Clinical Trials Methodology and Biostatistics
MARK F. BRADY JEFFREY C. MIECZNIKOWSKI
VIRGINIA L. FILIACI
Issues involved in the design, conduct, and analyses of clinical trials are presented in this chapter. Phase 1, 2, and 3 trials are presented, as well as screening trials. Statistical jargon and mathematical notation are avoided wherever possible. Studies from the field of gynecologic oncology are used as examples in order to illustrate specific points. This chapter is an overview of these very expansive topics. Details concerning the design, conduct, and analysis of clinical trials can be found in books dedicated to this subject, such as Piantadosi (2005) (1), Green et al. (2003) (2).
This chapter begins with some historical snippets in order to provide a sense of how the concepts of clinical trials evolved and matured from an intuition-based practice of medicine to the more sophisticated evidence-based medicine used today. The procedures for designing, conducting, and analyzing clinical trials continue to mature as the demand for evidence-based medicine increases. Next, a general system of classifying study designs is presented. The subsequent sections in this chapter describe the components of a clinical trial and essential considerations for developing new trials. Since translational research objectives are incorporated into many modern cancer trials, some issues related to design and analyses of these components are also considered.
HISTORICAL PERSPECTIVE
Clinicians make treatment recommendations daily. These recommendations arise from culling information from standardized clinical guidelines, published reports, expert opinion, or personal experiences. The synthesis of information from these sources into a particular recommendation for an individual patient is based on a clinician’s personal judgment. But what constitutes reliable and valid information worthy of consideration? Clinicians have long recognized that properly planned and conducted clinical trials are important sources of empirical evidence for shaping clinical judgment.
The Greek physician Hippocrates in the 5th century BCE had a profound influence in freeing medicine from superstition. He rejected the notion that the cause of an individual’s disease had a divine origin. Rather, he postulated that diseases originated from natural causes that could be determined from observing environmental factors like diet, drinking water, or local weather. He also proposed the revolutionary concept that a physician could anticipate the course of a disease after carefully observing a sufficient number of cases. Despite these insights, medicine all too frequently continued to consist of a mixture of herbal concoctions, purging, bloodletting, and astrology.
In the Middle Ages, Avicenna (980–1037 CE) wrote Canon of Medicine, a work considered to be the pre-eminent source of medical and pharmaceutical knowledge of its time. In his writings Avicenna proposed some rules for testing clinical interventions. First, he pointed to the need to experiment in humans, since “testing a drug on a lion or horse might not prove anything about its effect on man.” Also, he described the basic experiment as observing pairs of individuals with uncomplicated but similar forms of the disease. Finally, he emphasized the need for careful observation of the times of an intervention and then reporting the reproducibility of its effects (3). Despite these insights, his Canon of Medicine does not include any specific reports of clinical experimentation.
Early trials frequently lacked concurrent comparison groups. For example, Lady Mary Wortley-Montague in 1721 urged King George I to commute the sentences of 6 inmates from the Newgate prison if they agreed to participate in a smallpox inoculation trial (3). The prisoners were inoculated with smallpox matter from a patient with a naturally occurring form of the disease. Since these individuals remained free of smallpox, this finding was considered to be evidence supporting treatment by inoculation. Later, however, the results of this trial were considered less compelling, when it was discovered that some of the inmates might have been exposed to smallpox prior to the trial (4). Without a proper control group, it can be difficult to interpret the efficacy of an intervention. There are frequently many factors, both known and unknown, that influence an individual’s outcome.
Subjective assessments from either the subject or the practitioner may also influence the interpretability of a trial. In the late 18th century, the King of France selected Benjamin Franklin to head a royal commission to investigate the physician Franz Mesmer’s claims that he could promote good health by manipulating a force he called “animal magnetism” (5). Franklin devised several ingenious experiments in which the subjects were blindfolded and not told whether or not they were receiving Mesmer’s treatment. These trials led Franklin to conclude that the therapeutic effects of mesmerism were due to imagination and illusion. Several years later, John Haygarth demonstrated the importance of controlling for assessment biases. At that time, magnetic healing rods were commonly used to relieve pain due to chronic rheumatism (4). Haygarth treated 5 patients on 2 consecutive days, once with sham wooden rods and once with genuine magnetic rods. He noted that 4 individuals reported pain relief and 1 experienced no relief, regardless of which rods were used. Franklin’s and Haygarth’s trials established the importance of implementing experimental procedures aimed at removing subjectivity from the assessment of treatment effects.
It had long been appreciated that there is a potential for misguided inference about the effectiveness of a treatment when the comparison involves a group with a dissimilar prognosis. The apparent benefits attributed to a new treatment might in fact be biases due to prognostic differences in patients between treatment groups. One of the earliest clinical trials to attempt to address this bias was reported in 1898 by Johannes Fibiger (6). Four hundred and eighty-four patients admitted to his clinic with diphtheria were given either standard care or diphtheria serum twice daily. In order to disassociate the mode of treatment from the individual’s prognosis, the patient’s treatment was to be determined by the day of clinic admission, and it alternated from day to day (7). Using this type of procedure to disassociate prognosis from treatment is only partially effective, since the practitioner or the subject knew which treatment would be applied, and this knowledge may have influenced the individual’s decision to participate in the study. Therefore, masking the treatment assignment so that it cannot be part of the decision to participate in the trial is important for controlling this source of selection bias.
Treatment randomization in agricultural trials was performed as early as 1926 (8). However, it was not until the 1940s when Corwin Hinshaw (9), using a coin toss, and later Bradford Hill (10), using random numbers in sealed envelopes, established the methodologic importance of randomizing treatments in clinical trials. Balancing the unpredictable and spontaneous remissions exhibited by some patients with pulmonary tuberculosis motivated Amberson (8) and later Hinshaw (9). While methodologic principles motivated Hill, the short supply of streptomycin after World War II provided the opportunity to use randomization as an equitable way to distribute the drug (11).
One of the first randomized clinical trials in the study of gynecologic malignancies was initiated in 1948 (12). This trial compared ovarian irradiation to standard treatment for breast cancer. Initially, shuffled sealed envelopes were used to designate treatment. However, this procedure was later changed due to administrative difficulties so that treatment depended on the woman’s date of birth. This later procedure is susceptible to the same selection bias mentioned in Fibiger’s trials, since the treatments were not masked.
Despite these methodological advances, the inadequate justification for selecting one medical procedure over another led one contemporary physician to express his frustration:
Early in my medical career I was appalled at the ‘willy-nilly’ fashion by which treatment regimens slipped in and out of popularity. How many operations that I was trained to do or medicines that I was instructed to give because of somebody’s conviction they were beneficial passed into oblivion for no apparent reason, only to be replaced by others of equally dubious worth? (13)
The first randomized clinical trial at the National Cancer Institute (NCI) was begun in 1955 (14). This trial involved 65 evaluable patients with acute leukemia from 4 different institutions. The trial incorporated many elements that should be part of any modern clinical trial: uniform criteria for response assessment, a randomized comparison of at least 2 treatment regimens, and a complete accounting of all patients entered. The published report included considerations for such issues as patient selection bias and the study’s impact on the patient’s welfare (15). The first NCI randomized clinical trial in solid tumors followed shortly thereafter and was organized by the Eastern Group for Solid Tumor Chemotherapy (16). By 2010, there were 12 NCI-sponsored cooperative groups conducting clinical trials and the annual funding for these groups was $163 million dollars (17).
In the late 1950s, most patients with gynecologic cancers were included incidentally in small trials to screen new agents for broad-range activity. The first NCI-sponsored group to organize trials specifically for gynecologic malignancies was the Surgical Ovarian Adjuvant Group. This group was formed in the late 1950s, initiated a few trials, and then disbanded in 1964. Starting in 1963, the Surgical Endometrial Adjuvant Group was one of the earliest efforts toward a multidisciplinary group. This multidisciplinary cooperative group included gynecologic surgeons, medical oncologists, pathologists, radiation oncologists, and biostatisticians. It was from this group that the Gynecologic Oncology Group (GOG) was eventually formed in 1970 to deal with a broader range of gynecologic malignancies (18). Over the following 10 years, the field of gynecologic oncology matured. Board certification procedures for gynecologic oncologists were developed, fellowship programs were initiated in the comprehensive cancer centers, and a professional Society of Gynecologic Oncologists was organized in 1969.
Classification of Study Designs
In general, a clinical trial is any experiment involving human subjects that evaluates an intervention that attempts to reduce the impact of a specific disease in a particular population. When an intervention is applied in order to prevent the onset of a particular disease, the trial is classified as a primary prevention trial. For example, a primary prevention trial may evaluate healthy lifestyles or a vitamin supplement in a population of individuals who are considered to be at risk for a particular disease. Secondary prevention trials evaluate interventions that are applied to individuals with early stages of a disease in order to reduce their risk of progressing to more advanced stages of the disease. Tertiary intervention trials are aimed at evaluating interventions that reduce the risk of morbidity or mortality due to a particular disease.
Clinical trials that evaluate methods for detecting a disease in a preclinical state are called screening trials. Early detection may mean diagnosing a malignancy in an early stage (e.g., the use of mammography in the detection of breast cancer) or in a premalignant state (e.g., the use of Papanicolaou smear in the detection of cervical intraepithelial neoplasia). There are typically 2 interventions in a screening trial. The first intervention is the screening program (e.g., annual mammograms), which involves individuals who appear to be free of the disease. However, once the disease is detected in an individual, a secondary intervention (e.g., surgery) is performed in hopes of stopping the disease from progressing to more advanced stages. Consequently, screening trials require both interventions to be effective. An effective screening procedure is useless if the secondary intervention does not alter the course of the disease. On the other hand, an ineffective screening procedure would cause the secondary intervention to be applied indiscriminately.
