CHAPTER 12 Principles and new developments in molecular biology
Introduction
Expedited by completion of the draft version of the Human Genome Project in 2001 (Lander et al 2001, Venter et al 2001), the last decade has witnessed unprecedented advances in experimental methods in an ambitious effort to link genomics with whole-organism physiology and pathophysiology. The genome amongst individuals is 99.9% homologous, with diversity of function generated through postgenomic regulation at the mRNA and protein level. Detailed analysis of the human genome shows it to consist of approximately 3.1 billion base pairs (Little 2005). Of the genes, approximately 24,000, far fewer than the number expected, encode proteins with phenotypic diversity generated by multiple splice variants. It is estimated that over 800 additional genes are transcribed into small microRNAs (miRNA), although significantly more encode non-coding RNA (Little 2005). Clearly, the draft sequence has provided tantalising insight into the mysterious organization and complexity of the human genome that will be gradually unravelled for years to come.
Global Screening and Analysis
Traditionally, pursuit of scientific enquiry has been hypothesis-driven, which by its nature deconstructs complex biological systems into manageable ‘bite-sized’ studies with a very specific research question. In our wider quest to understand systems biology and networks, a reductionist approach is ill suited to investigating the integrative nature of whole-organism physiology, specifically the interactions between genes, protein and function. Given the immense volume of sequence data generated by the Human Genome Project, an escalation in research targeted towards population-based, high-throughput screening of gene expression has since been observed. The emphasis on networks, rapid advances in -omic technologies and high-dimensional biology has produced unparalleled insight into the relationships between the genome, transcriptome, proteome and, more recently, the metabolome. These innovative approaches have also necessitated unprecedented dependence on the discipline of bioinformatics. Table 12.1 lists key websites that are the backbone of such investigations requiring information on gene and protein sequences, gene annotation, sequence homology, microarray analysis etc. Many of these are freely available, with two of the most comprehensive and popular sources of sequence information being the National Center for Biotechnological Information (NCBI) and Ensembl.
Table 12.1 Commonly used web-based tools and databases for genome analysis
Function/ institute | Software | URL (http://…) |
---|---|---|
Nucleotide/protein sequence data | NCBI | www.ncbi.nlm.nih.gov |
European Bioinformatics Institute | EMBL | www.ebi.ac.uk |
Expert protein analysis system | Expasy | www.expasy.ch |
Human and other genome sequences | Ensembl | www.ensembl.org |
Human genome sequence data | NCBI | www.ncni.nlm.nih.gov/genome |
Basic local alignment search tool | BLAST | www.ncbi.nlm.nih.gov/blast |
ESTs, full-length clones, libraries | IMAGE | www.geneservice.co.uk/products/image/index.jsp |
Human gene expression | HuGE | www.HugeIndex.org |
Online Mendelian inheritance in man | OMIM | www.ncbi.nlm.nih.gov/omim |
Haplotype map project | HapMap | www.hapmap.org |
Microarray gene expression data | MGED | www.mged.org |
Stanford microarray database | SMD | smd.stanford.edu/ |
Microarray manufacture | Affymetrix | www.affymetrix.org, www.dnachip.org/ |
Primer design | Primer 3 | primer3.sourceforge.net/ |
EST, expressed sequence tag.
Microarray technology
Gene sequence data in isolation provide little information on protein function. ‘Functional genomics’, the term used to describe the relationship between genes and physiological mechanisms on a global rather than individual basis, has evolved to probe these interactions. In particular, pioneering research using genechip technology enabling simultaneous global gene expression of thousand of genes (Schena et al 1995, Brown and Botstein 1999) has been at the forefront of functional genomics, and is the mainstay of high-throughput assays. It is a powerful means of identifying subsets of genes that are either up- or downregulated in a particular research scenario (Duggan et al 1999, Hegde et al 2000). Specifically, gene expression may be compared: (1) in different tissues/cells, (2) under developmental regulation (fetal versus adult) and on ageing, (3) in normal and disease states, and (4) in response to, for example, drug treatments, environmental cues etc.
Practical Aspects and Analysis
Microarrays
The success of DNA microarray technology exploits the complementarity of Watson–Crick base pairing that underlies hybridization of sample cDNA to either short oligonucleotide or cDNA sequences immobilized in a grid-like fashion on a solid substrate (Duggan et al 1999, Hegde et al 2000). Typically, a glass slide, nylon membrane or silicon wafer is the preferred format, although bead-based arrays are also available. For cDNA arrays, probe sequences principally derive from the IMAGE (Integrated Molecular Analysis of Genomes and their Expression) clone library arising from the Human Genome Project. These arrays include known genes whose function is as yet unknown, and expressed sequence tags whose full sequence and function are yet to be determined. High-density oligonucleotide arrays, such as those provided by Affymetrix, may be fabricated in situ by solid-phase chemical synthesis combined with photolithography. The sequences on Affymetric chips derive from ‘refseq’ — the definitive version of the gene sequence — contained within the NCBI suite of programs (Table 12.1). Currently, the latest arrays from Affymetrix offer unparalleled whole-genome analysis with over 700,000 probes representing over 28,000 human genes on a single array. Despite the diversity of microarrays available, some may not include the gene of interest to the specific research group, leading to the loss of key information in the study of gene pathways. An alternative strategy is to manufacture tailored arrays that include a smaller set of known genes of interest.
