Fig. 33.1
The translational pathway
The focus on translation is due to the ever-increasing research budgets in the face of stubbornly stable global or national health indices. Research that has no potential for translation may be regarded as having less potential value. It is harder for basic science researchers to follow areas of interest or curiosity with no clear idea of how the outcomes of the research might eventually make a difference to health outcomes.
Traditionally the pathway has gone from basic science to clinical to community; discovery to innovation to application. However, the pathway can and should go both ways. For greater efficiency, public health imperatives should drive the questions that drive the basic science research.
There are two well-described blocks in translation. The first is often known as T1 and is the bottleneck between basic science discovery in the lab and early phase clinical studies. Many promising therapies never make it over the “valley of death” into clinical trials [5]. For every successful drug in clinical trials, dozens are tested in the lab. This greatly increases the cost of developing new drugs. The second block, known as T2, is between successful trials and change in practice and improved outcomes [6]. Implementation science addresses this block.
An important concept is research into translation. Funding bodies and pharmaceutical companies are looking for ways to improve the process of translation to make the whole medical research system more efficient using such technologies as advanced modeling and bioinformatics [7].
Quality Improvement
Quality improvement (QI) involves formal analysis of performance followed by the use of systematic efforts to improve it. The Institute of Medicine in the USA defines quality along six dimensions: safety, effectiveness, patient-centered, timeliness, efficiency, and equity [8].
Strictly speaking, outcomes reported in quality improvement programs are institution specific. Thus they are not generalizable knowledge and the results themselves are not publishable [9]. However, this distinction may be blurred. QI activity may reveal novel associations or insights into therapy that are indeed new, generalizable, and publishable; thus, while the QI data was not primarily collected for research, research may be performed on the data. As more and more data are accumulated by QI projects, more research studies are performed “mining” this data. There are, however, significant limitations inherent in this data mining (see later).
QI may also be publishable as a report of the system itself rather than the results. Such QI reports are an integral part of health service delivery research.
Audit is a term often used to mean the systematic collection of new or examination of existing clinical data. To avoid confusion, it is preferable to try and avoid the term if possible. If the study is a cohort study, then it is best to call it a cohort study rather than an audit. If the audit is part of a QI project, then indicate it is part of a QI project.
The Theory of Research
To fully understand the limitations of research requires an understanding of the theory and philosophy underlying research.
Mechanistic and Empiric Research
In ancient Greece, medical practitioners were either empiricist or dogmatists. An empiricist made no attempt to describe or understand the mechanism underlying disease but could predict an outcome from having seen the outcome after a large number of similar observations. A dogmatist relied on underlying truths or universal laws to explain mechanisms and hence predict the outcome. The two were merged by Galen who thought that treatment was best based on both reason and experience. In some ways, modern medicine is still a mix of empiricist and dogmatist. The dogmatist philosophy is that the molecular or even genomic mechanisms underlying disease and therapy can help clinicians understand what is going on with their patient and hence how best to treat them. On the other hand, the empiricist approach is to guide therapy based on large well-conducted observational studies. Sir Bradford Hills echoed Galen when he stated that causation was best proven with both strength of association and biologic plausibility. Clinicians seek to follow evidence-based practice. That evidence is based on both the well-described hierarchy of empiric evidence (often expressed in terms of level of evidence, with a meta-analysis of randomized trials being the highest level of evidence) and on an understanding of the mechanisms. Clinicians rarely rely on purely empiric or purely mechanistic evidence. Thus research can be either mechanistic or empiric and both are equally needed to guide practice. When designing studies, it sometimes helps to think if you are seeking empiric or mechanistic evidence. For example, is your study seeking to determine the pharmacokinetics of propofol in children, or is it seeking to see if propofol TIVA improves recovery? Mechanistic studies seek tightly controlled experimental conditions to reduce natural variability.
Hypotheses
Research is often centered on hypotheses. It is important to understand the limitations of hypothesis-driven research. Inductive reasoning is the generation of a “law” or “truth” based on a number of observations. In contrast, deductive reasoning is applying general laws to predict a particular outcome. The validity of inductive reasoning is inherently limited as the number of observations is always limited. For example, a man may see many white swans without ever seeing a black swan and incorrectly deduce that all swans are white.