Nonexperimental studies (or observational studies) can be classified into 3 broad design categories: cohort (or prospective), case-control (or retrospective), and cross-sectional. In a cohort study, individuals are initially identified according to their exposure status, which can include environmental, genetic, lifestyle, or therapeutic treatment factors. These individuals are then followed in order to determine who develops the disease. The aim of these studies is to assess whether the exposure is associated with the incidence of the disease under study. This design is in contrast to the case-control study, which identifies individuals on the basis of whether they have the disease and then measures and compares their exposure histories. Measuring exposure may be as simple as questioning the individuals about their personal or employment history, or it may involve a more sophisticated assessment such as analyzing the individual’s biologic specimens for markers of exposure. Though the case-control study is susceptible to several methodological flaws, it has the advantage of often being less expensive, easier, and quicker to perform than a cohort study, especially when the disease is rare. The power of the case-control design is apparent from a review of the case-control studies published between 1975 and 1985(19), which demonstrated the deleterious effects of exogenous estrogens in perimenopausal women. Finally, the goal of the cross-sectional study is to describe the prevalence of a disease and an exposure in a population during a specific period of time. These studies can often be conducted more quickly than either the cohort or case-control study, but they are often not used when the time between exposures and the disease onset is long, as it is for cancer.
Components of a Clinical Trial
Objectives
In clinical oncology research, where the disease is often fatal, the ultimate purpose of a research program is to develop a treatment plan that puts the patients into a disease-free state, reduces the risk of cancer recurrence, and allows patients to return to their normal lifestyle within a reasonable period of time. The objectives of a particular clinical trial are often more specific and less grandiose. A clinical trial attempts to answer a precisely defined set of questions with respect to the effects of a particular treatment(s) (20). These questions (objectives) form the foundation upon which the rest of the trial is built. The study objective typically incorporates 3 elements: the interventions to be evaluated, the “yardstick” to be used to measure treatment benefit (see Endpoints), and a brief description of the target population (see Eligibility Criteria). These 3 elements (i.e., “what,” “how,” and “who”) should be stated in the most precise, clear, and concise terms possible.
The choice of experimental therapy to be evaluated in a randomized clinical trial is not always easy. Both a plethora of new therapies and the absence of innovative concepts make for difficult choices. The former is problematic since many malignancies in gynecologic oncology are relatively uncommon. Definitive clinical trials may require 4 to 5 years to accrue a sufficient number of patients even in a cooperative group setting. This time-frame limits the number of new therapies that can be evaluated. The absence of new therapies is a problem since substantial increases in patient benefit are less likely to be observed in trials that merely alter doses or schedules of already acceptable therapies. An open dialogue among expert investigators remains the most effective approach for establishing the objectives of any clinical trial.
Endpoints
The endpoint of a trial is some measurable entity in the patient’s disease process that can be used to assess the effectiveness of an intervention. A study may assess more than 1 endpoint, but in these instances the endpoint of primary interest should be clearly specified or else the study design should carefully reflect the complexity of interpreting multiple outcomes. Endpoints can be a composite measure of multiple outcomes. For instance, some studies assessing quality of life aggregate patient reported scores from several related items or domains that are all considered components of a larger concept called quality of life.
Selecting endpoints that will reflect the effectiveness of an experimental therapy is not always obvious. First, endpoints should be a valid (unbiased) measure of the treatment effect on the disease process. Endpoints that are susceptible to a systematic error that favors one treatment lead to biased estimates of the treatment’s effect. For instance, trials assessing time to disease progression, in which the schedule for CT scans is different for each treatment group, are susceptible to this assessment bias. This problem is not uncommon in studies comparing two different modalities such as chemotherapy and radiation therapy. Second, the measurement of an endpoint should be reliable and not susceptible to subjective interpretation. In some cases clinical response can be considered unreliable, since it is not uncommon for experts to disagree when interpreting the same radiograms (21). Third, endpoints that are directly relevant to the patient are preferable, although valid surrogate endpoints are considered indirectly relevant to the patient. Finally, endpoints which are not too expensive or inconvenient for the patient are preferred. It is not always possible for a single endpoint to exhibit all of these characteristics simultaneously. For example, avoiding death is extremely relevant to a patient with a lethal disease like advanced gynecologic cancer. Also, survival can be measured very reliably. However, most cancer patients will not only receive the treatments prescribed by the study, but, after exhibiting signs of disease progression, they also receive other anticancer therapies. In this case, the validity of overall survival comparisons is suspected since they not only reflect the effects of the study treatment, but also the effects of other therapies that are external to the study. For example, a meta-analysis of those trials comparing the survival of patients, who were randomized to nonplatinum versus platinum regimens for the treatment of advanced ovarian cancer, concluded that these trials appear to have underestimated the true effect of platinum on survival. The reason is that many patients who were not randomized to the platinum regimen eventually received platinum treatment (22). For this reason, progression-free survival (PFS) is often considered a reasonable endpoint in those trials, since it is unaffected by subsequent therapies (23). Nevertheless, some authors have argued that survival remains an important endpoint to be assessed in Phase III trials, even when active second-line treatments are available (24). Moreover, overall survival remains the recommended preferred endpoint in trials that explicitly compare a strategy of immediate treatment versus delayed treatment for advanced stage disease.
Endpoints may be classified as categorical (e.g., clinical response), continuous (e.g., serum CA-125 values) or time-to-event (e.g., survival time). A time-to-event endpoint includes both time (a continuous measure) and censoring status (categorical measure). The data type influences the methods of analysis.
Measurement Errors
The susceptibility of an outcome to measurement errors is an important consideration when choosing an endpoint. Both random and systematic errors are components of measurement error. Random error refers to that part of the variation that occurs among measurements that is not predictable, and appears to be due to chance alone. For example, a serum sample could be divided into 10 aliquots and submitted to the laboratory for CA-125 determinations. If the laboratory returns nearly the same value for each aliquot, then the associated random error is low, and the measurement may be deemed reproducible. On the other hand, if the CA-125 values vary considerably among aliquots, perhaps due to inconsistent laboratory procedures, then individual values may be considered unreliable. In this case, taking the average CA-125 measurement across all 10 aliquots is expected to be a better estimate of the patient’s true CA-125 value than any single measurement.
Concern for reliability is one of the reasons for incorporating several items into a quality of life questionnaire. Quality of life is a complex entity and no single question can be expected to reliably measure it. A measurement of an individual’s quality of life is therefore typically composed from an individual’s responses to several items that are all considered to be associated with quality of life. Indeed, one step toward demonstrating the usefulness of any quality of life instrument is to show that it provides reproducible results when assessing individuals under the same conditions (25).
Systematic error, also called bias, refers to deviations from the true value that occur in a systematic fashion. For example, suppose an investigator initiates a randomized clinical trial comparing two treatments with time to disease progression being the primary endpoint. The protocol indicates that the patient should be assessed after each cycle of therapy. However, suppose that a cycle duration is 2 weeks for one treatment group, and 4 weeks for the other treatment group. Using a more intense assessment schedule for 1 treatment group would tend to detect failures earlier in that group. Therefore, the time-to-failure comparison between treatments would systematically favor the second treatment group.
When there are recognized sources of error, it is important that the study design implement procedures to avoid or minimize their effect. For example, random error in many cases can be accommodated by either increasing the number of individuals in the study, or in some cases by increasing the number of assessments performed on each individual. Systematic measurement error cannot be addressed by increasing the sample size. In fact, increasing the sample size may exacerbate the problem since small systematic errors in large comparative trials contribute to the chances of erroneously concluding that the treatment effect is “significant.” The approaches to controlling sources of systematic error tend to be procedural. For example, treatment randomization is used to control selection bias, placebos are used to control observer bias, standardized assessment procedures and schedules are used to control measurement bias, and stratified analyses are used to control biases due to confounding. For an extensive description of biases that can occur in analytic research see Sackett, 1979 (26).
Surrogate Endpoints
Surrogate endpoints do not necessarily have direct clinical relevance to the patient. Instead, surrogate endpoints are intermediate events in the etiologic pathway to other events that are directly relevant to the patient (27). The degree to which a treatment’s effect on a surrogate endpoint predicts the treatment’s effect on a clinically relevant endpoint is a measure of the surrogate’s validity. The ideal surrogate endpoint is an observable event that is a necessary and sufficient precursor in the causal pathway to a clinically relevant event. Additionally, the treatment’s ability to alter the surrogate endpoint must be directly related to its impact on the true endpoint. It is important that the validity and reliability of a surrogate endpoint be established with appropriate analyses and not simply presupposed (28,29). Surrogate endpoints are sometimes justified on the basis of an analysis that demonstrates a statistical correlation between the surrogate event and a true endpoint. However, while such a correlation is a necessary condition, it is not a sufficient condition to justify using a particular surrogate as an endpoint in a clinical trial. For example, CA-125 levels following 3 cycles of treatment of ovarian cancer have been shown to be associated with the subsequent overall survival (30). Women with newly diagnosed ovarian cancer whose serum CA-125 levels normalize at the end of 3 cycles of standard treatment tend to survive longer than those who have abnormal levels. However, it has not (yet) been demonstrated that the degree to which any particular treatment reduces CA-125 levels reliably predicts its effects on clinically relevant endpoints, like overall survival.
The most frequently cited reasons for incorporating surrogate endpoints into a study include reduction in study size and duration, decreased expense, and convenience.
Primary Endpoints in Gynecologic Oncology Treatment Trials
The Food and Drug Administration (FDA) organized a conference to consider endpoints for trials involving women diagnosed with advanced ovarian cancer (31). Meta-analyses were presented, which indicate that PFS can be considered a valid endpoint for trials involving women with advanced ovarian cancer. It is important to recognize that while the general validity of PFS for predicting overall survival in this patient population has been established, PFS comparisons in a particular study can be biased. One source of bias arises from using different disease assessment schedules for each treatment group either intentionally or unintentionally. Delaying assessments for 1 treatment group artificially increases the apparent time to progression. Survival time is generally not susceptible to this source of bias.
