Objective
We sought to determine whether maternal/fetal single-nucleotide polymorphisms (SNPs) in candidate genes are associated with preterm prelabor rupture of membranes (pPROM).
Study Design
A case-control study was conducted in patients with pPROM (225 mothers and 155 fetuses) and 599 mothers and 628 fetuses with a normal pregnancy; 190 candidate genes and 775 SNPs were studied. Single locus/haplotype association analyses were performed; false discovery rate was used to correct for multiple testing (q* = 0.15).
Results
First, a SNP in tissue inhibitor of metalloproteinase 2 in mothers was significantly associated with pPROM (odds ratio, 2.12; 95% confidence interval, 1.47–3.07; P = .000068), and this association remained significant after correction for multiple comparisons. Second, haplotypes for Alpha 3 type IV collagen isoform precursor in the mother were associated with pPROM (global P = .003). Third, multilocus analysis identified a 3-locus model, which included maternal SNPs in collagen type I alpha 2, defensin alpha 5 gene, and endothelin 1.
Conclusion
DNA variants in a maternal gene involved in extracellular matrix metabolism doubled the risk of pPROM.
Preterm prelabor rupture of membranes (pPROM) complicates approximately 3-4.5% of all pregnancies in the United States and it is responsible for about 30% of preterm births. A genetic predisposition to preterm birth has been suggested based upon: (1) demonstration of familial aggregation; (2) substantiation with segregation studies; (3) identification of disease-susceptibility genes; and (4) racial disparity in rates of pPROM and preterm birth.
Genetic factors are known to predispose to pPROM. First, patients with Ehlers-Danlos syndrome, a rare mendelian connective tissue disorder with mutations either in collagen genes or genes involved in collagen processing, have a substantial genetic predisposition to preterm delivery preceded by spontaneous rupture of the membranes. Although Ehlers-Danlos syndrome is a mendelian disorder and pPROM is not, the shared aspects of the phenotypes are indicative of related and perhaps common etiology, ie, genetic predisposition. Further supporting a genetic role with fetal effect is that pregnant women without Ehlers-Danlos syndrome but with an Ehlers-Danlos syndrome fetus, present with pPROM more than twice as often (50%) than in affected women (20%) with or without an affected fetus. Similarly, patients with Marfan syndrome, a disorder involving mutations of the fibrillin-1 gene leading to abnormalities of collagen structure and hyaluronic acid synthesis, have a 6% rate of pPROM, which is higher than the general population, supporting the view that a genetic factor that predisposes to Marfan plays a role in the risk for pPROM. These 2 syndromes only explain a small fraction of pPROM risk, but their existence demonstrates the principle of a genetic role in pPROM.
Polymorphisms in several genes have been studied in pPROM. Some genes include matrix metalloproteinase (MMP) (MMP1, MMP8, and MMP9) and SERPINH1 (serpin peptidase inhibitor, clade h [heat-shock protein 47]), all of which affect extracellular matrix protein degradation in fetal membranes. Variants in these genes could be associated with membrane weakening and rupture, although a direct functional link has yet to be established. Functional studies have also demonstrated a role for some of the variants in the expression and activity of these molecules involved in extracellular matrix metabolism.
The objective of this genetic association study was to determine if either maternal or fetal carriage of DNA variants predispose to pPROM. A total of 775 single-nucleotide polymorphisms (SNPs) from 190 candidate genes that have been implicated in the mechanisms of disease responsible for spontaneous preterm labor, pPROM, small for gestational age (SGA), and preeclampsia were analyzed. The study was conducted in a Hispanic population at a single site from Chile and with extreme care to phenotypic characterization.
Materials and Methods
Study design
This was a case-control study that included patients with pPROM and their neonates (mothers: 225 and fetuses: 155) who delivered preterm (21-36 weeks of gestation) as well as controls and their neonates (mothers: 599 and fetuses: 628). A patient was considered to have pPROM if she met the following criteria: (1) gestational age <37 weeks; (2) a history of leaking of fluid reported by the mother; (3) sterile speculum examination demonstrating pooling of fluid and a nitrazine test that was positive; (4) a ferning test was considered confirmatory but not necessary for the diagnosis of pPROM; and (5) the term “prelabor” rupture of membranes was used in our manuscript to indicate that the leaking of fluid was required to have occurred at least 1 hour prior to the onset of regular contractions. These criteria have been used by other investigators. The control group included women who delivered a neonate of appropriate weight for gestational age at term (37-42 weeks of gestation) without complications of pregnancy including preterm labor with term delivery, preeclampsia, eclampsia, HELLP syndrome, term PROM, SGA, large-for-gestational age neonate, fetal demise, placental abruption, placenta previa, or chorioamnionitis. Clinical chorioamnionitis was diagnosed according to the criteria proposed by Gibbs et al including maternal temperature of ≥37.8°C and ≥2 of the following criteria: uterine tenderness, malodorous vaginal discharge, maternal leukocytosis (≥15,000 cells/mm 3 ), maternal tachycardia (>100 beats/min), and fetal tachycardia (>160 beats/min).
