Background
Preeclampsia affects approximately 3% of all pregnancies and is a major cause of maternal and perinatal morbidity and death. In the last decade, extensive research has been devoted to early screening for preeclampsia with the aim of reducing the prevalence of the disease through pharmacologic intervention in the high-risk group starting from the first trimester of pregnancy.
Objective
The purpose of this study was to develop a model for preeclampsia based on maternal demographic characteristics and medical history (maternal factors) and biomarkers.
Study Design
The data for this study were derived from prospective screening for adverse obstetric outcomes in women who attended for their routine first hospital visit at 11-13 weeks gestation in 2 maternity hospitals in England. We screened 35,948 singleton pregnancies that included 1058 pregnancies (2.9%) that experienced preeclampsia. Bayes theorem was used to combine the a priori risk from maternal factors with various combinations of uterine artery pulsatility index, mean arterial pressure, serum pregnancy-associated plasma protein-A, and placental growth factor multiple of the median values. Five-fold cross validation was used to assess the performance of screening for preeclampsia that delivered at <37 weeks gestation (preterm-preeclampsia) and ≥37 weeks gestation (term-preeclampsia) by models that combined maternal factors with individual biomarkers and their combination with screening by maternal factors alone.
Results
In pregnancies that experienced preeclampsia, the values of uterine artery pulsatility index and mean arterial pressure were increased, and the values of serum pregnancy-associated plasma protein-A and placental growth factor were decreased. For all biomarkers, the deviation from normal was greater for early than late preeclampsia; therefore, the performance of screening was related inversely to the gestational age at which delivery became necessary for maternal and/or fetal indications. Combined screening by maternal factors, uterine artery pulsatility index, mean arterial pressure, and placental growth factor predicted 75% (95% confidence interval, 70-80%) of preterm-preeclampsia and 47% (95% confidence interval, 44-51%) of term-preeclampsia, at a false-positive rate of 10%; inclusion of pregnancy-associated plasma protein-A did not improve the performance of screening. Such detection rates are superior to the respective values of 49% (95% confidence interval, 43-55%) and 38% (34-41%) that were achieved by screening with maternal factors alone.
Conclusion
Combination of maternal factors and biomarkers provides effective first-trimester screening for preterm-preeclampsia.
Preeclampsia affects 2-3% of all pregnancies and is a major cause of maternal and perinatal morbidity and death. In the last decade extensive research has been devoted to screening for preeclampsia with the aims of (1) to reduce the prevalence of the disease through pharmacologic intervention in the high-risk group and (2) to minimize adverse perinatal events for those who experience preeclampsia by the determination of the appropriate time and place for delivery. The traditional approach to screening for preeclampsia is to identify risk factors from maternal demographic characteristics and medical history (maternal factors), but such an approach can identify only 35% of all preeclampsia and approximately 40% of preterm-preeclampsia, at false-positive rate (FPR) of 10%.
An alternative approach to screening, which allows estimation of individual patient- specific risks of preeclampsia that requires delivery before a specified gestation, is to use Bayes theorem to combine the a priori risk from maternal characteristics and medical history (maternal factors) with the results of various combinations of biophysical and biochemical measurements that are made at different times during pregnancy. We adopted this approach using a competing risk model for the time to delivery with preeclampsia. This model assumes that, if the pregnancy was to continue indefinitely, all women would experience preeclampsia; whether they do so before a specified gestational age depends on competition between delivery before or after the development of preeclampsia. The effect of maternal factors is to modify the mean of the distribution of gestational age at delivery with preeclampsia so that, in pregnancies that are at low-risk for preeclampsia, the gestational age distribution is shifted to the right with the implication that, in most pregnancies, delivery actually will occur before the development of preeclampsia. In high-risk pregnancies the distribution is shifted to the left, and the smaller the mean gestational age, the higher is the risk for preeclampsia. The distribution of biomarkers is specified conditionally on the gestational age at delivery with preeclampsia. For any women with specific maternal factors and biomarker multiple of the median (MoM) values, the posterior distribution of the time to delivery with preeclampsia, assuming that there is no other cause of delivery, is obtained from the application of Bayes theorem.