Progression-free interval (PFI) may be a reasonable endpoint in trials involving patients with early or locally advanced cancer. PFI should be distinguished from PFS. The difference resides in how patients, who die without any evidence of disease progression are handled in the analysis. Patients who die without evidence of progression are censored at the time of their death in a PFI analysis, but considered an uncensored event in a PFS analysis. If deaths due to non-cancer-related causes are common, then selecting PFI as the study endpoint will generally increase the study’s sensitivity for detecting active treatments. In this case, however, the analysis needs to consider procedures that will account for treatment-related deaths, which may occur prior to disease recurrence. Simply censoring the time to recurrence in these cases can make very toxic treatments appear more effective than they actually are.
There have been a number of studies that provided evidence that a new treatment increases the duration of PFS, but not overall survival. For example, OVAR 2.2, a second-line treatment trial involving patients with platinum-sensitive ovarian cancer, indicated that carboplatin and gemcitabine significantly decreased the hazard of first progression or death (PFS) 28% (hazard ratio = 0.72, p = 0.003) when compared to carboplatin alone (32). However, there was no appreciable difference between the treatment groups with regard to the duration of overall survival (hazard ratio = 0.96, p = 0.735). Also, 3 trials evaluating maintenance bevacizumab for first- and second-line treatment of ovarian cancer reported significant prolongation in the duration of PFS (33–35), but no difference in overall survival. Therefore, while PFS may be a good surrogate for overall survival, it appears to be susceptible to a small but not insignificant chance of false-positive prediction. It is noteworthy, however, that results from PFS seldom lead to false-negative predictions. In other words, it is very rare for the results of an oncology trial to indicate that a new treatment prolongs overall survival but does not delay the onset of recurrence or progression. It seems reasonable to expect that an anticancer treatment that prolongs survival should exert its influence by delaying the onset of new or increasing disease. This has prompted some investigators to recommend using both PFS and overall survival as trial endpoints (36–38). Specifically, trials involving patients with advanced-stage cancer are designed to assess overall survival in the final analysis, but PFS is monitored at scheduled interim analyses. If the trial’s evolving evidence indicates that there is insufficient PFS benefit, then the trial may be stopped early and concluded that the treatment has insufficient activity to warrant further investigation in the target population. This procedure tends to halt trials of inactive treatments early, but continues trials of active agents to completion.
Response (disease status) assessed via reassessment laparotomy following treatment has been proposed for use as a study endpoint in ovarian cancer trials (39). The justification is that patients with no pathologic evidence of disease are more likely to experience longer survival than those with evidence of disease. The principal drawback to this endpoint is that reassessment laparotomy is a very onerous procedure for the patient, and many patients refuse reassessment surgery, or the surgery may become medically contraindicated. Even among highly motivated and very persuasive investigators the percent of patients not reassessed is typically greater than 15%. These missing evaluations can significantly undermine the interpretability of a study.
To date, PFS has not been formally validated for use in trials involving patients with advanced cervical, endometrial, or vulvar cancers. In the absence of a formally validated endpoint, overall survival or symptom relief are reasonable endpoints. Since relief from symptoms is susceptible to assessment bias, trials utilizing this endpoint should use validated instruments (See Measurement Errors) and consider blinding the study treatments whenever possible.
The endpoint selection for trials involving patients with recurrent cervical cancer has been controversial. Historically, some trials have been designed with clinical response as the primary endpoint. However, survival is a preferable primary endpoint. The rationale for this choice arises from the observation that treatments that have demonstrated an improvement in the frequency of response have not consistently prolonged survival (40–42). While subsequent therapies may have distorted the cause-effect relationship, the effect is probably at best minimal since there are no known treatments that have consistently demonstrated an ability to influence the survival time of patients with advanced or recurrent cervical cancer.
In summary, the ideal endpoint provides reasonable assurance that inference about the causal relationship between the intervention and the endpoint is valid. It is either itself clinically relevant to the patient, or predictive of a clinically relevant outcome. The ideal endpoint can be measured reliably. It should be convenient and cost-effective to measure. Unfortunately, in some trials, these features are not always available simultaneously. If a surrogate endpoint is used, then its validity should be established, not presumed.
Eligibility Criteria
The eligibility criteria serve 2 purposes in a clinical trial. The immediate purpose is to define those patients with a particular disease, clinical history, and personal and medical characteristics that may be considered for enrollment in the clinical trial. The subsequent purpose of eligibility criteria comes after the clinical trial is completed and the results are available. Physicians must then decide whether the trial results are applicable to their particular patient. A physician may consider the trial results to be applicable when the patient meets the eligibility criteria of the published study. Unfortunately, this principle is problematic. The necessary sampling procedure for selecting patients for the study (i.e., a random sample of all eligible patients) is never actually applied in clinical trials. Therefore, extrapolation of study results becomes an inherent part of medical practice.
Within the general population, there is a target population that includes those patients for whom the results of the trial are intended to apply (e.g., advanced endometrial cancer with no prior systemic cytotoxic therapies). While an investigator can typically specify an idealized definition for the target population, additional practical issues also frequently need to be considered. For example, there are varying opinions about whether patients diagnosed with an adenocarcinoma of the fallopian tube or primary peritoneal cancer in studies which target the treatment of patients with adenocarcinomas of the ovary. Some clinical investigators will argue for the need to study a “pure” study population and ignore the fact that their treatment decisions for patients with cancers originating in the fallopian tube or peritoneum frequently come from the results of trials involving patients with advanced ovarian cancer. Ideally, the size of a subgroup represented in a study should be in proportion to their presence in the target population. The degree to which a study sample reflects the target population determines the generalizability or external validity of the study results. Eligibility criteria should reflect a concern for the generalizability of the trial results. When clinicians frequently resort to extrapolating the results of a trial to patient groups that were not eligible for the trial, this may be considered a serious indictment of the study’s eligibility criteria.
The ideal situation is when every patient who meets the eligibility criteria of a clinical trial is asked to participate. This is seldom possible since not all physicians, who treat such patients, participate in the study. Also, not all patients wish to be involved in a clinical trial. Impediments in traveling to a participating treatment center further reduce the target population. The source population is the subset of patients in the target population who have access to the study. Figure 17.1 displays common restrictions that can limit the entry of patients to a clinical trial.
Restricted access to the study may contribute a biased sample from the target population, referred to as selection bias. For example, participating investigators at university hospitals might tend to enroll disproportionately more patients with cancer who have undergone very aggressive initial cytoreductive surgeries than their counterparts at community hospitals.
A potentially useful approach for determining the necessity of a particular eligibility criterion is to clearly identify its function. In addition to defining the target population, there are 4 distinct functions that an eligibility criterion may serve: benefit-morbidity equipoise (safety), homogeneity of benefit (scientific), logistic, and regulatory (43).
Eligibility criteria for safety are frequently imposed to eliminate patients for whom the risk of adverse effects from treatment is not commensurate with the potential for benefit. This concern can manifest in two ways. First, in oncology trials, there is often some concern that study treatments may be too toxic for patients with compromised bone-marrow reserves or kidney function. These patients are frequently excluded from trials when the potential benefits of treatment are not consistent with the risk associated with treatment. Second, even otherwise healthy cancer patients may be eliminated from the study when the risks from treatment are considered too great. For example, chemoradiation after hysterectomy is normally considered excessive treatment for a patient with Stage IA cervical cancer, since even without this treatment the risk of relapse is relatively small, but the morbidity of this combined modality following hysterectomy may be substantial. Therefore, eligibility criteria that eliminate these patients can be justified.
Eligibility criteria may be warranted when there is a scientific or biologic rationale for a variation in treatment benefit across patient subgroups. There is no scientific reason to expect that the effect of treatment is entirely the same across all subgroups of patients included in the study. The effect of a new therapy may be expected to have such dramatic inconsistencies across the entire spectrum of the target population that statistical power is compromised (43). One example of this type of exclusion criterion is found in GOG Protocol 152 (A Phase 3 Randomized Study of Cisplatin [NSC#119875] and Taxol [Paclitaxel] [NSC#125973] with Interval Secondary Cytoreduction versus Cisplatin and Paclitaxel in Patients with Suboptimal Stage III & IV Epithelial Ovarian Carcinoma). This study was designed to assess the value of secondary cytoreductive surgery in patients with Stage III ovarian cancer. All patients who entered into this study were to receive 3 courses of cisplatin and paclitaxel. After completing this therapy they were then randomized to either 3 additional courses of chemotherapy or interval secondary cytoreductive surgery followed by 3 additional courses of chemotherapy. The eligibility criteria exclude those patients who had only microscopic residual disease following their primary cytoreductive surgery since there is no scientific reason to expect that interval cytoreduction would be of any value to patients with no gross residual disease.
FIGURE 17.1. Sequential reduction of patient population.
Clinical investigators frequently implement eligibility criteria in order to promote homogeneity in patient prognoses. The desire to attain a study population with homogeneous prognoses is a common reason for excessive eligibility criteria. However, the concept is both unattainable and overemphasized. This notion may arise from an investigator’s attempt to duplicate the experimental method conducted in the laboratory. It is standard practice in laboratory experiments on animals to use inbred strains in an effort to control genetic variability. In large-scale clinical trials, this approach seldom has merit in light of the cost to generalizability. Eligibility criteria should be as broad as possible in order to enhance generalizability. For example, GOG Protocol 165 (A Randomized Comparison of Radiation Plus Weekly Cisplatin versus Radiation plus PVI [Protracted Venous Infusion] 5-FU in Patients with Stage II-B, III-B and IV-A Carcinoma of the Cervix) includes patients with no surgical sampling of the paraaortic lymph nodes. Previous GOG trials required sampling of these nodes. However, there is no evidence or sound biological justification to support the notion that the relative treatment benefit depends on the extent of surgical staging. This is not to say whether or not surgical staging is itself beneficial.
Eligibility criteria can be justified on the basis of logistic considerations. For example, a study that requires frequent clinic visits for proper evaluation or toxicity monitoring may restrict patients who are unable to arrange reliable transportation. The potential problem with such a restriction is how it is structured. A criterion requiring that the patient have a car at her disposal is probably too restrictive in a trial of women with advanced cervical cancer, since patients from this target population tend toward poorer socio-economic status (SES) and may not have access to private transportation. Such a restriction erodes the generalizability of the trial by over sampling patients with higher SES and may prolong study accrual. Moreover, complicated eligibility criteria are more likely to function ineffectively. In general complicated eligibility restrictions should be avoided.