Patients of Hispanic origin were recruited at the Sotero del Rio Hospital, in Santiago, Chile. All eligible mothers were enrolled in a research protocol, which requested permission to collect DNA from the mother and her neonate for research purposes. The exclusion criteria, besides those explained above for controls, included: (1) known major fetal chromosomal and/or structural anomalies; (2) multiple pregnancy; (3) serious medical illness (eg, chronic renal failure, congestive heart failure, connective tissue disorders); (4) refusal to provide written informed consent; and (5) a clinical emergency, which prevented counseling of the patient about participation in the study, such as fetal distress or maternal hemorrhage. A blood sample was obtained from the mother at the time of enrollment in the protocol, and from the umbilical cord (fetal blood) after delivery. Demographic and clinical characteristics of the mothers were obtained from a data collection form administered by trained medical and paramedical personnel. The collection of samples and their utilization for research purposes was approved by the institutional review boards of the Sotero del Rio Hospital, Santiago, Chile (an affiliate of the Pontificia Catholic University of Santiago, Chile), and the Eunice Kennedy Shriver National Institute of Child Health and Human Development, National Institutes of Health, Department of Health and Human Services.
Genotyping
Candidate genes were selected for analysis based on biological plausibility for a role in pPROM and other pregnancy complications including spontaneous preterm labor with intact membranes, SGA, and preeclampsia. Genes involved in processes such as the control of the immune response (pattern recognition receptors, cytokines, chemokines and their respective receptors), uteroplacental ischemia, or angiogenesis were considered appropriate candidates for this study. A complete list of the 190 genes and all SNPs genotyped are included in the Appendix ( Supplemental Table 1 ).
SNP discovery within the candidate genes was performed by DNA sequencing at Genaissance Pharmaceuticals Inc (New Haven, CT) using its Index Repository that includes a total of 93 subjects with Native American, Hispanic/Latino, European, Asian, and African American ancestry. The protocol for this has been previously described. SNPs selected for genotyping were intended to capture at least 90% of the haplotypic diversity of each gene covering variation in the coding regions, 100 bases at each end of the introns, 1000 bases upstream of the start codon, and 100 bases downstream of the stop codon.
Template DNA for genotyping was obtained by whole-genome amplification of genomic DNA isolated from blood using an automated DNA isolation protocol (BioRobot 9604; Qiagen, Valencia, CA). Genotyping was carried out using the MassARRAY System (Sequenom Inc, San Diego, CA) at the high-throughput genotyping facility at Genaissance. Each genotyping assay involved polymerase chain reaction amplification from template DNA in a target region defined by specific primers for the respective polymorphic sites, purification of the amplicon, annealing of the indicated extension primer to 1 strand of the amplicon adjacent to the polymorphic site, extending the primer by 1 nucleotide using the MassEXTEND reaction (Sequenom Inc), and detection of the allele-specific extension product by mass spectrometry.
Quality control
SNPs were verified for mendelian consistency and genotyping efficiency of both SNPs and samples as described elsewhere. Briefly, we considered the number of mendelian inconsistencies between mother and fetus to identify potential relationship errors (eg, sample mix-ups or mislabeling). In the case of multiple inconsistencies in a given pair, the pair was excluded from further analysis (10 pairs in controls and 5 pairs in cases). Tests for deviations from Hardy-Weinberg equilibrium (HWE) were performed for mothers and fetuses separately and again separately for diagnostic subgroups. Because it is currently unclear how to unequivocally distinguish between deviations from HWE due to genotyping error, and deviations from HWE due to biological causes, such as location at or near a disease susceptibility locus, we noted SNPs that deviate from HWE, but we did not remove them from the analysis. If necessary, we could follow-up these observations with additional testing. Therefore, in the case of deviations from HWE we tagged the SNPs but proceeded with the analyses.
Finally, we tested for population stratification in cases and controls using STRUCTURE, which indicated that case and control Chilean samples both cluster with HapMap European samples (data not shown).
Statistical analysis
Continuous demographic and clinical characteristics of cases and controls (gestational age, birthweight, maternal age, and body mass index [BMI]) were tested for normality using Shapiro-Wilks test. All measurements deviated significantly from normality; therefore, Mann-Whitney 2-sample rank sum tests were used for case-control comparisons. The χ 2 tests were used to test for differences in parity, Apgar scores at 1 and 5 minutes, smoking, and differences in fetal sex between cases and controls. Statistical software (Stata 10.0; StataCorp, College Station, TX) was used for all analyses.
Single locus tests of association
Statistical tests for single locus association and for deviations from HWE were calculated using PLINK software. Statistical significance for deviations from HWE in cases and controls was determined using Fisher’s exact test. Single locus tests of association were performed with logistic regression using an additive genotypic model where the minor allele was coded as the risk allele. Standard summary statistics, odds ratios (ORs), and confidence intervals (CIs) were reported for these tests of association. Prior to performing single locus and haplotype analyses, rare SNPs in our dataset (allele frequency <0.01) were removed (21 SNPs in maternal samples and 44 in fetal samples) as were redundant SNPs (those in strong linkage disequilibrium [LD]). LD-based SNP pruning was performed using PLINK software, with a cutoff of r 2 = 0.8. In all, 52 maternal SNPs and 59 fetal SNPs were removed because they were in LD with other SNPs in the dataset. Of the 775 SNPs that passed quality control, we analyzed 702 maternal and 665 fetal SNPs. We also excluded a small number of X chromosome SNPs (7 total) for fetal data, as neonates included in the study were both male and female, and power is greatly reduced in the male and female samples analyzed separately. These criteria accounted for the difference in the number of SNPs tested in mothers and fetuses.
