Causation and causal inference are of utmost importance in obstetrics and gynecology. In many clinical situations, causal reasoning is involved in etiological explanations, diagnostic considerations, and conversations about prognosis. In this paper, we offer an overview of the philosophical accounts of causation that may not be familiar to, but still be appreciated by, the busy clinician. In our discussion, we do not try to simplify what is a rather complex range of ideas. We begin with an introduction to some important basic ideas, followed by 2 sections on the metaphysical and epistemological aspects of causality, which offer a more detailed discussion of some of its specific philosophical facets, using examples from obstetrical and gynecologic research and practice along the way. We hope our discussion will help deepen the thinking and discourse about causation and causal inference in gynecology and obstetrics.
Introduction
Causation is the concept that relates antecedents to consequences, or to put it in causal terms, determinants to their effects. As such, causal knowledge and the ability to infer causation from observed data (causal inference) play important roles in medicine, and therefore, in obstetrics and gynecology. In this paper, we focus on 3 main aspects of causal knowledge in biomedical research and clinical practice—etiology, pathogenesis, and prognosis—to examine what kind of knowledge do causal claims express and under what conditions are such claims warranted. These 3 aspects of causal knowledge bring different problems that causal claims face to light.
First, the etiology of an illness, or its causal history, needs to be understood to explain why certain diseases occur, so that interventions can be designed to reduce the health risks. But what exactly are we talking about when we talk about the causes of cervical, endometrial, or breast cancer? When does a factor count as a cause of an illness as distinguished from background factors that contribute to the manifestation of the illness without being a cause in any proper sense of the term? How do we establish the (probable) presence of a causal relationship if we don’t already know that a particular factor should count as a cause?
Second, the pathogenesis of a disease, or the underlying causal mechanism of a disease, needs to be considered when thinking about pharmacologic and surgical interventions. For example, how do we move from identifying intrauterine infection as a statistical risk factor (to use standard epidemiologic terminology) for preterm delivery to calling it a causal risk factor and a plausible target for medical interventions? What justifies the notion that the breast cancer ( BRCA ) genes play a causal role in the development of breast cancer, rather than being mere bystanders that are associated with an increased risk of developing breast cancer?
Third, knowledge about the causal influence of illness characteristics and therapeutic effects on clinical outcomes plays a crucial role in assessing and predicting the clinical course in a patient. In all these situations, causation is often mentioned as the primary basis of such predictions, but it is rarely explicated as a concept in terms of its nature and specific functions in the aforementioned etiologic, pathogenetic, and prognostic processes. When it comes to singling out the causes of an illness as the basis for predicting the future clinical course in a patient, it is crucial to have a clear understanding of how causal claims provide a basis for predictions, and therefore, what kind of knowledge causal claims express.
In all these 3 respects, there is a persistent lack of clarity regarding the meaning of causation and the conditions under which a particular factor can be singled out as a cause of an effect (rather than a mere background factor in the occurrence of the effect). Although many medical decisions are on the basis of beliefs about causal connections, the topics of causation and causal inference are rarely discussed in medical textbooks. Obstetrical and gynecologic texts are no exception. A part of the reason may be that causal questions are pondered in 2 fields of inquiry, namely epidemiology and philosophy of science, which are rarely considered by practicing physicians. Our goal in this article is to provide a broad, integrated overview of the rich epidemiologic and philosophical literatures on causation and causal inference and to offer a discussion of their aspects that bear importance for obstetrics and gynecology and for biomedicine more generally. We do not cover the legal concepts of causation.
In the remainder of this introduction (part I of the article), we briefly introduce some more or less undisputed preliminaries. In part II, we discuss the metaphysics of causation, asking what is meant when we use the term. Part III is devoted to the epistemology of causation, that is, the question of causal inference. Part IV is a brief conclusion.
Perspective on crucial concepts
The philosophical and epidemiologic literatures are vast, heterogenous in content and quality, and have only recently begun to connect. Many concepts and definitions are still discussed controversially. However, let us briefly lay some terminological groundwork and also touch on some characteristics of causation and causal inference that are (more or less) undisputed.
First, philosophers and epidemiologists distinguish between sufficient and necessary causes. Being a sufficient cause means that nothing but such a cause is needed for the effect to occur. Being a necessary cause means that without its presence the effect cannot occur. Rarely in biology or medicine is a cause both sufficient and necessary. For example, HIV infection is not sufficient for the development of AIDS, as evidenced by HIV long-term nonprogressors and “elite controllers” . However, HIV infection is necessary for the development of AIDS. Similarly, human papillomavirus (HPV) infection is not sufficient for the development of cervical cancer, as evidenced by a large proportion of females where the infection regresses or remains latent. However, with only a very few exceptions, HPV infection is necessary for the development of cervical cancer. This is because HPV triggers the malignant transversion of a vulnerable host cell that is compromised by other epidemiologic and genetic factors. Because these multiple host-related factors are generally weak and increase the risk by less than 2-fold, the strength of the association of the cancer with HPV has led to its description as a necessary cause and may have led to an underestimation of the role of genetics in this cancer. Conversely, breast cancer 1 (BRCA1) or breast cancer 2 (BRCA2) mutations may be largely sufficient for breast or ovarian cancer development (although there is still a reduced penetrance in carriers), but they are certainly not necessary.
Second, we have to distinguish between talk about the causal aspects of general events (what philosophers often call ‘type causation’), for example, the general causation of preeclampsia, and single events (often called ‘token causation’), such as the specific causation of the last patient’s case of preeclampsia. The practical value of this terminology is that distinguishing discussions about, for example, cancer causation in a single patient (token) from discussions about cancer causation in general (type), may reduce the likelihood that we inappropriately draw inferences from one to the other, for instance, in legal and forensic settings.