Eligibility criteria based on regulatory considerations include institutional and governmental regulations that require a signed and witnessed informed consent and study approval by the local Institutional Review Board. These restrictions are required in most research settings and are not subject to the investigator’s discretion.
Currently many biostatisticians believe that eligibility criteria in oncology trials tend to be too restrictive and complicated (43,44). Overly restrictive or complex eligibility criteria hamper accrual, prolong the study’s duration, and delay the reporting of results. The Medical Research Council has demonstrated that it is possible to conduct trials with simple and few eligibility criteria (45). The ICON3 (International Collaborative Ovarian Neoplasms) trial compares standard carboplatin or cisplatin adriamycin cyclophosphamide regimen with paclitaxel plus carboplatin in women with newly diagnosed ovarian cancer. This trial has 6 eligibility criteria, 3 of which are for safety: (a) fit to receive, and no clear contraindication to, chemotherapy, (b) Absence of sepsis, and (c) bilirubin less than twice the normal level for the center. This is in sharp contrast to GOG Protocol 162 (A Phase 3 Randomized Trial of Cisplatin [NSC #119875] with Paclitaxel [NSC #125973] Administered by Either 24-Hour Infusion or 96-Hour Infusion in Patients with Selected Stage III and Stage IV Epithelial Ovarian Cancer), which compares 2 different paclitaxel infusion durations in patients with advanced ovarian cancer and has 34 eligibility criteria.
Phases of Drug Development trials
The traditional approach to identifying and evaluating new drugs has relied on sequential evidence from Phase 1, 2, and 3 clinical trials. Each of these study designs stems from very distinct study objectives. Phase 2 trials build on the evidence gathered from Phase 1 trial results, and similarly Phase 3 trials build on Phase 2 and Phase 1 trial results. The investigation of a given treatment may be halted at any phase, either due to safety and/or efficacy issues. Depending upon the underlying investigation, the time from the initiation of a Phase 1 trial for a given treatment to the completion of a Phase 3 trial often spans several years.
Phase 1 Trials
The purpose of a Phase 1 therapeutic trial in cancer-related research is to determine an acceptable dose or schedule for a new therapy as determined by toxicity and/or pharmacokinetics. A Phase 1 trial marks the first use of a new experimental agent in humans. Most Phase 1 trials escalate the dose or schedule of the new agent after either a pre-specified number of consecutive patients has been successfully treated or within an individual as each dose is determined to be tolerable. The usual Phase 1 trial of a cytotoxic agent attempts to balance the delivery of the greatest dose intensity against an acceptable risk of dose limiting-toxicity (DLT). The conventional approach increases the dose after demonstrating that a small cohort of consecutive patients (3 to 6) is able to tolerate the regimen. However, once an unacceptable level of toxicity occurs (e.g., two or 3 patients experiencing DLT), the previously acceptable dose level is used to treat a few additional patients in order to provide additional evidence that the current dose has an acceptably low risk of DLT. If this dose is still regarded as acceptable, it becomes the dose and schedule studied in subsequent trials and it is referred to as the recommended dose level (RDL). The RDL should not be confused with the maximum tolerated dose (MTD). The MTD is a theoretical concept used to design a Phase 1 trials, while the RDL is an estimate of the MTD, which may or may not be accurate. Due to the limited number of patients involved in a Phase 1 trials outcome measures such as response and survival are not the primary interest in these studies. When these outcomes are reported, they are considered anecdotal evidence of treatment activity. Eligibility criteria for Phase 1 trials in oncology often limit accrual to patients in whom conventional treatments have failed.
Alternative strategies for estimating the MTD have been proposed. The primary motivation for these newer strategies is to reduce the number of patients treated at therapeutically inferior doses and to reduce the overall size of the study. One of these alternatives implements a Bayesian approach and is referred to as the continual reassessment method (CRM) (46). It has the attractive feature of determining the dose level for the next patient based on statistically modeling the toxicity experience of the previously treated patients. While the traditional approach has been criticized for treating too many patients at subtherapeutic doses and providing unreliable estimates of the MTD, CRM has been criticized for tending to treat too many patients at doses higher than the MTD (47). Refinements to CRM have been proposed (48) and found to have good properties when compared to alternative dose-seeking strategies (49). Another family of designs termed the accelerated titration design (ATD) allows doses to be escalated within each patient and incorporates toxicity or pharmacologic information from each course of therapy into the decision of whether to further escalate or not (50). Both the modified CRM and ATD designs can provide significant advantages over the conventional Phase 1 design.
Special Phase I study designs are often recommended for targeted agents. These studies will often incorporate a biomarker endpoint that signals either the activation or deactivation of a targeted pathway. As the dose is increased for small cohorts of individuals, the biomarker is measured and toxicity is monitored simultaneously. Then, statistical models are used to determine a safe dose where the targeted pathway is consistently activated or inhibited (51).
Even though the majority of Phase 1 trials in cancer research follow what was described above, it should be mentioned that alternative Phase 1 trials may arise in other settings such as medical device trials, prevention trials, education intervention trials, and behavior modification trials, where the first phase of investigation may actually utilize healthy subjects, such as studies interested in determining the utility of a new educational intervention on smoking cessation. The Phase 1 trial may simply be utilized as an approach towards gaining some experience with the intervention prior to moving forward to the next phase of investigation.
Phase 2 Trials
Once a dose and schedule for a new regimen has been deemed acceptable, a reasonable next step is to seek evidence that the new regimen is worthy of further evaluation in a particular patient population. The principal goal of a Phase 2 trial is to prospectively quantify the potential efficacy of the new therapy. Since a Phase 2 trial treats more patients at the RDL than a Phase 1 trial, it also provides an opportunity to more reliably assess toxicities. A Phase 2 trial is often referred to as a drug-screening trial because it attempts to judiciously identify active agents worthy of further study in much the same way a clinician screens a patient for further evaluation. The study should have adequate sensitivity to detect active treatments and adequate specificity for rejecting inactive treatments. A Phase 2 trial may evaluate a single new regimen, or incorporate randomization to evaluate several new therapies or treatment schedules simultaneously.
There are several concerns that need to be considered when weighing the decision to conduct a single-arm versus a multiarm randomized Phase 2 study. While the required sample size is smaller for the single-arm trial, this design is susceptible to several sources of biases (52). Single-arm trials often assume that the probability of response to standard treatment in the target population is known with certainty. However, this assumption is often suspect. There are often unanticipated or unknown differences between the study sample and the historical sample which can introduce significant biases into the comparisons. For instance, there may be differences in the patients’ prognoses unintentionally introduced by the study’s eligibility criteria or changes in patient referral patterns. Differences in the response assessment schedule, the modalities for evaluation, or the definition of response may change over time and therefore distort comparisons with historical results. Generally, randomized controlled Phase 2 studies are preferred over single-arm studies. However, when the probability of response to standard treatment is widely accepted to be very low (e.g., <5%), it may be reasonable to conduct a single-arm studies. Investigators who conduct Phase 2 studies in rare diseases often argue in favor of single-arm designs; however, these are often the cases where the historical data is usually least understood and most susceptible to the previously mentioned biases.
Phase 2 trials can have a single-stage or multistage design. In a single-stage design, a fixed number of patients is treated with the new therapy. The goal of the single-stage design is to achieve a predetermined level of precision in estimating the endpoint. While precision is one important goal in cancer trials, there is also a concern for reducing the number of patients treated with inferior regimens. For this reason many Phase 2 cancer trials use multistage designs. Multistage designs implement planned interim analyses of the data and apply predetermined rules to assess whether there is sufficient evidence to warrant continuing the trial. These rules, which are established prior to initiating the study, tend to terminate those trials with regimens having less than the desired activity, and tend to continue to full accrual of those trials with regimens having at least a minimally acceptable level of activity. Two-stage designs that minimize the expected sample size when the new treatment has a clinically uninteresting level of activity have been proposed for single-arm studies (53) and multiarm studies (54). In the cooperative group setting, there is often a need for flexibility in specifying exactly when the interim analysis will occur. This is due to the significant administrative and logistic overhead of coordinating the study in several clinics. Therefore, designs that do not require that the interim analyses occur after a precise number of patients is entered, are useful in the multi-institutional setting (55).
Regardless of the measure of treatment efficacy, most designs treat toxicity as merely a secondary observation. This approach is not likely to be appropriate in Phase 2 trials of very aggressive treatments, such as high-dose chemotherapy with bone-marrow support. In these trials, stopping rules that explicitly consider both response and the cumulative incidence of certain toxicities may be more appropriate (56–58). Bayesian designs, which permit continuous monitoring of both toxicity and response, have also been proposed (59).
Phase 3 Trials
The goal of a Phase 3 trial is to prospectively and definitively determine the effects of a new therapy relative to a standard therapy in a well-defined patient population. Phase 3 trials are also used to determine an acceptable standard therapy when there is no prior consensus on the appropriate standard therapy. Some Phase 3 trial methodologies such as randomization and blocking have origins in comparative agricultural experiments. However, in clinical trials the experimental unit is a human being and consequently, there are 2 very important distinctions. First, each individual must be informed of the potential benefits as well as the risks and must freely consent to participate before enrollment in the study. Second, an investigator has very limited control over the patient’s environment during the observation period. This later distinction can have a profound statistical implication, which will be discussed later.
Phase 3 Trials with Historical Controls
The strict definition of a Phase 3 trial does not necessitate concurrent controls (i.e., prospectively enrolled patients assigned the standard treatment) or randomization (i.e., random treatment allocation). However, these 2 features are almost synonymous with Phase 3 trials today. The principal drawback from inferring treatment differences from a historically controlled trial is that the treatment groups may differ in a variety of characteristics that are not apparent. Differences in outcome, which are in fact due to differences in characteristics between the groups, may be erroneously attributed to the treatment. While statistical models are often used to adjust for some potential biases, adjustments are possible only for factors that have been recorded accurately and consistently from both samples. Shifts in medical practice over time, differences in the definition of the disease, eligibility criteria, follow-up procedures or recording methods can all contribute to a differential bias. Unlike random error, this type of error cannot be reduced by increasing the sample size. Moreover, the undesirable consequences of moderate biases may be exacerbated with larger sample sizes. When a trial includes concurrent controls, the definition of disease and the eligibility criteria can be applied consistently to both treatment groups. Also, the standard procedures for measuring the endpoint can be uniformly applied to all patients. Inclusion of prospectively treated controls within the clinical trial requires a method of assigning the treatments to patients. Randomization and its benefits are discussed in a later section.