Multiple testing corrections
A false discovery rate (FDR) correction was performed to adjust for multiple comparisons using a q* of 0.15 in single locus tests of association in maternal and fetal analyses separately. The q* indicates the expected proportion of results that are identified as interesting that are actually false. This is in contrast to α (typically set to 0.05), which indicates the probability of obtaining even 1 false-positive result among all tests for which the null hypothesis is rejected. FDR is used to measure global error, that is, the expected number of false rejections of the null hypothesis among the total number of rejections. The critical significance level was calculated by ranking the results by P values and then multiplying this rank by q* divided by the total number of tests using the step-up approach of Benjamini and Hochberg. The threshold q* = 0.15 is deliberately generous, for the purposes of discovery, in which false acceptance of the null is more problematic than false rejection.
Haplotype tests of association
Haplotype analyses were performed on genes with at least 1 significantly associated SNP ( P < .01) and at least 2 SNPs in the same gene. Haplotype frequencies, as well as haplotype-based association analyses for pPROM with 2- and 3-marker sliding windows, were calculated using PLINK software. Only haplotypes that had a frequency of ≥0.05 were analyzed, and only SNPs that had <5% missing data were used. The strongest associated haplotype windows are reported and those that demonstrated marginal significance with an omnibus test ( P ≤ .05) were analyzed for haplotype-specific effects. We present the calculation of OR for each haplotype (using the most common haplotype as referent), as well as determination of case and control haplotype frequencies. Standard summary statistics for pairwise LD were calculated using Haploview. Haplotype blocks were assigned using the CI algorithm created by Gabriel et al.
Histologic chorioamnionitis analysis
A systematic histologic examination of all placentas available was performed based on diagnostic criteria previously described. All statistically significant single locus and haplotype associations were further analyzed for allele and haplotype differences between patients with pPROM with histologic chorioamnionitis alone or with funisitis (n = 85) and term controls (delivered >37 weeks without histologic chorioamnionitis or funisitis, n = 488). The purpose of these analyses was to further evaluate whether histologic chorioamnionitis was driving the observed associations.
Multilocus analysis
Exploratory multilocus analyses were performed using multifactor dimensionality reduction (MDR) to identify interactions among maternal, fetal, and maternal/fetal SNPs. MDR has been previously described by Ritchie et al and is available as open-source software at www.epistasis.org . Briefly, MDR is a nonparametric (does not assume any statistical model) and model-free (no assumption mode of genetic inheritance) unique tool for identifying gene-gene interactions. MDR collapses all of the genetic data into 2 categories (high and low risk) by comparing all single locus and all multilocus combinations, and then categorizing each genotype into either high risk or low risk on the basis of the ratio of cases to controls that have that genotype. MDR ultimately selects 1 genetic model, either single or multilocus, that most successfully predicts phenotype or disease status. Analyses were performed: (1) separately for maternal and fetal data (tag SNPs only); and (2) combined for available maternal and fetal paired DNA samples. In our case, we analyzed 672 fetal and 702 maternal SNPs for a total of 1374 in the combined analysis. The different number in maternal and fetal samples was due to different quality control results for the 2, and the fact that we did not want to remove possible interactions among genes in mothers and fetuses. Data were analyzed for 2- and 3-way interactions with 10-fold cross-validation and average balanced accuracy as the metrics for evaluating a model. Several filtering steps and parameters were explored and are described on Supplemental Table 2 . The MDR algorithm was implemented with the full array of tag SNPs as well as after filtering, using the Tuned ReliefF (TuRF) approach as described in detail by Moore and White. TuRF is a modification of ReliefF. Briefly, ReliefF is a method that estimates the quality of attributes (eg, SNPs) through a nearest neighbor algorithm that selects neighbors from the same and different classes based on the values of the SNPs (in this case genotypes). TuRF is a modification of ReliefF method that systematically removes SNPs that poorly differentiate cases and controls. The motivation behind this algorithm is that the ReliefF estimates of the true associating SNPs will improve as the nonassociating SNPs are removed from the dataset. In addition, SNPs were filtered based on results of the SNP analyses and only SNPs that had a marginal P value of ≤ .1 were included, or only those with a P value < .05 were analyzed separately. Permutation testing with 1000 permutations was used to determine statistical significance of all MDR models, addressing potential multiple testing issues.
MDR as described above is ideal for a balanced dataset where the number of cases and controls are the same or close to the same. However, computational methods have been developed since the initial development of MDR to test for prediction accuracies in an imbalanced dataset, such as ours. The method, termed “balanced accuracy,” corrects for imbalanced data by taking an average of the sensitivity and specificity and is defined as the arithmetic mean of sensitivity and specificity. We tested for balanced accuracy in this manuscript.
