In the roundtable that follows, clinicians discuss a study published in this issue of the Journal in light of its methodology, relevance to practice, and implications for future research. Article discussed:
Bahado-Singh RO, Akolekar R, Mandal R, et al. First-trimester metabolomic detection of late-onset preeclampsia. Am J Obstet Gynecol 2013;208:58.e1-7.
Discussion questions
- ■
How can metabolomics be used to identify biomarkers?
- ■
What were the authors’ aims?
- ■
What was the study design?
- ■
What were the statistical challenges?
- ■
What information is presented in the tables and figures?
- ■
What additional research is needed?
Introduction
Preeclampsia occurs in 5-8% of pregnancies, most commonly presenting at term as late-onset disease. Primary prevention is an enticing goal for researchers and clinicians, but any strategy would require accurate identification of high-risk women early in pregnancy. In a new study, Bahado-Singh and colleagues demonstrated that metabolomics—the comprehensive examination of metabolites—might help distinguish these patients during the first-trimester. In short, metabolomics considers the structure of these small molecules, their interactions, and reactions to modifications in their surroundings. Journal Club members agreed that while the researchers’ results must be validated in other populations, their work was well-designed and provocative.
See related article, page 58
For a summary and analysis of this discussion, see page 87
Heather A. Frey, MD and George A. Macones, MD, MSCE, Associate Editor
Background
Frey: What are biomarkers? How can metabolomics be used to identify biomarkers for diseases like preeclampsia?
Spain: Biomarkers are indicators of a biologic state. They can be objectively measured and evaluated as a sign of a physiologic or pathologic process. Metabolomics can be used to identify metabolites that are present in patients who develop preeclampsia. If some prove to have good sensitivity and specificity for predicting the disorder, these may then be used as a biomarker to determine which patients are more likely to develop preeclampsia.
Frey: How is research that uses biomarkers to predict disease clinically relevant?
Spain: Identification of biomarkers that can predict or diagnose a disease—particularly one in which early intervention might make a significant difference in outcome—or assess a therapeutic response with both a high sensitivity and specificity can be extremely useful clinically.
Frey: What were the authors’ aims?
Theilen: The authors wanted to identify first-trimester maternal serum biomarkers for late-onset preeclampsia. They also wanted to determine the diagnostic accuracy of these biomarkers for predicting late-onset preeclampsia and to assess the ability of metabolomic analysis to distinguish late-onset from early-onset preeclampsia.
Study Design
Frey: What was the study design? What are the pros/cons of this type of design?
Spain: This was a nested case-control study. In a case-control study, predictor variables are compared among a group of individuals with a particular outcome and a group without the outcome. Case-control studies are useful as they are relatively quick, inexpensive, and capable of showing associations that can then be used as a basis for a larger randomized controlled trial. The cons of this study design are that an association can be implied, but causality cannot be proven, and as an observational study, it is subject to confounding and bias. A nested case-control study is performed within an existing study cohort, which reduces selection bias.
Frey: What are some of the statistical challenges when analyzing metabolomic data?
Allsworth: As demonstrated by this study of late-onset preeclampsia, metabolomic methods may generate large numbers of possible predictive variables for analysis. The analyst may also find that there are more dependent variables than individual study participants. These complexities make visualization and summarization difficult when using standard statistical approaches. Moreover, the presence of a large number of dependent variables with varying extents of correlation raise other methodologic concerns, particularly, multicollinearity. Multicollinearity is a common concern when analyzing metabolomic data, as it is likely that multiple first-trimester metabolites observed may be correlated with one another; this correlation may adversely influence variance estimates of regression parameters.
Frey: What are principal component analysis (PCA) and partial least squares discriminant analysis (PLS-DA)?
Allsworth: PCA is a nonparametric technique that reduces the number of predictive variables through the estimation of a few linear combinations—called principal components. The first estimated component accounts for the maximum amount of total variance in the observed variables. The second component is not correlated with the first component and accounts for the maximum amount of variance not accounted for by the first component; and so on. The total number of components may equal the number of variables included, but typically, the first few components account for a significant extent of the variance. Once estimated, components may then serve as predictive variables in analysis.
PLS-DA, an approach based on multiple linear regression, was used in this study for classification and biomarker selection. It reduces data through the estimation of latent variables. If a statistically significant discrimination between classes—in this instance, cases and controls—is detected, metabolic biomarkers could be found.
Frey: How does permutation testing minimize the possibility that the observed data separation in PLS-DA is due to chance?
