Background
Identification of preterm births and accurate estimates of gestational age for newborn infants is vital to guide care. Unfortunately, in developing countries, it can be challenging to obtain estimates of gestational age. Routinely collected newborn infant screening metabolic analytes vary by gestational age and may be useful to estimate gestational age.
Objective
We sought to develop an algorithm that could estimate gestational age at birth that is based on the analytes that are obtained from newborn infant screening.
Study Design
We conducted a population-based cross-sectional study of all live births in the province of Ontario that included 249,700 infants who were born between April 2007 and March 2009 and who underwent newborn infant screening. We used multivariable linear and logistic regression analyses to build a model to predict gestational age using newborn infant screening metabolite measurements and readily available physical characteristics data (birthweight and sex).
Results
The final model of our metabolic gestational dating algorithm had an average deviation between observed and expected gestational age of approximately 1 week, which suggests excellent predictive ability (adjusted R-square of 0.65; root mean square error, 1.06 weeks). Two-thirds of the gestational ages that were predicted by our model were accurate within ±1 week of the actual gestational age. Our logistic regression model was able to discriminate extremely well between term and increasingly premature categories of infants (c-statistic, >0.99).
Conclusion
Metabolic gestational dating is accurate for the prediction of gestational age and could have value in low resource settings.
Identification of preterm birth and accurate estimates of gestational age (GA) for newborn infants is vital for several reasons. These estimates can provide guidance as to what treatments and investigations are most appropriate for the newborn infant and can assist with accurate assessments of neurocognitive development. Unfortunately, in developing countries, it can be challenging to obtain estimates of GA because of a lack of prenatal ultrasound dating and unreliable patient recall of menstrual period history. Obtaining accurate estimates of GA has been recognized by the Gates Foundation as a priority for infant health. As part of their Grand Challenges Explorations 13 competition entitled “Explore New Ways to Measure Fetal and Infant Brain Development,” the Foundation sought new approaches for measuring GA accurately at birth to support the creation of developmental standard curves.
We postulated that a newborn infant’s GA could be estimated from newborn infant analyte values in conjunction with other readily available information, such as sex and birthweight. Analyte data are obtained from examination of dried blood spot samples taken from heel pricks typically used for newborn infant screening. Our hypothesis stemmed from our previous work that revealed a metabolic distinction between preterm children and term children, as indicated by patterns of amino acids and endocrine markers at birth. We identified that metabolic patterns varied depending on the degree of prematurity. Therefore, in this study, we sought to develop an algorithm that could estimate GA at birth, based on the analytes that are obtained from newborn infant screening.
Methods
Design
We conducted a population-based cross-sectional study to predict GA with the use of newborn infant screening analyte data and readily available physical characteristics from infants who were born in the province of Ontario, Canada.
Data
We included data for infants who were born in Ontario, Canada, from April 1, 2007, to March 31, 2009, who completed newborn infant screening. Virtually all infants who are born in Ontario undergo newborn infant screening via heel prick blood spot, which is typically obtained between 24 and 72 hours of age. The Newborn Screening Ontario (NSO) program screens each infant for 29 conditions with the use of a panel of screening analytes, most of which are measured by tandem mass spectrometry. The exceptions are 17 hydroxyprogesterone (17OHP) and thyroid-stimulating hormone (TSH), which are measured using a fluorescent immunoassay (autoDELFIA, Perkin Elmer, Waltham, MA); biotinidase, measured using a colorimetric enzyme assay (Spotchek Pro; Astoria-Pacific, Inc, Clackamas, OR); and galactose-1-phosphate uridyltransferase (GALT) measured by fluorescent enzyme assay (Spotchek Pro). The analyte levels for all infants who complete screening are available in the NSO database. Broadly, the newborn infant screening analytes include acyl-carnitines, amino acids, endocrine markers, and markers of biotinidase deficiency and galactosemia ( Table 1 ).