Bioinformatics tools
The SNPper ( http://snpper.chip.org ) database using dbSNP Build 125 was used to determine marker positions (bp), determine marker function, and identify amino acid changes.
Pathway analysis
To examine whether the SNPs found to be putatively associated with pPROM mapped to different biological networks and disease functions, an exploratory analysis was performed using Ingenuity Pathway Analyses (IPA; Ingenuity Systems Inc, Redwood City, CA). The genes with variants that were associated with pPROM ( P < .05) were entered into IPA and were termed “focus genes.” The IPA measured associations of these molecules with other molecules, their network interactions, and biological functions stored in its knowledge base. The knowledge base is scientist curated and encompasses relationships among proteins, genes, cells, tissues, xenobiotics, and diseases. Our focus genes served as seeds for the IPA algorithm, which models functional networks by identifying interconnected molecules, including molecules not among the focus genes from the IPA knowledge base. The software illustrates the networks graphically, and calculates a score for each network, which represents the approximate “fit” between the eligible focus molecules and each network. The network score is based on the hypergeometric distribution and is reported as the –log (Fisher’s exact test result). The IPA software was used to calculate the most significant biological processes associated with each network modeled by IPA. The top functions for a network were ascertained in IPA using the right-tailed Fisher’s exact test for overrepresentation of network molecules in a given process.
Results
Table 1 displays the clinical and demographic characteristics of the study population. Women with pPROM had a lower median gestational age at delivery, a lower birthweight, and different distributions of Apgar scores at 1 and 5 minutes and BMI. These differences were expected (except BMI) by the design of the study. The cases had more male than female newborns. Analyses were adjusted for potential confounders (BMI and fetal sex) in all single locus tests of association.
Variable | Cases (n = 225) a | Controls (n = 599) a | P value | ||
---|---|---|---|---|---|
Median (25th-75th) | Mean (SD) | Median (25th-75th) | Mean (SD) | ||
Parity (no. of previous pregnancies) | 1 [0–2] | 1.39 (1) | 1 [0–1] | 0.91 (1) | < .0001 |
Maternal age, y | 27 [21–34] | 28 (8) | 24 [20–30] | 25 (6) | < .0001 |
BMI | 24 [22–27] | 25 (5) | 24 [22–26] | 24 (4) | .018 |
Smoking | 15% | – | 14% | – | .910 |
Clinical chorioamnionitis | 11% | – | 0% | – | – |
Gestational age at delivery, wk | 32 [28–34] | 31 (4) | 40 [39–41] | 40 (1) | < .0001 |
Birthweight, g | 1730 [1200–2200] | 1676 (642) | 3440 [3230–3650] | 3449 (287) | < .0001 |
Fetal sex male | 61% | – | 51% | – | .015 |
1-min Apgar score | 8 [4–9] | 6 (3) | 9 [9–9] | 8 (1) | < .0001 |
5-min Apgar score | 9 [7–9] | 7 (3) | 9 [9–9] | 9 (0.3) | < .0001 |
a Maternal samples: 225 cases and 599 controls; fetal samples: 155 cases and 628 controls.
Single locus tests of association
Summary information for the SNPs with the most significant associations ( P < .01) with pPROM in maternal and fetal DNA is provided on Table 2 ( Table 3 for unadjusted analyses). There was 1 significant deviation from HWE in maternal controls at prostaglandin E receptor 1, subtype EP1 SNP rs3745459 ( P = 9 × 10 –5 ) that also significantly deviated from HWE in cases ( P = 4 × 10 –5 ). There were also significant deviations from HWE in controls in the corticotropin-releasing hormone receptor-1 gene, SNP rs28364026 (in fetal samples P = 3 × 10 –8 ; in maternal samples P = 4 × 10 –6 ), but not in cases. Therefore, although these 2 SNPs were analyzed and were found to associate with pPROM, these results should be interpreted with caution.
