Objective
The purpose of this study was to develop a reliable model for the calculation of gestational age (GA) in second and third trimesters with the use of amniotic fluid (AF) metabolite profiles that were determined by magnetic resonance spectroscopy.
Study Design
High-resolution (11.7 T) ex vivo magnetic resonance spectroscopy was performed on 95 AF samples (mean, 31.7 weeks; range, 15.6–39.9 weeks). GA was determined by last menstrual period or first-trimester ultrasound scanning. Concentrations of 21 AF metabolites were measured with automated techniques. Metabolite concentrations, inverses, natural logs, and squares were entered as predictive variables in a stepwise linear regression model.
Results
The following formula was derived: GA = 64.922 – (14.456 × alanine) + (4.965 × natural log [creatinine]) – (0.931 × glucose) – (5.202 × valine). This model fit the data with an R 2 value of 0.926. Average error among all samples was ±1.75 weeks (SD, ±1.43 weeks), for the second trimester was ±2.21 weeks (SD, ±1.78 weeks), and for the third trimester was ±1.59 weeks (SD, ±1.26 weeks).
Conclusion
Statistical modeling accurately predicted GA with amniotic fluid metabolite profiles that were obtained by magnetic resonance spectroscopy, which may represent a significant improvement over conventional ultrasound dating in the third trimester. Future studies should compare these techniques directly.
Reliable information about gestational age (GA) is necessary for clinicians to assess fetal growth accurately and to interpret certain screening and diagnostic tests and is critical for management of pre- and postterm pregnancies. It has been demonstrated that improved estimates of GA have led to a decrease in women being identified with postterm pregnancies or requiring induction of labor. As a result, improvements in dating have been associated with significant reductions in both fetal and maternal morbidity and mortality.
GA can be predicted with the last menstrual period (LMP), ultrasound-based techniques, or gross estimates based on clinical examination. However, the accuracy of these methods varies significantly and is subject to misclassification bias as a result of incorrect patient recall of LMP, oligo- and polyovulation, and timing of ultrasound examinations. The inaccuracy of GA computed by LMP has been well documented, with reported “estimated date of confinement” errors of ±1.37 weeks in recent studies. To calculate GA by ultrasound examination, biometric measurements (eg, fetal limb length, biparietal diameter, and crown-rump length) are compared with GA-specific references. It has been established that early ultrasound examination that uses crown-rump length provides a more accurate estimate of GA, compared with LMP dating. The crown-rump length measurement produces an accurate prediction of the estimated date of confinement with an error of ±0.67 weeks.
It has been demonstrated that the improved accuracy of first- and early second-trimester ultrasound, compared with LMP, is the result of the small variability in fetal growth rates early in pregnancy. Fetal biometry in the third trimester, however, is subject to much greater individual size variations, hence the higher ranges of error in estimation of GA by ultrasound examination in the late second and third trimesters. Previous studies have shown that the utility of ultrasound examination in the third trimester is limited, with estimated date of confinement errors of up to ±3.6 weeks.
For women who come for prenatal care late in gestation or arrive in labor at sites where prenatal clinical history is unavailable, accurate assessments of GA are often unattainable. In this high-risk population, a reliable and robust method for the assessment of GA in the latter half of pregnancy would help guide management and could significantly improve fetal and maternal outcomes.
High-resolution magnetic resonance spectroscopy (MRS) of human amniotic fluid is a potentially useful method for the evaluation of fetal health and development. Ex vivo analyses with MRS are rapid, require minimal sample preparation, and can be used to quantify several metabolites simultaneously. Previous studies have identified numerous magnetic resonance–detectable metabolites and have demonstrated significant variations in amniotic fluid metabolite profiles as GA advances. These amniotic fluid metabolite profiles are the result of the byproducts of fetal pulmonary, renal, and gastrointestinal development. Therefore, these profiles may contain biomarkers that depend on organ system development and can be used to assess GA independently of the variations in fetal size.
In this study, we performed high-resolution (11.7 T) ex vivo MRS on amniotic fluid samples from women who underwent clinical examination of fetal lung maturity or karyotyping to develop a statistical model that is predictive of GA. The objective of our study was to develop a reliable model for the calculation of GA late in pregnancy, with accuracy superior to existing modalities.
Materials and Methods
Population
The accessible population included women in the San Francisco Bay Area in their second or third trimesters of pregnancy who were examined at the University of California, San Francisco Hospitals, San Francisco General Hospital, or a regional referral center for amniocentesis for fetal karyotype analysis or to determine fetal lung maturity. The sample population included all members of the accessible population whose amniotic fluid samples were processed and stored by the University of California, San Francisco Chemistry or Cytogenetics Laboratories during a 12-month period (June 1, 2007 to May 31, 2008). Samples of multiple gestations, abnormal karyotype, inborn errors of metabolism, known renal malformations, and samples without “certain LMP” or first-trimester ultrasound dates were excluded. All samples were collected for clinical indications, and a waiver of consent that satisfies federal regulations for Protection of Human Subjects and Standards for Privacy of Individually Identifiable Health Information for analyses of existing biofluids was granted for this study. This protocol was reviewed and approved by the University of California, San Francisco Institutional Review Board.