It is useful to distinguish Phase 3 objectives as having an efficacy, equivalency, or noninferiority design consideration. An efficacy design is characterized by the search for an intervention strategy that provides a therapeutic advantage over the current standard of care. An equivalency trial seeks to demonstrate that 2 interventions can be considered sufficiently similar on the basis of outcome that 1 can be reasonably substituted for the other. Noninferiority trials seek to identify new treatments that reduce toxicity, patient inconvenience, or treatment costs without significantly compromising efficacy.
Efficacy trials. Efficacy designs are very common in oncology trials. Examples include: trials that assess the benefit of adding chemotherapy to radiation for the treatment of early-stage cervical cancer or trials that compare standard versus dose intense platinum regimens for treating ovarian cancer. In each of these cases, the trial seeks to augment the standard of care in order to attain a better treatment response. From the outset of these trials it is recognized that the treatment benefit may be accompanied by an increased risk of toxicity, inconvenience, or financial cost. However, it is hoped that the benefits will be sufficiently large to offset these drawbacks. Suppose treatment A is the standard of care for a particular target disease population. The quantitative difference between treatments with respect to a particular outcome (B–A) can be described on a horizontal axis as in Figure 17.2. If we are reasonably certain that the difference between treatments is less than 0 (left of 0) then we would consider treatment A to be better. On the other hand, if the treatment difference is greater than 0 (right of 0) then we would conclude that the new treatment, B, is better. Furthermore, we can use dotted lines to demarcate on this graphic a region in which the difference between A and B is small enough to warrant no clinical preference for A or B. Consider the results from a trial expressed as the estimated difference between treatments and the corresponding 95% or 99% confidence interval superimposed on this graph. The confidence interval depicts those values of the treatment difference that can be reasonably considered consistent with the data from the trial. An inconclusive trial is characterized by having such broad confidence intervals that the data cannot distinguish between A being preferred or B being preferred (see Figure 17.2). This is a typical consequence of a small trial. On the other hand, if the confidence interval entirely excludes the region where A is better than B, then we can conclude that B is significantly better than A (see Fig. 17.2). Note that in this case the lower bound of the confidence interval may extend into the region of clinical indifference, but the confidence interval must exclude the region below (left of) 0 difference.
Equivalency trials. The equivalency study design is perhaps a misnomer, since it is actually impossible to generate enough data from any trial to definitively claim that the 2 treatments are equivalent. Instead, an investigator typically defines the limits for treatment differences that can be interpreted as clinically irrelevant. If it is a matter of opinion for what differences in effect sizes can be considered clinically irrelevant, this issue can become a major source for controversy in the final interpretation of the trial results. Survival or progression-free survival endpoints are seldom used in equivalency trials; however, bio-equivalency designs are sometimes used for drug development. For example, if 1 agent is known to influence a particular biologic marker, then it may be desirable to design a trial to determine whether a new agent is as effective at modifying the expression of this biomarker. In this case, an investigator has some notion about the acceptable range of activity that can be considered clinically biologically equivalent. These studies are designed so that, within tolerable limits, the treatments can be considered equivalent.
Notice that the results from the inconclusive trial in Figure 17.2 cannot be interpreted as demonstrating equivalency. Even though the estimated difference between the treatments is nearly 0, the confidence interval cannot rule out treatment differences that would lead to preferring A or B. Caution should be exercised in interpreting the results from studies that conclude “therapeutic equivalency” when only a small difference between treatments with regard to the outcome is observed and it is declared to be not statistically significant. Even results from moderately large trials, which suggest therapeutic equivalence, may in fact be due to inadequate statistical power to detect clinically relevant differences.
FIGURE 17.2. Graphical representation of the point estimates and confidence intervals describing the results from 4 hypothetical trials.
Noninferiority trials. A noninferiority study design may be considered when the currently accepted standard treatment is associated with significant toxicity and a new and less toxic treatment becomes available. The goal of this type of study is to demonstrate that substituting the new treatment for the current standard treatment does not compromise efficacy appreciably (60–63). Referring to Figure 17.2, the trial seeks to provide sufficient evidence to be reasonably certain that the difference between A and B lies above the lower boundary of the indifference region. This lower boundary is often called the “noninferiority margin.”
The justification for the noninferiority margin selected in a particular study is often controversial. If this margin is set too low, then the study has an unacceptably high probability of recommending an inferior treatment. If it is too high, then the trial utilizes too many clinical and financial resources. In order to select an appropriate margin of noninferiority, it is important to recognize that even though a noninferiority trial may explicitly compare only 2 treatments, implicitly there is a third treatment to be considered. For example, suppose that several historical studies indicate that treatment A is better than a placebo for treating a specific disease. In this case, the goal of a noninferiority study is to demonstrate that a new experimental treatment, B, does not significantly compromise efficacy when compared to currently accepted active standard treatment, A. However, it should also demonstrate that B would have been better than a placebo, if it had been included in the current trial. In other words, the current trial will directly estimate the effectiveness of B relative to A, but it must also indirectly consider the effectiveness of B relative to the previous control treatment (placebo in this case). This indirect comparison relies on obtaining a reliable and unbiased estimate of the effectiveness of the current active control to the previous control from previous trials. Sometimes the margin of noninferiority is expressed as a proportion of the effectiveness of A relative to the previous standard treatment. For example, a noninferiority study could be designed to have a high probability of concluding that a new treatment retains at least 50% of the activity of the standard regimen, A. Note that an investigator may decide that she is unwilling to give up any of the benefit attributed to the current standard treatment. In this case, the margin of noninferiority is set at 0 (see Fig. 17.2) and the design is the same as the efficacy trial. Indeed an efficacy trial can be considered a study in which the investigator is willing to accept the new treatment B, only if the trial results indicate that B is superior to A.
Obtaining reliable estimates for the activity of the currently accepted active standard treatment can be a very troublesome aspect of noninferiority oncology trials. For example, cisplatin 75 mg/m2 and paclitaxel 135 mg/m2 infused over 24 hours was the first platinum-taxane combination to demonstrate activity in the treatment of advanced ovarian cancer (64). Subsequently, several trials were conducted to assess whether carboplatinum could be safely substituted for cisplatinum (65–67) or whether taxotere could be substituted for paclitaxel (68). However, there has been some controversy about the size of the benefit provided by paclitaxel (69). An investigator can reasonably ask, “What is the effect size of paclitaxel, and how much of this effect can I reasonably be certain is preserved by taxotere?”
Randomized Phase 3 Trials
There are several design features that may be considered for Phase 3 trials. Some are more pertinent than others to gynecologic oncology trials. The most important feature to be considered is treatment randomization. A study with this feature, a randomized clinical trial (RCT), has several scientific advantages. First, both the known and unknown prognostic factors tend to be distributed similarly across the treatment groups when a trial implements randomized treatments. Second, a potential source of differential selection bias is eliminated. This bias could occur when there is an association between treatment choice and prognosis. It need not be intentional. When a physician’s interest in a trial or a patient’s decision to participate in the trial depends on the assigned treatment, a nonrandom association between treatment and prognosis can be introduced. Finally, randomization provides the theoretical underpinning for the significance test (70). In other words, the probability of a false-positive trial as stated in the study design is justified with randomization. It is important to recognize that these advantages, which are provided by randomizing the study treatments, are forfeited when all of the randomized patients are not included in the final analyses.
It is sometimes argued that since many factors that influence prognosis are known, perhaps other approaches to allocating treatments can be considered, and statistical models should be used to adjust for any imbalances in prognosis. However, the conclusions from this type of trial must be conditioned on the completeness of knowledge about the disease and the acceptability of the modeling assumptions. If the disease is moderately unpredictable with regard to the outcome, or the statistical model is inappropriate, then the conclusions are suspect. Results from nonrandomized studies can polarize the medical community. They frequently provide enough evidence for those who already support the conclusions but insufficient evidence for those who are skeptical.
Kunz and Oxman (71) have compared the results from overviews of randomized and nonrandomized clinical trials that evaluated the same intervention. They reported that the nonrandomized studies tended to overestimate the treatment effect from the randomized trials by 76% to 160%. Schulz et al. (72) compared 33 randomized controlled trials that had inadequate concealment of the random treatment assignments to studies that had adequate concealment. They found that studies with inadequate concealment tended to overestimate the treatment effect (relative odds) by 40%. Some investigators do not appreciate the importance of concealment and will go to considerable lengths to subvert it (73). When the randomization technique requires pregenerated random treatment assignments, one must guarantee that the investigators, who are enrolling patients, do not have access to the assignment lists.
The patient–physician relationship can occasionally be challenged by introducing the concept of treatment randomization (74). Patients may prefer a sense of confidence from their physician regarding the “best” therapy for them. However, physicians involved in a RCT must honestly acknowledge that the best therapy is unknown and that a RCT is preferred to continued ignorance. One survey of 600 women seen in a breast clinic suggests that 90% of women prefer their doctor to admit uncertainty about the best treatment option, rather than give them false hope (75).