Third, we subscribe to a comprehensively multivariable view of causation. We hold that all causation in real life always involves multiple causal factors that together lead to the effect. Cases in which one single cause leads to a phenomenon are exceedingly rare and presumably only occur under highly controlled experimental conditions. This view was summarized for the health sciences in a way that integrates causal sufficiency and necessity as follows : Consider a set S 1 =(a, b, c) that represents a multicausal constellation with 3 components—causes a, b, and c—none of which is individually sufficient to cause a particular disease D. If D tends to occur when all the 3 component causes are present, we can say that they are jointly sufficient to cause D. If there is no other set of component causes that also causes D, all the 3 component causes are necessary for D to occur. If there are other sets that also cause D , that is, S 2 =(a, d, e) and S 3 =(a, e, f), all sets may be sufficient to cause D , but none is necessary, as each one of the others could do the same. In this situation, only a is a necessary causal component, as it is a member of all the sets of causes that cause D. We hold the view that it is usually multiple-component causes and conditions, and often multiple different sets of them, that contribute to the occurrence of health phenomena. We also hold that it is usually the joint function and interaction of multiple causal factors in a pathomechanism that culminates in clinical illness or changes a patient’s long-term outcome. Indeed, this is the main reason why it is so difficult, and sometimes impossible, to identify individual, independent causal risk factors. ,
Fourth, we will touch on a variety of philosophical accounts of causation in this paper. Ours, however, is a pragmatic pluralist position. This position acknowledges that there may be multiple fundamentally different accounts of causation that zoom in on, among other things, the regularity, probability, counterfactuals, manipulations, processes, dispositions, and mechanism-related aspects of causation. Paraphrasing Vandenbroucke, Broadbent, and Pearce, we think it is neither necessary nor useful to take a strong philosophical position about the nature of causation. Instead, we prefer what Illari and Russo have called a cheerful conceptual pluralism, that is, a pluralist view of causal concepts that do not necessarily all fit into a single, uniform metaphysical account of causation. Indeed, we consider ourselves agnostics with regard to the metaphysical question about whether or not there is something as one causal principle in nature that unifies all kinds of causation that we perceive and talk about.
Another kind of pluralism concerns what might be called as modes of causation in medicine. Genes, infections, environmental factors, social determinants, and even mental events clearly affect our health causally in different ways. But these are different ways of highlighting the possible kinds of factors that can play a causal role in the health sciences and are not necessarily different kinds of causation.
Fifth, we are no hardliners with regard to the distinction between a cause and a condition. In the philosophical literature a conceptual distinction is commonly made between the causes of an effect and its boundary conditions, which contribute to the occurrence of the effect but should not be seen as its cause. For practical purposes, however, our view is that irrespective of whether a given factor is best described as a cause or a condition, if it is worthy of consideration for the development of preventive or therapeutic intervention, we consider it worthy of consideration in biomedical discussions of causation and causal inference. Therefore, it is our opinion that in biomedical, epidemiologic, and clinical contexts, “the search (for causes) includes all the determinants of a health outcome.”
Metaphysics: What is Causation?
Let us begin with the metaphysical question: What does it mean to say that some factor is a cause of an event or a state of affairs? What is the contrast between causes and noncausal (but still relevant) factors that play a role in the occurrence of an event or a state of affairs? In other words, what are we talking about when we talk about causes and causation?
In this section, we give a sweeping overview of the philosophy of causation, focusing only on a few main aspects of causation that have been discussed in philosophy. We do this to give the reader some necessary background and pointers for further reading and to show how various aspects of the philosophy of causation connect to biomedical research and clinical practice. In particular, we aim to show how different conceptions of causation affect what researchers should be looking for when searching for the causes of diseases. Our discussion clearly involves a subjective selection from the vast philosophical literature on the topic. By no means do we consider this an exhaustive or even comprehensive overview, which is available elsewhere. , ,
Starting with Aristotle: 4 kinds of cause
The question regarding an event’s or object’s cause has been a topic of philosophical investigation since philosophy arose in ancient Greece. As is often the case in philosophy, a good place to start a discussion is with Aristotle. According to Aristotle, 4 metaphysically distinct kinds of causes as follows are always involved in the production of entities and events: a material cause, a formal cause, an efficient cause, and a final cause. An event’s material cause is the substance of which it is made; its formal cause is its essence (what kind of thing it is), which encompasses not only its shape but also other aspects of what makes it into the kind of thing it is; its efficient cause is its origin (in the sense of its maker or the process that produced it); and its final cause refers to the purpose for which it was made. A snowball, for example, is made of snow (material cause); it is roughly shaped like a sphere (formal cause); it originates from being formed by someone out of snow (efficient cause); and it exists for the purpose of being thrown (final cause).
For Aristotle, specifying an object’s 4 causes provide an exhaustive answer to the question what the object is. The snowball in our example would have been a different object if it had been made of sticky wet sand (it wouldn’t have been a snowball), if it had had the rough shape of a human being (it would have been a small snowman and not a ball), if it had been made by a different person than it actually was (it would have been a different snowball), or if it had been made for using as an ingredient in a snow cone (it would have been a culinary ingredient in dessert making). The third and fourth of these 4 points are probably the most controversial.
Regarding the third point (origins), consider the question whether a given human being would have been the same human being had he or she been born of different parents. Although there are different views on this issue in the literature (discussed under the keyword ‘origin essentialism’), most people would probably say that one’s biological parents make some difference with respect to who one is. For example, if on the day of a person’s fiftieth birthday, the person finds out that the parents who he or she was raised by aren’t his or her biological parents, nothing about the traits or the course of life of the person will change; yet, the person will have a different understanding of who he or she actually is. The importance of Aristotle’s efficient cause should be understood along these lines.
The fourth point can be clarified by looking at the question what determines what an artifact is. Philosophers of technology have long been concerned with the question to what extent do the designers,’ makers,’ and users’ intentions determine what kind of a thing an artifact is. Notwithstanding the differences of opinion in the relevant literature, it is clear that such intentions play some role in determining the identity of artifacts. For example, if someone molds a piece of clay into the prototypical shape of an ashtray with the intention of using it to collect the ashes of his favorite cigars, it is appropriate to say that the object is an ashtray. It was intended for this use from the start. But if the same person would have molded the same piece of clay into the same shape with the intention of using it to hold water for his dog, it seems appropriate to say that what the person made is a drinking bowl that looks a lot like an ashtray. In the latter case, it is what it was intended to be (a drinking bowl), and the fact that it looks like an ashtray is secondary.
Although Aristotle’s theory of 4 causes is strongly rooted in his metaphysical views and accordingly runs into problems when applied in contemporary scientific contexts where Aristotelian metaphysics often fails to fit, it can still be illuminating with respect to our question what we can mean when talking about causes. For one, asking what the cause of an object, event, state of affairs, or phenomenon is amounts to asking what kind of object, event, state of affairs, or phenomenon we are confronted with. The kinds of objects, events, states of affairs, or phenomena are inextricably connected to specific sets of causes, such that a given case that is not caused by precisely the kinds of causes associated with particular kinds of phenomena cannot be counted as a case of that kind.
