The volume of scientific articles being published continues to grow. Simultaneously, the ease with which both patients and providers can access scholarly articles is also increasing. One potential benefit of easy accessibility is broader awareness of new evidence. However, with a large volume of literature, it is often difficult to thoroughly review each article. Therefore, busy clinicians and inquisitive patients may often read the conclusion as their take-away message. In this article, we provide an overview of a few challenging study designs that are at increased risk for over- or understating conclusions, potentially leading to changes in clinical practice.
Availability of high-quality medical literature is critical to providing excellent health care. The number of studies being published and types of study designs being utilized have significantly increased in the past 10 years. As with any situation, the benefits of accessing numerous articles with the click of a mouse is balanced by the challenges of adeptly reviewing the data from each study presented. Every study design has specific advantages and limitations that have an impact on its conclusions. Therefore, when stating study conclusions, it is vital that authors accurately report the study findings without over- or understating its implications.
For the practicing clinician, it can be challenging to thoroughly analyze multiple studies, determine applicability, and ultimately decide whether there is sufficient evidence to warrant a change in clinical practice. This prompts physicians to rely heavily on the author’s stated conclusions to assist in the interpretation of study findings. In addition, because patients can also access articles through the Internet, they may also take conclusions made at face value. This challenges practicing physicians to have a thorough understanding of the literature to appropriately address patient questions and concerns. Therefore, the onus and responsibility of accurately reporting the study design, statistical analyses, and conclusions rest heavily on the investigators, peer reviewers, and editorial boards of journals to ensure that study conclusions do not go beyond what accurately reflects the methods and results of a study.
Studies generally fall into 2 broad categories: analytic studies and descriptive studies. Study subtypes exist within these categories. Analytical studies include clinical trials, retrospective and prospective cohort studies, and case control studies. Descriptive studies include analysis of secular trends (eg, ecological studies), case series, and case reports. Certain study designs are more challenging to power, analyze, and interpret. In this clinical opinion, we will discuss some designs and their respective analytical methods. Hopefully this will increase awareness of the potential limitations and implications pertaining to these study designs.
Changing times influences study designs
Historically most studies were based on the concept of superiority or establishing a difference between 2 or more exposure or treatment groups. In a superiority trial design, the null hypothesis states that the 2 exposures being studied are equal, and the alternative hypothesis states that the 2 exposures being studied are different. For example, when comparing intervention A to intervention B, the null hypothesis would be there is no difference in the outcome between the 2 interventions, and the alternative to that is noticing 1 intervention has a superior or better outcome than the other. In this scenario, type I (alpha) error occurs when the study incorrectly concludes the interventions are different (rejects the null). A type II (beta) error occurs when the study incorrectly concludes that the outcomes are similar between the interventions or fails to conclude that the outcomes are different (rejects the alternate hypothesis).
In current practice, multiple interventions may already exist for various clinical scenarios. In such situations, it may be unethical to withhold treatment or use a placebo for comparison. This has led to a dramatic increase in the number of comparative effectiveness research studies (studies assessing a direct comparison of existing interventions). Instead of demonstrating superiority, these trials seek to conclude whether a new treatment is equivalent to or at least no worse than a second or reference treatment within a preset margin. These studies are referred to as equivalence or noninferiority designs.
Interestingly, compared with superiority trials, noninferiority trials are significantly more complex to design. In noninferiority trials, the null hypothesis is that the new treatment is inferior to the standard of care, and as such the null and alternative hypotheses are reversed in this type of trial compared with a superiority design. In other words, when comparing interventions A and B, and presuming intervention A is the standard of care, the null hypothesis would be that B is an inferior intervention compared with A.
The alternate hypothesis then would note that B is not inferior or is superior or equal to A. This is completely the opposite of the superiority trial. Hence, the type 1 (alpha) error in this trial represents the erroneous rejection of the null hypothesis, B is worse than A, and the type II (beta) error is the erroneous rejection of the alternate hypothesis, that B is not inferior to or is superior or equal to A ( Table ). The practical implication of this reversal of type I and type II errors is generally different standards for acceptable errors, which results in the requirement of a much larger sample size to demonstrate that treatments or exposures are similar or that one is not inferior to the other.