Randomization techniques. The simplest approach to randomization is to assign treatments based on a coin flip, sequential digits from random number tables, or computerized pseudo-random number generators. On average, each patient has a defined probability of being allocated to a particular study treatment, when he or she enters the study. While this approach is simple, the statistical efficiency of the analyses can be enhanced by constraining the randomization so that each treatment is allocated an equal number of times. Permuted block randomization is sometimes used to promote equal treatment-group sizes. A block can be created by shuffling a fixed number of cards for each treatment and then assigning the patients according to the random order of the deck. After completing each block there are an equal number of patients assigned to the treatment groups. For example, consider a trial comparing treatments A and B. There are 6 possible ways the block can be ordered when the block size is 4: AABB, ABBA, BBAA, BABA, ABAB, and BAAB. A sufficient number of assignments for a RCT can be created by randomly selecting a series of blocks from the 6 distinct possibilities. There are 3 features of blocked randomization that may be problematic. First, the probability of a particular treatment being allocated is not the same throughout the study, as in simple randomization. Taking the example above, every 4th treatment is predetermined by the previous 3 allocations. Second, the use of small blocks in a single-clinic study may undermine concealment and allow an investigator to deduce the next treatment. This potential problem can be corrected by continually changing the block size throughout the assignment list. Third, large block sizes can subvert the benefits of blocking. As block sizes increase, the procedure resembles simple randomization.
The statistical efficiency of the study can be further enhanced by stratifying patients into groups with similar prognoses and using separate lists of blocked treatments for each stratum. This procedure is called stratified block randomization. It is worth noting that using simple randomization within strata would defeat the purpose of stratification, since this is equivalent to using simple randomization for all patients. Likewise, trials that stratify on too many prognostic factors are likely to have many uncompleted treatment blocks at the end of the study, which also defeats the intent of blocking (76).
When it is desirable to balance on more than a few prognostic factors, an alternative is dynamic treatment allocation; one particular type being minimization. Whereas stratified block randomization will balance treatment assignments within each combination of the various factor levels, minimization tends to balance treatments within each level of the factor separately. Each time a new patient is entered into the study, the number of individuals who share any of the prognostic characteristics of the new patient is tabulated. A metric, which measures the imbalance of these factors among the study treatments, is computed as if the new patient were allocated to each of the study treatments in turn. The patient is then allocated to the treatment that would favor the greatest degree of balance. In the event that the procedure indicates equal preference for two or more possible treatment allocations, simple randomization can be used to determine the patient’s treatment assignment.
Masking Treatments
Concealment Concealment refers to the procedure in which the assigned study treatment is not revealed to the patient or the investigator until after the subject has successfully enrolled in the study. The purpose of concealment is to eliminate a bias which can arise from an individual’s decision to participate in the study depending on the treatment assignment (77). Concealment is an essential component of randomized clinical trials.
Blinding Blinding is a procedure that prevents the patient or physician from knowing which treatment is being used. In a single-blinded study the patient is unaware of which study treatments she is receiving. A double-blinded study results in a situation in which neither the patient nor the health care provider is aware of that information. One purpose of blinding is to avoid measurement bias, particularly differential measurement bias (see Endpoints section). This type of bias occurs when the value of a measurement is influenced by the knowledge of which treatment is being received (see Historical Perspective section). It can occur when the measurement of an endpoint is in part or totally subjective. Most methods for assessing pain are subjective and require treatment blinding in order to promote the study’s validity.
Oncology trials frequently do not implement blinding for several reasons. It is rather difficult to blind treatments when various treatment modalities are used (e.g., surgery versus radiation therapy, or intravenous versus oral administrations), when good medical practice is jeopardized (e.g., special tests are required to monitor toxicity due to particular treatments), or when it is logistically difficult (e.g., the evaluating physician must be kept isolated from the treatment of the patient). In the absence of blinding, care should be taken that the method of measuring the endpoint is precisely stated in the protocol and consistently applied to each patient uniformly. Trials that assess quality of life or relief from symptoms should give serious consideration to treatment blinding.
Schulz et al. (78) have reviewed 110 randomized clinical trials published between 1990 and 1991 from 4 journals dedicated to obstetrics and gynecology. Thirty-one of these trials reported being double-blinded. Schultz et al. conclude that blinding should have been used more often, despite frequent impediments. Moreover, blinding seemed to have been compromised in at least 3 of the trials where it was implemented.
Placebo A placebo is an inert treatment. Placebos blind the patient, and usually the physician as well, to the knowledge of whether an experimental or an inert treatment is being given. Placebos are frequently used in trials where there is no accepted standard treatment and the endpoint is susceptible to measurement bias. The use of a placebo is also important when the endpoint can be affected by the patient’s psychological response to the knowledge of receiving therapy combined with a belief that the therapy is effective. This phenomenon is aptly named the “placebo effect.” In such circumstances, the use of a placebo provides a treatment-to-control comparison that measures only the therapeutic effect. Note that the “placebo effect” is a distinctly different type of measurement bias from those that have been previously discussed. Careful ethical considerations must precede the use of a placebo or sham procedure in any clinical trial (79).
GOG Protocol 137 (A Randomized Double-Blinded Trial of Estrogen Replacement Therapy versus Placebo in Women with Stage I or II Endometrial Adenocarcinoma) was a randomized double-blinded comparison of estrogen replacement therapy versus a placebo in women who had a total abdominal hysterectomy and bilateral salpingo-oopherectomy for early-stage endometrial cancer. One primary reason for the use of a placebo in this trial was the potential for differential measurement bias being introduced by the physician monitoring patients on estrogens much more closely due to the anticipation of adverse events.
Factorial Designs
Factorial designs are used when several interventions are to be studied simultaneously. The term factorial arises from historical terminology in which the treatments were referred to as factors. Each factor has corresponding levels; for example, an investigator may wish to compare a study agent administered at 3 dose levels: high-dose, medium-dose, and none. The total number of factor combinations being studied is the product of the number of levels for each factor or treatment. For example, a trial that evaluates treatment A at three levels and treatment B at 2 levels is called a 3-by-2 (denoted 3 × 2) factorial design. If the relative effects due to the various levels of treatment A are independent of the levels of B, the two treatments (A and B) can be evaluated simultaneously. The factorial design provides a significant reduction in the required sample size when compared to a study that evaluates all levels of A and B separately. The key assumption necessary for a factorial design is that all treatments can be given simultaneously without interaction or interference.
The most commonly utilized factorial design is the 2 × 2 factorial design that includes 2 distinct treatment regimens at each of 2 factor levels. For example, suppose individuals entering a cancer prevention trial are randomly assigned to receive vitamin E and beta carotene while the respective factor levels are placebo-A or 50 mg/day for vitamin E, and placebo-B or 20 mg/day for beta carotene. There are 4 treatment combinations. In a standard 2 × 2 design the main effect of vitamin E can be ascertained by utilizing information from each of the 4 treatment groups. In some studies, however, the main effects may be of secondary importance compared to the “interaction” between each factor. An interaction exists when the effect due to 1 of the factors (i.e., treatment A) depends on the level of the other factor (treatment B). In drug discovery, a “positive” interaction may imply a synergistic effect of 2 drugs in combination, that is, the effect of the combination therapy is greater than the sum of the individual additive effects. Reliable tests of an interaction require a relatively large number of patients in each of the 4 treatment groups. If potential treatment interaction cannot reasonably be ruled out or there is interest in possible interactions, then attention to the statistical power of such tests is an important part of designing and interpreting the study results (80).
Hypothesis Test
A hypothesis is a conjecture based on prior experiences that leads to refutable predictions (81). A hypothesis is frequently framed in the context of either a null or an alternative hypothesis. A null hypothesis may postulate that a treatment does not influence patients’ outcomes. The alternative hypothesis is that a particular, well-defined treatment approach will influence the patients’ outcomes to a prespecified degree. These hypotheses cast the purpose of the trial into a clear framework. During the study design the investigators select a test statistic from an appropriate statistical procedure (e.g., an F-statistic from an analysis of variance, or a chi-square statistic from a logistic model) that evaluates the degree to which the study data support the null hypothesis. A Type I error is committed when the null hypothesis is in fact true, but the test statistic leads the investigator to incorrectly conclude it is false. Committing the Type I error would be disastrous if it means discontinuing the use of an active control treatment that is well tolerated and substituting an experimental therapy that is more toxic but, in reality, not better. A Type II error is committed when the null hypothesis is not true, but the test statistic leads the investigator to erroneously conclude that it is true. Type II errors commonly occur in studies that involve too few subjects to reliably estimate clinically important treatment effects. Prospectively specifying the null hypothesis, the appropriate statistical method for the analysis, the test statistic and quantifying the acceptable probabilities of Type I error (i.e., α-level) and Type II error are essential elements for determining the appropriate design and sample size for a particular trial.
p-Value
At times statisticians play the role of the conservative physician, cautiously prescribing a significance test only when it is appropriate. There is a general concern that the p-value is overused, even abused, and overemphasized. A common misconception is that the p-value is the probability that the null hypothesis is true. The null hypothesis is either true or false, and so it is not subject to a probability statement. It is the inference that an investigator makes, based on his or her data, that is susceptible to error. The p-value is simply the probability that the test statistic would be as extreme or even more extreme, if the null hypothesis was in fact true (see hypothesis test section).
Misconceptions about the p-value may arise in part from a poor distinction between the p-value and the α-level of a study (82). The α-level is the probability of the test statistic rejecting the null hypothesis when it is true. It is specified during the design phase of the study, and is unaltered by the results obtained. The p-value results from a statistical test performed on the observed data.
Translational Research
Many modern clinical trials often involve the systematic collection and banking of biologic materials from the participants. The materials may include serum, plasma, urine, buccal cells, or tumor tissue, which are collected and stored in a bank. The biologic materials together with the clinical data can then be used to understand how a biologic effect translates into the treatment effect on the patient population. This translation gives rise to the concept of translational research (TR). In translational research, discoveries in laboratory science are translated into practical applications. The flow of information from TR studies is a 2 way street: 1) more complete understanding of tumor biology and 2) more effective treatments for patients.
The goal in this section is to provide clinical and laboratory scientists with some fundamental information needed to design, implement, and analyze the data from TR studies. The following sections develop the concepts for: (a) biomarker discovery and verification, and (b) biomarker validation. In short, these steps are presented in Figure 17.3 (Discovery and Test Validation Stage). We provide insights into what goals and expectations a researcher can have when performing a biomarker study. With this knowledge, the reader can avoid some of the errors and biases that have plagued translational research and led to false, misreported, or misleading reports.