Population | Gene name | Gene code | SNP rs no. | Chromosome | Position (bp) | Function |
---|---|---|---|---|---|---|
Maternal | Tissue inhibitor of metalloproteinase 2 | TIMP2 | rs2277698 | 17 | 74378612 | Coding exon (S101S) |
Angiogenin, ribonuclease, RNase family 5 | ANG | rs11701 | 14 | 20231893 | Intron | |
Toll-like receptor 1 | TLR1 | rs3923647 | 4 | 38475934 | Coding exon (H305L) | |
Nitric oxide synthase 3 (endothelial cell) | NOS3 | rs3730305 | 7 | 1.5E+08 | Intron | |
Alpha 3 type IV collagen isoform 5 precursor | COL4A3 | rs1882435 | 2 | 2.28E+08 | Intron | |
Prostaglandin E receptor 1, subtype EP1 | PTGER1 | rs3745459 | 19 | 14445317 | Coding exon (A272A) | |
Fetal | Chemokine (C-C motif) receptor 2 | CCR2 | rs3749461 | 3 | 46370317 | Promoter |
Matrix metalloproteinase 19 isoform rasi-1 | MMP19 | rs1056784 | 12 | 54519580 | Coding exon (P245S) | |
Corticotropin-releasing hormone receptor-1 | CRHR1 | rs28364026 | 17 | 41268075 | Promoter | |
Collagen, type IV, alpha 3 | COL4A3 | rs1882435 | 2 | 2.28E+08 | Intron | |
CD55 molecule, decay accelerating factor for complement | CD55/DAF | rs10746462 | 1 | 2.06E+08 | Intron | |
Defensin, beta 1 preproprotein | DEFB1 | rs5743418 | 8 | 6722970 | Promoter | |
Lipase C precursor | LIPC | rs6080 | 15 | 56625225 | Intron | |
Insulin-like growth factor 1 receptor | IGF1R | rs3743262 | 15 | 97282996 | Coding exon (T766T) | |
Plasminogen activator, tissue type isoform 3 | PLAT | rs8178750 | 8 | 42164028 | Intron | |
Tumor necrosis factor alpha | TNF | rs1800610 | 6 | 31651806 | Intron | |
Lymphotoxin alpha precursor | LTA | rs1041981 | 6 | 31648763 | Coding exon (T60N) |
Population | Gene code | SNP rs no. | Minor allele | Minor allele frequency | OR | 95% CI | P value | ||
---|---|---|---|---|---|---|---|---|---|
Cases | Controls | Lower | Upper | ||||||
Maternal (cases n = 225; controls n = 599) | REN | rs8192282 | A | 0.13 | 0.08 | 1.68 | 1.18 | 2.39 | .004 |
COL4A3 | rs1882435 | A | 0.33 | 0.26 | 1.4 | 1.1 | 1.78 | .006 | |
TLR1 | rs3923647 | T | 0.06 | 0.02 | 2.59 | 1.47 | 4.58 | .001 | |
CSPG2 | rs2287926 | A | 0.14 | 0.09 | 1.57 | 1.12 | 2.22 | .009 | |
RNASE4 | rs11701 | G | 0.13 | 0.18 | 0.64 | 0.47 | 0.89 | .008 | |
IGF1R | rs3743262 | T | 0.20 | 0.14 | 1.58 | 1.18 | 2.12 | .002 | |
TIMP2 | rs2277698 | A | 0.13 | 0.07 | 1.88 | 1.32 | 2.69 | .0005 | |
Fetal (cases n = 155; controls n = 628) | F5 | rs6019 | C | 0.07 | 0.04 | 1.99 | 1.18 | 3.33 | .0096 |
CD55 | rs10746462 | A | 0.31 | 0.24 | 1.5 | 1.13 | 1.99 | .005 | |
COL4A3 | rs1882435 | A | 0.35 | 0.26 | 1.51 | 1.16 | 1.97 | .002 | |
CCR2 | rs3749461 | G | 0.07 | 0.03 | 2.24 | 1.26 | 3.97 | .006 | |
FGF1 | rs34003 | G | 0.30 | 0.40 | 0.67 | 0.51 | 0.88 | .004 | |
IL18BP | rs5743658 | C | 0.05 | 0.02 | 2.59 | 1.32 | 5.09 | .006 | |
MMP19 | rs1056784 | T | 0.02 | 0.00 | 7.16 | 2.07 | 24.79 | .002 | |
IMP5 | rs28364026 1 | A | 0.08 | 0.15 | 0.57 | 0.38 | 0.85 | .007 |
The most significant association in maternal DNA, after adjusting for fetal sex and BMI, was at a synonymous coding SNP (S101S) in tissue inhibitor of metalloproteinase 2 ( TIMP2 ) rs2277698 (OR, 2.12; 95% CI, 1.47–3.07; P = 6.8 × 10 –6 ) ( Table 4 ). The minor allele frequency of this SNP (A) was 0.13 in cases and 0.07 in controls. The most significant association observed in fetal DNA was in a SNP in the chemokine (C-C motif) receptor 2 ( CCR2 ) promoter region, rs3749461 (OR, 2.62; 95% CI, 1.44–4.75; P = .002). The minor allele frequency for this SNP (G) was 0.07 in cases and 0.03 in controls. Only the association with maternal SNP rs2277698 remained statistically significant after correction for multiple testing using FDR.