Sample acquisition
Ninety-five amniotic fluid specimens (mean, 31.7 weeks; range, 15.6–39.9 weeks) were identified retrospectively and transferred from their initial storage locations to −80°C freezers until use. No first-trimester samples were included. GA was determined by crown-rump length at the time of first-trimester ultrasound examination, or certain LMP dates were confirmed by a second-trimester ultrasound examination in instances in which no first-trimester ultrasound results were available, per the standard of practice within our obstetric clinics. Demographic information is listed in Table 1 .
Demographic | Measure |
---|---|
Maternal age, y | |
Mean | 31.82 ± 6.29 |
Range | 16-51 |
Gravidity | |
n | 87 |
Mean | 3.31 ± 2.23 |
Range | 1-13 |
Parity | |
n | 87 |
Mean | 1.28 ± 1.40 |
Range | 0-7 |
Ethnicity, n | |
Black | 12 |
Asian/Pacific Islander | 17 |
White | 28 |
Hispanic/Latina | 20 |
Middle Eastern | 4 |
Undisclosed | 14 |
Comorbidity, n | |
Advanced maternal age, ≥35 y | 30 |
Chronic myelogenous leukemia | 1 |
Congestive heart failure | 1 |
Diabetes mellitus | |
Type 1 | 13 |
Type 2 | 13 |
Gestational class A2 | 4 |
Fetal gastroschisis | 4 |
Hypertension | 8 |
Hypothyroidism | 2 |
Neural tube defect | 5 |
Nonalcoholic fatty liver disease | 1 |
Preeclampsia | 3 |
Sickle cell anemia | 2 |
Reference metabolite spectra
A reference database was created that contained quantitative one-dimensional spectra of the 21 most common and routinely quantifiable metabolites in amniotic fluid and urine and metabolites that are associated with fetal maturity. The database included quantitative spectra of 3-hydroxybutyrate, acetate, alanine, betaine, choline, citrate, creatine, creatinine, glycerophosphocholine, glutamine, glutamate, glucose, isoleucine, lactate, leucine, lysine, phosphocholine, phosphocreatine, pyruvate, succinate, and valine. All chemicals were purchased from Sigma-Aldrich (Sigma, Aldrich, Fluka and Supelco, St. Louis, MO). Stock solutions of each compound were prepared with 1× phosphate buffered saline solution and pH adjusted (between 7.0 and 8.0) with the use of concentrated hydrochloric acid or sodium hydroxide to match the physiologic pH of amniotic fluid. Frequency shifts because of differences in pH between reference metabolites and amniotic fluid samples were accounted for in each spectrum by the high-resolution quantum estimation (HR-QUEST) fitting algorithm. Metabolite solutions of known concentrations (between 0.01 and 100.0 mmol/L) were diluted to approximate physiologic concentrations found in human amniotic fluid. Samples were prepared for analysis and spectral data were acquired as described later.
MRS protocol
Proton ( 1 H) MRS was performed at 11.7 T (500 MHz for 1 H), 1°C, and a 2250-Hz spin rate with a spectrometer (Inova; Varian Inc, Palo Alto, CA) equipped with a 4-mm gHX nanoprobe under high-resolution magic angle spinning (HR-MAS) conditions. Amniotic fluid samples were thawed to room temperature and vortexed for approximately 5 to 10 seconds. To provide a frequency and lock reference, 3.0 μL of deuterated water (D 2 O) that contained 0.75% trimethylsilyltetradeuteriopropionate (TSP) was pipetted into a 35-μL volume zirconium oxide rotor and weighed to ±0.01 mg, after which the amniotic fluid was added to the rotor and weighed. The rotor was carefully assembled to prevent leakage and placed into the probe for analysis. Quantitative 1-dimensional spectra were acquired with a 90° pulse, 4-second presaturation of the water peak, 2-second acquisition (total repetition = 6 seconds), 256 transients, 40,000 points, 20,000-Hz spectral width, for a time of 26 minutes. Metabolite concentrations were determined with the Electronic Reference To access In vivo Concentrations (ERETIC) method. The ERETIC signal was created using the “Pandora’s Box” pulse-generating tool (Varian Inc, Palo Alto, CA) and transmitted during the acquisition period with 0 dB of power, a full width at half height of 3.5 Hz, and an offset frequency equivalent to −0.5 ppm. The ERETIC signal was phase cycled in synchronization with the receiver to maintain phase coherence across scans. At the beginning of each acquisition, the phase of the ERETIC signal was adjusted manually to match the other signals in the spectrum. The ERETIC signal was calibrated daily with a standard amount of D 2 O plus TSP.