Study | H 0 (null hypothesis) | H A (alternate hypothesis) | Type 1 error (erroneous rejection of null hypothesis) | Type II error (erroneous rejection of alternate hypothesis) |
---|---|---|---|---|
Superiority | Intervention A = B | Intervention A ≠ B | Erroneously reject the truth A = B | Erroneously reject truth A ≠ B |
|
|
|
Erroneously reject truth B less than A | Erroneously reject truth B = A or greater |
The other challenging component involved in calculating sample size for a noninferiority trial depends on the choice of the clinically acceptable difference between the 2 groups being compared, otherwise known as the margin of noninferiority. This margin should be smaller than the clinically relevant effect with the aim to prove similarity. Furthermore, some would argue that the true goal of a noninferiority trial is not only to demonstrate no worse effects than the reference treatment but also to indirectly infer superiority to placebo through a comparison with an intervention already proven to be superior to placebo. Therefore, if outcomes with intervention A are better than placebo by 10%, then a new intervention B might need to be at least 5% better than placebo and thus no more than 5% worse than intervention A.
In situations in which effective trials have been performed comparing placebo with current accepted clinical regimens, this information can add to the process of setting the margin of noninferiority. However, it is not unusual for current treatments or interventions to be considered standard of care because of years of clinical efficacy without accurate trials comparing placebo vs current treatment. This increases the difficulty in accurately setting a margin of noninferiority. In these situations, combining available objective data and expert opinion is necessary in setting a clinically acceptable noninferiority margin.
Whereas equivalence trials share similar methodologies with noninferiority trials, the aim of an equivalence trial is to determine whether one intervention is truly equivalent to another for a particular outcome. Equivalence is often incorrectly inferred when the results are within a preset margin. To state equivalence, a much smaller margin or observed difference between the 2 groups is required, which results in larger sample size requirements than are necessary for a noninferiority trial. Therefore, noninferiority does not imply equivalence and the 2 terms are not interchangeable.
An obstetrics example of comparative effectiveness research: conclude with caution
One recent study by Khandelwal et al, attempted to establish noninferiority between 12 and 24 hour dosing intervals of antenatal steroids. Although this study proposed to determine noninferiority, it is unclear whether the appropriate type I and type II errors were utilized to adequately power this study. For example, to accurately demonstrate noninferiority, a higher threshold for power is typically assumed over and above what is used for a standard superiority study, most commonly 90% or higher. However, this example study assumed a power of 80%. Furthermore, a margin of 20% was set to observe noninferiority for the primary outcome of respiratory distress syndrome (RDS) between the 2 strategies being compared. Based on guidelines, that margin is a bit too generous for a noninferiority trial.
Whenever possible, data from published meta-analyses or use of clinical judgment should be used to set a noninferiority margin that could be designated a minimally important difference. Therefore, given the present margin, achieved power, and sample size, it is difficult to conclude noninferiority with such a large allowable difference in the outcome between the 2 groups. By reducing the noninferiority margin to 10% and raising the power to 90%, a sample size of 225 patients should have been randomized to each group. This is about 3 times larger than the study sample size 161 and 67 patients in the 2 groups. Despite these issues, the conclusions stated that 12 hour dosing “is equivalent to 24-hour dosing.”
As mentioned earlier, a noninferiority trial cannot conclude equivalence, and a noninferiority margin of 20% would allow neither the conclusion of noninferiority or equivalence. In addition, this study was also unable to definitively demonstrate no harm with this dosing interval. Inarguably, studying the dosing interval of antenatal corticosteroids inherently lends itself to a noninferiority study design. However, this study by Khandelwal et al illustrates the difficulties of accurately powering a study to demonstrate noninferiority and the potential clinical pitfalls in concluding no difference or equality in 2 treatments.
Another component of understanding noninferiority or equivalence involves reviewing the necessary statistical analysis. Superiority trials are analyzed based on the intention-to-treat principle. In this process, patients are grouped according to their initial randomization, regardless of whether they received the intervention or treatment. This analysis errs on the side of biasing findings toward no difference (the null hypothesis). However, in a noninferiority trial, an intent-to-treat analysis alone would not be clinically acceptable because this is the hypothesis being tested is trying to prove similarity or no difference.
Whereas, generally, an as-treated analysis may bias the results toward the null hypothesis (ie, inferiority), it is possible that bias toward noninferiority can occur when deviation from treatment is related to treatment efficacy. It is therefore important to consider the impact of both approaches: intent-to-treat and as-treated analyses.
A study analyzing induction vs expectant management of intrauterine growth restriction from the Disproportionate Intrauterine Growth Intervention Trial at Term (DIGITAT) data attempted to establish equivalence between these 2 clinical modalities. This study appropriately switched the alpha and beta error in establishing power. However, the analysis was performed based on only intention-to-treat principles. Therefore, their conclusion stating no difference was noted between the induction of labor group and expectant monitoring group could be untrue because the intention-to-treat analysis in this study would bias the results toward an erroneous conclusion of no difference. This further demonstrates the complexity involved in accurately designing and executing noninferiority and equivalence trials to draw appropriate conclusions that are clinically meaningful and useful.