Some authors have suggested that Aristotle’s (and his predecessor, Plato’s) concept of “cause” is close to that of “because,” and therefore, serves to explain the existence or occurrence of a phenomenon. , Indeed, the 4 kinds of causes refer to factors that are necessary for the phenomenon’s existence and that explain how it came into being. The snowball from our example would not have existed in the absence of the material from which it was made, it would not have existed if its maker had decided to give it the shape of a human being, it would not have existed if someone else had made it (as it would not have been the same snowball), and it would not have existed if its maker had intended to make ingredients for snow cones (as then it would not have been a snowball but something else).
Our current concept of “cause” in modern biomedicine is probably closest to the Aristotelian concept of “efficient cause,” that is, the “primary source of the change” in a situation. Whereas we do not have space to discuss Aristotle’s theory in further detail (and many of the details would not be relevant for our purposes in this paper), an important observation that can be drawn from Aristotle’s theory for biomedical research and clinical practice is that identifying a case as instantiating a particular kind of phenomenon inevitably involves identifying its underlying causes, and vice versa. The causes are what make a case that one is confronted with (a particular set of symptoms one finds in a patient) into a case of a particular kind (a case of a particular disease).
However, whereas asking what a phenomenon is and asking what its causes are inevitably go together, the answers to the 2 questions often diverge. In biomedical research and clinical practice, for example, it has long been clear that many diseases are multicausal and many causal factors underpin multiple diseases, such that it is not possible to simply map every phenomenon onto a unique set of causes. This means that even though Aristotle’s theory of causation provides some clarity regarding what we mean when talking about causes in the biomedical and clinical domain, it can only provide a part of the whole picture.
The idea of constant conjunctions
An important skeptical turn in thinking about causation occurred 2 millennia after Aristotle. The Scottish empiricist philosopher David Hume (1711–1776) noted that we do not have empirical access to the causes that underlie events but only to the conjunctions of events. That is, we can observe that a particular kind of event is typically followed by an event of another kind and on the basis of multiple such observations, we can hypothesize that the first of the 2 events, causes the second to occur, but we can never directly observe this causation relation between the 2 events. On Hume’s view, knowledge about causes is derived or inferred knowledge: if an event is regularly followed by another, the occurrence of the former event makes us think of the latter event (leads us to predict that the latter event will occur) and makes us assume that there is a causal connection between the 2 ( Enquiry Concerning Human Understanding , 7.2.29, pages 76–77). However, it is important to note that we do not possess knowledge about the causes themselves: when one event regularly occurs in conjunction with another event that follows it in time, the case might be that the former event directly causes the latter, or that it is merely an indirect cause connected to the other event by a chain of intermediate events, or they might both be caused by a third event that is hitherto unobserved.
This is a regularity account of causation, on which causation is understood in terms of the constant conjunction between one thing we call “cause” and another we call “effect.” We observe a temporal and spatial conjunction between 2 events in a multitude of cases (ie, an empirical regularity in the occurrence of distinct events), assume on the basis of these observations that this is a constant conjunction of the 2 kinds of events (ie, that events of these 2 kinds always occur together), and subsequently assume that there exists a (so far unspecified) causal connection between the 2. We call this “causation” by custom: we have not actually identified any causes but can still legitimately talk about causation because of the observed stability of the conjunction.
Hume’s German contemporary Immanuel Kant (1724–1804) famously confessed that Hume’s account of causation woke him from his “dogmatic slumber.” Kant’s work on causality is extensive, and it differs from Hume’s in that he appears to subscribe to a rather strong view of causation that posits that everything has a cause and that the observed regularity of causal processes suggests the presence of a deterministic causal law. Still, for Kant too, causes were located in our way of interpreting the world. Kant strongly subscribed to the view that we do not have any empirical access to the world as it is in itself; we only have access to the phenomena, that is, to the world as it appears to us. In the same way as for Hume, for Kant, knowledge about causes is derivative, as under certain conditions we interpret observed joint occurrences of events in causal terms.
An important problem with respect to the regularity accounts of causation was famously pointed out by Hume and discussed later by John Stuart Mill (1806–1873), among others. This is the problem of induction, the question on what basis we can make inferences from what has happened in the past to an expectation of what will happen in the future. The problem of induction is a core problem in epistemology and plays a central role in the philosophy of science to the present day. For example, if you have observed only black ravens until now, this does not justify the belief (ie, the inductive inference) that all ravens are black? Induction is fundamentally different from deduction, where the truth of a conclusion can be deduced from true premises as in the following: Jack and Jill are twins. Monozygotic twins are always of the same sex except if a mutation has occurred during development; therefore, Jack and Jill are not monozygotic twins unless a mutation occurred. A third kind of inference is inference to the best explanation championed by Gilbert Harmann and Peter Lipton. For example, if a pregnancy ends spontaneously with chorioamnionitis and preterm prelabor rupture of membranes, and the newborn sustains brain damage without any clinical or laboratory signs of hypoxia-ischemia, intrauterine infection would be a better explanation of the brain damage than subclinical hypoxia-ischemia.
Hume noted that every inductive inference necessarily rests on the assumption that nature is sufficiently uniform for the future cases of a particular phenomenon to be the same as the past cases ( Enquiry Concerning Human Understanding , Section iv). In other words, we can only reliably infer what future cases of a particular kind of phenomenon will look like, or how future instances of a particular process will behave, if we assume that there is a general uniformity to nature that manifests itself in natural processes, events, and so on. But, Hume observed, there is no possible foundation for such an assumption—all we can do is rely on nature to be sufficiently uniform (motivated by our past experiences that have shown us some degree of uniformity in nature) without being able to establish that this is indeed the case. Therefore, even if we could infer from the constant conjunction of 2 events that a causal relationship exists between them, this inference, strictly speaking, only applies to the past. The future might well be different.
Mill saw causation as Hume did, as a mere sequence of events that we consider causal on the basis of repeated observation and experience, but he also proposed a “law of causation,” which states that an “invariability of succession is found by observation to obtain between every fact in nature and some other fact which has preceded it” ( A System of Logic , ASL, Book III, chapter 5, §2) . However, we are also inclined to infer from past observations the belief that similar connections will be happening in the future just as they do now and as they did in the past. As Mill puts it, “we should believe not only that the antecedent always has been followed by the consequent, but that […] it always will be so” (ASL, Book III, ch.5, §6; italics in original). We face this problem whenever we want to learn from observed data: what justifies the inferential leap from past experience (as seen in the data) to future situations that are similar but have (by definition) not yet occurred, or, for that matter, even to past situations that have not been observed?