We have reported previously on the development and performance of a maternal factor–derived algorithm for the prediction of preeclampsia. We have also proposed a model for combining the maternal factor–derived previous risk with the results of uterine artery pulsatility index (PI), mean arterial pressure (MAP), serum placental growth factor (PLGF), and pregnancy-associated plasma protein-A (PAPP-A). However, the performance of screening was assessed by simulation from the fitted model, and such an approach generally is biased optimistically because it ignores errors of estimation and departures from the assumed model.
The objective of this study of 35,948 singleton pregnancies, which included 1058 patients (2.9%) who experienced preeclampsia, with complete data on uterine artery PI, MAP, serum PLGF, and PAPP-A, is to examine the potential improvement in performance of screening by maternal factors alone with the addition of each biomarker and combinations of biomarkers. Performance of screening was assessed with the use of 5-fold cross validation.
Methods
Study population
The data for this study were derived from prospective screening for adverse obstetric outcomes in women who were attending for their routine first hospital visit in pregnancy at King’s College Hospital and Medway Maritime Hospital, UK. This visit, which was held at 11 +0 to 13 +6 weeks gestation, included (1) the recording of maternal characteristics and medical history, (2) measurement of the left and right uterine artery PI by transabdominal color Doppler ultrasound scanning and calculation of the mean PI, (3) measurement of MAP by validated automated devices and standardized protocol, and (4) measurement of serum concentration of PLGF and PAPP-A (DELFIA Xpress system, PerkinElmer Life and Analytical Sciences, Waltham, MA). Gestational age was determined from the fetal crown-rump length. The women were screened between February 2010 and July 2014 and gave written informed consent to participate in the study, which was approved by the NHS Research Ethics Committee.
The inclusion criteria were singleton pregnancy undergoing first-trimester combined screening for aneuploidy and subsequently delivering a phenotypically normal live birth or stillbirth at ≥24 weeks gestation. We excluded pregnancies with aneuploidies and major fetal abnormalities and those ending in termination, miscarriage, or fetal death before 24 weeks gestation.
Outcome measures
Data on pregnancy outcome were collected from the hospital maternity records or the general medical practitioners of the women. The obstetric records of all women with preexisting or pregnancy-associated hypertension were examined to determine whether the condition was preeclampsia, as defined by the International Society for the Study of Hypertension in Pregnancy.
Statistical analyses
Our model for the gestational age at delivery with preeclampsia was defined by 2 components: first, the previous distribution based on maternal factors and, second, the conditional distribution of MoM biomarker values, given the gestational age with preeclampsia and maternal factors. Values of uterine artery PI, MAP, PAPP-A, and PLGF were expressed as a MoM adjustment for those characteristics that were found to provide a substantive contribution to the log 10 transformed value that included the maternal factors in the previous model. In the preeclampsia group, the mean log 10 MoM was assumed to depend linearly with gestational age at delivery; this linear relationship was assumed to continue until the mean log 10 MoM of zero, beyond which the mean was taken as zero. Multivariable Gaussian distributions were fitted to the log 10 MoM values of the biomarkers, and a common covariance matrix was assumed for these distributions. Analysis of residuals was used to check the adequacy of the model and assess the effects of maternal factors on log 10 -transformed MoM values in pregnancies with preeclampsia.
Five-fold cross validation was used to assess the performance of screening for preeclampsia that delivered at <37 weeks gestation (preterm-preeclampsia), ≥37 weeks (term-preeclampsia), and subgroups of preeclampsia that delivered at <32, 32 +0 -36 +6 , 37 +0 -39 +6 , and ≥40 weeks by models that combined maternal factors with individual biomarkers and their combination with screening by maternal factors alone. The data were divided into 5 equal subgroups; the model was then fitted 5 times to different combinations of 4 of the 5 subgroups and used to predict a risk of preeclampsia in the remaining one-fifth of the data. In each case, the maternal factor model, the regression models, and the covariance matrix were fitted to the training data set comprising four-fifths on the data and used to produce risks for the hold out sample that comprised the remaining one-fifth of the data.