Biospecimen Collection
A widely used definition of a biomarker is “a characteristic that is objectively measured and evaluated as an indicator of normal biological processes, pathogenic processes, or pharmacologic responses to an intervention” (83). Biomarkers can be measurements of macromolecules (DNA, RNA, proteins, lipids), cells, or processes that describe a normal or abnormal biologic state in a patient. From the paradigm of translational research described above, biomarkers can inform investigators about a patient’s diagnosis, prognosis, prediction of response to therapy (i.e., effect modifier), or prediction of a clinical outcome (i.e., surrogate endpoint).
Generally speaking, biomarkers can be diagnostic or prognostic. A diagnostic biomarker is designed to identify the presence of a disease or other condition, while a prognostic biomarker is an indicator that helps researchers in predicting the course of a patient’s disease. Prognostic biomarkers are used to estimate the risk of a future clinical outcomes, and examples include stage of the disease, histology of the tumor, or patient’s performance status. A biomarker may also be deemed predictive, and as such, it can be used to modify the estimated risk of a future outcome, but only when a particular type of treatment is taken. In breast cancer patients, examples of predictive biomarkers are estrogen receptor status when the treatment is tamoxifen or Her2/neu status when the treatment is trastuzumab. The phrase “treatment selection marker” has become the preferred terminology for a predictive biomarker to avoid confusion with other uses of the word “predictive”. For our immediate purposes here we will view predictive markers as a special class of prognostic markers and primarily focus on prognostic biomarker studies. Only in the section describing studies involving targeted therapies will the distinction be made.
The biologic specimens used in a study are typically gathered either prospectively or retrospectively. In the prospective approach, patients are identified and then followed forward in time. There are several advantages to the prospective approach. First, patient enrollment can focus on enrolling only individuals from the intended target population. Second, the procedures for collecting and preparing specimens can be tailored to the intended laboratory procedures. Third, the quality control procedures for collecting the clinical data can target those data items that are required for the specific study objectives. Fourth, the patient’s treatment and follow-up assessments can be standardized and optimized in accordance with the goals of the study. When samples are retrospectively collected, the laboratory analysis is performed using previously archived specimens that may have been originally collected for another purpose. The investigator using a retrospective approach is a prisoner to the procedures that were set down before the current study goals were contemplated and therefore, they may not be optimal for his intended objectives. For instance, the samples may have come from patients who are not representative of the target population. This reduces the generalizability of the study results. The specimens may have been obtained, prepared or stored using outdated or less than optimal procedures for his laboratory tests. This could introduce biases in the measurements or reduce the power of the study.
FIGURE 17.3. Biomarker development for high-throughput technologies.
Biomarker Development Process
In the following sections, we discuss a common TR study design for a biomarker: (a) biomarker discovery and (b) biomarker validation. In short, these steps are presented in Figure 17.3 (Discovery and Test Validation Stage).
Discovery Phase
Regardless of whether the samples were retrospectively or prospectively collected, many studies are designed to discover biomarkers with clinical utility, from among many potentially useful biomarkers.
Since the invention of microarray technology and related high-throughput technologies, researchers have been able to compile large amounts of information and an enormous pool of potential biomarkers. These so called high-throughput platforms have become commonly used experimental platforms in the biologic realm (84). A high-throughput platform is designed to measure large numbers (thousands or millions) of signatures in a biologic organism at a given time point. These platforms are a function of the postgenomic era and are often used to determine how genomic expression is regulated or involved in biologic processes. The technologies in Table 17.1 use hybridization and sequence-based platforms such as gene expression microarrays and RNA-Seq to obtain data matrices.
Several of the common high-throughput assay platforms used in experiments designed for cancer diagnosis are listed in Table 17.1. These platforms were chosen to illustrate the diversity of platforms available for interrogating deoxyribonucleic acid (DNA), ribonucleic acid (RNA), or proteins.
Preprocessing High-Throughput (HT) Platforms
In short, pre-processing algorithms are required in nearly all high-throughput technologies listed in Table 17.1. This is due to the fact that HT platforms measure both biologic signal and technical signal. Therefore, the goal of preprocessing algorithms is removal of the technical signal. This technical signal can be considered in terms of background correction and normalization to adjust across experiments. The background corrections and normalization account for technical artifacts that can occur due to either the array construction or laboratory procedures that introduce systematic errors in the signal on individual arrays or across arrays. Often these pre-processing techniques are specific to the platform employed (see, for example, Miecznikowski et al. [85]) and therefore it is impractical to review all of the available pre-processing methods here.
Type I Error and Multiple Testing
The experiments performed in the HT discovery phase often have a goal of simply narrowing down the genome or proteome to a subset of potentially relevant biomarkers. In this sense, the scientists are performing a data reduction where the goal is to choose a subset of markers from the high-throughput scope that are related or associated with clinical outcome. The association with the outcome is assessed using a null hypothesis for each biomarker and summarizing “significance” with a p-value. In this case, the interpretation of each p-value differs slightly from a study with a single null hypothesis (See Hypothesis Test and p-value sections).
When the Type I error is limited to 5% for a single hypothesis, then there is only a 1-in-20 chance of rejecting that null hypothesis, if it is in fact true. This is a relatively uncommon occurrence. When there are 10,000 null hypotheses tested, as may be typical for some HT studies, even if all of the null hypotheses were true, one would expect 500 null hypotheses to be rejected at the 5% significance level. The chance of committing a Type I error over the entire study should no longer be considered an uncommon occurrence.
A Summary of Discovery Platforms, The Material Analyzed in the Platform and Recent Cancer Biomarker Discovery Studies Using the Given Platform |
Platforms | Material | Cancer Studies |
aCGH microarray | DNA | |
Gene expression microarray | mRNA | |
RNA—Seq | Transcriptomics | |
DNA methylation | DNA | (108) |
Mass spectrometry | Peptide/proteomics |
Therefore, during the discovery phase of a HT experiment the goal is to limit the Type I error rate. That is, HT studies are designed to limit the number of false positives, denoted by V in Table 17.2A. Table 17.2B lists some alternative approaches to controlling the Type I error rate during the discovery phase of the experiment. Statistically significant or interesting markers are determined from hypothesis testing in light of controlling one of the Type I error rates listed in Table 17.2B.
Confirmation and Verification
In order to have reasonable power in light of multiple testing, the Type I errors in Table 17.2A tend to be more liberal (more likely to reject null hypotheses) than standard scientific significance testing procedures. Thus, any markers from the discovery phase should be confirmed or verified using other methods, such as immunohisotchemical (IHC) assays or reverse transcription polymerase chain reaction (RT-PCR). These verification platforms could use either the same samples from the discovery phase or a new independent set of samples. The goal for this step is to confirm that the biomarker signal from the discovery platform is accurately measuring the expression of the desired gene, protein or RNA.
Including a confirmation step ensures researchers that their discovery markers can be confirmed using other platforms. This provides some reassurance in the ability of the markers to be confirmed on independent samples from possibly different institutions with, possibly, differing methods of assessment. Methods that describe how to correlate RT-PCR and IHC signals with their microarray counterparts can be found in Press et al. (115), van den Broek and van de Vijver (116), McShane et al. (117), Esteban et al. (118).
A summary of results from analyzing multiple hypothesis tests. |
M0 and M1 are considered as fixed (unknown) parameters representing the number of true nulls and the number of true alternatives, respectively. The random variables U and Q represent the number of the correct decisions, while the random variables T and V represent the number of incorrect decisions. V is the number of false positives.
In certain immunohistochemistry assays the results should be performed by at least 2 different observers who are blinded to the clinical data. This may alleviate the subjectivity in these experiments since IHC assays require selection of best regions to score and subjective measurements of staining intensity and percentage of stained cells.
Validation Phase
Various statistical models can be fit using the data in the discovery phase. At the end of the discovery phase there should be a complete specification of the marker assay method and model, and thorough documentation of the (final) lock-down model. The fully specified precise lock-down model will be evaluated using a validation dataset. Note, for example, this must include specifying all coefficients in the classification model and any of the rules for deciding the level of a biomarker.
Validation in biomarker experiments should always involve an independent dataset, that is, data from patients that were not included in any of the discovery dataset(s). Note that internal validation procedures such as cross-validation, boot strapping, or other data resampling methods are useful to give insights into issues such as bias and variance of regression parameter estimates and stability of the model derived. In these internal validation procedures, some portion of the data is held out (test set), while a model is built on the remaining portion (training set). A limitation of these internal procedures is that there may be biases that affect the training and test sets equally. For example, if the set of specimens for confirmation are collected in the same laboratory, processed by the same technician, and run on the same equipment, then peculiarities of that data (equipment, lab, technician) will be shared between the samples used to develop the computational model and the samples used to evaluate the model.
Summary of Type 1 Errors |
Abbreviation | Name | Quantity |
FWER | Family Wise Error Rate | Pr(V > 1) |
k-FWER | Generalized Family Wise Error Rate | Pr(V > k) |
FDR | False Discovery Rate | E[V/R] |
k-FDR | Generalized False Discovery Rate |
|
PCER | Per comparison error rate | E[V]/M |
TPPFP | Tail probabilities for the proportion of false positives | Pr(V/R) < q |
Summaries of Type I errors using random variables defined in Table 2a. See similar tables in Nichols and Hayasaka [2003] (Nichols and Hayasaka 2003) and Table 15.1 in Gentleman et al. [2005](Gentleman, Carey et al. 2005). Note that I() in the equation for k-FDR denotes the indicator function and max() denotes the maximum operator. Further note that q in TPPFP should be determined prior to testing. Pr() denotes probability, and E[ ] denotes expectation.