In his discussion of causal inference, Hume concluded, after much deliberation, that “appeal to past experience decides nothing in the present case” ( Treatise of Human Nature 1, III, 6) . In contrast, we almost always rely on past experience when planning our future. We often base our decision-making in one clinical case on our own experience with a previous one, and we typically are unable to avoid relying on past experiences of our own and of others. Perhaps, this led philosopher Bertrand Russell (1872–1970) to state that if the problem of induction is indeed insoluble, “there is no difference between sanity and insanity.” However, if we agree with Russell, we have to answer the question what else above and beyond observation and experience we have at our disposal as a foundation for causal inference. We will explore this issue in the next section.
Before turning to this issue, several aspects of the regularity views of causation are noteworthy. First, contrary to Aristotle’s account of causes (which specifies the nature of 4 distinct kinds of causes), regularity accounts do not tell us what causes are. Rather, they tell us what causal inferences are and that such inferences, strictly speaking, do not yield knowledge about causes, but are nevertheless legitimate under certain conditions (namely in cases in which stable constant conjunctions are observed).
Second, a regularity account does explain why the search for correlations is a crucial aspect of biomedical research: when searching for causes, we should look for associations because the existence of an association is the only empirically accessible indication we have of possible causation. However, the account also complicates matters in this respect, because when it comes to finding causal relations, we have nothing more to go by than correlations. Although a crucial aspect of biomedical research consists of differentiating between mere correlations between factors and actual causal relations between them, a regularity account seems to give us reasons to give up hope in this respect.
Third, however, a regularity account does seem appropriate to biomedical research in that all possible causal factors are treated equally. Many philosophical accounts of causation aim to distinguish between the cause of an event and the background factors that were necessary for the event to occur, but they should not be singled out as causes. This is because the philosophical accounts of causation are often aimed at clarifying why we consider certain factors but not others to be causes of events. A very basic example is the igniting of a match that is struck on a rough surface. We usually say that the striking of the match caused it to ignite, not the presence of oxygen in the air and the sulfur in the match’s head (even though oxygen and sulfur are necessary factors for the event’s occurrence). But in biomedical research, which is often aimed at interventions that prevent effects from occurring, it matters less when we would say that a factor is a cause or that it is merely a background factor. With respect to the question how we can prevent a match from igniting, preventing it from being struck is on a par with preventing oxygen from being present–both in principle could work, even though the latter is much harder to implement than the former. In research aimed at finding potential interventions rather than identifying the cause of a phenomenon, looking for regularities suffices and no account of the nature of causes is required. This is particularly true in cases of strong host-environment interactions, such as the interaction of alcohol intake with a deficiency in alcohol dehydrogenase, which causes a severe disease that would not happen with either factor alone. Such relationships can become very complex in multifactorial diseases such as preeclampsia, which seem to involve different inflammatory pathways triggered by the impaired invasion of the trophoblast and modulated by genomic and environmental factors, including genome variation ( s-flt ) and maternal nutritional status (vitamin D).
Still, the fundamental problem of distinguishing associations on the basis of causal connections and accidental correlations remains an important issue in biomedical research, even if it is less important when searching for effective interventions, entailing the need for a more refined view of causation.
Counterfactual reasoning
It is easily demonstrated that there must be more to causation than just the regular succession of antecedent and consequent. For example, every instance of intrauterine device (IUD) implantation has so far been followed, sooner or later, by an episode of sleep. Therefore, we can indeed postulate a Humean constant connection between IUD implantation and falling asleep. Do IUDs really have a narcotic effect? Probably not, because of the simple counterfactual recognition that had the same person not have an IUD implanted they would have, at some point, fallen asleep just as well. This is an example of counterfactual reasoning, that is, the more or less well-founded speculation about what would have occurred if a particular factor had not been present, comparing it with what happens when that factor is present.
Counterfactual reasoning lies at the heart of counterfactual accounts of causation. On such accounts, a factor counts as a cause of an event if the event would not have occurred without the occurrence of the factor in question. Counterfactual accounts treat factors equally in the same way as regulatory accounts do (provided above): if I had not struck the match, it would not have ignited, but if no oxygen and sulfur had been present, it would not have ignited either. Accordingly, counterfactual reasoning is a mainstay of the potential outcomes framework that currently dominates causal inference in the health sciences.
Counterfactual views of causation also face difficulties, however. For one, it is valid to ask the question how we would ever know that an event that has in fact occurred would not have occurred had a particular factor not been present. This poses what has been called the “fundamental problem of causal inference (…) causal effects are defined as a contrast of the values of counterfactual outcomes, but only one of these outcomes is observed.” The answer to this problem clearly is the comparison of cases in which a particular factor is present with cases in which that factor is not present but all else is equal—something that often, but not always, can be done. All contrastive study designs in epidemiology and clinical research, such as cohort studies, case-control studies, randomized trials etc., can be seen as a methodological response to this problem. But comparisons can only achieve so much—they can provide indications of causal relationships but they cannot provide much insight into the nature of this relationship. An additional perspective on causation is required to achieve such deeper insight and we turn to such a perspective in what follows.
From laws to mechanisms
Three decades before Russell published the comment cited above, he had written what is arguably one of the most frequently-cited quips on causation: “The law of causality, I believe, like much that passes muster among philosophers, is a relic of a bygone age, surviving, like the monarchy, only because it is erroneously supposed to do no harm.” This is sometimes interpreted as a call to abandon the idea of causation. However, it is merely the above-mentioned law of causality, as proposed by Mill, that Russell suggests abandoning. Instead, he asks not to focus on the “sameness of causes and effects, but on sameness of relations” (page 14). Russell rejects the assumption that the same cause always leads to the same effect (and thus that we would infer causation on the basis of constant conjunctions in which events of one kind tend to be followed by events of another kind). Russell instead suggests that we should focus on the relations between phenomena and that causation should be thought of as a relation of determination between 2 factors or events: a factor can be said to cause an event if the factor determines the occurrence of the event, for example, if there is a pattern of covariation of the factor and the event. Examining the relations that are obtained between phenomena will thus be more helpful when searching for causal connections that examining the extent to which different kinds of phenomena co-occur. Russell considered differential equations as the only way to describe such relations, which suggests that he thought of the relation between cause and effect as deterministic. This would dovetail with a mechanistic conceptualization of causation in medicine, in which causes lead to health outcomes in a particular disease-specific pattern by means of a machine-like mechanism. This is what we call a pathomechanism—a process that can be traced from cause to outcome. In the philosophy of science, mechanistic views of causation have flourished in the past 2 decades. , Some of the more influential formulations of a mechanism are Glennan’s (“[a] mechanism underlying a behavior is a complex system which produces that behavior by of the interaction of a number of parts according to direct causal laws”) and Machamer, Darden, and Craver’s (“[m]echanisms are entities and activities organized such that they are productive of regular changes from start or set-up to finish or termination conditions.”) William Bechtel has suggested a version of a mechanistic view that accommodates feedback loops that clearly matter in biology and medicine.