Population | Gene code | SNP rs no. | Minor allele | Minor allele frequency | OR | 95% CI | P value | ||
---|---|---|---|---|---|---|---|---|---|
Cases | Controls | Lower | Upper | ||||||
Maternal (cases n = 225; controls n = 599) | TIMP2 | rs2277698 | A | 0.13 | 0.07 | 2.12 | 1.47 | 3.07 | .000068 a |
ANG | rs11701 | G | 0.13 | 0.18 | 0.58 | 0.41 | 0.83 | .003 | |
TLR1 | rs3923647 | T | 0.06 | 0.02 | 2.40 | 1.30 | 4.43 | .005 | |
NOS3 | rs3730305 | A | 0.07 | 0.04 | 1.91 | 1.21 | 3.01 | .005 | |
COL4A3 | rs1882435 | A | 0.33 | 0.26 | 1.42 | 1.10 | 1.83 | .007 | |
PTGER1 | rs3745459 b | T | 0.05 | 0.02 | 2.00 | 1.20 | 3.34 | .008 | |
Fetal (cases n = 155; controls n = 628) | CCR2 | rs3749461 | G | 0.07 | 0.03 | 2.62 | 1.44 | 4.75 | .002 |
MMP19 | rs1056784 | T | 0.02 | 0.00 | 6.81 | 1.95 | 23.83 | .003 | |
CRHR1 | rs28364026 b | A | 0.08 | 0.15 | 0.52 | 0.33 | 0.80 | .003 | |
COL4A3 | rs1882435 | A | 0.35 | 0.26 | 1.51 | 1.14 | 1.99 | .004 | |
CD55/DAF | rs10746462 | A | 0.31 | 0.24 | 1.52 | 1.13 | 2.04 | .006 | |
DEFB1 | rs5743418 | T | 0.04 | 0.02 | 2.84 | 1.33 | 6.07 | .007 | |
LIPC | rs6080 | A | 0.04 | 0.02 | 2.74 | 1.31 | 5.73 | .007 | |
IGF1R | rs3743262 | T | 0.20 | 0.14 | 1.62 | 1.14 | 2.30 | .008 | |
PLAT | rs8178750 | T | 0.05 | 0.09 | 0.42 | 0.22 | 0.80 | .008 | |
TNF | rs1800610 | A | 0.25 | 0.32 | 0.67 | 0.50 | 0.91 | .009 | |
LTA | rs1041981 | A | 0.34 | 0.27 | 1.47 | 1.10 | 1.97 | .0097 |
a Significant after false discovery rate correction;
b These single-nucleotide polymorphisms are deviated from Hardy-Weinberg equilibrium.
Additional SNPs that were associated with pPROM at a P value < .05 are presented in Tables 5 and 6 . Although we have not emphasized these findings in the present report, in some instances they represent associations in genes reported that may lend support to previous findings or may be additional SNPs in genes reported with a P value < .01. Such findings strengthen the likelihood of an association because it will be based on multiple SNPs for the same gene (eg, NOS3 in mothers, collagen genes in mothers, and MMP19 in the fetus).
Gene | SNP rs no. | A1 | OR | 95% CI | P value | |
---|---|---|---|---|---|---|
Lower | Upper | |||||
TIMP2 | rs2277698 | A | 2.12 | 1.47 | 3.07 | .000068 |
ANG | rs11701 | G | 0.58 | 0.41 | 0.83 | .003 |
TLR1 | rs3923647 | T | 2.40 | 1.30 | 4.43 | .005 |
NOS3 | rs3730305 | A | 1.91 | 1.21 | 3.01 | .005 |
COL4A3 | rs1882435 | A | 1.42 | 1.10 | 1.83 | .007 |
PTGER1 | rs3745459 | T | 2.00 | 1.20 | 3.34 | .008 |
CSF1 | rs1058885 | C | 1.43 | 1.09 | 1.87 | .011 |
IL6R | rs8192282 | A | 1.64 | 1.12 | 2.39 | .011 |
VWF | rs1800377 | A | 0.42 | 0.21 | 0.83 | .012 |
COL4A4 | rs12475686 | T | 1.36 | 1.07 | 1.74 | .013 |
IGF1 | rs5742620 | A | 2.36 | 1.19 | 4.67 | .014 |
REN | rs3730103 | G | 1.87 | 1.13 | 3.10 | .015 |
CSF1 | rs333970 | C | 1.34 | 1.05 | 1.72 | .019 |
ACE | rs4311 | T | 1.34 | 1.05 | 1.72 | .019 |
MMP10 | rs486055 | A | 1.84 | 1.11 | 3.05 | .019 |
LPL | rs270 | A | 0.65 | 0.45 | 0.93 | .019 |
IL1A | rs3783550 | C | 1.32 | 1.05 | 1.68 | .019 |
TNFRSF1B | rs5746051 | G | 0.66 | 0.47 | 0.94 | .020 |
IGF1R | rs3743262 | T | 1.45 | 1.06 | 1.98 | .021 |
NOS2A | rs2779248 | G | 1.36 | 1.05 | 1.77 | .022 |
CSPG2 | rs2287926 | A | 1.52 | 1.06 | 2.18 | .023 |
COL1A1 | rs17639446 | G | 1.67 | 1.07 | 2.59 | .023 |
TNR | rs1385540 | T | 1.37 | 1.02 | 1.83 | .035 |
