© Springer International Publishing AG 2017
Christina A. Di Bartolo and Maureen K. BraunPediatrician’s Guide to Discussing Research with Patients10.1007/978-3-319-49547-7_33. Study Design
(1)
The Child Study Center, NYU Langone Medical Center, New York, New York, USA
(2)
Department of Pediatrics, The Mount Sinai Hospital, New York, New York, USA
Keywords
Inferential statisticsStudy rigorMethodologyRandomizationLong-term outcomesStudy Rigor
By this point, it should be clear which kinds of studies warrant an in-depth look from pediatricians and patients. First, an obvious and meaningful conflict of interest (whether disclosed outright or inferred based on funding streams) should be absent. Second, patients have performed their own due diligence: applying guiding principles, ascertaining the legitimacy of the Web site presenting the study, or reviewing the overview of the study on ClinicalTrials.gov. Physicians and patients must next determine if the study’s design is rigorous enough to incorporate its conclusions into the shared decision-making process.
Broadly, rigor is an assessment of the methodologies used in a particular study. We touched on rigor when discussing the experiment examining how conflict of interest disclosures affect doctors’ perceptions of the quality of the study [1]. The quality of a study can be defined in many ways, but rigor is a key determinant. Not all studies can or even should follow the same design.
To Explain to a Patient
Think of research studies like custom-made suits, and methodologies like the information the tailor uses to craft the suit. Each suit needs certain measurements, like sleeve length and shoulder width. They can also be customized according to the wearer’s needs or preferences (extra pockets, double-breasted, single vent or two, etc.). While each one is a suit, they will not all look the same. In fact, one of the defining characteristics of a custom-made suit is that it is expected to vary from wearer to wearer. However, a suit should still fit the wearer. A poorly designed research study is like a custom-made suit that fits poorly. It is still a suit, but would you want to buy it? Examining the rigor of a study is like checking to see if the custom-made suit fits.
More precisely, rigor represents how easy or challenging it is for a study to report significant and meaningful results. The researchers choose methodologies that directly influence the study’s rigor.
To Explain to a Patient
Think of methodological rigor as hurdles of various heights, and a significant result as a jumper who makes it over a hurdle. We award more points to a jumper who clears a high hurdle. We should place more consideration in significant results when they come from a study with a highly rigorous design. A hurdler who clears a high hurdle can obviously clear a lower one as well. While a jumper who clears a low hurdle is still technically successful, we award fewer points to that jumper. We have limited information about the overall skill of that hurdler. We don’t know if he would have cleared a higher one. We have less confidence in that jumper. On the other hand, a jumper who knocks a hurdle of any height over represents a nonsignificant result. It doesn’t matter how high the hurdle was; not clearing it earns the jumper no points anyway.
Study rigor ranges from none at all to the highest level the scientific process currently has available. The lowest level of rigor would be equivalent to no study. Patients, for example, often present to their pediatricians anecdotal statements such as the following: “My friend started giving her son linseed oil for teething; do you think I should do that?” This is an example of the lowest level of rigor. Anecdotal evidence does not constitute a study, it therefore has no rigor.
At this stage in science, the highest level of rigor at researchers’ disposal is the randomized controlled trial (RCT). If researchers have RCTs—the highest hurdle that will award their jumper the maximum number of points should it be cleared—at their disposal, patients often implicitly wonder why they do not employ it every time. It appears neglectful to choose a less rigorous methodology when RCTs exist. This is essentially the question patients ask when they assert that until an RCT is conducted disproving their personal viewpoints; they will continue to believe in an unproven treatment or scientific hypothesis.
The answer lies in that RCTs are not always available: they can be impractical, impossible, unlikely to be implemented with proper fidelity, or unethical. Again, research methodologies are not a one-size-fits-all scenario. Later in this chapter, we will review in detail how RCTs can be unavailable to researchers who would otherwise want to perform their study with the highest rigor level.