In the early twentieth century, the hypothetico-deductive method was introduced to address the limitations of inductive reasoning. This involves a hypothesis being generated and then tested with an experiment or observation. If the hypothesis does not fit with the observation, it is rejected. To test a hypothesis, the null hypothesis is first generated; a statement that the intervention has no effect on the outcome of interest. The complementary alternative hypothesis is that the intervention does have some effect. In clinical research, a sample is tested and an inference made on the population from which the sample is drawn. In particular, the null hypothesis is that there is no effect seen in the population from which the sample was drawn. The P value is the probability that the result seen in the sample could have occurred randomly or by chance. If the P value is less than an arbitrary set point (often 0.05), then the null hypothesis is rejected and it is thus assumed that the intervention has some effect. A type I error is if the null hypothesis is incorrectly rejected while a type II error is if the null hypothesis is incorrectly accepted.
Most trials are superiority trials where the null hypothesis is that there is no effect. A trial may also be designed as an equivalence trial where the null hypothesis is that the effect is greater than some set level (a point predetermined as being clinically significant). Equivalence and superiority trials have subtle differences in design and analysis.
There are limitations in using P values. Firstly, it tells the reader nothing about the size or magnitude of the effect. A P value may be <0.05 but the magnitude of the effect or difference might be clinically irrelevant. Secondly, the set point of 0.05 is entirely arbitrary. Using a P value and the underlying hyothetico-deductive method forces clinical research into a dichotomous outcome; either something has an effect or not. This is not well suited to clinical research where we may be more interested in the size or magnitude of an effect. Both the P value and hypotheses are likely to be gradually phased out of clinical research.
Instead of testing hypotheses and generating P values, a superior method is to consider a trial as a way to estimate the size of effect along with an indication of the precision around the estimate of that effect. This is expressed as reporting the actual effect (often a difference in means, risk ratio, odds ratio, or something similar) and the 95 % confidence intervals around the effect. This gives the reader an idea of the magnitude of the effect and the precision around the estimate.
The word “statistical significance” is often used to indicate a result with a P value <0.05. In contrast, “clinical significance” is used to indicate that the magnitude of the effect is enough to be clinically important. Using the word “significant” alone is ambiguous and thus meaningless. As mentioned before, “statistically significant” is entirely arbitrary and should perhaps be avoided. Similarly “clinically significant” can also be problematic; as what is clinically important may vary between populations and clinicians. If the words “clinically significant” are to be used, then it is optimal to justify why that size of effect is indeed important.
Problems also arise differentiating “no difference” from “equivalence.” These problems are more acute if P values are used. No difference usually means the P value is greater than 0.05. However, this does not mean equivalence. Firstly, strictly speaking, equivalence can only be determined if the trial was designed and analyzed as an equivalence trial. Secondly, equivalence can only be assumed if the 95 % confidence interval around the observed difference does not cross what would be regarded as a clinically significant difference.
Lastly, as previously mentioned, even if the P value is >0.05, the breadth of the 95 % confidence interval still needs to be considered in terms of what might be clinically relevant. If the 95 % confidence interval is entirely beneath what would be regarded as clinically relevant, then the result shows a “statistically significant” difference that is “clinically insignificant.” If the P value is >0.05 and the 95 % interval partly lies below what may be regarded as clinically relevant. The result is “statistically significant” and possibly “clinically significant.”
In summary, it is best to avoid P values and better to report 95 % confidence intervals. Authors should only claim there is a difference, no difference, or equivalence if the magnitude and precision of the result clearly fit these criteria. If there is any doubt, then report the findings and let the reader decide if the precision and magnitude of effect warrant a conclusion that the result is a clinically relevant difference, no difference, or equivalence.
Doing a Clinical Research Project
For research to be useful and not misleading, it must be of high quality. Doing high-quality research is not a simple task. The study must be well designed and carefully conducted. Researchers now rarely work in isolation. The simple questions have been answered and the remaining more challenging questions require efficient and effective research teams maximizing collaboration and sharing skills and resources; the wise saying of “publish or perish” has been replaced by “collaborate or collapse.”
The Research Question
The most important element in any research study is the research question. Without a clear prospective research question, there is no research project. If you have ever read a research paper and had no idea what the paper was about, then the researchers either had no coherent questions or they were unable to clearly articulate their question. The question drives all stages of the project: from protocol, to conduct, to analysis, and publication.
