© Springer International Publishing AG 2017
Christina A. Di Bartolo and Maureen K. BraunPediatrician’s Guide to Discussing Research with Patients10.1007/978-3-319-49547-7_44. Significance: Statistical Versus Clinical
(1)
The Child Study Center, NYU Langone Medical Center, New York, New York, USA
(2)
Department of Pediatrics, The Mount Sinai Hospital, New York, New York, USA
Keywords
Clinical relevanceMisrepresented researchClinical significanceP-hackingReplication studiesOverview
A core aspect of informed consent in medical decision-making is full comprehension of research findings. We have been reviewing the criteria a study must meet before it merits consideration in medical decision-making. The implicit and explicit biases affecting research at various stages and levels are taken seriously, thus weeding out questionable results. Indicators of methodological rigor then provide insight into the overall quality of the study. Once these criteria are met, physicians and patients must still determine if the study results have any plausible clinical applicability. Some studies, despite passing these criteria, yield results that remain academic in nature only. Put bluntly, not every statistically significant research result is ecologically valid. To explain to patients which results apply clinically and which do not, physicians can make clear the difference between statistical significance and clinical significance. Only once this distinction is apparent to patients are they adequately informed to consent.
The Original Plan for Statistical Significance
Not only should statistical significance not conclude the decision-making process, it was never intended to. We caution in Chap. 3 that statistical significance has a narrow and precise definition. Statistical significance provides a guideline as to the likelihood that an observation in a study is due to chance [1]. Originally devised in 1920, statistician Ronald Fisher proposed that his p-value (the statistical metric of significance) provide an indication as to whether or not study results warrant a second examination [1]. Studies with p-values less than 0.05 might yield valuable information if investigated further. P-values higher than 0.05 were not as likely to produce additional results of importance.
To Explain to a Patient
A statistically significant result acts like a traffic “stop” sign. A p-value less than 0.05 does not signal the end of the trip. Instead, it tells the researchers to pause long enough to consider where they came from, what they have observed so far, and where they might like to go next based on what they observed.
Fisher carefully defined the limitations of the p-value out of a nuanced understanding of the role statistics should play in the scientific method. Fisher placed his statistic as one discrete part of the scientific process as a whole. If the process was to unfold in the ideal, researchers would review the preexisting work in the field, incorporate those previous findings into their ideas, set hypotheses, examine their resulting data, share their findings, collaborate with others in the field, and adjust their hypotheses accordingly.
This collaborative, evolving, and messy approach to science sounds foreign to many. The scientific process is often presented as completely methodical, objective, and rational. Consider instead, the scientific process as an art. If the scientific process were compared to the creation of an oil painting, statistics would represent the brushes. While crucial to the endeavor, the brushes alone do not create the resulting image. Other factors must be considered for the process to unfold as originally conceptualized. Describing a particular vein of scientific inquiry by stating that “the study has statistically significant findings” is akin to describing a painting solely by stating that brushes were used.
Popularity of Statistical Significance
In the years since Fisher first proposed that his statistical procedures be used in a limited fashion within a broader context, scientists, publishers, and journalists have removed his p-value from its recommended position of precision [2]. The media use his commonly misunderstood statistic as a catchall for the importance of a study’s findings [2]. The scientific field has taken his statistical tool and raised it to the position of prominence in most published findings. High-impact journals display a preference for publishing papers with statistically significant results [2]. In turn, career academics value publication in high-impact journals [2]. These mutually beneficial incentives conspire to promote an overreliance on statistically significant findings [2]. We propose two reasons for the overinflated popularity of Fisher’s p-value: One is practical and the other psychological.
One factor contributing to the popularity of the p-value within the scientific community was the practical benefit. At the time of Fisher’s writing, computers with statistical capabilities were expensive, making them scarce commodities [2]. Most researchers computed their statistical analyses by hand, a painstaking process. They then compared their results to a table Fisher developed that provided data at various interval levels (e.g., p = 0.05, p = 0.01). At the time, a table with clearly delineated intervals assisted researchers in understanding whether or not their results had any merit. They simply needed to find the lowest possible interval on the table corresponding to their result.
The overreliance on tables with arbitrary guidelines, forgivable one hundred years ago, has overstayed its welcome. Computers, now ubiquitous, calculate statistical results and precise p-values at very little time and cost. Researchers do not need to settle for the closest interval that best describes their findings. While Fisher’s p-value of 0.05 had an outsized effect on the field of statistics when he created it, the practical utility is no longer applicable.
With the practical aspect of Fisher’s p-value clearly technologically outdated, the psychological need for simplicity marches on. It is as an inborn human trait. Cognitive psychologists Tversky and Kahneman studied processes the human brain utilizes to efficiently and rapidly perceive, judge, and make choices about the world [3]. These processes are called heuristics [3]. Compared to other organs, the brain requires a great deal of energy to operate even its most basic functions. The body therefore prefers to run the brain as efficiently as possible. Additionally, humans evolved when speed was crucial to physical survival. If the brain took too long to determine if a stimulus was dangerous, it often resulted in death. Brains, then, also prefer speed. Heuristics provide the advantages of efficiency and speed.