Quantification
Spectra were processed and displayed with the Advanced Chemistry Development 1-dimensional nuclear magnetic resonance processor (ACD/Labs, Toronto, Ontario, Canada), the Java-based graphical user interface for the magnetic resonance user interface quantitation package, and MATLAB software (The MathWorks, Natick, MA) and were quantified with the use of a custom version of the semiparametric quantum estimation program that was developed by Ratiney et al, which was adapted for analysis of short-echo time HR-MAS spectra that contained 40,000 points (HR-QUEST). The first 2 points of each free-induction decay were backward linear-predicted in the Advanced Chemistry Development processor with the use of base points 3-1000 and 32 coefficients. Each spectrum was then saved as a text file and imported into the Java-based graphical user interface for the magnetic resonance user interface. The spectra were reversed, phased, and referenced to TSP. Spectral regions downfield of 4.75 ppm (including residual water) and upfield of −1 ppm were deleted. The HR-QUEST program estimated the macromolecule signals using the Hankel-Lanczos singular value decomposition algorithm and iterated between fitting the metabolites and modeling the macromolecules 12 times. HR-QUEST results were displayed in MATLAB, and metabolite peak areas were then exported into an Excel spreadsheet (Microsoft Corporation, Redmond, WA) and converted to concentrations with a conversion factor for the ERETIC signal, as previously described.
Statistical analyses
The linear relationship between concentration and GA was examined for each metabolite. Correlation coefficients (and R -squares) were calculated in the usual fashion. Metabolite concentrations (mmol/kg), their corresponding inverses, natural logs, and squares were entered as explanatory variables (84 total variables) in a stepwise multiple linear regression model (stepwise forward procedure) with GA as the outcome variable. These additional permutations were included in the model to allow for possible nonlinear changes in metabolite concentration with respect to time. To account for multiple comparisons, terms were retained or dropped at a significance level of probability of .001. Missing values were input based on the mean metabolite concentration for the entire study population. Linear regression modeling and descriptive statistics were performed with a statistical software package (SPSS Inc, Chicago, IL). Calculated GA for each of the 95 amniotic fluid samples was determined on the basis of individual metabolite concentrations and the predictive formula that was produced from the analysis described earlier. Documented GA was determined by crown-rump length at the time of the first-trimester ultrasound scan or known LMP in instances in which no first-trimester ultrasound results were available as described in the “Sample acquisition” section of this article. Differences between calculated GA and documented GA were determined for each sample. Average error and SDs were calculated for all samples (n = 95). Separate error calculations for second (n = 24) and third (n = 71) trimester subgroups were also performed.
Results
Figure 1 shows an example of a high-resolution ex vivo MRS obtained from a third-trimester amniotic fluid sample. Selected peaks are labeled to demonstrate metabolite overlap and spectral complexity. The water resonance at approximately 4.8 ppm is beyond the scale of the graph, which shows the region from 0.5–4.5 ppm.

Concentrations for the 21 metabolites within each of the 95 amniotic fluid samples are presented in the “ supplemental data ” section at the end of the article. The strongest independent linear relationship between GA and metabolite concentration were found with alanine ( R 2 = 0.796), lysine ( R 2 = 0.624), glutamate ( R 2 = 0.581), pyruvate ( R 2 = 0.574), valine ( R 2 = 0.564), and glucose ( R 2 = 0.533). The R 2 for each of the remaining metabolites was <0.500. For each of the metabolites listed above, we observed a trend toward decreasing concentration with advancing GA. This trend was not true for all compounds.
Table 2 displays results from the stepwise multiple linear regression model. This model yielded a significant additive contribution from 4 of the 84 variables. Six of 380 values that were used in the ultimate regression model were derived from imputed data (0 for alanine, 4 for creatinine, 1 for glucose, and 1 for valine). The final prediction formula follows: GA = 64.922 – (14.456 × alanine) + (4.965 × natural log [creatinine]) – (0.931 × glucose) – (5.202 × valine). When applied sequentially, the combination of alanine, the natural log of creatinine, glucose, and valine created a linear prediction model that fit the data with an R 2 value of 0.926.