Given that RCTs are not always possible, patients are left to determine the level of rigor of studies they encounter. We will outline the various signposts patients can use to approximate the level of rigor. The signposts stem from the mathematical tools underlying the scientific method. Patients who would like to understand the specific details can access resources written for a general audience, such as Naked Statistics [2]. Here, we will discuss the signposts and rationale for their importance.
Inferential Statistics
In statistics, a population refers to the set of individuals implicated in a phenomenon. For example, the typical population in cancer studies is patients with cancer. Some phenomena affect a large number of individuals, while others implicate far fewer people. Consider how many more people have cancer than, for example, the number of people with a rare genetic disorder. Many researchers are interested in wide-scale phenomena, such as children with ear infections, mothers who breast-fed, or fathers who were over forty when their first child was born. Because researchers cannot observe everyone in those populations, they use inferential statistics to learn more about the phenomena. In inferential statistics, researchers must first select individuals that represent the entire population. This selection is called the sample. One foundation of inferential statistics is that a properly drawn sample will represent its population well. After selecting participants to comprise their sample, researchers observe them. Once sufficient observations have been collected, the researchers use statistical methods to infer conclusions about the population based on the sample’s data.
Inferential statistics derive from the assumption that two samples (or two groups) will not differ from each other if they come from the same population. Statistics provides the likelihood that any observed difference between the two groups is due to chance. A difference attributed to chance is the null hypothesis, which we discussed in Chap. 2. The alternative option is the likelihood that the difference is a result of the two groups coming from different populations. This is called the experimental hypothesis. The careful reader will notice that statistics cannot definitively state whether or not a difference is due to chance or a difference in population.
Individuals within a sample will not be identical. There will be some amount of variability which can be measured and factored into analyses. Because individuals within a sample differ, statistics provides simplifying metrics to describe the sample as a whole. This simplifier permits researchers to compare one group to another, despite the individuals’ variability within each group. These simplifying metrics are called “measures of central tendency,” and they serve to reduce data from multiple individuals from a sample into one number. The measure of central tendency patients are most familiar with is the mean, or average.
Statistics assumes that truly different populations will have means that differ from one another. The further apart the means of two groups, the less likely the difference is due to chance. Put another way, the farther apart the means of two samples are, the less likely the null hypothesis is to be true. Therefore, large differences between the means represent an increased likelihood that the difference is due to an underlying difference in the populations that the samples are representing.
Combining these two foundations together, researchers can infer that two samples come from different populations without being able to infer one step further: to infer from one individual’s information to which population they belong. Statistics can only tell us the likelihood that an observed difference is due to chance. While this is a crucial limitation, statistics is still the most powerful and valuable tool available in the field of research. Yet because inferential statistics have serious limitations, physicians need to be clear about these limits with their patients.
To Explain to a Patient
Statistics is powerful, but limited. Statistics can tell us that on average, adult males are taller than adult females. This means the average height of a group of males is very likely to be taller than the average height of a group of females. But that doesn’t mean that all groups of men will be taller than all groups of women. Sometimes men are short and women are tall. If you sample enough groups of men and women, over and over again, eventually, by chance alone, you’ll find one group of men who is, on average, shorter than your group of women. This is because height varies among individual women and individual men. If we know the height of a person is 5 ft, 7 in., statistics cannot definitively tell whether that person is a man or woman.
Acceptable Uncertainty
When determining whether or not an observed difference between two samples is likely due to an underlying difference of populations, statisticians must decide what the word likely stands for. Perceptions of likelihood change depending on the circumstances. An individual packing for a trip to Kansas or Seattle might consider the likelihood of rain when choosing whether or not to bring an umbrella. There may reasonably be a lower threshold for likeliness when packing for the Seattle trip, given how notoriously wet the Pacific Northwest is. The traveler might accept only 0% chance of rain in the forecast as the umbrella threshold for Seattle, whereas at least 50% chance of rain in the Kansas forecast would be warranted to pack the umbrella.