If we adopt a mechanistic account of causation in medicine, the cause determines the outcome (1) by producing it (via a mechanism), so that (2) the outcome somehow depends on the cause. This account posits that (3) a cause makes a difference to the outcome, which can be exploited such that (4) manipulation of the mechanism will make a difference to the occurrence or clinical trajectory of the outcome. Although the first 3 characteristics are mere descriptors of the relation between cause and effect, the fourth is a bit of an inferential leap that will need to be supported by pertinent evidence. Note that characteristics (1), (2), and (3) can be seen as concretizations of Russell’s suggestion to focus on relations, whereas characteristics (2), (3), and (4) reflect aspects of the counterfactual view of causation. After all, an outcome’s dependence on a cause, entails that the outcome will not occur without the cause being present (2), a cause’s making a difference to an outcome encompasses that the presence or absence of the cause also makes a difference to the outcome (3) and manipulation of the mechanism may involve disabling the cause (4).
An approach focusing on causal production rather than on causes simpliciter postulates the existence of a mechanism that connects the cause and its effect. The cause is like the first click in a causal machine, the functional task of which is to bring about the effect. Illari, using an example offered by Wesley Salmon, states that tracing the link between pushing a button and the garage door opening depicts this sense of causation as the production of an effect. Moreover, in this sense, the outcome somehow depends on the cause: the outcome does not occur without the cause (the garage door does not open if the button is not pushed), and in many cases, the intensity of the effect depends on the intensity of the cause. This sense of causation as machine-like production is, we believe, at the core of the current medical thinking about causation. We also think that health scientists have a primarily mechanistic understanding of causal processes and look for evidence that supports the postulation of such mechanisms that will justify interventions that interfere with etiological and pathogenetic processes in helpful ways. The associations between phenomena (as emphasized in the regularity account of causation) help to identify cases in which such mechanisms may be found.
Probabilistic views of causation
The philosophical discussion of causation took a bit of a technical turn in the first half of the 20th century. Arguably, the same happened in biology and medicine. Hans Reichenbach (1891–1953), a philosopher of science, wrote extensively about causation, often in the context of discussions of probability. He offered both an (earlier) epistemic and a (later) metaphysical account of causality. The earlier account states that “the claim of causality in its most general form is nothing but the scientific method of ordering facts somehow, thereby becoming a claim about regularity in nature” (translation O.D.). Reichenbach emphasizes the importance of a functional relationship between a cause and its effect, which resonates with Bertrand Russell’s definition of causation in terms of differential equations cited above and the mechanistic view of causation. The repetitive nature of situations in which such function materializes, said Reichenbach, is used to infer that it applies to all similar situations (page 32). He acknowledges that this is some sort of extrapolation from the observed to the unobserved and asks whether it is possible to test such causal claims empirically (pages 34 and 35). Obviously, this is Hume’s induction problem all over again (provided above).
Reichenbach outlined a “probabilistic” conceptualization of causal claims. He thereby laid the groundwork for what are now called probabilistic theories of causation. Reichenbach’s main distinction was between causal and statistical laws, which he considered as 2 different “special forms of a more general claim which posits the validity of laws of probability in reality” (page 63). This view of causation involves thinking of causes as increasing the probability that their effects will occur such that one can say “smoking causes lung cancer, not because all or only smokers develop lung cancer, but because smokers are more likely to develop lung cancer than nonsmokers.” Indeed, this approach defines causation by referring to the observation that Hume’s and Mill’s views of causation as a constant conjunction of cause and effect simply don’t hold in many cases. Particularly in biology and medicine, events may co-occur regularly, but often not in a rigid, deterministic fashion. In the life sciences, regularities are almost never perfect.
This view of causation is, of course, closely related to the medical view of disease risks. We will take BRCA1 and BRCA2 as a prominent example to discuss the problem. It is neither the case that all women with a certain mutation in BRCA1 or BRCA2 develop breast or ovarian cancer, nor it is that all women with breast or ovarian cancer will have such a mutation. While the latter is known as polygenic predisposition, the first has been called incomplete penetrance. A large study comparing BRCA1 or BRCA2 mutation carriers born before and after 1940 has shown considerable differences in the age-dependent penetrance, suggesting life-style factors as a modifier of disease risk in BRCA1 and BRCA2 mutation carriers. Moreover, a recent genome-wide study has uncovered additional genetic variants that modulate the disease risk of BRCA1 and BRCA2 mutation carriers. Thus, BRCA1 and BRCA2 are not the sole causal factors for breast or ovarian cancer and need to be seen in a multifactorial context.
However, more interesting may be the question whether BRCA1 and BRCA2 mutations can be termed as a cause at all. Neither clinicians nor most biomedical scientists would doubt this claim. But there may be reasons of philosophical concern. One minor aspect is the fact that the germline variants are in the heterozygous state and need a second hit in the respective cell to initiate the tumor. So, the putative cause would be the biallelic deficiency rather than 1 mutation. But more importantly, BRCA1 and BRCA2 are repair proteins. Therefore, there must be unrepaired damage that because of the biallelic deficiency is not repaired to develop the breast or ovarian tumor. Indeed, every tumor cell that has so far been characterized harbors functional genomic alterations compared with its normal precursor cell. These genomic alterations, as a bunch of “teamwork bandits,” are necessary and sufficient for driving tumor development (on the condition that specific hallmarks of cancer are targeted). The source of these genomic alterations can be stochastic errors in replication, genotoxic intermediates of the cellular metabolism, or environmental DNA-damaging agents. Consider a cell that would be error-free in its replication and cell division, would quickly scavenge and consume its metabolic products, and would live happily in a danger-free environment: BRCA1 and BRCA2 would have little to do here, and their mutation may remain without any consequence. From this point of view, one could rather claim the continuously damage-prone situation of the cell as the cause of the cancer than the deficiency of BRCA1 or BRCA2 . Is it the fire that causes the catastrophe, or do we blame the absence of the fire brigade? The genetic mutation merely increases the risk for breast or ovarian cancer but does not determine it. Epidemiologic risk analyses that focus on measures of association such as risk ratios, hazard ratios, or odds ratios are based on statistical concepts that appreciate these probabilistic phenomena. Indeed, one could argue that successful risk alteration is a unique epidemiologic-biostatistical approach to causal inference—to which we will now turn our attention.