Even with the requirement of an independent dataset, there may still be levels of validation evidence. For example, a lower level of validation evidence may be independent sets of specimens and clinical data collected at a single institution using carefully controlled protocols, with samples from the same patient population. Meanwhile, a higher level of validation evidence would be independent sets of specimens and clinical data collected at multiple institutions
Additionally, it should be stressed that the independent validation dataset must be relevant to the intended use of the candidate biomarker test. Patients in the independent dataset should have the same type of disease, the same stage and same clinical setting for which the candidate test is intended to be used in the future. Ideally, the specimens for independent confirmation will have been collected at a different point in time, at different institutions, from a different patient population, with samples processed in a different laboratory to demonstrate that the test has broad applicability and is not overfit to any particular situation.
Publicly Available Data
These datasets can help with the development process, and further they can complete the puzzle in systems biology, for example, provide the proteomics story if you only have genomic data. They can be used in an integrative analysis and a meta-analysis. These meta-analyses can strengthen the conclusions drawn from an individual researcher’s study. They may also offer insights from other study populations.
As deoxyribonucleic acid (DNA) microarray technology has been widely applied to detect gene activity changes in many areas of biomedical research, development and curation of online microarray data repositories are at the forefront of research endeavors to use and reuse this mounting deluge of data. Several representative repositories of microarray datasets are ArrayExpress at the webpage http://www.ebi.ac.uk/arrayexpress/, Gene Expression Omnibus (GEO) (119) at the webpage http://www.ncbi.nlm.nih.gov/geo/, Stanford Microarray Database (SMD) at the webpage http://smd.stanford.edu/ and Center for Information Biology Gene Expression Database (CIBEX) (120) at the webpage http://cibex.nig.ac.jp/index.jsp. Also a new database called Anduril which has been recently developed and described in Ovaska et al. (121) now provides comprehensive storage of the massive data including single base pair mutation, copy number change or deletions of genes, differential gene expression, and epigenetic changes like methylation profiles. A somewhat recent comparison of the available microarray databases was provided in Gardiner-Garden and Littlejohn (122). Statistical packages in R have also been created that allows users to easily import data from databases like ArrayExpress into Bioconductor packages (123).
Concordant with the development of online data repositories, researchers have developed specific data standards required for microarray analysis. The data standard concept describes the minimum information about a microarray experiment (MIAME) that is needed to enable the interpretation of the results of the experiment and to potentially reproduce the experiment (124). MIAME compliance will ensure that biological properties of the samples and the phenotypes that were assayed were correctly recorded, thus, ensuring, that the data can be quickly assessed for its suitability in studying new questions. The public repositories including ArrayExpress (146), GEO (125), and CIBEX (120) are all designed to hold MIAME compliant microarray data.
Especially exciting for oncologists, multiple platforms of microarray data from The Cancer Genome Atlas (TCGA) are now available through the data portal at http://tcga-data.nci.nih.gov/tcga/tcgaHome2.jsp. The TCGA project is further described in Stratton et al. (126), but in short, represents one of the first large-scale attempts to study the multiple types of genetic mutations involved in multiple cancer types from different cohorts of patients. Initially, the pilot projects studied glioblastoma multiforma (GBM) and cystadenocarcinoma of the ovary, but have now expanded to cover roughly 20 different cancer types. For each cancer type, the patient cohorts (collected from different sites) include several hundred individuals and the platform techniques include gene expression profiling, copy number variation profiling, SNP genotyping, methylation profiling, and microRNA profiling. These data will be made publicly available, making the TCGA dataset a great resource for future research using meta-analysis and integrative analysis techniques that were not previously available.
Developing Translational Research Studies and Reporting Results
Guidelines have been developed to promote accurate and complete reporting of results from biomarker studies. Throughout this section, the importance of having well-defined questions for the proposed data is stressed. In other words, serious thought, planning, and discussions amongst a team of scientists are necessary to successfully perform biomarker analysis, including discovery, verification, and validation.
In the discovery phase it is important that the proposed technology has been validated, see for example, technical replication studies in Strand et al. (127), Callesen et al. (128); Leyland-Jones et al. (129), De Cecco et al. (130), Hicks et al. (131), Benton et al. (132), Freidin et al. (133), and Lawrie et al. (134). During the discovery phase, it is also important to consider differences in material preparation, for example, frozen tissue samples versus paraffin embedded tissues as discussed in Nowak et al. (135), and Mittempergher et al. (136). During the verification phase, these issues also may play a role; however, other concerns may arise such as the level of concordance in signal necessary between the discovery platform and the verification platform. Ultimately, after discovery and verification, a lockdown model is carried forth to validation. This, so called, lockdown model can be interpreted in a decision theoretic setting; each future sample must be classified or the outcome predicted based only on the model and a given sample signal from the intended technology.
In the validation phase, it is important to note that there is a major difference between answering a priori defined hypotheses and providing conclusions from exploratory analyses. Conclusions drawn from exploratory data analysis are descriptive results and typically need to be confirmed in a validation dataset, while a priori hypothesis lead to stronger conclusions and do not necessarily need an external validation. Care should be given in reporting unanticipated significant effects, as these are most likely due to chance and thus unlikely to be validated in other studies. Most importantly, researchers should keep in mind that, in the long term, the success of biomarker studies should be measured by the clinical improvement in patient outcomes.
There have been a prodigious number of biomarker studies reported in the literature; however, a surprising number of these results are irreproducible. The reasons for these discrepancies may lie in the differences in methodological procedures, inadequate control of false-positive findings, improper validation procedures, variability in the patient sampling, or any number of other differences in study design, conduct, or analytical procedures. Many published studies lack adequate information that would allow an evaluation of quality or comparability. In order to promote clear and complete reporting of biomarker studies, the REMARK guidelines have been developed (137). These guidelines make specific recommendations for preparing translational research presentations, reports, and publications with regard to describing patient and sample characteristics, assay methodology, study design, methods of data analysis, and results. While these recommendations have been distilled into bullets in Table 17.3, useful additional information can be found in the REMARK document (137).
REMARK Criteria: REporting Recommendations for Tumor MARKer Prognostic Studies |
Reporting Recommendations for Tumor Marker Prognostic Studies (REMARK)
Introduction
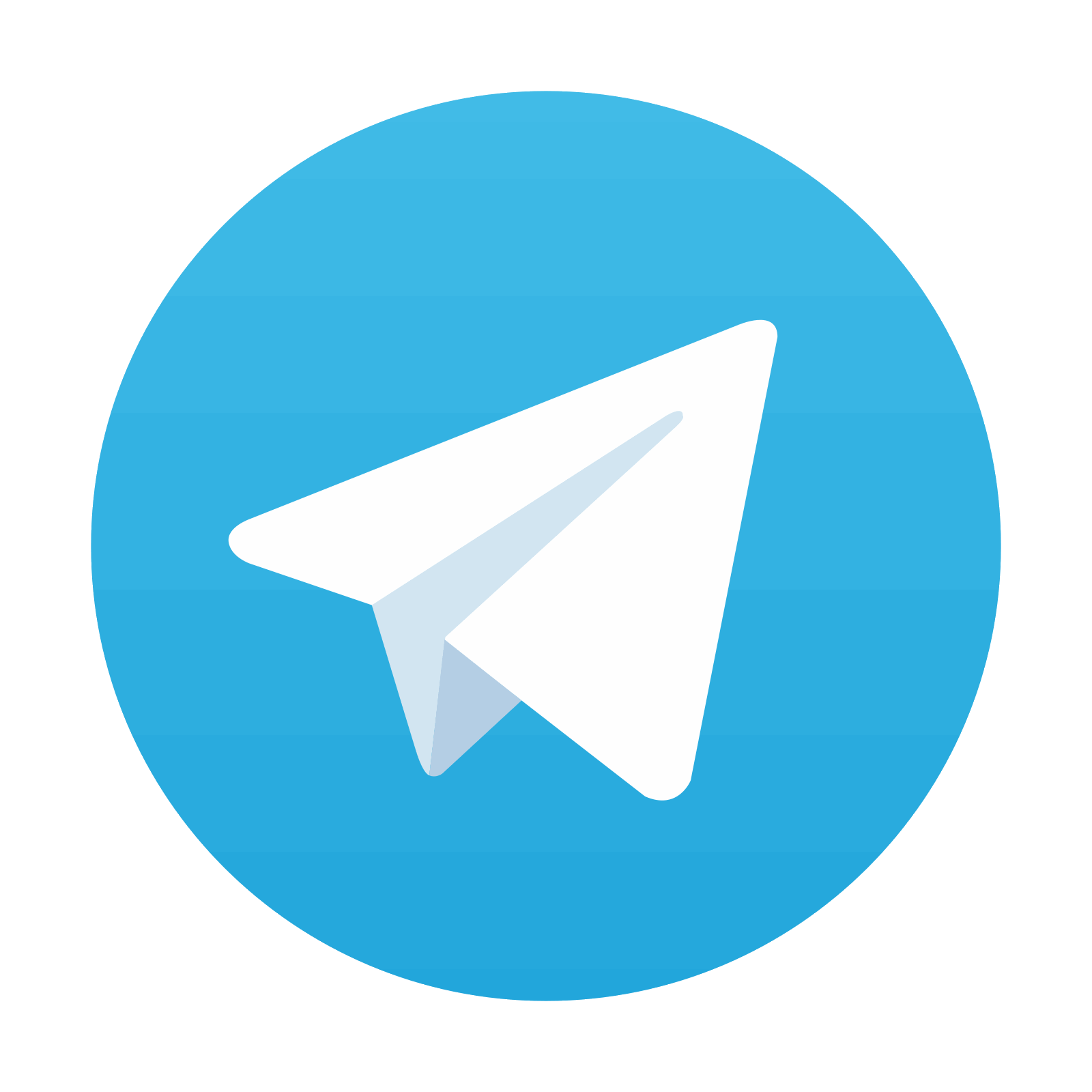
Stay updated, free articles. Join our Telegram channel

Full access? Get Clinical Tree
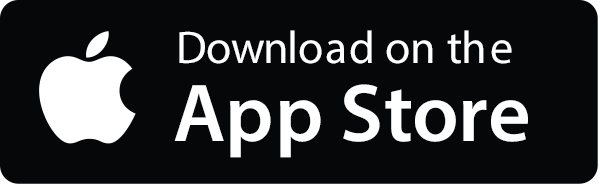