F3 | rs610277 | C | 2.15 | 1.06 | 4.38 | .035 |
IL1A | rs17561 | T | 0.74 | 0.55 | 0.98 | .036 |
ACE | rs4354 | T | 0.28 | 0.08 | 0.93 | .038 |
TBXAS1 | GNSC_53711588 | T | 3.07 | 1.05 | 9.01 | .041 |
MMP1 | rs470132 | T | 1.39 | 1.01 | 1.90 | .041 |
IFNGR2 | rs9808753 | G | 1.41 | 1.01 | 1.97 | .042 |
PLAT | rs8178750 | T | 0.63 | 0.40 | 0.98 | .042 |
MMP10 | rs17860949 | T | 1.47 | 1.01 | 2.13 | .042 |
FGF4 | rs3740640 | G | 1.64 | 1.02 | 2.63 | .043 |
LIPC | GNSC_16324977 | A | 1.89 | 1.01 | 3.56 | .047 |
NOS3 | rs1800782 | T | 1.66 | 1.01 | 2.76 | .048 |
Gene | SNP rs no. | A1 | OR | 95% CI | P value | |
---|---|---|---|---|---|---|
Lower | Upper | |||||
CCR2 | rs46370317 | G | 2.62 | 1.44 | 4.75 | .002 |
MMP19 | rs54519580 | T | 6.81 | 1.95 | 23.83 | .003 |
CRHR1 | rs41268075 | A | 0.52 | 0.33 | 0.80 | .003 |
COL4A3 | rs227810996 | A | 1.51 | 1.14 | 1.99 | .004 |
DAF | rs205577171 | A | 1.52 | 1.13 | 2.04 | .006 |
DEFB1 | rs6722970 | T | 2.84 | 1.33 | 6.07 | .007 |
LIPC | rs56625225 | A | 2.74 | 1.31 | 5.73 | .007 |
IGF1R | rs97282996 | T | 1.62 | 1.14 | 2.30 | .008 |
PLAT | rs42164028 | T | 0.42 | 0.22 | 0.80 | .008 |
TNF | rs31651806 | A | 0.67 | 0.50 | 0.91 | .009 |
LTA | rs31648763 | A | 1.47 | 1.10 | 1.97 | .010 |
PROS1 | rs95129086 | A | 1.45 | 1.09 | 1.92 | .011 |
SERPINE1 | rs100567623 | T | 4.52 | 1.40 | 14.60 | .012 |
GNB3 | rs6820171 | A | 2.01 | 1.17 | 3.45 | .012 |
COL4A1 | rs109659786 | G | 1.60 | 1.11 | 2.31 | .012 |
PLAUR | rs48851659 | G | 2.08 | 1.17 | 3.69 | .013 |
COL4A4 | rs227681867 | T | 1.42 | 1.08 | 1.86 | .013 |
FGF1 | rs141955251 | G | 0.72 | 0.54 | 0.95 | .019 |
IGF2R | rs160443699 | T | 0.41 | 0.19 | 0.86 | .019 |
IL5RA | rs3093142 | A | 1.53 | 1.07 | 2.19 | .020 |
COL5A2 | rs189683203 | C | 1.42 | 1.06 | 1.92 | .021 |
TLR2 | rs154844859 | C | 1.88 | 1.09 | 3.25 | .024 |
IL18BP | rs71387372 | C | 2.22 | 1.08 | 4.59 | .031 |
MMP16 | rs89409417 | A | 1.52 | 1.04 | 2.24 | .032 |
MMP19 | rs54521518 | T | 0.44 | 0.20 | 0.94 | .034 |
CSF1 | rs110267989 | C | 1.39 | 1.02 | 1.88 | .036 |
PTGS1 | rs124173328 | T | 0.29 | 0.09 | 0.94 | .039 |
IL12RB1 | rs18031384 | T | 1.43 | 1.02 | 2.02 | .041 |
APOC3 | rs116206884 | A | 1.35 | 1.01 | 1.80 | .043 |
IGF1 | rs101398994 | C | 0.59 | 0.35 | 0.98 | .043 |
AGT | rs228912600 | T | 0.65 | 0.42 | 0.99 | .045 |
FLT1 | rs27910114 | A | 0.73 | 0.53 | 0.99 | .046 |
FN1 | rs215951895 | C | 0.75 | 0.56 | 0.99 | .046 |
F5 | rs167808137 | C | 1.74 | 1.01 | 3.02 | .048 |
Haplotype tests of association
Haplotype analyses of genes with at least 1 significant SNP ( P < .05) documented a haplotypic association between maternal Alpha 3 type IV collagen isoform precursor gene and pPROM ( Table 7 ). The haplotype included markers rs1882435-rs10178458-GNSC_634673878 (global P = .003). This haplotype had rs1882435, a SNP that was associated with the risk of pPROM ( Table 4 ) ( P = .007) where the (A) allele is the risk allele. Upon examining the individual haplotypes, it was clear that all statistically significant haplotypes contained the rs1882435 risk allele, although the effect size is greater and the P value is much less for the haplotype than for the SNP results. Examination of the LD plot for COL4A3 ( Figure 1 ) demonstrated that these 3 markers were in overall weak LD (r 2 ≤ 0.03), further supporting a true haplotype effect.