The following screening strategies were considered: (1) the mini-combined test that comprised maternal factors, MAP and PAPP-A; (2) the biophysical test that comprised maternal factors, uterine artery PI, and MAP; (3) the biochemical test that comprised maternal factors and serum PLGF and PAPP-A, and (4) the quadruple test that comprised maternal factors and all 4 biomarkers. This choice covers the different combinations likely to be considered in clinical practice: the mini-combined test includes the least expensive biochemical and biophysical measurements; the biophysical and biochemical test may be preferred in ultrasound scanning only or laboratory-only settings, respectively; and the quadruple test combines all 4 markers. For each combination of biomarkers, backward elimination was used to determine the subset of biomarkers that contributed to the screening performance.
The statistical software package R was used for data analyses. The survival package was used for fitting the maternal factors model, and the package pROC was used for the receiver operating characteristic curve analysis.
Results
The characteristics of the study population are summarized in Table 1 .
Variables | Unaffected (n = 34,890) | Preeclampsia (n = 1058) | P value |
---|---|---|---|
Maternal age, y a | 31.3 (26.8–35.0) | 31.5 (27.0–35.6) | .34501 |
Maternal weight, kg a | 66.5 (59.0–77.0) | 72.1 (63.0–86.7) | .37555 |
Maternal height, cm a | 164.5 (160.0–169.0) | 163.2 (159.0–168.0) | .19445 |
Body mass index, kg/m 2 a | 24.5 (21.9–28.3) | 27.1 (23.5–32.1) | .66575 |
Gestational age, wk a | 12.7 (12.3–13.1) | 12.7 (12.3–13.1) | .19424 |
Racial origin, n (%) | < .00001 | ||
White | 25,315 (72.6) | 564 (53.3) | |
Afro-Caribbean | 6,287 (18.0) | 394 (37.2) | |
South Asian | 1,567 (4.5) | 56 (5.3) | |
East Asian | 829 (2.4) | 17 (1.6) | |
Mixed | 892 (2.6) | 27 (2.6) | |
Medical history | |||
Chronic hypertension | 421 (1.2) | 140 (13.2) | < .00001 |
Diabetes mellitus | 303 (0.9) | 22 (2.1) | .00008 |
Systemic lupus erythematosus/antiphospholipid syndrome | 48 (0.1) | 5 (0.5) | .01679 |
Cigarette smokers, n (%) | 3,195 (9.2) | 68 (6.4) | .00278 |
Family history of preeclampsia, n (%) | 1,428 (4.1) | 90 (8.5) | < .00001 |
Parity, n (%) | < .00001 | ||
Nulliparous | 16,739 (48.0) | 622 (58.8) | |
Parous with no previous preeclampsia | 17,028 (48.8) | 283 (26.8) | |
Parous with previous preeclampsia | 1,123 (3.2) | 153 (14.5) |
Distribution of preeclampsia according to gestational age at delivery
In the study population, there were 1058 pregnancies that experienced preeclampsia. The gestational age at delivery of these pregnancies was <32 weeks in 66 cases (6.2%), 32 +0 -36 +6 weeks in 226 cases (21.4%), 37 +0 -39 +6 weeks in 514 cases (48.6%) and ≥40 weeks in 252 cases (23.8%). Therefore, 292 of the cases (27.6%) of preeclampsia delivered at <37 weeks, and 766 cases (72.4%) delivered at ≥37 weeks.
Distribution of preeclampsia in parous and nulliparous women
In 17,361 of the 35,948 pregnancies (48.3%), the women were nulliparous; in 18,587 pregnancies (51.7%), they were parous, which included 1276 women (6.9%) with a history of preeclampsia in a previous pregnancy and 17,311 women (93.1%) without a history of preeclampsia. In the current pregnancy, preeclampsia occurred in 1058 cases (2.9%), which included 292 cases (0.8%) of preterm-preeclampsia and 766 cases (2.1%) of term-preeclampsia. The contribution of parous women was 45.2% (132/292) to preterm-preeclampsia and 39.7% (304/766) to term-preeclampsia; the respective values were 35.6% (47/132) and 34.9% (106/304) for parous women with preeclampsia in a previous pregnancy and 64.4% (85/132) and 65.1% (198/304) for parous women without a history of preeclampsia.