It would be terribly confusing if each researcher used his or her own threshold for likelihood. Accordingly, statisticians commonly use one agreed-upon threshold as their definition of likelihood. This threshold is that if an observed difference could be due to chance (and not an actual difference in the populations) 5 times out of 100 or less, researchers typically report they found a “significant” result. The probability of 5 times out of 100 is commonly reduced to decimal format: .05. Any observed difference that could be due to chance only .05 or less is called a “significant” result. Because the scientific community has agreed upon this threshold, a result below .05 allows researchers to reject the null hypothesis and proclaim a difference between the two groups as likely to be due to a difference in underlying populations.
The word significant in this case is defined very precisely. Its meaning is limited to the likelihood that the observed difference is due to chance less than .05. In research parlance, significance is not synonymous with importance, or even clinical relevance. We devote the next chapter to this foundational distinction.
The statistical measure of significance is called the p-value. The p-value is akin to the weather forecast. Saying the researchers “used a p-value of 0.05” mirrors the traveler deciding “I will only pack my umbrella if the forecast says at least 50% chance of rain.” The traveler’s significance threshold, 0.5, is relatively low. The commonly used statistical threshold, a p-value of 0.05, is sufficiently difficult to overcome. As far as hurdles go, it’s fairly high. A p-value of, for example, 0.01, is even harder to overcome. A p-value of 0.01 represents a likelihood of 1 in a 100 that the observed difference is due to chance. The researcher would have decreased even further the likelihood that the observed difference was due to chance, placing more confidence in a result that surpassed the threshold. The significance threshold could of course be set even more strictly for more assurance that an observed difference is truly part of an underlying difference of populations.
Yet researchers do not regularly set the threshold at 0.01. This was a decision born of a desire to avoid the occurrence of false negatives. In statistics terminology, a false negative is called a Type II error. What most researchers aim for—finding a true difference between groups to reject the null hypothesis—is the target. The threshold for the p-value is the size of the bull’s eye. The smaller the threshold p-value, the smaller and smaller the area of the bull’s eye shrinks. Accordingly, it becomes harder and harder for the data to show a difference between two groups that meets this strict criteria. With strict criteria (such a p-value of 0.01), the torturously small bull’s eye could show a “miss,” even when the data truly do represent a true underlying population difference. The drawbacks, or even dangers, of setting the p-value threshold too low are all associated with situations when missing phenomena that could be there would be detrimental.
In some circumstances, misses are the least-preferred outcome. If a child remains nonverbal at age 3, there is a chance that the child will still develop speech in future without any additional intervention. There is also a chance the child has some specific problem that lies outside the population norms of typical development. If there is a problem, finding it via evaluation represents a “hit.” Failing to observe a problem where there is one would be a “miss.” Physicians must decide whether to set the threshold high and require that a child age further before intervening with evaluations and services, or set a low threshold and evaluate right away.
Most parents would agree to a lower testing threshold. They do not tolerate much chance their child might have a true difficulty that they miss. This miss would be more likely to occur when the threshold for significance is too strict. Setting the threshold is more than a statistical exercise. It represents the tradeoff between the confidence someone can place in a “hit” result, versus their concerns that they not “miss” something that may be there and require attention.
A “miss” is an error reporting nothing is there when something is. But errors can report the opposite: that something is there when nothing is. Statisticians call these kinds false positives Type I errors. A Type I error occurs when the bull’s eye is too large. A large p-value (say, accepting that an observed difference is due to chance 50 in 100 times, or 0.5) is the equivalent of a large bull’s eye. The data can score a point for “significance” due to the large bull’s eye even when there is no true difference in the two groups. We have seen that setting the p-value threshold too low is problematic when the risk of missing is intolerable. There are also situations when the risk of false positives takes precedence.