To assist the brain with rapid and efficient processing, heuristics simplify where complexity is encountered. Rather than examine every square inch of an object before determining its type, the human brain will identify key markers (legs, seat, back, wood, right angles, parallel lines) and identify the object as a chair. Fisher’s p-value supplied the statistical equivalent of a heuristic. He provided one key marker (a p-value) by which researchers now identify their work as a whole (significant or not).
Oversimplification and Confusion
Utilizing procedures that simplify come with a cost. The limitations of the human brain mean that giving speed preference over accuracy will lead to an increased error rate. Most humans can be quick or accurate, but it is very challenging to be both. In cognitive terms, the errors that emerge due to heuristics are our biases. One bias that emerges from heuristics’ tendency to simplify is oversimplification. In the case of Fisher’s p-value, the temptation to oversimplify research results is strong.
Not only does relying solely on the p-value oversimplify findings within statistics, it also causes a similar error in the clinical interpretation of the findings. The simplicity error has wide-ranging effects due to the use of the word “significance.” Even though statistics uses the word “significance,” common parlance utilizes it also [4]. Unfortunately, the definitions are not the same in both settings. We will explain the confusion by first providing another example of a term with a different meaning depending on the context in which it is used: negative. In the medical setting, a negative finding often represents good news, such as when screening results for a disease are negative. In quotidian use, negative has the opposite meaning.
Significance suffers from this dual-definition conundrum. In the case of significance, however, the difference between the two definitions is nuanced. Authors often fail to bother at all with the distinction, or neglect to clarify when they see the word used erroneously. An indication of the strong propensity to oversimplify is the frequency with which popular media shortens “statistical significance” to “significance.” For example, one news article reporting an epidemiological study result states, “postmenopausal women with gum disease and history of smoking have a significantly higher risk for breast cancer” [5]. Missing is the key word “statistically”—the researchers calculated a statistically significant association. Omitting this word could reflect a minor editorial decision. In fact, its absence results in the complete dismissal of Fisher’s original intended use for his measure. The common definition of significance is commonly erroneously applied when the much narrower statistical definition is actually needed [6].
Overreliance on Statistical Significance in Publishing
Lay media aside, evidence of publication bias in the scientific literature depicts the field’s overreliance on statistical significance. As discussed in Chap. 2, publication bias results when papers with significant findings are published at higher rates than papers with non-significant ones. The bias is so prevalent that results with statistically significant findings are colloquially referred to as “positive” and those without statistical significance as “negative” [7]. (This shorthand assessment of value is not applicable for types of studies that do not employ hypothesis testing as a matter of form, such as case studies.)
Before addressing the myriad ways publication bias detrimentally affects the field of scientific inquiry, we must first establish how its presence is observed. A number of researchers have studied the mathematical markers that serve as evidence of publication bias and have repeatedly found a great deal of evidence that the bias has infiltrated the literature [7]. Recall that biases affect outcomes in a systematic fashion. When researchers find systematic outcomes instead of the randomness they would expect, they infer the presence of a bias. There is no justifiable reason for a collection of p-values to cluster in any particular formation. Without publication bias, the p-values drawn from a sample of published papers should be randomly distributed. If a pattern of p-values, particularly p-values clustered at just below 0.05 were observed, this marker would imply that investigators mainly submit and journals mostly publish findings using Fisher’s p-value as the driving criterion. Various researchers have repeatedly found this exact clustering of p-values just under 0.05. For example, one team of researchers examined articles reported in three psychology journals. They found that a disproportionately high number of papers report p-values just under 0.05 [8]. We can also observe the bias increasing over time: in 1990, 30% of papers published had negative findings; by 2013 it had dropped to 14% [9].
Costs of Publication Bias
Publication bias obstructs the actions involved in the true directive of the scientific process: knowledge acquisition. First of all, uncovering what is false is just as much a goal of science as discovering what is true, a fact publication bias blithely ignores [7]. Second, publication bias stymies scientific process via redundancy and false leads. In terms of redundancy, the lack of published negative findings presents unnecessary challenges to other researchers who would seek to examine the same phenomena. Without access to accounts of prior work that did not lead to significant results, other researchers (whether future or contemporary) repeatedly test hypotheses that their predecessors and colleagues have already examined and discarded. The time and money spent investigating paths that have already been tested and jettisoned could have been more effectively utilized if the negative findings were readily available [10].
While publication bias has always been a matter of concern, the more studies published, the more the bias affects the field overall with the introduction and permeation of false positives. In 1950, a few hundred thousand researchers worked and published [9]. Even in 1959, researchers were writing about their concerns that publication bias resulted in an abundance of false conclusions [11]. In 2013, the field grew to approximately 6–7 million researchers working and publishing [9]. Recall that a p-value of 0.05 represents a one-in-twenty probability that a finding is due to chance. The result is that even among a few dozen studies, one could reasonably anticipate that at least one of those statistically significant results is, in fact, a false positive. Therefore, as more studies are published, the number of false positive results will increase, even as the rate of false positive findings remains the same.