Step | Metabolite | R | R 2 | P value |
---|---|---|---|---|
1 | Alanine | 0.892 | 0.796 | < .001 |
2 | Natural log creatinine | 0.932 | 0.869 | < .001 |
3 | Glucose | 0.952 | 0.907 | < .001 |
4 | Valine | 0.962 | 0.926 | < .001 |
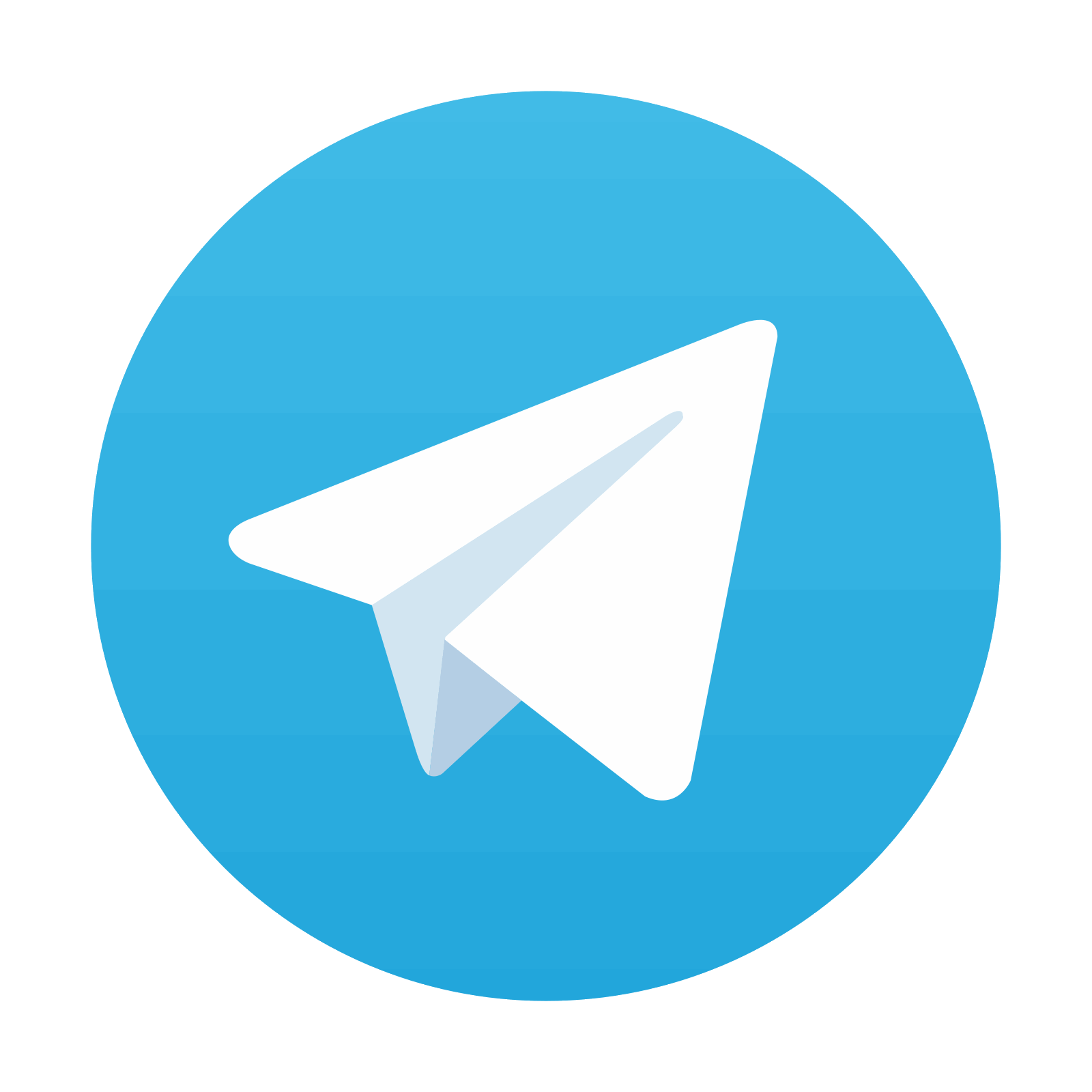
Stay updated, free articles. Join our Telegram channel

Full access? Get Clinical Tree
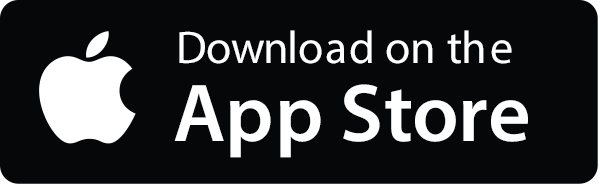

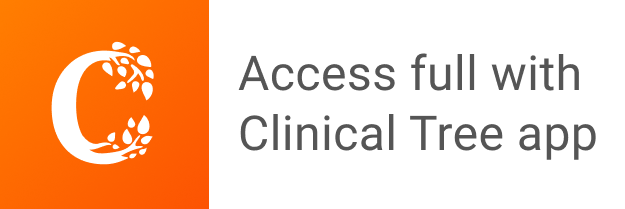