False positive results lead to over-intervention for the many in service of catching a problem for the few. Situations where the burden of over-intervention of the many is unacceptable require setting the p-value threshold sufficiently low. As with misses, what burden is too large is not a challenge for statisticians; rather, it is a challenge for the people who use statistics to inform their decision-making.
An example of well-intentioned people deciding what amount of false positives to accept is the recent revision of the American Cancer Society’s recommendations for women’s breast cancer screenings. The previous rationale held that more screenings were better, following a “better safe than sorry” approach. The many false positives (Type I errors) seemed preferable than a few misses (Type II errors). Oncologists deliberated over the harm of few more painful mammogram procedures, a few more weeks of worry while more precise results come back. What they failed to adequately grasp was that this “few more” was multiplied by the millions of women across the country. Over the years, the answer to this perplexing question took shape. Women with benign tumors were undergoing painful, health-damaging, and costly procedures for tumors that otherwise would not have an impact on their lives. The stress of women being asked back for more scans, the lost productivity as they took time off of work and other duties for these visits, and the anxious waiting for results are all now recognized as an unfair burden to place on otherwise healthy women in the interest of a very few who might benefit from such aggressive screening. Once the data painted a clearer picture of the tradeoff between testing and not testing, the American Cancer Society revised its recommendations for screening to reduce these Type I errors [3].
Sample Size
While specific numbers vary depending on what statistics are applied to the samples, a general rule of thumb is that even the most basic inferential statistical test requires at least 30 participants per group to provide adequate confidence in the results. Inferential statistics are generally not required when studying extremely rare phenomena. In those cases, the researcher would simply observe those entities and describe the phenomenon based on the observations.
On an intuitive level, it follows that the larger the sample studied of a given population, the more faith we can place in the conclusions drawn from that sample. As the sample size gets larger, it gradually approaches the total number of individuals in the population. Some populations are bigger than others, so studies of smaller populations may employ smaller sample sizes and still be considered adequately rigorous. Technically, the aspect of the sample size patients should care most about is how close it is to the population total, so as to not discount studies with small populations. But the shorthand becomes: the larger the sample size, the better. Many studies reported in the popular media, if one digs deeper, studied only a handful of people. For example, in addition to the outright fraud involved in Andrew Wakefield’s autism research, a basic design flaw is that he studied only 12 children [4].
While large sample sizes are generally preferable, they do not automatically convey more confidence in a study’s results. Large sample sizes increase the likelihood that researchers reject the null hypothesis. Increasing the sample size is another way of making the “bull’s eye” larger, and the result is a higher chance of Type I errors, or false positives. The next chapter will cover this in close detail.
Variables and Level of Control
The next methodological choice in a study is the level of control. Control refers to the researcher’s ability to either direct or account for variability among their sample. In theory, the scientific method is designed to test the impact of one variable on another variable. The first variable, the one that researchers are interested in examining the effects of, is called the independent variable. The second variable, the one that researchers then observe the first variable’s effects on, is called the dependent variable.
To Explain to a Patient
Think back to your fourth grade science class—maybe you conducted an experiment on bean plants with sunlight. Your teacher asked you to put one plant in the window under direct sunlight, another plant elsewhere in the classroom to receive diffuse light, and the third in the supply closet, which was dark. Even small children can exert control in this study. Children assigned the plants to various levels of light, the independent variable. After 1 week, your fourth grade teacher asked you and your classmates to measure the height of the bean plants. The height is the dependent variable, because children cannot directly influence the height of a plant the way they can directly influence the sunlight it receives. The variable is dependent on other actions for its outcome.