Allsworth: One of the potential limitations of PLS-DA is the accuracy of estimation of class assignment; researchers have proved that this approach may attempt to separate classes when no difference exists. Therefore, validation of PLS-DA models is essential. One approach, permutation testing, evaluates whether the specific class assigned to an individual study participant is significantly better than random classification into arbitrary groups. In this instance, the permutation tests were repeated 2000 times.
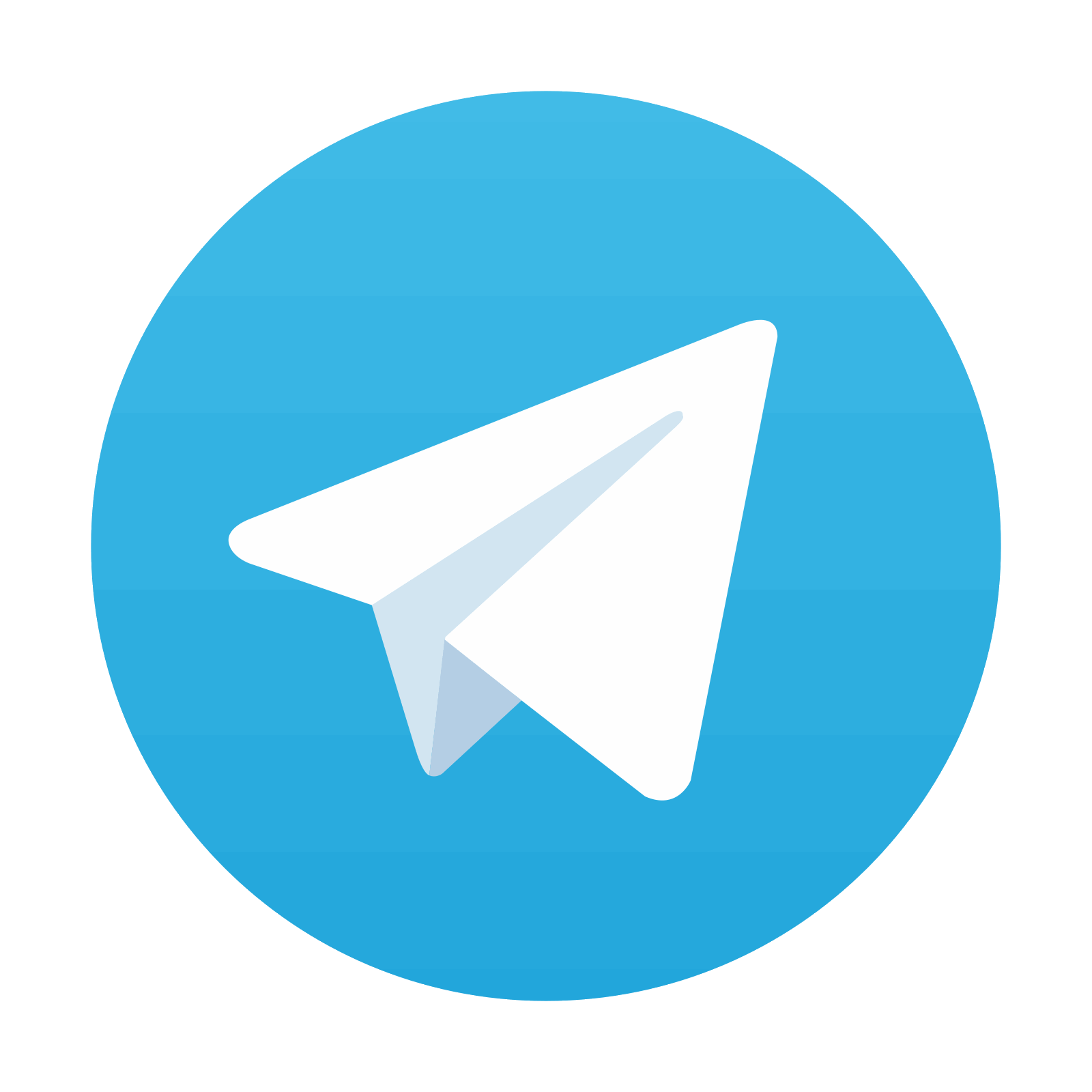
Stay updated, free articles. Join our Telegram channel

Full access? Get Clinical Tree
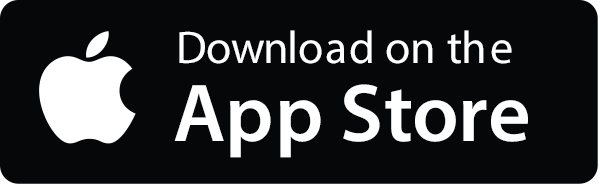