Epistemology: causal inference
The discussion in the preceding section has clarified a number of aspects that are commonly associated with causes and that we briefly summarize before examining causal inferences. First, causes do not just underlie a phenomenon but also contribute to defining what a phenomenon is (Aristotle). That is, knowing the cause of a particular phenomenon implies knowing (to some extent, at least) what kind of phenomenon one is confronted with. Second, for a factor to count as a cause of a phenomenon, it must always, or at least very often, be present whenever the phenomenon is observed (conjunction), and the phenomenon should not occur (or typically not occur) whenever the factor is not present (counter factuality). These latter 2 aspects are represented in the mechanistic views of causation and the views of causation in terms of the production of effects as follows: a mechanism that produces a cause constitutes the connection between causes and an effect, and the absence of a cause from the mechanism will affect the functioning of the mechanism, and hence, the production of the effect.
Note, though, that even in a mechanistic or production view of causation, the aspects of causation discussed in the preceding section remain important. Not every factor in a mechanism necessarily counts as a cause of the effect after all, nor does only the first factor that starts up the mechanism (the door opener button in the example discussed above) count as “the” cause of the effect. On a mechanistic or production view, too, we have to infer which factors should count as causes of the effect. This brings us to the problem of causal inference: we now move from the metaphysical question that asks what causation is (or, what causes are), to the epistemological problem how to conclude that an observed association is causal on the basis of mere experience. Causation is the purported causal relation between a cause and an effect, whereas causal inference is inferring causation from an observed association. How do we establish that “correlation is causation” in a particular context or even in general?
The main methodological distinction between approaches to causal inference in epidemiology and medicine is between quantitative and qualitative analysis. The quantitative approach is mainly about the counterfactual interpretation of observed data on the basis of potential outcomes reasoning (POR) , and Bayesian reasoning (BR). Both rely heavily on mathematical techniques such as multivariable regression analysis (eg, in POR) and extended probability calculus (in BR).
The qualitative approach tackles causal inference from a different angle. In some ways it does incorporate POR- and BR-based approaches, but it does not replace them. The best example of this qualitative perspective is the one put forward by Austin Bradford Hill in his classic article “The environment and disease: association or causation.” In this article, Hill proposed to look at observational epidemiologic data from 9 viewpoints to decide whether an observed association is causal—among them strength of association, consistency of findings, time order of purported cause and effect, experimental evidence, and consistency. Hill’s proposal was deeply rooted in the work by many others during the decade before his publication. One of Hill’s viewpoints that not just stands among the other viewpoints, but also qualifies as an overarching concept uniting all 9 is coherence. One of the authors of the present article (O.D.) has previously argued that the wide appeal of the Hill heuristics originates from the sheer strength of explanatory coherence of evidence. Indeed, the method has been shown not to derive from the arguments made by Hume or Mill, but to be a genuinely epidemiologic approach to causal inference. To see the Hill approach in action, look no further than to a causal question only too familiar to obstetricians, that is, whether the association between Zika infection in pregnancy and neonatal microcephaly is causal. Hill’s viewpoints have been adopted and modified by others with the goal to reduce their complexity while preserving their epistemological value. ,
It goes far beyond the scope of this paper to discuss these methods in further detail. However, 4 major concepts need to be explicated to grasp the most important aspects of causal inference in the health sciences: production, difference-making, statistical causality, and intervention. These aspects should not to be viewed as separate, but as overlapping and integrated.
Production and difference-making
At the core of the health science approach to causal inference is the idea of a contrast. Identifying such contrast, which is a difference between a healthy state and a state in which an intervention is required to return a person to the healthy state, is the initial step. The notion of difference-making thus features prominently in epidemiologic definitions of cause. As stated most clearly by Mervyn Susser (1921–2014), “(i)n a pragmatic perspective, a cause is something that makes a difference.” In a similar vein, Ken Rothman and Sander Greenland describe the difference made by causes in this counterfactual way: “(A) cause of a disease event is an event, condition, or characteristic that preceded the disease event and without which the disease event either would not have occurred at all or would not have occurred until some later time.” On this epidemiologic view, background conditions can be considered as causes. For example, maternal obesity appears to play a causal role in the occurrence of developmental disability in the offspring. We think what matters most in the present context is to determine whether one can make a difference to the outcome by intervening on the etiological factor in question, whether it is a cause or a condition.
Difference-making is a conceptualization of causation that is sometimes discussed in juxtaposition with the production-accounts of causation. Philosopher Peter Godfrey-Smith gives 2 examples as follows: a bullet producing the victim’s injury and the failure to issue a warning to the pilots of 2 airplanes on a collision course making a difference to the course of events. These can be viewed as being equivalent to examples such as intrauterine infection producing preterm labor and the failure to perform a cesarean delivery at the appropriate time as making a difference to the neonatal outcome. One difficulty with this separation of causal concepts into production and difference-making is that, at least in our view, causal producers and causal difference-makers both make an observable difference; conversely, making a difference amounts to making a difference in the way in which the effect is produced and often also in the effect that is produced. Thus, the distinction is not too helpful when it comes to causal inference, and production and difference-making are probably best seen as 2 different aspects of causation. Still, keeping the 2 conceptually separate is helpful when thinking about the ways to interfere with the therapeutic and preventive goals in mind.