Distribution of biomarkers
The distributions of log 10 MoM values of the biomarkers in unaffected pregnancies and in those that experienced preeclampsia are shown in Tables 2 and 3 . The MoM values in the preeclampsia group and the fitted regression relationships with gestational age at delivery are shown in Figure 1 . All markers showed more separation at earlier, rather than later, gestations; this is reflected in their superior performance at detection of early, rather than late, preeclampsia. It is notable that the regression lines for uterine artery PI and PAPP-A intersect 1 MoM close to term. These markers show little or no discriminatory power beyond approximately 40 weeks gestation, and they perform relatively poorly in screening for late preeclampsia. Conversely, MAP shows a degree of separation from 1 MoM at term, and the performance of MAP for term preeclampsia is relatively good.
Marker | Intercept | Standard error | Slope | Standard error | P value |
---|---|---|---|---|---|
Uterine artery pulsatility index | 0.54453 | 0.05300 | –0.013143 | 0.001401 | < .0001 |
Mean arterial pressure | 0.095640 | 0.014420 | –0.0018240 | 0.0003811 | < .0001 |
Pregnancy associated plasma protein-A | –0.62165 | 0.09721 | 0.014692 | 0.002569 | < .0001 |
Placental growth factor | –0.93687 | 0.07573 | 0.021930 | 0.002002 | < .0001 |
Variable | No preeclampsia (95% confidence interval) | Preeclampsia (95% confidence interval) | Pooled (95% confidence interval) a |
---|---|---|---|
Standard deviation | |||
Uterine artery pulsatility index | 0.12852 (0.12757–0.12948) | 0.14234 (0.13653–0.14868) | 0.12894 (0.12801–0.12989) |
Mean arterial pressure | 0.03719 (0.03691–0.03746) | 0.03873 (0.03715–0.04045) | 0.03724 (0.03697–0.03751) |
Pregnancy-associated plasma protein-A | 0.23457 (0.23284–0.23632) | 0.26108 (0.25042–0.2727) | 0.23539 (0.23368–0.23712) |
Placental growth factor | 0.17645 (0.17515–0.17777) | 0.20141 (0.19318–0.21038) | 0.17723 (0.17595–0.17854) |
Correlation | |||
Uterine artery pulsatility index, mean arterial pressure | –0.05132 (–0.06178– –0.04085) | –0.05229 (–0.11223–0.00803) | –0.05133 (–0.06163– –0.04101) |
Uterine artery pulsatility index, pregnancy-associated plasma protein-A | –0.16039 (–0.1706– –0.15015) | –0.14735 (–0.20582– –0.08784) | –0.15992 (–0.16998– –0.14983) |
Uterine artery pulsatility index, placental growth factor | –0.14953 (–0.15977– –0.13925) | –0.18512 (–0.24271– –0.12623) | –0.15084 (–0.16093– –0.14072) |
Mean arterial pressure, pregnancy-associated plasma protein-A | –0.00565 (–0.01614–0.00484) | 0.01349 (–0.04685–0.07373) | –0.00497 (–0.01531–0.00537) |
Mean arterial pressure, placental growth factor | –0.02969 (–0.04017– –0.0192) | 0.02101 (–0.03933–0.08121) | –0.02791 (–0.03824– –0.01758) |
Pregnancy-associated plasma protein-A, placental growth factor | 0.31983 (0.31037–0.32923) | 0.34729 (0.2931–0.39925) | 0.32085 (0.31154–0.3301) |
a Estimates obtained from pooling data for the preeclampsia and no-preeclampsia groups.

Performance of screening for preeclampsia
The areas under the receiver operating characteristic curves and performance of screening for preeclampsia by maternal factors and biomarkers are given in Figure 2 and Tables 4 and 5 . The performance of each biomarker in combination with maternal factors was superior to that of screening by maternal factors alone. Similarly, the performance by a combination of ≥2 biomarkers was superior to that of screening by individual biomarkers. The only exception was serum PAPP-A, which did not provide significant improvement to any combination of biomarkers that included serum PLGF. Starting with the full model, incorporating maternal factors with all 4 biomarkers and applying backward elimination resulted in the removal of PAPP-A at the first step for both preterm-preeclampsia ( P = .15) and term-preeclampsia ( P = .98). In the backward elimination, after the removal of PAPP-A, all other variables made significant contributions ( P < .05). There is evidence therefore of a benefit for screening with the combination of maternal factors, uterine artery PI, MAP, and serum PLGF (triple test), but not for the inclusion of PAPP-A.