Acyl-carnitines | C0, C2, C3, C4, C5, C6, C8, C8:1, C10, C10:1, C12, C12:1, C14, C14:1, C14:2, C16, C18, C18:1, C18:2 |
Amino acids | arginine, phenylalanine, alanine, leucine, ornithine, citruline, tyrosine, glycine, argininosuccinate, methionine, valine, biotinidine |
Fatty acid oxidation | C3DC, C4DC, C5OH, C5DC, C6DC |
Endocrine disorders | 17OHP, TSH |
Galactosemia and biotinidase deficiency | GALT (Galactose-1-Phosphate Uridyltransferase), biotinidase |
The NSO analyte data have been linked securely with the use of unique encoded identifiers to health administrative data at the Institute for Clinical Evaluative Sciences, which captures data on health services use, including hospitalizations, for virtually all Ontario residents. Data on birthweight, GA, ultrasound timing, and other perinatal factors were obtained from the birth admission in the Canadian Institute for Health Information’s (CIHI) Discharge Abstract Database, the Ontario Health Insurance Plan database, and the newborn infant screening record. GA was based on best obstetric estimate, a combination of self-reported first day of last menstrual period and ultrasound measurement, when available. Most mothers in Ontario receive prenatal care, including ultrasound-guided gestational dating. Small for gestational age (SGA10, below 10th percentile for birthweight given gestational age) and large for gestational age (LGA90, above 90th percentile for birthweight given gestational age) were calculated based on standard cutpoints developed in a Canadian population.
Analysis
We divided our cohort of live born infants into 3 subsamples: 1 for model development, 1 to validate independently the choice of terms that were included in the final model, and 1 dataset to assess independently the performance of the final model. These subsamples were generated by randomly partitioning infants according to a 2:1:1 ratio, stratification by term, near term, premature, and extremely premature status and sex to ensure balance across the 3 subsamples.
Data preparation for regression modeling
We removed the data of infants who screened positive for any disorder from the cohort, which had the effect of removing most extreme outliers. Even after extreme outliers were removed, most analyte distributions were strongly right skewed. To pull outliers closer to the rest of the data and stabilize the variance, analyte levels were natural log transformed. We then standardized each analyte value by subtracting the sample mean (on the log scale) and dividing the result by the sample standard deviation (on the log scale), such that the resulting transformed variable had a mean of 0 and a standard deviation of 1. This allowed for easier interpretation when we compared the relative influence of analytes in a multivariable regression model, such that the regression coefficients represented the change in GA in weeks for an increase of 1 standard deviation in the (log) analyte value.
Predictive modeling
We fit a multivariable linear regression model with continuous GA in weeks as the dependent variable and used a variable selection algorithm to select terms for inclusion in the model. The full set of analyte main effects, as well as quadratic and cubic effects, was included in all models to account for a non-linear association between analyte and GA. We then conducted a backwards elimination procedure that initially included all of the main effect terms and all pairwise interactions between analytes. The Schwarz Bayesian Criterion (SBC) was used to guide the sequential removal of interaction terms from the model. SBC is a penalized likelihood criterion that quantifies how well the model fits the data, while penalizing model complexity. Models with smaller SBCs are favored. Once no more interaction terms could be removed from the model based on SBC as evaluated in the model development subsample, the backwards elimination procedure was stopped. We then calculated the square root of the mean square error (RMSE) based on fitting the development models at each step of the backwards elimination in the independent validation set and choosing the model with the lowest RMSE in the validation set. The RMSE reflects how close the model estimate is to the true GA on average across all observations. Finally, the development model performance was evaluated in the test dataset, which had no role in model fitting or validation. This process provided maximum protection from overfitting and over-optimism about model performance.
Evaluation of model performance
The model built with the use of the development and validation datasets was evaluated in the test dataset in terms of adjusted R -square, square root-mean-square error (RMSE), and proportion of infants with predicted GA within ±1, 2, 3, and 4 weeks of true GA. RMSE is in the units of GA and hence represents the average deviation of predicted GA from actual GA over all infants in the test dataset. Model performance was evaluated for all infants, for different levels of prematurity, and for infants who were small for their GA to determine whether the model performed well in babies with low birthweight/intrauterine growth restriction. We defined prematurity in the following manner: term, ≥37 weeks; near term, 33-36 weeks; very preterm, 28-32 weeks, and extremely preterm, <28 weeks. We also evaluated model performance according to history of maternal ultrasound during pregnancy. We categorized infants based on whether the mother received her first ultrasound within 16 weeks, 17-20 weeks, ≥21 weeks and those with no record of their mother receiving an ultrasound during pregnancy according to Ontario Health Insurance Plan claims for diagnostic ultrasound scans that were specific to pregnancy.