Once a research question has been formulated, the basic steps in a DNA microarray experiment, illustrated in Figure 12.1, commence with extraction and reverse transcription of total RNA or mRNA into cDNA from samples under investigation. Sample cDNA is then labelled either with radioactive or fluorescent probes and hybridized to the array. The main advantage of radioactive over fluorescent labelling is the enhanced sensitivity of the former. A currently popular approach involves differential labelling of test and control sample cDNA with the fluorophores Cy3 and Cy5. Following stringent washing to remove non-specific binding, image analysis of the emitted signal is performed either by quantitative phosphorimaging of radioactively labelled samples or by powerful laser scanning of fluorescence. These raw data are subsequently processed to generate scatter plots that offer a quick and easy means of surveying unaltered, up- or downregulated genes. A gene expression matrix, consisting of data organized in rows and columns reflecting the output of each individual gene in a particular sample, allows the data to be ranked and probabilities determined in order to identify individual fold changes in gene expression as a result of a biological effect. Typically, more than a two-fold change in transcript expression is taken to indicate biological relevance.
Inherent in these methods is the technical variation arising from a number of sources including RNA quality, variable probe labelling, high background etc. Distinguishing between real experimental changes and those due to technical variation is achieved by some form of normalization designed to remove bias and to ensure that the results obtained are an accurate depiction of a true biological effect. Normalization generally requires subtraction of background intensity from the signal of each gene. Other forms of normalization include comparison of gene expression against a known set of reference (housekeeping) genes included on the array that demonstrate constant expression irrespective of tissue type and conditions. The Human Gene Expression database (Table 12.1) is a useful source of information of such genes expressed in a variety of tissues.
Statistical analysis
An alternative approach is to use fold change, in which the within-treatment variance is ignored. The problem with this is that variances do matter and vary between genes and treatments. Moreover, fold change may stem from an initially low level of expression, possibly reducing the biological significance of the change. A method of improving the power of the t-test is to use a Bayesian statistical approach that uses prior knowledge of within-treatment measurement (Baldi et al 1998). In the case of arrays, this is achieved by assuming that genes with similar expression levels have similar measurement errors (Baldi and Long 2001). In addition, the data are not viewed in isolation but in the context of known biological interactions.
Given the caveats that apply to the use of the t-test for statistical analysis of array data, an alternative method used to derive probability values that has been applied to microarray data is the use of permutation tests. Unlike the t-test, permutation tests do not require data to be normally distributed nor variances to be equal, and are therefore more likely to detect real changes in expression. Tusher et al (2001) have developed a method that uses permutation tests known as ‘Significance Analysis of Microarrays’ (SAM), where each gene is assigned a score taking into account its expression and standard deviation. The uptake of the SAM method has been greatly facilitated by the availability of an Excel plug-in. It also provides information on the false discovery rate, which corrects for false-positive results in order to eliminate random changes in gene expression.
Exploratory data analysis and data models
Since the aim of most microarray experiments is to identify differential gene expression compared with a control sample/condition, some means of organizing genes into meaningful subsets forms part of exploratory analysis. For example, a comparison between normal and endometriotic tissue may unveil changes in multiple genes involved in inflammation or cell adhesion. Similarly, treating a cell line with steroids may lead to a downregulation of genes encoding inflammatory cytokines. Exploratory analysis investigates such relationships using unsupervised or supervised classification to identify patterns of gene expression based on similarity measurements, yet providing little or no information on the statistical significance of the findings. Supervised classification is distinct from unsupervised methods since it involves making prior assumptions about the data sets but may introduce bias (Shipp et al 2002).
Broadly speaking, unsupervised methods that include cluster analysis (Kerr and Churchill 2007) or principal components analysis (Hilsenbeck et al 1999) identify groups of genes that change and cluster them into cognate groups. One of the shortfalls of this approach is that genes that do show changes with expression but have no perceived function may be overlooked. This is more so when one considers that it is likely that several genes may be implicated in a physiological response. A further problem is in identifying the best model within which to place the individual gene expression changes. Genes may be clustered using hierarchical clustering (Eisen et al 1998), two-way clustering (Alon et al 1999), k-mean clustering, principal components analysis (Tavazoie et al 1999) and self-organizing maps (Tamayo et al 1999). It is also preferable to determine the validity of applying particular clustering algorithms to one’s data by carrying out bootstrap or jack knife analysis (Reimers 2005). Detailed mathematical arguments relating to supervised and unsupervised methods are beyond the scope of this chapter; readers are referred to the preceding references and the following sources for further information: Reimers (2005), D’Haeseleer (2005), Thalamuthu et al (2006) and Kerr et al (2008).