Method of screening | Preeclampsia | |||||
---|---|---|---|---|---|---|
<37 Weeks gestation | ≥37 Weeks gestation | |||||
Area under the curve | False-positive detection rate (95% confidence interval) | Area under the curve | False-positive detection rate (95% confidence interval) | |||
5% | 10% | 5% | 10% | |||
Maternal factors | 0.800 | 36 (30–41) | 49 (43–55) | 0.745 | 28 (24–31) | 38 (34–41) |
Maternal factors plus | ||||||
Mean arterial pressure | 0.845 | 44 (38–50) | 59 (53–65) | 0.781 | 30 (27–34) | 43 (40–47) |
Uterine artery pulsatility index | 0.841 | 46 (40–52) | 60 (54–66) | 0.749 | 29 (26–33) | 39 (35–43) |
Pregnancy-associated plasma protein-A | 0.822 | 40 (34–46) | 53 (48–59) | 0.748 | 28 (25–31) | 39 (35–42) |
Placental growth factor | 0.872 | 50 (44–56) | 65 (60–71) | 0.764 | 29 (25–32) | 42 (38–45) |
Mean arterial pressure, uterine artery pulsatility index | 0.876 | 53 (47–59) | 70 (64–75) | 0.785 | 32 (28–35) | 44 (41–48) |
Mean arterial pressure, pregnancy-associated plasma protein-A | 0.860 | 48 (42–54) | 61 (55–66) | 0.783 | 31 (28–35) | 45 (41–49) |
Mean arterial pressure, placental growth factor | 0.896 | 59 (53–64) | 73 (67–78) | 0.794 | 32 (29–36) | 47 (44–51) |
Uterine artery pulsatility index, pregnancy-associated plasma protein-A | 0.851 | 48 (42–54) | 60 (54–65) | 0.751 | 29 (26–32) | 40 (36–43) |
Uterine artery pulsatility index, placental growth factor | 0.884 | 58 (52–63) | 70 (64–75) | 0.766 | 30 (26–33) | 42 (38–46) |
Placental growth factor, pregnancy-associated plasma protein-A | 0.873 | 50 (44–56) | 66 (60–71) | 0.764 | 29 (25–32) | 42 (38–46) |
Mean arterial pressure, uterine artery pulsatility index, pregnancy-associated plasma protein-A | 0.884 | 55 (49–61) | 70 (65–75) | 0.787 | 32 (29–35) | 45 (41–48) |
Mean arterial pressure, pregnancy-associated plasma protein-A, placental growth factor | 0.897 | 59 (53–65) | 73 (67–78) | 0.794 | 32 (29–36) | 48 (44–51) |