Model performance for classification as ≤34 or >34 weeks GA
Thirty-four weeks gestation is an important threshold because it represents the lower limit of late preterm infant period. It is the GA after which the health risks of preterm infants are reduced, while still remaining elevated compared with term infants. To classify infants according to GA ≤34 or >34 weeks, we conducted logistic regression analysis on the test data with actual GA dichotomized as ≤34 vs >34 weeks as the outcome, and the final set of predictors that was chosen for the multiple linear regression model as covariates. The logistic regression model was fit in the model development subset as mentioned earlier, then the c-statistic (area under the receiver operating characteristic curve) as well as sensitivity, specificity, positive predictive value, and proportion of infants who were classified correctly were calculated to quantify the success of the discrimination between the groups with the use of the validation subsample. The test performance was evaluated by adjustment of the GA cutpoint to determine the optimal tradeoff (higher sensitivity comes at the cost of lower specificity and lower positive-predictive value).
All analyses were conducted with SAS software (version 9.4; SAS Institute Inc, Cary, NC) and R (version 3.1.2).
This study was approved by the institutional review board at Sunnybrook Health Sciences Centre, Toronto, Canada, and by the Ottawa Health Science Network Research Ethics Board, and the Institute for Clinical Evaluative Sciences’ Privacy Office.
Results
Characteristics of sample
Data were available for virtually all of the 270,000 live born infants who were delivered in Ontario between April 1, 2007, and March 31, 2009. Complete data for all newborn infant screening study analytes were available for 249,700 infants. The sample characteristics are presented in Table 2 . There were 128,079 male infants (51.3%), 230,067 term infants (92.1%), 21,039 small for GA (SGA10) infants (8.7%), 26,406 large for GA (LGA90) infants (11.0%), and 8494 babies from multiple births. We randomly partitioned the dataset into 50% model development (n = 124,854), 25% validation (n = 62,412), and 25% test (n = 62,434) subsets, while maintaining the proportions of term/near term/very preterm/extremely preterm delivery and sex ratio across subsets.
Variable | N (%) |
---|---|
Sex | |
Male | 128,079 (51.29) |
Female | 121,621 (48.71) |
Prematurity categories | |
Extremely preterm (≤27 wk) | 555 (0.22) |
Very preterm (28-32 wk) | 2,616 (1.05) |
Near term (33-36 wk) | 16,462 (6.59) |
Term (≥37 wk) | 230,067 (92.14) |
Small for gestational age (below 10th percentile) | |
Not small for gestational age | 220,167 (91.28) |
Small for gestational age | 21,039 (8.72) |
Large for gestational age (above 90th percentile) | |
Not large for gestational age | 214,800 (89.05) |
Large for gestational age | 26,406 (10.95) |
Multiple births | |
No | 241,206 (96.60) |
Yes | 8,494 (3.40) |
Overall model performance
Our final model included 43 effects that included birthweight and sex and a total of 311 model terms, which consisted of linear, squared, and cubed main effect terms and pairwise linear interaction terms ( Appendix ). The 10 most predictive analytes (in terms of the change in log-likelihood) were alanine, C5, C16, C18:2, C4DC, C5DC, tyrosine, TSH, leucine and 17OHP.
Table 3 presents model performance overall and in term children (≥37 weeks) and in increasing categories of prematurity. Results are shown for the full model that considered all analytes plus sex and birthweight, for the model excluding birthweight and for a model including sex and birthweight alone.