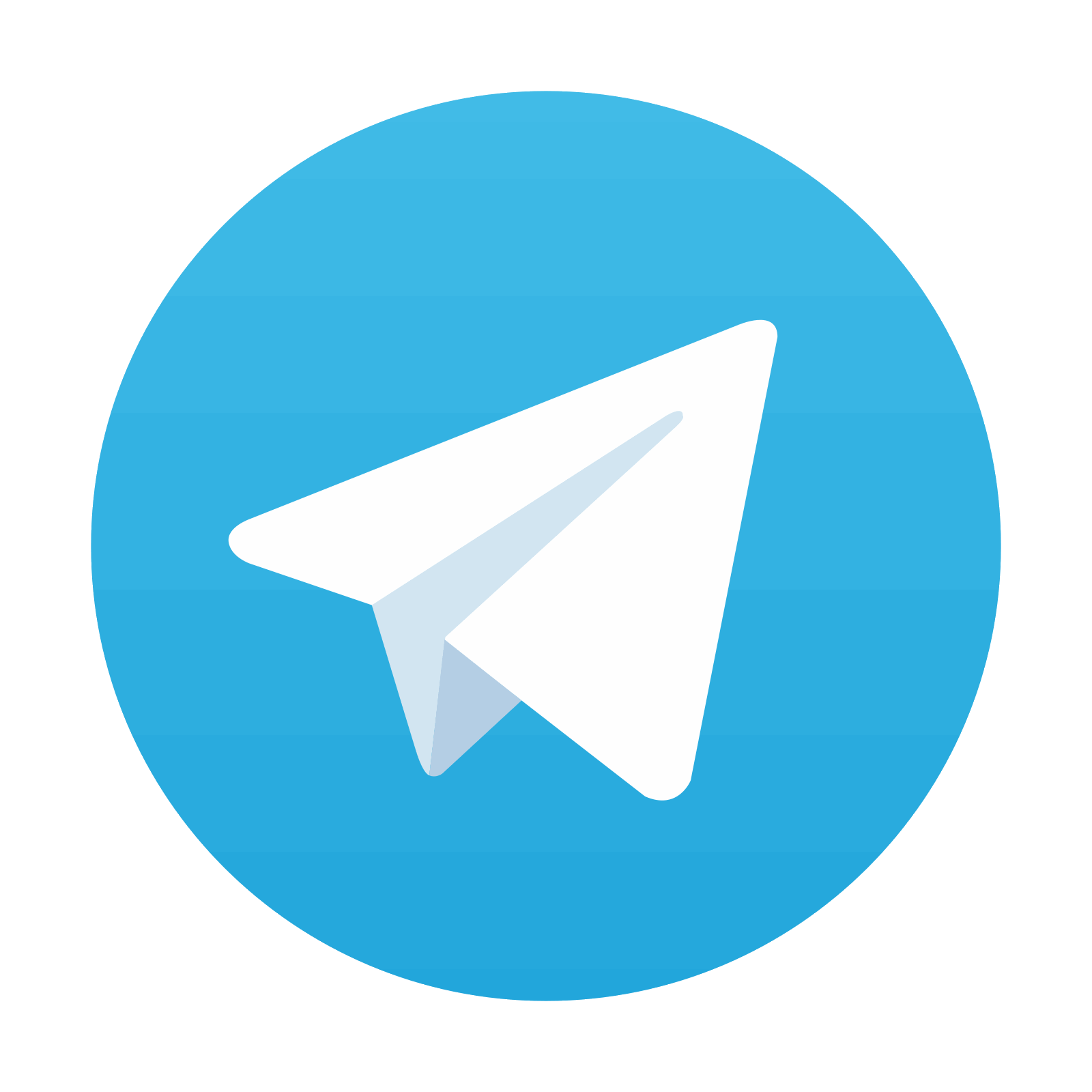
Stay updated, free articles. Join our Telegram channel

Full access? Get Clinical Tree
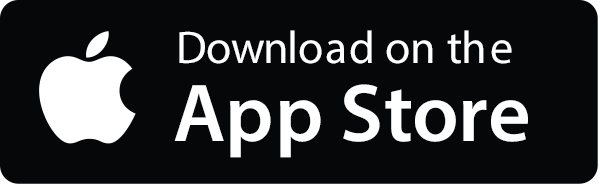