Limitations of microarrays
Although the use of microarrays has dramatically altered the field of molecular biology, it is no easy task to compare data across groups and arrays due to the variation in arrays used, normalization protocols employed, and statistical analyses and exploratory models applied. In an attempt to reach a consensus on sharing and storing array data, Brazma et al (2001) have launched Minimum Information About A Microarray Experiment (MIAME) as a mechanism for recording detailed relevant information on the execution and analysis of array experiments made publicly available for use by independent researchers.
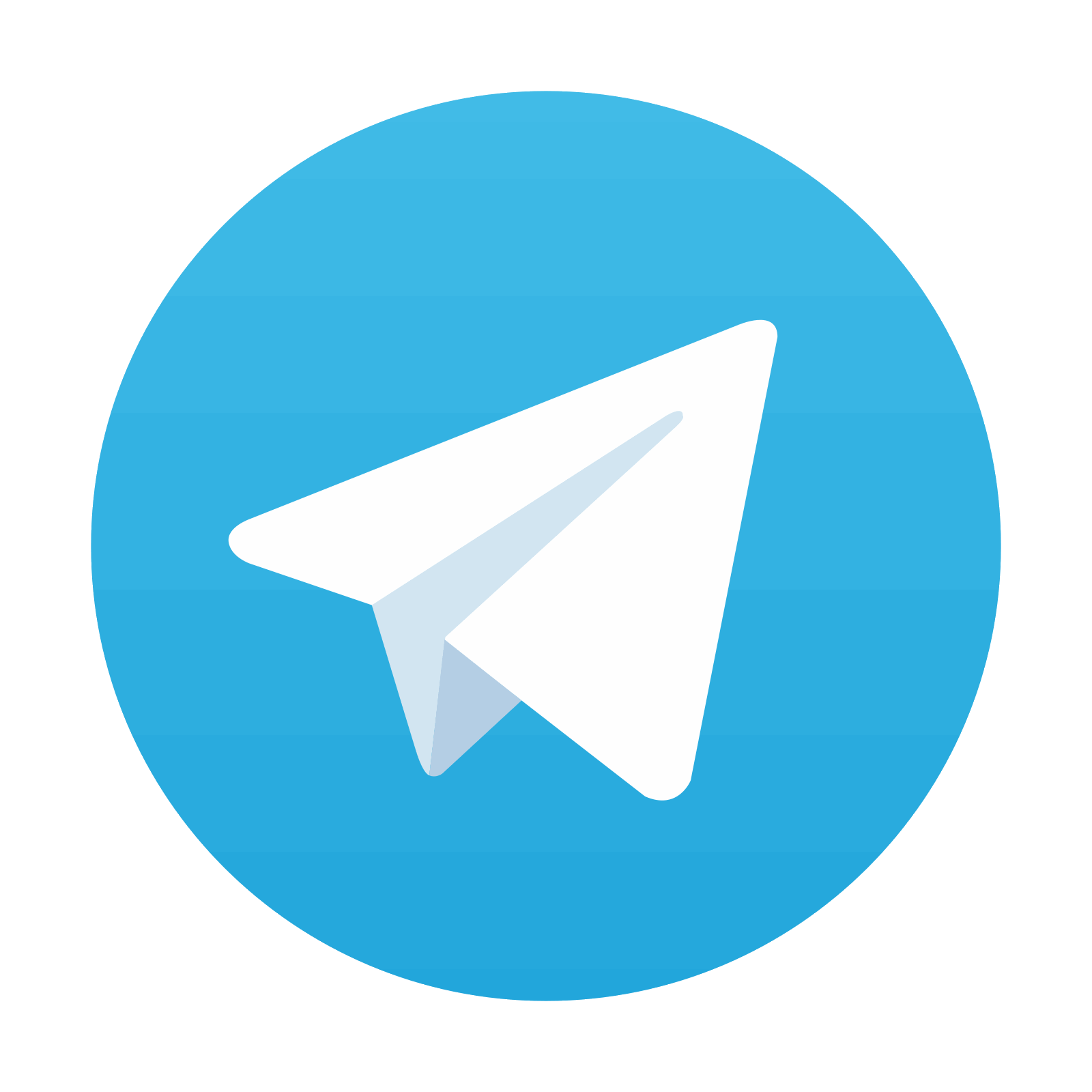
Stay updated, free articles. Join our Telegram channel

Full access? Get Clinical Tree
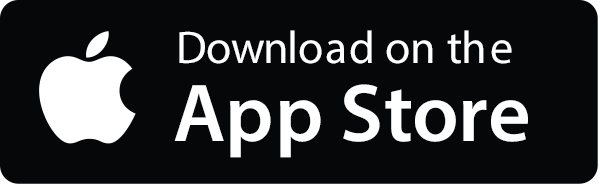