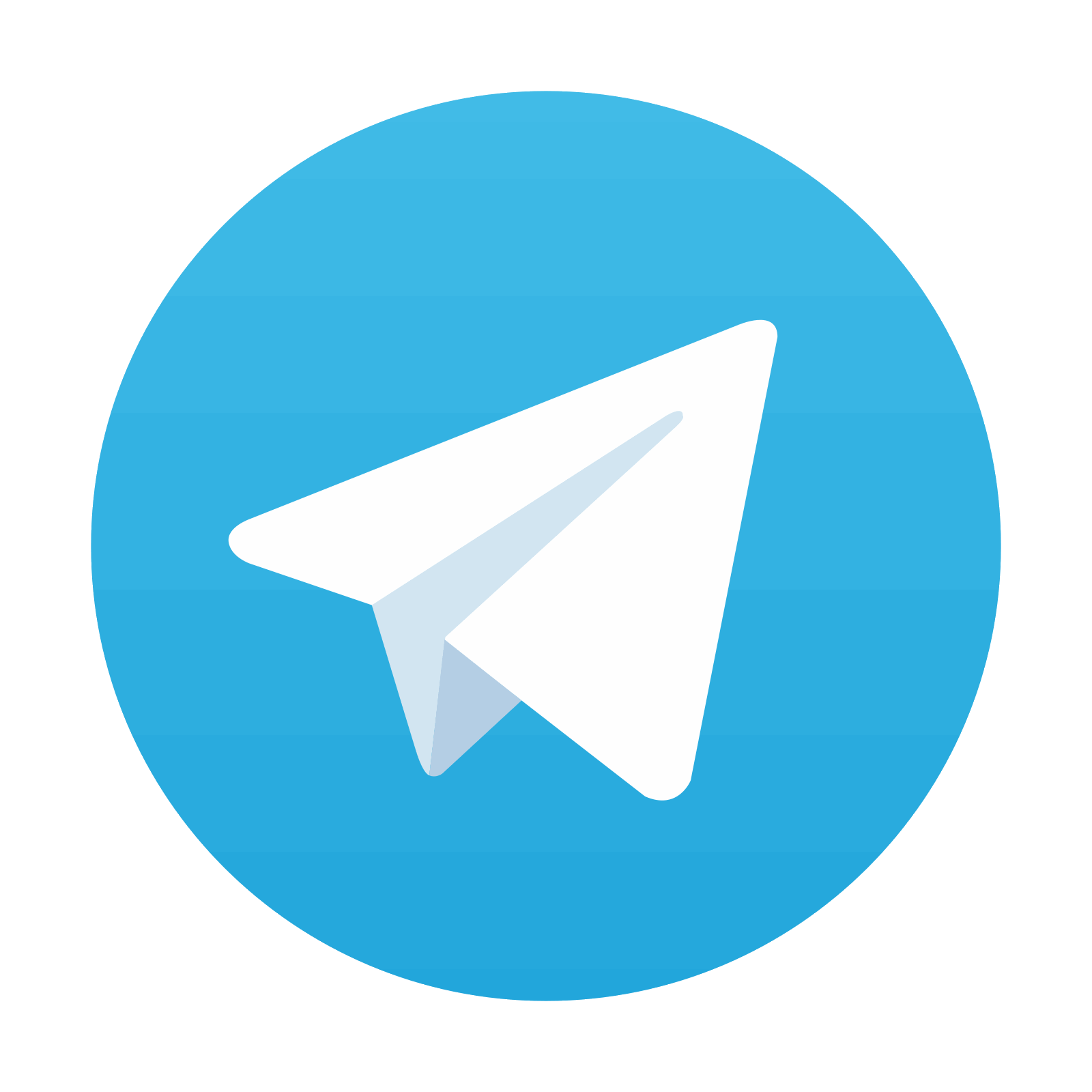
Stay updated, free articles. Join our Telegram channel

Full access? Get Clinical Tree
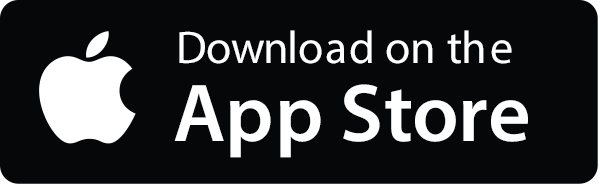