Model | Adjusted R 2 | Overall (n = 51,161) | Term (≥37 wk; n = 47,317) | Near term (33-36 wk; n = 3295) | Very preterm (28-32 wk; n = 456) | Extremely preterm (≤27 wk; n = 93) | |||||
---|---|---|---|---|---|---|---|---|---|---|---|
Root-mean-square error, wk | Correctly classified ±1/2/3/4 wk, % | Root-mean-square error, wk | Correctly classified ±1/2/3/4 wk, % | Root-mean-square error, wk | Correctly classified ±1/2/3/4 wk, % | Root-mean-square error, wk | Correctly classified ±1/2/3/4 wk, % | Root-mean-square error, wk | Correctly classified ±1/2/3/4 wk, % | ||
Full model | 0.65 | 1.06 | 66.8/94.9/99.3/99.8 | 0.97 | 69.1/96.4/99.8/99.97 | 1.70 | 39.0/75.6/94.8/98.9 | 2.30 | 46.5/76.9/90.4/95.0 | 2.10 | 50.7/77.5/89.4/95.1 |
Without birthweight | 0.56 | 1.24 | 61.2/91.4/98.2/99.5 | 1.02 | 64.4/94.5/99.5/99.9/ | 1.80 | 24.4/56.6/85.7/97.1/ | 2.60 | 25.3/49.2/69.7/83.7/ | 3.60 | 23.2/46.1/61.5/73.6/ |
Sex and birthweight only | 0.54 | 1.26 | 58.2/90.73/98.1/99.5/ | 1.11 | 61.3/94.1/99.6/99.9/ | 2.30 | 21.0/50.1/81.1/99.6/ | 3.00 | 24.0/50.3/50.1/73.3/ | 1.90 | 44.4/78.2/92.3/97.9/ |
Overall, the final model, as evaluated in the test subsample, had an adjusted R -square of 0.67 and a root-mean-square error (RMSE) of 1.06 (meaning the average deviation between observed and expected GA was approximately 1 week), with two-thirds of predicted GAs falling within ±1 week of actual GA ( Table 2 ). In term children, 69% of infant GAs were predicted within ±1 week, and 96% were predicted within ±2 weeks. In near term infants, 39% were predicted within ±1 week, and 76% were predicted within ±2 weeks. In very preterm infants, 51% were predicted within ±1 week, and 77% were predicted within ±2 weeks.
Model performance in subgroups
The overall RMSE in low birthweight infants (SGA10) was 1.34, compared with 1.03 in non-SGA10 infants across all categories of prematurity. However, the increased prediction error was limited to term children (≥37 weeks), because the model performed slightly better in every category of SGA10 infants who were preterm (<37 weeks).
Table 4 provides a breakdown of the estimated category of GA compared with the actual category of GA. GA for term SGA10 infants tended to be underestimated by the model, which resulted in some SGA10 infants (10%) being misclassified as near term. However, <0.1% were misclassified as very preterm, and none were misclassified as extremely preterm ( Table 5 ). Conversely, the model tended to overestimate GA in infants classified as LGA90. For example, >80% of LGA90 near term babies were misclassified as full term.