The larger the number of studies, the more false positives will emerge. Additionally, the larger the amount of data collected within a study, the more false positives it will produce. While a sufficiently large sample size is needed for appropriate methodological rigor, the rise of big data has also contributed to an overabundance of false positive results that pervade the literature. While it would seem that the larger the sample size, the more confidence one can place in results, this is not true [11]. What is more precisely accurate is that the more participants in a study, the more easily the data will be able to provide an outcome that reaches statistical significance. Large sample sizes’ ability to detect differences between samples is called statistical power. However, increasing the power also increases the rate of false positives as well. Large sample sizes magnify both true and false differences. A property inherent in large datasets is great variability [12].
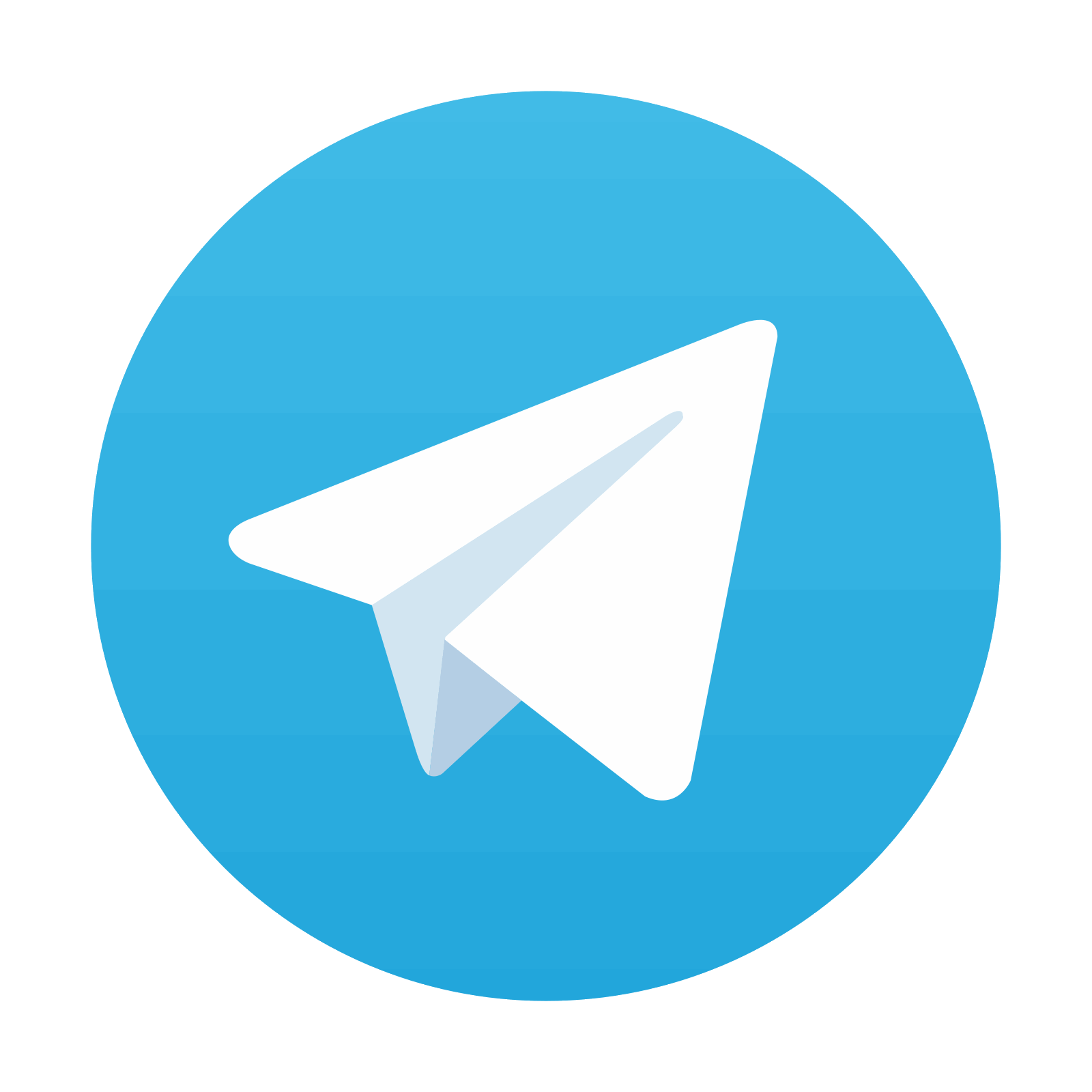
Stay updated, free articles. Join our Telegram channel

Full access? Get Clinical Tree
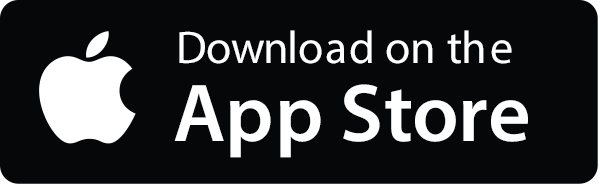
