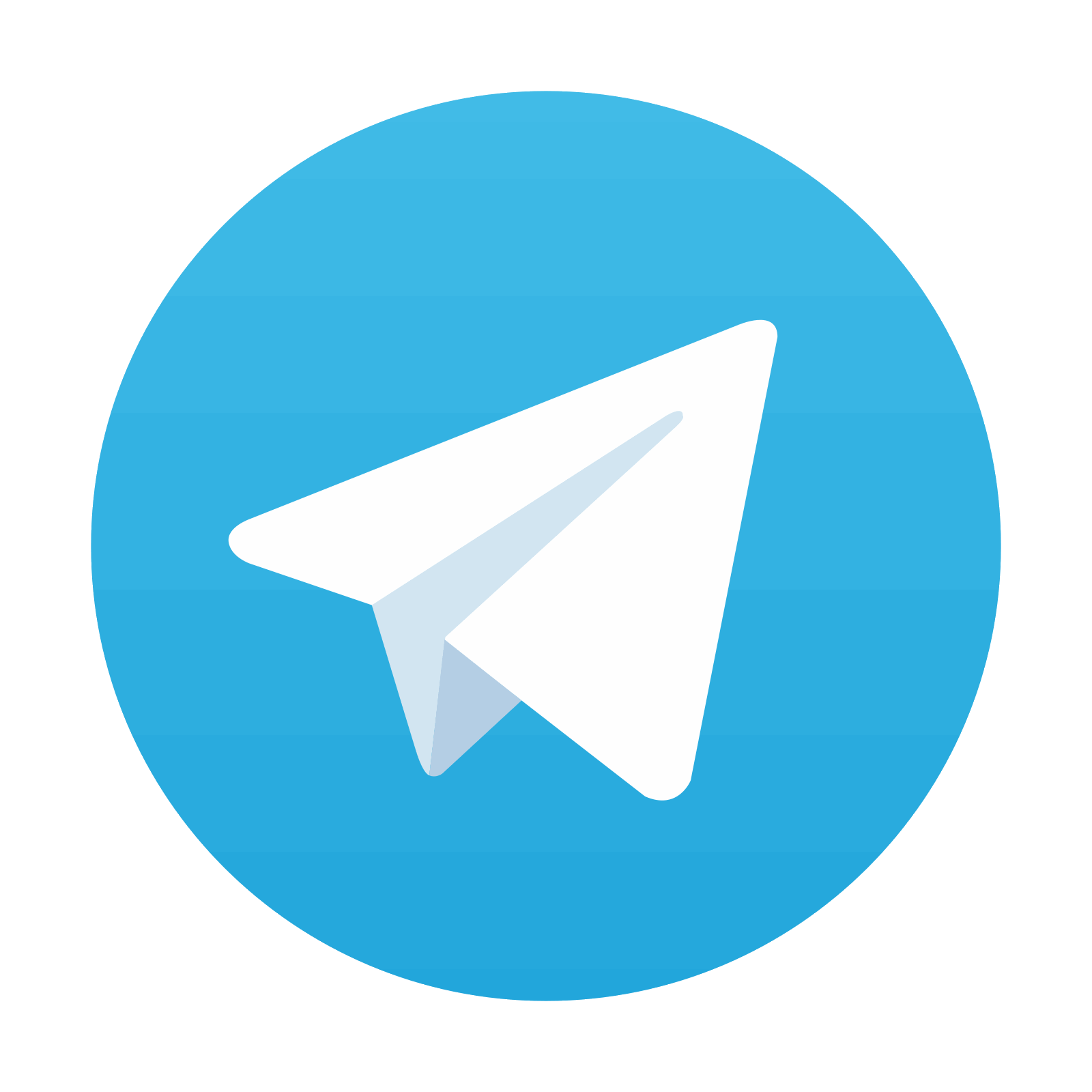
Stay updated, free articles. Join our Telegram channel

Full access? Get Clinical Tree
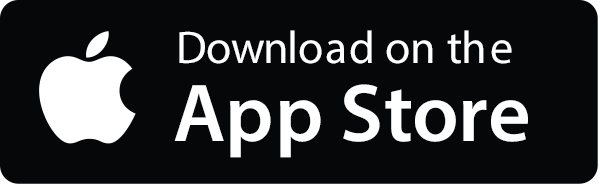