Actual gestational age, wk | Predicted, % | Total | |||
---|---|---|---|---|---|
≤27 | 28-32 | 33-36 | ≥37 | ||
≤27 | 79.3 | 20.0 | 0.0 | 0.7 | 100 |
28-32 | 8.1 | 66.7 | 21.9 | 3.3 | 100 |
33-36 | 0.0 | 3.6 | 59.7 | 36.7 | 100 |
≥37 | 0.0 | 0.0 | 2.0 | 98.0 | 100 |
Actual gestational age, wk | Predicted, % | Total | |||
---|---|---|---|---|---|
≤27 | 28-32 | 33-36 | ≥37 | ||
≤27 | 100.0 | 0.0 | 0.0 | 0.0 | 100 |
28-32 | 22.7 | 75.0 | 2.3 | 0.0 | 100 |
33-36 | 0.0 | 14.6 | 79.9 | 5.5 | 100 |
≥37 | 0.0 | 0.1 | 10.4 | 89.5 | 100 |
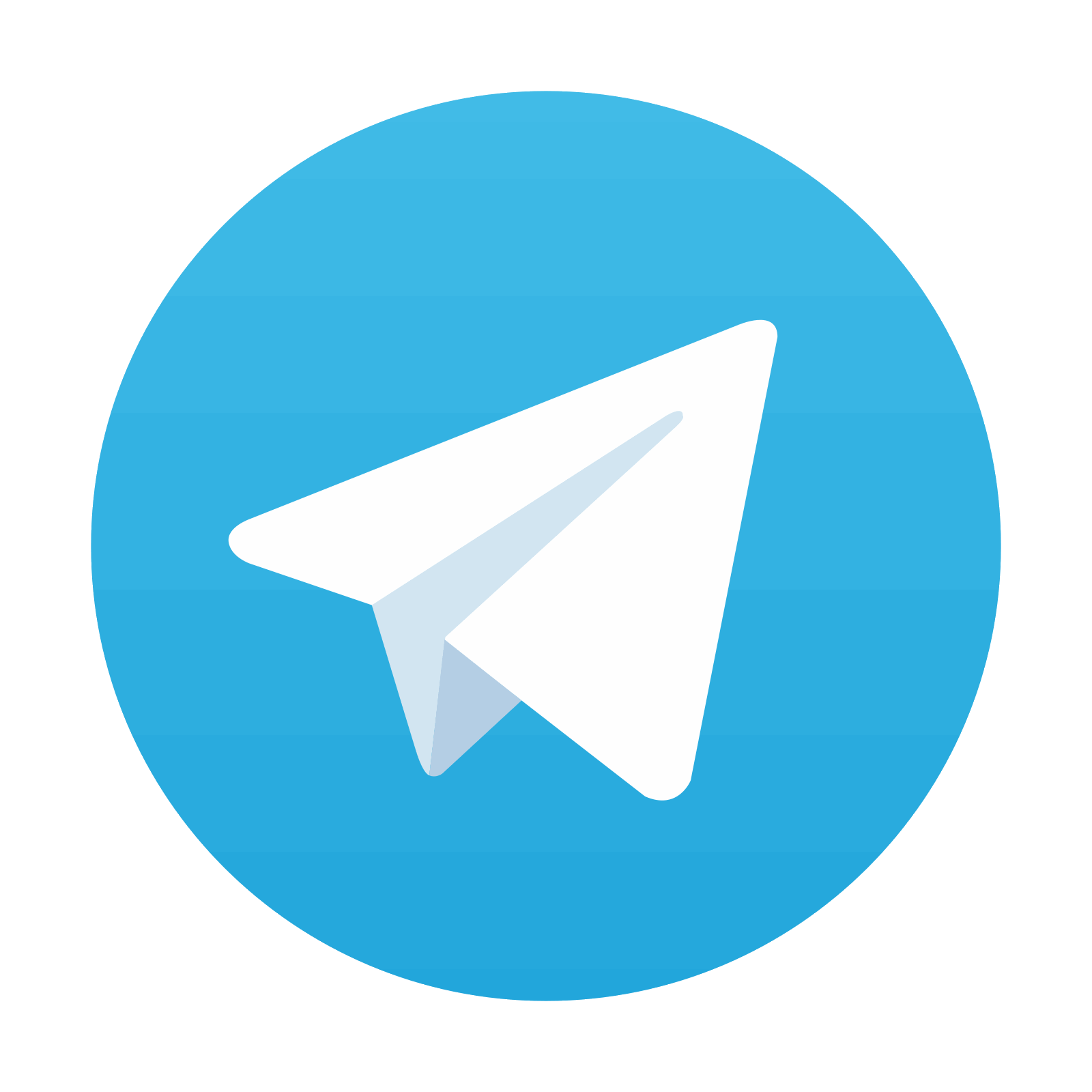
Stay updated, free articles. Join our Telegram channel

Full access? Get Clinical Tree
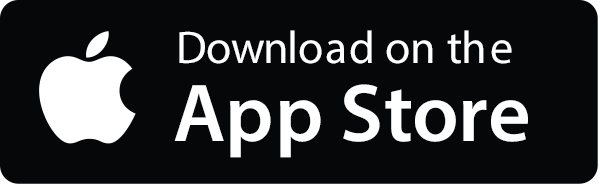