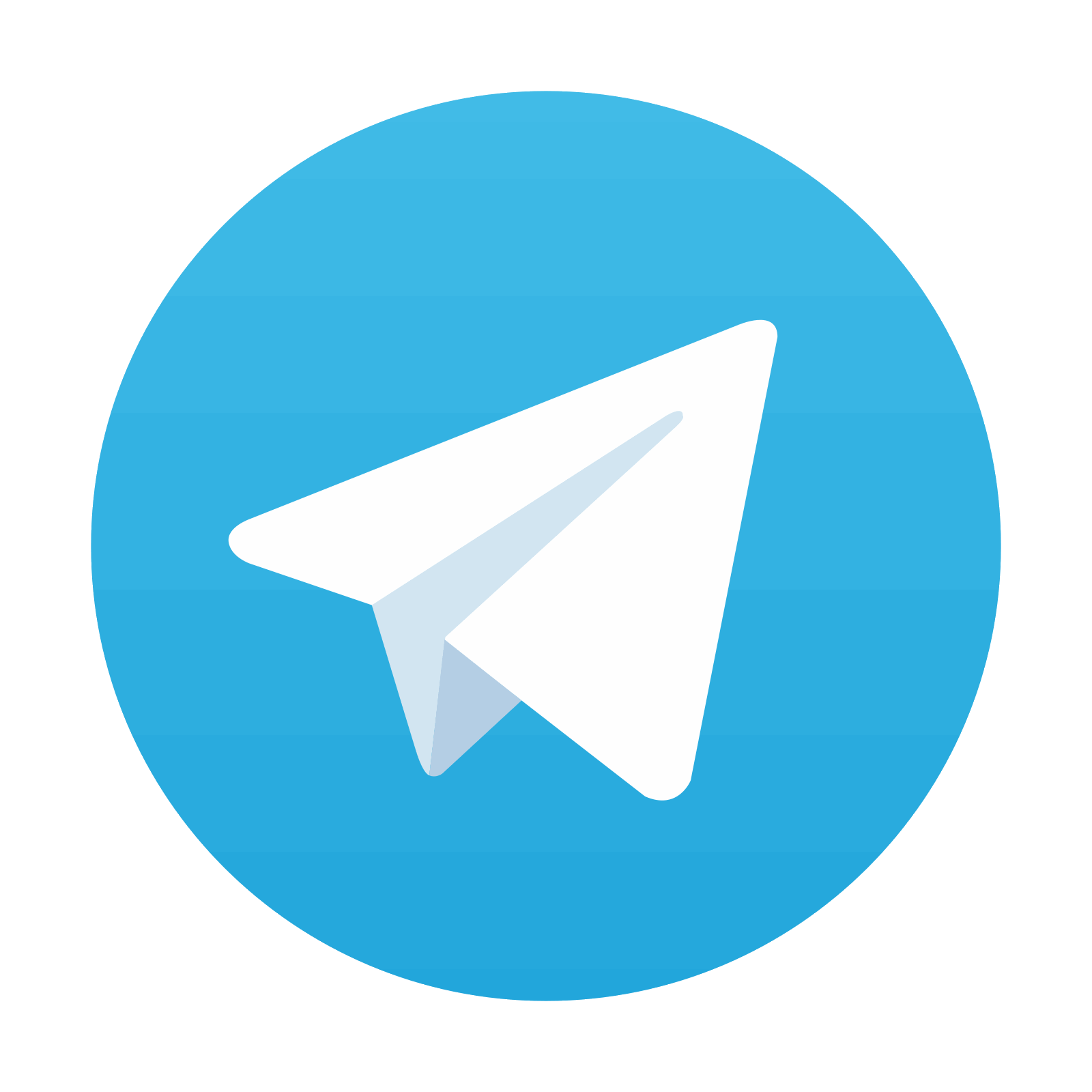
Stay updated, free articles. Join our Telegram channel

Full access? Get Clinical Tree
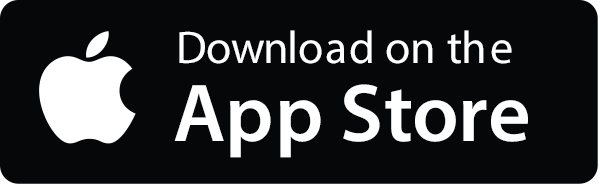