Population | Gene code | SNP rs no. | Haplotype | Frequency | OR | 95% CI | P value | ||
---|---|---|---|---|---|---|---|---|---|
Cases | Controls | Lower | Upper | ||||||
Maternal | COL4A3 | rs1882435-rs10178458-GNSC_634673878 | Global p | .003 | |||||
CCT (Referent) | 0.59 | 0.66 | |||||||
ACT | 0.32 | 0.23 | 1.55 | 1.20 | 1.99 | .0004 | |||
CTT | 0.09 | 0.11 | 0.92 | 0.62 | 1.36 | .680 |

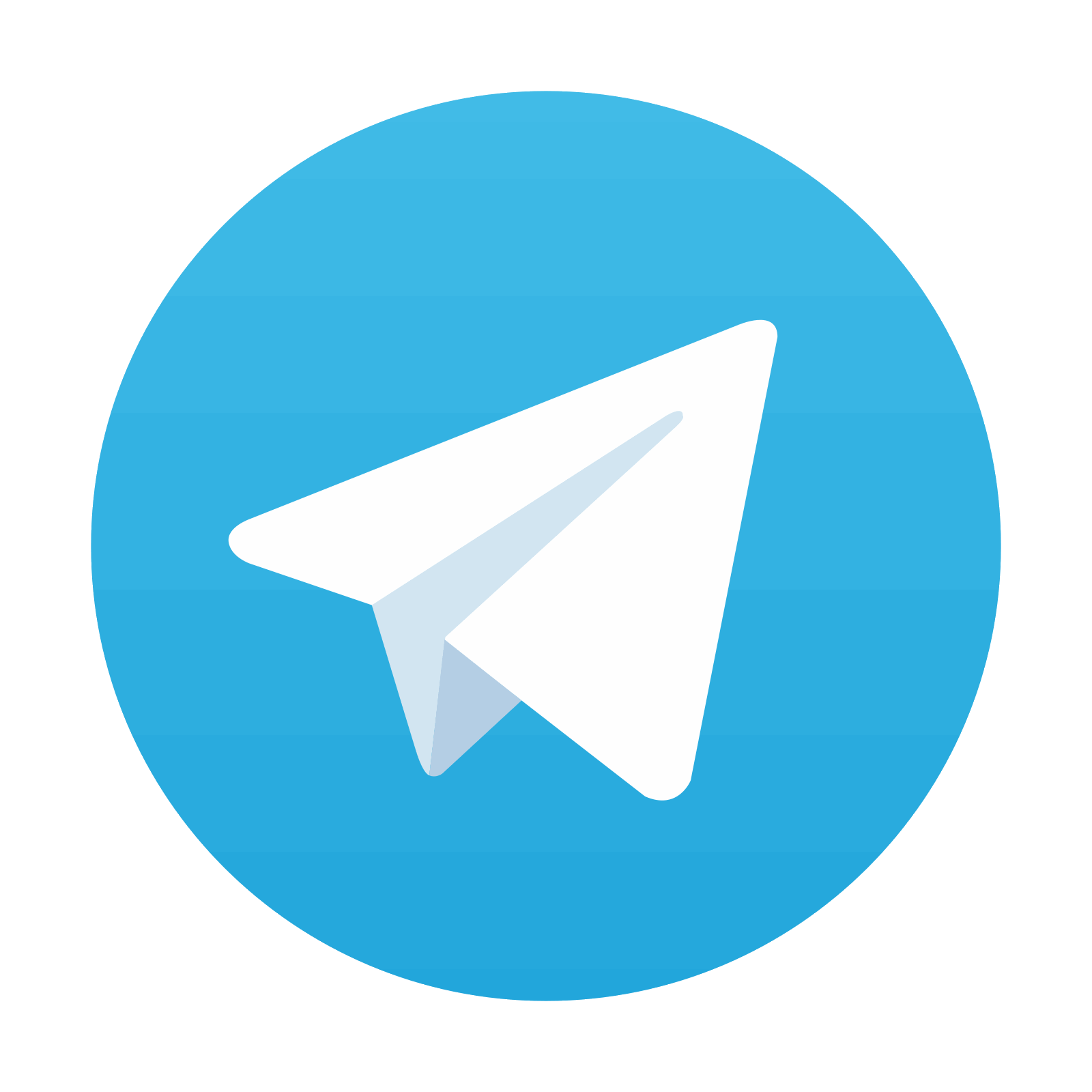
Stay updated, free articles. Join our Telegram channel

Full access? Get Clinical Tree
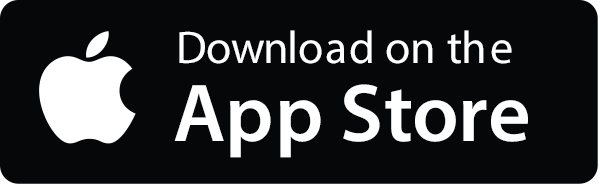

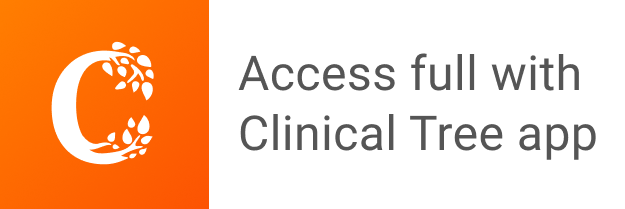