Mean arterial pressure, uterine artery pulsatility index, placental growth factor | 0.906 | 65 (59–71) | 75 (70–80) | 0.796 | 33 (30–37) | 47 (44–51) |
Uterine artery pulsatility index, pregnancy-associated plasma protein-A, placental growth factor | 0.885 | 57 (51–63) | 69 (64–74) | 0.766 | 30 (26–33) | 43 (39–46) |
Mean arterial pressure, uterine artery pulsatility index, pregnancy-associated plasma protein-A, placental growth factor | 0.907 | 64 (58–70) | 75 (70–80) | 0.796 | 33 (29–36) | 48 (44–52) |
Method of screening | False-positive rate (95% confidence interval) | |||||||
---|---|---|---|---|---|---|---|---|
5% | 10% | |||||||
<32 Wk | 32 +0 – 36 +6 Wk | 37 +0 – 39 +6 Wk | ≥40 Wk | <32 Wk | 32 +0 – 36 +6 Wk | 37 +0 – 39 +6 Wk | ≥40 Wk | |
Maternal factors | 42 (30–55) | 34 (27–40) | 31 (27–35) | 21 (16–26) | 53 (40–65) | 48 (42–55) | 41 (37–45) | 30 (25–36) |
Maternal factors plus | ||||||||
Mean arterial pressure | 48 (36–61) | 43 (36–50) | 35 (31–39) | 21 (17–27) | 65 (52–76) | 58 (51–64) | 48 (44–52) | 34 (28–40) |
Uterine artery pulsatility index | 55 (42–67) | 43 (37–50) | 33 (29–37) | 21 (16–27) | 65 (52–76) | 58 (52–65) | 43 (38–47) | 31 (26–37) |
Pregnancy-associated plasma protein-A | 44 (32–57) | 39 (33–46) | 32 (28–36) | 20 (15–25) | 59 (46–71) | 52 (45–58) | 42 (38–47) | 31 (25–37) |
Placental growth factor | 67 (54–78) | 46 (39–52) | 33 (29–38) | 19 (15–25) | 80 (69–89) | 61 (54–67) | 45 (41–50) | 35 (29–42) |
Mean arterial pressure, uterine artery pulsatility index | 56 (43–68) | 52 (45–58) | 36 (32–41) | 23 (18–29) | 80 (69–89) | 67 (60–73) | 49 (44–53) | 36 (30–42) |
Mean arterial pressure, pregnancy-associated plasma protein-A | 52 (39–64) | 47 (40–54) | 36 (32–40) | 22 (17–28) | 65 (52–76) | 59 (53–66) | 50 (45–54) | 36 (30–42) |