Role of Statistics in Causal Inference
Much of the causal inference in the health sciences in on the basis of mathematical modeling such as statistical hypothesis testing, machine learning algorithm fitting, or the construction of Bayesian causal networks. This literature is not as readily accessible as, for example, the clinical epidemiologic literature; nevertheless, it is highly illuminating. , , It promises to help in situations where the “gold standard” for causal inference in epidemiology and medicine—the randomized trial—is either infeasible, as exposing study participants to a potentially harmful intervention is simply unethical, or it is apparently epistemologically unnecessary as indicated by the classic systematic review that established the glaring absence of randomized trials designed to establish the preventive effect of parachutes from the medical literature. Instead, much of the causal inference in statistics is devoted to the situation where we have to make do with data from observational studies. Of course, this bears the risk of drawing wrong conclusions from a spurious co-occurrence of unrelated factors (“cum hoc ergo propter hoc”) or from an unobserved factor that influences the observed factors. Here, 1 of the strongest factors working against our desire to infer causation from observation is confounding.
Confounders are factors (or variables) that distort our perception (on the basis of statistical results) of a causal estimate such as a risk ratio or odds ratio. , Confounders do that by being statistically or causally associated with both the risk factor and the health outcome under investigation. For example, if an observational study identifies caffeine intake as a risk factor for low birthweight and caffeine and nicotine exposure are assessed by a questionnaire, it can be expected that caffeine will seem to be a risk factor independent of smoking, because smoking is socially less accepted than caffeine intake and the smoking response on the questionnaire, therefore, is potentially less truthful than the information on caffeine intake.
Reichenbach (provided above) analyzed causation in terms of the temporary relationship between the purported causes and their effects in time. He proposed a Common Cause Principle, according to which if the joint occurrence of 2 events is more frequent than expected by chance, either one event is the cause of the other or there is a common cause of both events. , In statistical and epidemiologic language, a common cause is a confounder.
Multiple methods have been proposed for causal analyses using statistical methods. Following the paradigm of the randomized trial as the gold standard, these are mainly design and analysis methods that emulate data randomization in situations where this is not possible. One such method is the use of propensity scores; another is Mendelian randomization, , which is feasible only in observational studies that have comprehensive and pertinent genetic information on all study participants readily available. The interested reader is encouraged to consult this “consumer’s guide” when thinking through the potential pitfalls posed by big data analytics in obstetrics and gynecology.
Among philosophers, the randomized trial as the gold standard perspective has not been universally accepted without resistance. Perhaps the most prominent critic among the randomized controlled trial (RCT) skeptics is John Worrall, who published a provocative piece in 2007 entitled “Why there is no cause to randomize.” In his thoughtful article, Worrall joins forces with an earlier critic, Peter Urbach and concludes that “the problems arise—just as Peter Urbach’s earlier treatment claimed—when randomization is treated as a sine qua non of scientific ‘validity.’ When pressed, even the most avid advocate of randomization admits that this is not true, and yet, the medical profession is encouraged to act as if it is true” (page 486, italics in original). Another philosopher of science, Nancy Cartwright, has written extensively about randomized trials and summarizes her views eloquently in a paper with Angus Deaton as follows: “RCTs can play a role in building scientific knowledge and useful predictions but they can only do so as part of a cumulative program, combining with other methods, including conceptual and theoretical development, to discover not ‘what works,’ but ‘why things work.’” This need for combining RCT with other methods also follows from our discussion of the philosophical accounts of causation in the preceding section. There, we pointed out that contrastive studies can provide indications of causal relationships but cannot provide a deeper insight into the nature of the causal relationship. To obtain such a deeper insight, additional perspectives such as mechanistic views and views of causes as producing effects on causation must be brought into play.
This is a symptom of the general epistemological role of statistical analyses in biomedical research: they enable us to find correlations between events that are strongly indicative of causal relations, but they do not allow us to establish that there is a causal relation of the sort we are looking for. After all, statistically, strongly correlated events may also be connected by common causes in Reichenbach’s sense (ie, confounders) or may not be causally connected at all but may be merely accidental. Strictly speaking, then, it does not make sense to speak of statistical causality. Rather, we should think of statistical correlations as indications of possible causality, where most often, additional methods are required to establish the actual existence of causal connections, while “in just the right circumstances and with just the right kind of starting information it is in principle possible (to get from probability to causes)” (page 35).
In biomedical settings such as the assessment of HPV vaccination for the prevention of cervical cancer and its precursors, 1 RCT is not considered sufficient to establish the efficacy of an HPV vaccine against HPV-induced disease; a series of similar trials with the same vaccine in slightly different populations is required, although a causal relationship may already be reflected by 1 positive RCT. Evidence hierarchies such as the one provided by the Centre for Evidence-Based Medicine rates the evidence provided by systematic reviews of RCTs higher than evidence from a single RCT ( https://www.cebm.ox.ac.uk/resources/levels-of-evidence ). A more sophisticated evidence rating scale—the Grading of Recommendations Assessment, Development, and Evaluation —has been developed. In contrast, philosopher Jacob Stegenga has raised multiple strong arguments against the use of evidence hierarchies.
Intervention
If a cause is mechanistically connected with a health outcome, manipulation on the cause should make a difference to the outcome. Of note is that manipulation of the causal mechanism would also make this difference. As we have argued before, this does not necessarily require elimination of the cause. For example, a cause may no longer be a productive cause (of, let’s say, a recurrence) when new medications or therapies have entered clinical practice. Interventions that potentially help a patient (ie, make a desired difference to the outcome) need to be identified. Obviously, manipulation (doing, as in “First, do no harm.”) has therefore always been at the center of medical thinking. Indeed, some authors take the idea of manipulation as central to moving from observed data (and statistical analyses that identify possible causal connections) to well-established causal claims, and for that matter, as central to the scientific approach per se. As statistician Donald Rubin states, “There exists no causation without manipulation.” (p. 238)
Some authors also acknowledge that it is not necessarily the randomization but the intervention that carries the actual epistemological weight in randomized trials. Philosopher James Woodward, for example, suggests that a reasonable causal explanation of a phenomenon would be provided by a feasible intervention (the cause) that leads, under ideal experimental conditions, to an appreciable change in the outcome of the intervention (the effect). The crucial idea here is that events can occur in different ways according to variations in their causes. For example, smoking causes cancer not in a simple absence-presence way of causation (ie, absence of the cause correlates with absence of the effect and presence of the cause correlates with presence of the effect), but in a way in which the degree of the cause affects the degree of the effect (heavy smoking increases the likelihood of getting cancer more than light smoking). Interventions on the cause, thus, do not necessarily lead to the effect not occurring but to a different way in which the effect occurs. In this sense, the epistemological weight in RCTs lies more with the intervention than the randomization as follows: the degree of the intervention (eg, administering several different dosages of a medication in a trial) is much more informative than the randomization (which is a methodological background condition that has to be satisfied for the intervention to be informative). Perhaps the most problematic aspect of this view is that it requires interventions to be merely possible in principle: a factor can be cited as a causal explanation of a phenomenon if a manipulation of the factor is possible in principle that would change the way the phenomenon occurs. But as this view is intended as a philosophical account of causation and not as a guide to finding causes in practice, this should perhaps not be taken as a weakness of this view. Further discussion can be found in current philosophical work on the topic. For example, Alexander Reutlinger has offered a critique of Woodward’s account and a counterproposal, and a comprehensive overview of manipulation theories of causation is provided in Henning Strandin’s doctoral thesis “Taking Control.”