Mean arterial pressure, placental growth factor | 76 (64–85) | 54 (47–60) | 36 (32–40) | 26 (21–32) | 83 (72–91) | 70 (63–76) | 53 (48–57) | 37 (31–44) |
Uterine artery pulsatility index, pregnancy-associated plasma protein-A | 59 (46–71) | 44 (38–51) | 33 (29–37) | 21 (16–27) | 68 (56–79) | 57 (50–64) | 43 (39–48) | 33 (27–39) |
Uterine artery pulsatility index, placental growth factor | 74 (62–84) | 53 (46–59) | 34 (30–39) | 21 (16–26) | 83 (72–91) | 65 (59–72) | 46 (41–50) | 35 (29–41) |
Placental growth factor, pregnancy-associated plasma protein-A | 67 (54–78) | 45 (39–52) | 33 (29–37) | 20 (15–26) | 79 (67–88) | 62 (55–68) | 46 (41–50) | 36 (30–42) |
Mean arterial pressure, uterine artery pulsatility index, pregnancy-associated plasma protein-A | 65 (52–76) | 52 (45–59) | 36 (31–40) | 25 (19–30) | 83 (72–91) | 66 (60–73) | 50 (45–54) | 35 (29–41) |
Mean arterial pressure, pregnancy-associated plasma protein-A, placental growth factor | 76 (64–85) | 54 (47–61) | 36 (32–40) | 26 (21–32) | 85 (74–92) | 69 (63–75) | 53 (48–57) | 39 (33–45) |
Mean arterial pressure, uterine artery pulsatility index, placental growth factor | 82 (70–90) | 60 (53–67) | 37 (33–42) | 27 (21–32) | 89 (79–96) | 71 (64–77) | 53 (48–57) | 38 (32–44) |
Uterine artery pulsatility index, pregnancy-associated plasma protein-A, placental growth factor | 76 (64–85) | 52 (45–58) | 34 (30–38) | 21 (16–26) | 83 (72–91) | 65 (58–71) | 46 (42–51) | 36 (30–42) |
Mean arterial pressure, uterine artery pulsatility index, pregnancy-associated plasma protein-A, placental growth factor | 82 (70–90) | 59 (52–65) | 37 (33–41) | 26 (21–32) | 89 (79–96) | 71 (64–77) | 54 (49–58) | 38 (32–44) |
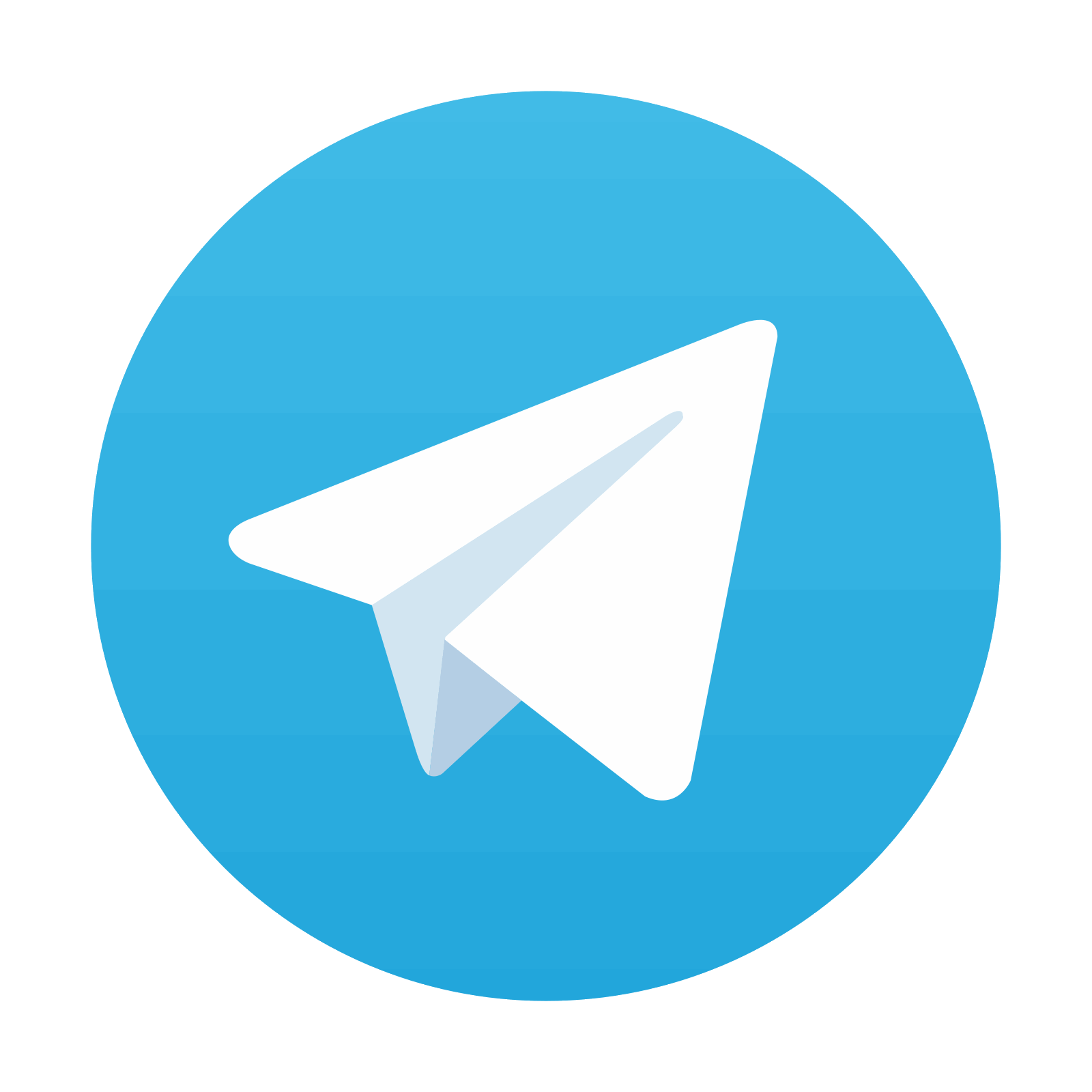
Stay updated, free articles. Join our Telegram channel

Full access? Get Clinical Tree
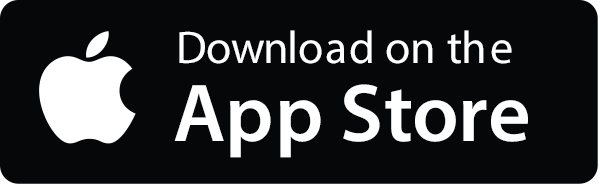