Conclusion
Causation and causal inference have been studied and discussed for a long time in metaphysics, epistemology, and the philosophy of science, and they are focal topics in epidemiology at least since the mid-20th century. Still, the theoretical basis of these concepts is often side-tracked or even neglected in biomedical research and clinical medicine. Many aspects of clinical obstetrics and gynecology and their scientific underpinnings hinge on knowing a clear concept of what a cause is, under what conditions can factors that contribute to a phenomenon be considered as causes of the phenomenon (rather than merely contributing background conditions), and how we identify a cause when we see noteworthy correlations. We strongly believe that a serious reflection of different accounts of causation as provided by various areas of research in philosophy will be helpful to obstetricians and gynecologists in their daily practice and in academic research. We hope that the review of the main philosophical aspects of causation and causal inference and our discussion of how these pertain to biomedical research and clinical practice has opened a door for this.
Supplementary Data
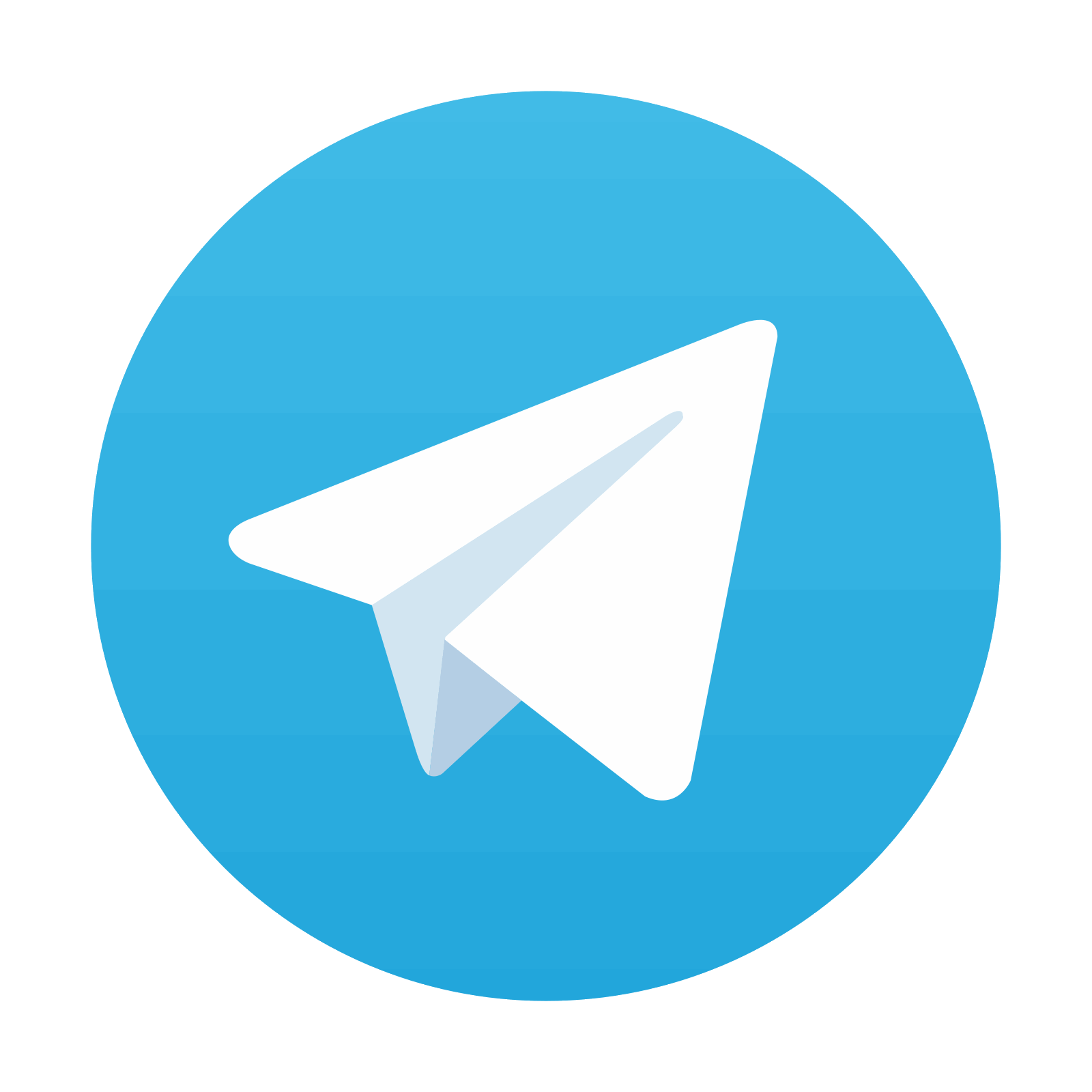
Stay updated, free articles. Join our Telegram channel

Full access? Get Clinical Tree
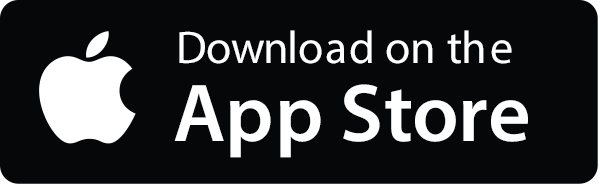

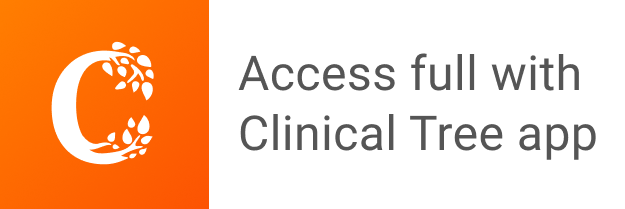